Unlocking AI Product Success: Essential Metrics and Frameworks for Business Alignment
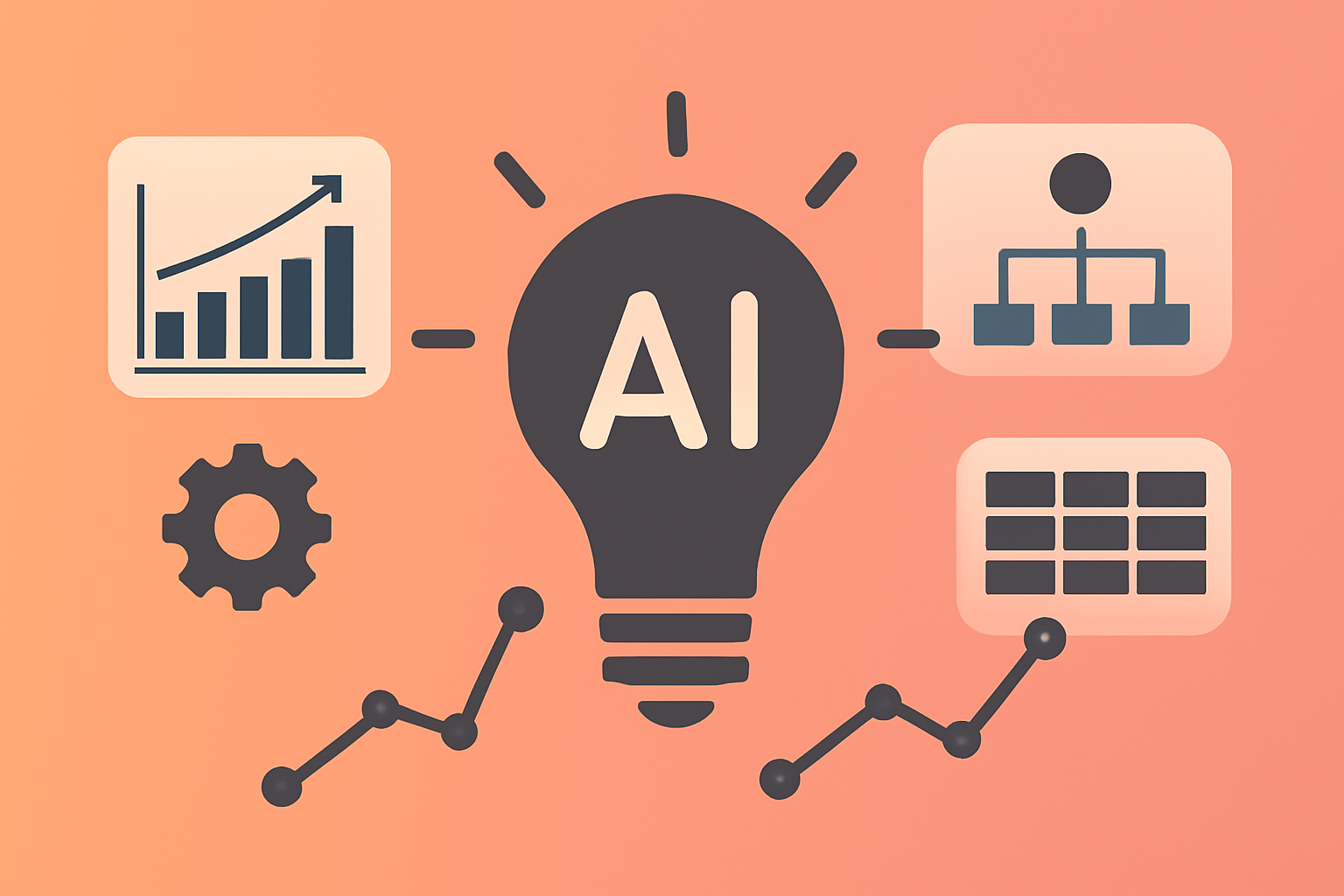
Artificial Intelligence (AI) is revolutionizing industries and reshaping the way businesses operate. Whether in the form of automated decision-making systems, machine learning models that enhance customer experiences, or intelligent virtual assistants, AI products are becoming integral to a wide range of services and solutions. As organizations continue to develop and deploy AI technologies, it is essential for them to ensure these products deliver tangible value and meet both user and business expectations. This is where the importance of key metrics and frameworks comes into play.
AI product development, unlike traditional software engineering, involves the integration of complex algorithms, data analysis, and iterative learning processes. As a result, defining success for AI products is not as straightforward as measuring traditional software metrics. To navigate this complexity, businesses must rely on a variety of metrics and frameworks that can evaluate the performance, scalability, and overall effectiveness of their AI products. These tools provide critical insights into whether an AI product is achieving its intended outcomes, delivering on customer expectations, and contributing to the strategic objectives of the organization.
In this post, we will explore the essential metrics and frameworks that organizations can use to measure the success of their AI products. We will examine the core metrics that provide a quantitative basis for evaluating AI performance, as well as the frameworks that guide the development and evaluation of AI projects. Additionally, we will address the challenges companies face in defining and measuring AI success, and highlight best practices for aligning AI product metrics with broader business goals.
By the end of this post, readers will have a clear understanding of how to effectively measure AI product success and how to implement the right metrics and frameworks to ensure continuous improvement and value generation. Whether you are a product manager, a data scientist, or a business executive, this guide will equip you with the tools needed to assess the effectiveness of AI products and drive successful AI outcomes in your organization.
Core Metrics for AI Product Success
When assessing the success of AI products, it is essential to utilize a combination of core metrics that offer both technical and business insights. These metrics help quantify the performance of AI models, track their operational efficiency, and evaluate how well they contribute to business objectives. Below are some of the most critical metrics to consider when evaluating the success of AI products.
Adoption Metrics: User Growth, Engagement, and Retention
One of the most fundamental indicators of AI product success is user adoption. Adoption metrics measure how well the product resonates with its intended audience, and they provide insights into the product’s reach and acceptance. For AI-driven solutions, user engagement and retention are key components that reflect long-term value.
- User Growth: This metric tracks the increase in the number of users interacting with the AI product over a set period. High user growth indicates that the product is gaining traction and attracting new customers or users. It can also provide insights into the effectiveness of marketing strategies and the overall market demand for the AI solution.
- Engagement: For AI products, engagement refers to how often users interact with the system and how much value they derive from it. Metrics like session frequency, time spent on the product, and the depth of interaction are all critical for understanding user behavior. Engagement metrics help ensure that the AI product isn’t just attracting users but also keeping them engaged and satisfied.
- Retention: Retention is one of the most powerful indicators of an AI product’s success. It measures the ability of the product to keep users over time, often analyzed through cohort analysis. High retention rates indicate that users find value in the product and are willing to continue using it. For AI products, retention can also be a sign that the system is evolving to meet user needs through continuous learning or updates.
Performance Metrics: Accuracy, Precision, Recall, F1 Score, and More
The performance of an AI product hinges on its ability to meet predefined objectives and deliver accurate results. A set of performance metrics is used to assess how well the AI models are functioning, both in terms of individual predictions and in the context of broader system goals.
- Accuracy: Accuracy is a straightforward metric that measures the proportion of correct predictions made by an AI model out of the total predictions. While it is an essential metric, it can sometimes be misleading in the case of imbalanced datasets where the model may favor the more frequent class. Thus, accuracy alone does not provide a comprehensive view of an AI system’s effectiveness.
- Precision and Recall: These metrics are particularly useful in classification tasks where the class distribution is imbalanced. Precision evaluates the ratio of true positive predictions to the total predicted positives, while recall assesses the ratio of true positive predictions to the total actual positives. A model that is high in both precision and recall is considered to be effective at correctly identifying relevant instances without missing important cases.
- F1 Score: The F1 score is the harmonic mean of precision and recall, balancing the two to provide a single value that reflects both aspects. It is particularly useful when both false positives and false negatives are costly, and you want to ensure that the model does not skew towards one type of error.
- AUC-ROC (Area Under the Curve - Receiver Operating Characteristic): AUC-ROC is a performance measurement for classification problems at various thresholds settings. It is useful for evaluating the performance of a model across all classification thresholds, giving a broader picture of its effectiveness than accuracy alone.
Operational Metrics: Latency, Throughput, and Scalability
Operational efficiency is another crucial factor in AI product success, particularly in real-time or high-throughput applications. These metrics assess the AI product’s capacity to handle large volumes of data and provide quick responses without compromising performance.
- Latency: Latency measures the delay between input and output within the AI system. In applications such as autonomous vehicles, real-time customer support, or financial trading systems, low latency is critical to ensuring timely decision-making and actions. High latency can result in a poor user experience, which undermines the product's value.
- Throughput: Throughput quantifies how many data points, tasks, or requests an AI system can process in a given period. For AI systems involved in high-volume tasks, such as fraud detection in banking or processing user interactions in chatbots, throughput is a key operational metric that ensures the system can scale effectively under increased demand.
- Scalability: Scalability refers to the ability of the AI system to handle increasing amounts of work or to be expanded to accommodate growth without sacrificing performance. A scalable AI product can process additional users or data without experiencing degradation in performance, making it essential for products that are expected to grow over time.
Business Metrics: ROI, Cost-Effectiveness, and Customer Satisfaction
While technical metrics are essential for evaluating AI product success, business metrics are equally important in ensuring that the product aligns with organizational goals and delivers measurable value. These metrics provide insight into the economic and customer-centric impact of AI solutions.
- Return on Investment (ROI): ROI measures the financial return generated by the AI product relative to its cost of development, deployment, and ongoing maintenance. Positive ROI indicates that the AI product is creating value for the business by increasing revenue, reducing costs, or improving operational efficiency. In the case of AI solutions aimed at automating processes, calculating ROI is particularly useful for demonstrating cost savings.
- Cost-Effectiveness: This metric evaluates the AI product’s performance relative to its cost. For instance, the cost of developing and deploying AI models may be substantial, but if the product can reduce operational costs or improve productivity, it can be considered cost-effective. This metric helps organizations understand whether their investment in AI is justified by the tangible benefits it delivers.
- Customer Satisfaction: Ultimately, the success of any product—AI or otherwise—can be measured by how satisfied the end users are. Customer satisfaction for AI products can be evaluated through user surveys, Net Promoter Scores (NPS), or direct feedback from clients. High customer satisfaction indicates that the AI product is meeting user expectations and providing a positive experience, which often correlates with higher retention and advocacy.
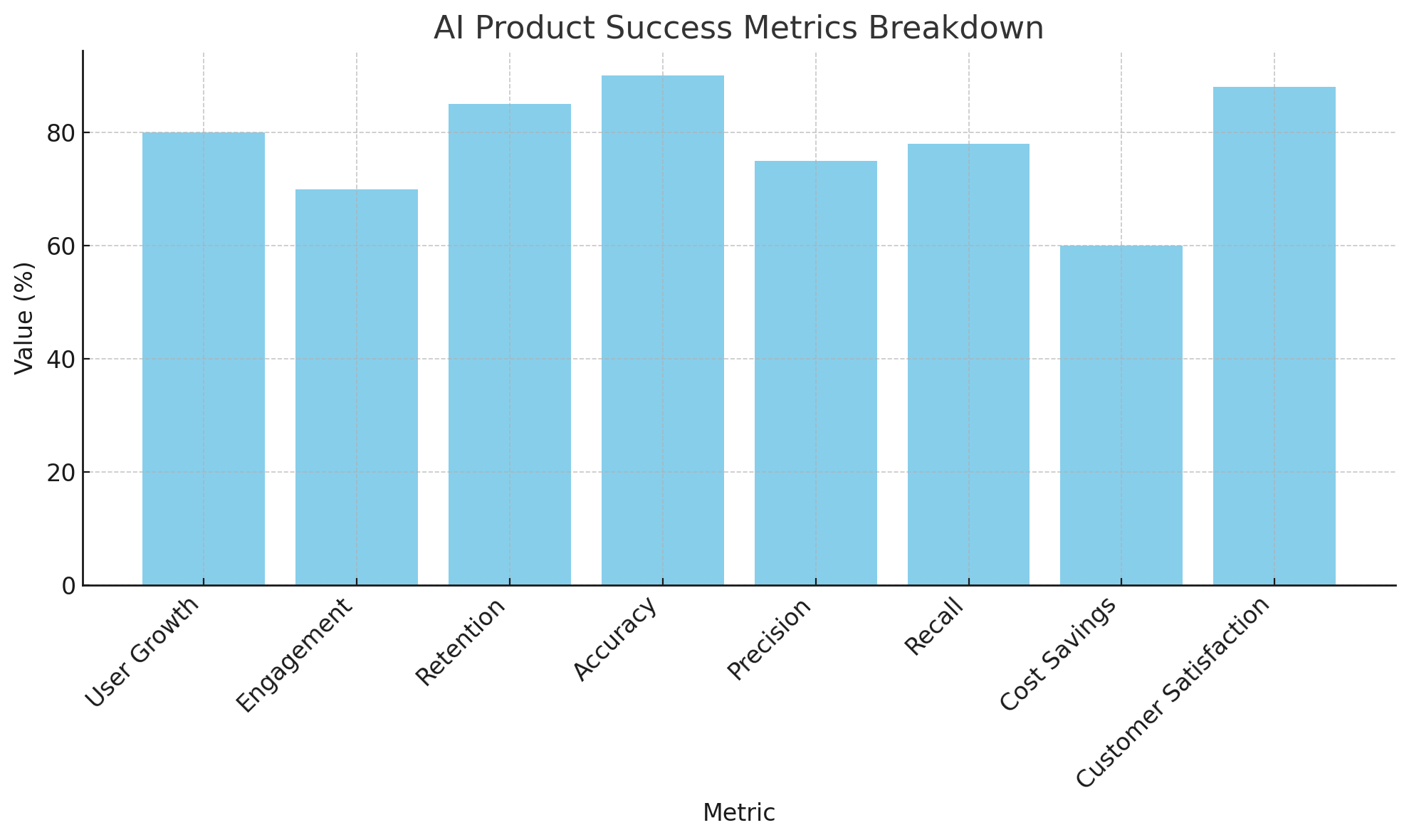
Conclusion
The key metrics for AI product success are multifaceted, involving both technical performance and business outcomes. Adoption metrics such as user growth, engagement, and retention provide insights into the product’s market fit and long-term viability. Performance metrics, including accuracy, precision, recall, and F1 score, help gauge the effectiveness of the AI model in fulfilling its purpose. Operational metrics like latency, throughput, and scalability are essential for ensuring the system can handle real-world demands efficiently. Finally, business metrics such as ROI, cost-effectiveness, and customer satisfaction highlight the broader value the AI product brings to the organization.
Key Frameworks for AI Product Evaluation
Evaluating the success of an AI product goes beyond tracking individual metrics. To ensure long-term effectiveness, AI products must be developed and assessed through structured frameworks that guide their design, iteration, and integration into broader organizational processes. These frameworks provide a systematic approach to understanding an AI product’s lifecycle, its alignment with business objectives, and its ability to evolve as new challenges arise. Below are some of the most influential frameworks used in AI product evaluation and development.
CRISP-DM: Cross-Industry Standard Process for Data Mining
The Cross-Industry Standard Process for Data Mining (CRISP-DM) is one of the most widely adopted frameworks for AI project development, particularly in the context of machine learning and data mining. It offers a structured approach for managing the entire lifecycle of an AI product, from data collection to model deployment and beyond. CRISP-DM is highly adaptable and can be applied to any industry or AI use case, making it invaluable for organizations seeking a comprehensive evaluation methodology.
- Business Understanding: The first step in CRISP-DM is to define the business objectives that the AI product aims to achieve. This involves aligning the AI solution with organizational goals and clarifying the problem to be solved. Establishing a clear business understanding is crucial for ensuring that the AI product delivers measurable value.
- Data Understanding: Data understanding involves gathering relevant datasets, cleaning them, and analyzing their quality. High-quality, well-curated data is essential for AI products to function effectively. This stage emphasizes the importance of data preprocessing and identifying any potential issues, such as bias or insufficient data, that could affect model performance.
- Data Preparation: After data collection and understanding, the next phase is preparing the data for modeling. This includes transforming data into a suitable format, handling missing values, and performing feature engineering. The goal is to ensure that the dataset is optimized for training machine learning algorithms, which directly impacts the AI product's performance.
- Modeling: In this phase, machine learning algorithms are applied to the prepared data to build predictive models. The process of model selection, tuning, and evaluation occurs here. This is also where the performance metrics, such as accuracy, precision, and recall, come into play, allowing teams to assess the model's efficacy.
- Evaluation: The evaluation phase involves assessing the model’s performance against predefined business objectives. This stage is critical for identifying areas of improvement and refining the model further to ensure it meets the organization's goals. It is also an opportunity to assess whether the AI product aligns with user needs and expectations.
- Deployment: After successful evaluation, the AI model is deployed into a production environment where it can begin to deliver value. Continuous monitoring during this stage is essential to ensure the AI product performs as expected under real-world conditions. Deployment also involves iterating on the model to incorporate new data and feedback from users.
CRISP-DM emphasizes an iterative approach to AI development, encouraging teams to revisit previous steps as new insights emerge. This cyclical process ensures that AI products remain aligned with business objectives, evolve with new data, and continually improve over time.
OODA Loop: Observe, Orient, Decide, Act
The OODA Loop is another influential framework used in decision-making processes, particularly in fast-paced environments where rapid iteration and agility are paramount. Originally developed by military strategist John Boyd, the OODA Loop is applicable to AI product evaluation in contexts that require continuous feedback and adaptation.
- Observe: The observation phase involves gathering data and understanding the current state of the AI product. This includes monitoring performance metrics, tracking user behavior, and collecting feedback from customers. In the context of AI products, observation is crucial for detecting potential issues, such as biases in predictions or operational inefficiencies.
- Orient: After gathering information, the next step is to analyze and interpret the data. This phase involves understanding the insights derived from the observation stage and determining how they relate to the AI product’s goals. In AI product evaluation, this is the time to assess whether the product’s performance is aligning with business objectives and user expectations.
- Decide: Once the relevant data has been analyzed, decisions must be made regarding how to proceed. This could involve adjusting the model, refining features, or enhancing the data pipeline. The decision phase is crucial in ensuring that the AI product is iteratively improved and fine-tuned to achieve optimal performance.
- Act: The final phase of the OODA Loop involves implementing the decisions made in the previous stage. For AI products, this could mean updating the model, launching new features, or making operational changes. Act ensures that decisions lead to tangible changes, and this phase feeds back into the observation stage, allowing the cycle to begin again.
The OODA Loop is particularly well-suited for AI products in dynamic environments, such as autonomous systems, real-time decision-making applications, or adaptive AI platforms. Its iterative, continuous feedback mechanism enables AI products to remain agile and responsive to changing conditions.
AI Maturity Model: Assessing the Evolution of AI Capabilities
The AI Maturity Model is a framework that evaluates an organization's progress in integrating AI into its operations. This model provides a structured approach to understanding where a company stands in its AI journey, from experimentation to full AI-driven transformation. It helps organizations assess their current AI capabilities, identify gaps, and establish a roadmap for future growth.
- Stage 1: Ad Hoc: In this early stage, AI initiatives are typically experimental and not integrated into the broader business strategy. Organizations may use AI in isolated use cases, but there is little coordination across departments. Evaluation at this stage focuses on pilot projects, experimentation, and initial assessments of AI's feasibility.
- Stage 2: Opportunistic: At this stage, AI is being applied to specific business problems, and organizations start to see the potential value of AI. However, AI projects are still largely decentralized, and there is limited collaboration between teams. Evaluating AI success at this stage involves measuring the performance of individual AI models and their impact on targeted business outcomes.
- Stage 3: Systematic: In the systematic stage, organizations begin to establish AI-driven processes and integrate AI into core business functions. AI projects are aligned with strategic goals, and the focus shifts to scaling AI solutions across the organization. Evaluation criteria now include not only technical performance but also the alignment of AI products with business objectives.
- Stage 4: Transformational: At the transformational stage, AI is deeply embedded into the organization's culture, operations, and decision-making processes. AI products are central to business strategy, and the organization continuously adapts to new technological advancements. Evaluating success at this stage involves assessing the broader impact of AI on the business, including innovation, competitive advantage, and long-term sustainability.
The AI Maturity Model helps organizations track their progression and determine the most effective strategies for advancing their AI capabilities. By evaluating AI success at each stage, organizations can prioritize resources and efforts to accelerate their AI journey and ensure continuous improvement.
Conclusion
The frameworks discussed—CRISP-DM, the OODA Loop, and the AI Maturity Model—offer valuable guidance for evaluating AI products at different stages of their development. CRISP-DM provides a structured approach to AI project life cycles, ensuring that AI products are aligned with business objectives and are built on high-quality data. The OODA Loop emphasizes agility and continuous feedback, making it ideal for AI products that require constant iteration and real-time decision-making. Finally, the AI Maturity Model helps organizations assess their progress and maturity in integrating AI into their operations, allowing them to set realistic goals for future growth.
Challenges in Defining Success for AI Products
Defining and measuring success for AI products is inherently more complex than traditional software products due to the dynamic nature of AI models, the reliance on large datasets, and the continuous evolution of machine learning algorithms. While the adoption of key metrics and frameworks can provide valuable insights, organizations often face numerous challenges when attempting to accurately define and evaluate AI product success. These challenges arise from technical, ethical, and business considerations, all of which must be addressed to ensure that AI products not only perform well but also meet broader organizational and societal goals.
The Complexity of AI Systems and Performance Metrics
One of the primary challenges in evaluating AI product success is the complexity of the systems themselves. AI models, particularly deep learning models, are often referred to as "black boxes" due to their intricate architectures and non-transparent decision-making processes. This complexity makes it difficult for organizations to fully understand how decisions are made by the AI system, which in turn complicates efforts to define success.
Performance metrics, such as accuracy, precision, and recall, can sometimes provide only a partial view of a model's success. In many cases, AI models are evaluated based on a single metric or a set of traditional metrics that fail to capture the full spectrum of the model's capabilities. For instance, high accuracy may not necessarily translate to business success if the AI model's predictions are biased or fail to align with user expectations. Similarly, models that perform well on one task may underperform in another, particularly when they are applied to real-world scenarios that differ from the training data.
To overcome this challenge, organizations must adopt a multi-metric approach, utilizing a combination of technical, operational, and business metrics. This holistic view ensures that AI products are evaluated from various perspectives, taking into account both short-term performance and long-term impact.
Data Quality and Bias in AI Models
Another significant challenge in defining AI success is the issue of data quality and bias. AI products rely heavily on data for training and decision-making. However, the data used to train AI models is often incomplete, noisy, or biased, which can significantly impact the model's performance and the accuracy of success evaluations. Bias in AI models is particularly concerning because it can lead to unfair or discriminatory outcomes, which can undermine the product's effectiveness and ethical standing.
Bias can arise at multiple stages in the AI development lifecycle, from data collection to model training and deployment. For example, if the training data is not representative of the target population, the resulting AI model may be biased toward certain groups, leading to skewed predictions. Similarly, the algorithms used in AI models can themselves introduce biases if they are not properly designed or tested.
To address these issues, organizations must prioritize data quality and fairness throughout the AI development process. This includes ensuring that data is diverse, representative, and free from systemic biases. Additionally, ethical frameworks and bias mitigation techniques should be incorporated into AI product evaluation to ensure that the models are not only effective but also fair and responsible. This can involve using fairness metrics, auditing AI systems for bias, and conducting thorough testing across different demographic groups to detect potential disparities.
Evolving Nature of AI Models and Continuous Learning
AI models are not static; they continuously evolve as they are exposed to new data and are refined through ongoing training processes. This dynamic nature of AI systems introduces a significant challenge in measuring long-term success. Unlike traditional software, which can be tested and evaluated based on a fixed set of features and performance metrics, AI models often change over time, which means their performance can fluctuate as well.
For instance, an AI model that initially performs well on a specific dataset may start to degrade in performance as it encounters new, unseen data or as the underlying data distribution changes. This is particularly true for models that are designed to continuously learn and adapt, such as those used in recommendation systems, autonomous vehicles, or predictive maintenance. The challenge, then, is to define success not only in terms of initial performance but also in terms of the model’s ability to adapt and remain effective over time.
To address this challenge, organizations must implement continuous monitoring and evaluation processes that track AI product performance throughout its lifecycle. This includes periodically reassessing performance metrics, conducting drift detection (to identify shifts in data distributions), and making necessary updates or adjustments to the model. It is also crucial to implement feedback loops that allow the AI product to learn from real-world data and improve its performance based on user interactions and new information.
Managing Ethical and Societal Impacts
The societal impact of AI products is an increasingly important consideration when evaluating their success. AI systems have the potential to affect a wide range of stakeholders, from end-users and employees to entire communities and industries. As such, defining success must go beyond traditional metrics of performance and business outcomes to include ethical considerations and social responsibility.
AI technologies, especially those used in sensitive applications such as healthcare, criminal justice, and hiring, have profound implications for fairness, privacy, and equity. The deployment of biased or harmful AI systems can result in significant societal harm, including reinforcing stereotypes, discriminating against marginalized groups, and violating individual rights. These concerns necessitate a broader understanding of AI product success that includes ethical principles such as fairness, transparency, accountability, and privacy protection.
Organizations must integrate ethical guidelines into their AI product evaluation processes to ensure that their AI products are developed and deployed responsibly. This includes conducting ethical impact assessments, involving diverse stakeholders in the development process, and establishing clear accountability mechanisms for AI decisions. Furthermore, businesses should work to foster transparency in AI systems by ensuring that users and other stakeholders understand how the AI models work and how their data is being used.
Balancing Innovation and Risk Management
In the pursuit of AI product success, organizations must strike a balance between innovation and risk management. AI products often push the boundaries of technology, introducing new capabilities and functionalities that have the potential to revolutionize industries. However, these innovations also introduce significant risks, including technological uncertainty, regulatory challenges, and public skepticism.
For example, AI technologies such as facial recognition and surveillance systems raise concerns about privacy and civil liberties, while AI-driven financial models may face regulatory scrutiny regarding fairness and transparency. As a result, organizations must carefully evaluate the potential risks and rewards of AI products and ensure that they are managing these risks in a responsible and transparent manner.
A successful AI product evaluation framework must consider both the potential benefits of innovation and the risks associated with new technologies. This involves assessing the regulatory landscape, staying informed about evolving industry standards, and ensuring that AI products comply with relevant laws and ethical guidelines.
Conclusion
Defining and measuring success for AI products presents a host of challenges, ranging from the complexity of AI systems and data quality issues to the evolving nature of AI models and the broader ethical implications. As AI continues to mature, organizations must adopt a comprehensive approach to evaluation that considers technical performance, business outcomes, ethical responsibility, and societal impact. By addressing these challenges head-on, organizations can ensure that their AI products not only succeed in achieving their intended goals but also contribute positively to users, businesses, and society at large.
Best Practices for Aligning AI Product Metrics with Business Goals
Aligning AI product metrics with overarching business goals is a critical step in ensuring that AI technologies provide tangible value to an organization. While technical performance metrics such as accuracy and precision are essential for assessing AI systems, they often do not directly correlate with the strategic objectives of a business. To maximize the value of AI products, organizations must develop a clear alignment between AI metrics and business outcomes. This alignment ensures that AI products not only perform well in technical terms but also drive profitability, innovation, and long-term growth. Below are key best practices for aligning AI product metrics with business goals.
Define Clear Business Objectives from the Outset
The first step in aligning AI product metrics with business goals is to clearly define the business objectives that the AI product is meant to achieve. Without a well-articulated understanding of what the organization hopes to accomplish, AI development can easily become a series of disjointed technical efforts with no direct business impact.
For example, if the goal of an AI-powered recommendation engine is to increase customer engagement, success metrics should reflect engagement-related outcomes such as click-through rates, time spent on the platform, or repeat purchases. If the AI product is intended to optimize operational efficiency, metrics like cost savings, process time reduction, and improved throughput will be more relevant. Clearly defining these objectives ensures that all subsequent development and evaluation efforts are directly linked to measurable business outcomes.
Establish Key Performance Indicators (KPIs) that Tie AI Performance to Business Impact
Once business objectives are established, the next step is to define Key Performance Indicators (KPIs) that directly connect AI performance to business impact. KPIs provide measurable benchmarks for evaluating whether an AI product is successfully contributing to the organization's strategic goals. These KPIs should be both specific and quantifiable, allowing teams to track progress over time.
For instance, if an organization’s goal is to improve customer satisfaction with an AI chatbot, relevant KPIs could include metrics such as customer satisfaction scores (CSAT), Net Promoter Score (NPS), and issue resolution time. Similarly, for AI models aimed at fraud detection, KPIs may involve the reduction in fraudulent transactions, the detection rate of suspicious activity, and the cost savings from fraud prevention.
Importantly, these KPIs must be aligned not only with technical outputs but also with broader business objectives such as revenue growth, cost reduction, and customer experience enhancement. Regularly monitoring and reporting on these KPIs ensures that the AI product is continuously evaluated against the goals it was designed to achieve, helping organizations make data-driven decisions about future iterations and enhancements.
Incorporate Cross-Functional Collaboration in the Development Process
AI product development should not be isolated within the data science or engineering teams; instead, it should involve cross-functional collaboration to ensure that business goals are represented throughout the product lifecycle. By engaging stakeholders from marketing, operations, finance, and other relevant departments early in the AI development process, organizations can ensure that the AI product meets diverse business needs and aligns with organizational priorities.
Cross-functional teams can help define the business problems the AI product is designed to solve, prioritize the metrics that matter most to the business, and provide ongoing feedback as the AI product evolves. For example, while data scientists may focus on model accuracy and precision, the marketing team can provide insights into how AI-driven customer segmentation can lead to improved targeting and conversion rates. Similarly, operations teams can offer valuable feedback on how an AI product can streamline processes or reduce costs.
By incorporating input from a wide range of departments, organizations can ensure that their AI product metrics reflect a holistic view of business objectives, enhancing the chances of successful product adoption and maximizing value creation.
Focus on Customer-Centric Metrics to Drive Value
AI products, whether they are customer-facing tools or internal solutions, must ultimately provide value to end users. Therefore, aligning AI product metrics with business goals requires a strong focus on customer-centric outcomes. Metrics such as user satisfaction, engagement, and retention should be prioritized to gauge the success of AI products in delivering a positive customer experience.
For example, if an AI-powered recommendation system is deployed on an e-commerce platform, the success of the product should not only be evaluated based on how accurate the recommendations are but also how much they contribute to customer loyalty and sales growth. Tracking metrics like customer lifetime value (CLV), repeat purchase rate, and customer satisfaction scores helps ensure that the AI product is meeting the needs and expectations of its users.
Customer-centric metrics also provide valuable insights for continuous improvement. Regularly measuring user feedback and engagement allows organizations to refine their AI models, update features, and adjust strategies to better meet customer demands, driving long-term success and business growth.
Foster Continuous Monitoring and Iteration to Maintain Alignment
The business environment is constantly evolving, and so too are user needs and expectations. As such, the alignment between AI product metrics and business goals must be maintained through continuous monitoring and iteration. AI products are rarely perfect from the outset; they must evolve based on real-world usage and emerging trends.
Organizations should establish a framework for regularly assessing AI product performance against business objectives and adjusting metrics as needed. For example, if a new competitor enters the market or shifts consumer behavior significantly, the AI product may need to adapt to stay competitive. This ongoing monitoring process ensures that AI products remain relevant and aligned with business goals over time.
In addition, AI products often require fine-tuning and updates based on user feedback and changing data patterns. By fostering an iterative development cycle, organizations can ensure that AI products continue to meet business goals and deliver value. This includes performing regular model evaluations, updating data pipelines, and incorporating insights from new customer feedback.
Align AI Product Roadmaps with Strategic Business Priorities
To ensure long-term success, AI product roadmaps should be aligned with the strategic priorities of the business. The roadmap should outline how the AI product will evolve over time, with clear milestones linked to business goals. These goals could include entering new markets, launching new features, or improving customer retention, and the roadmap should include timelines, resources, and metrics for achieving these targets.
For example, if an organization’s strategic priority is to improve sustainability, the AI product roadmap might include developing AI models that optimize energy use or reduce waste. If the focus is on customer acquisition, the roadmap may prioritize features that improve customer segmentation or personalization. By aligning the roadmap with business strategy, organizations ensure that the AI product evolves in a way that supports long-term objectives.
This approach also enables teams to prioritize resources effectively, making sure that the most important features and capabilities are developed first to meet business goals.
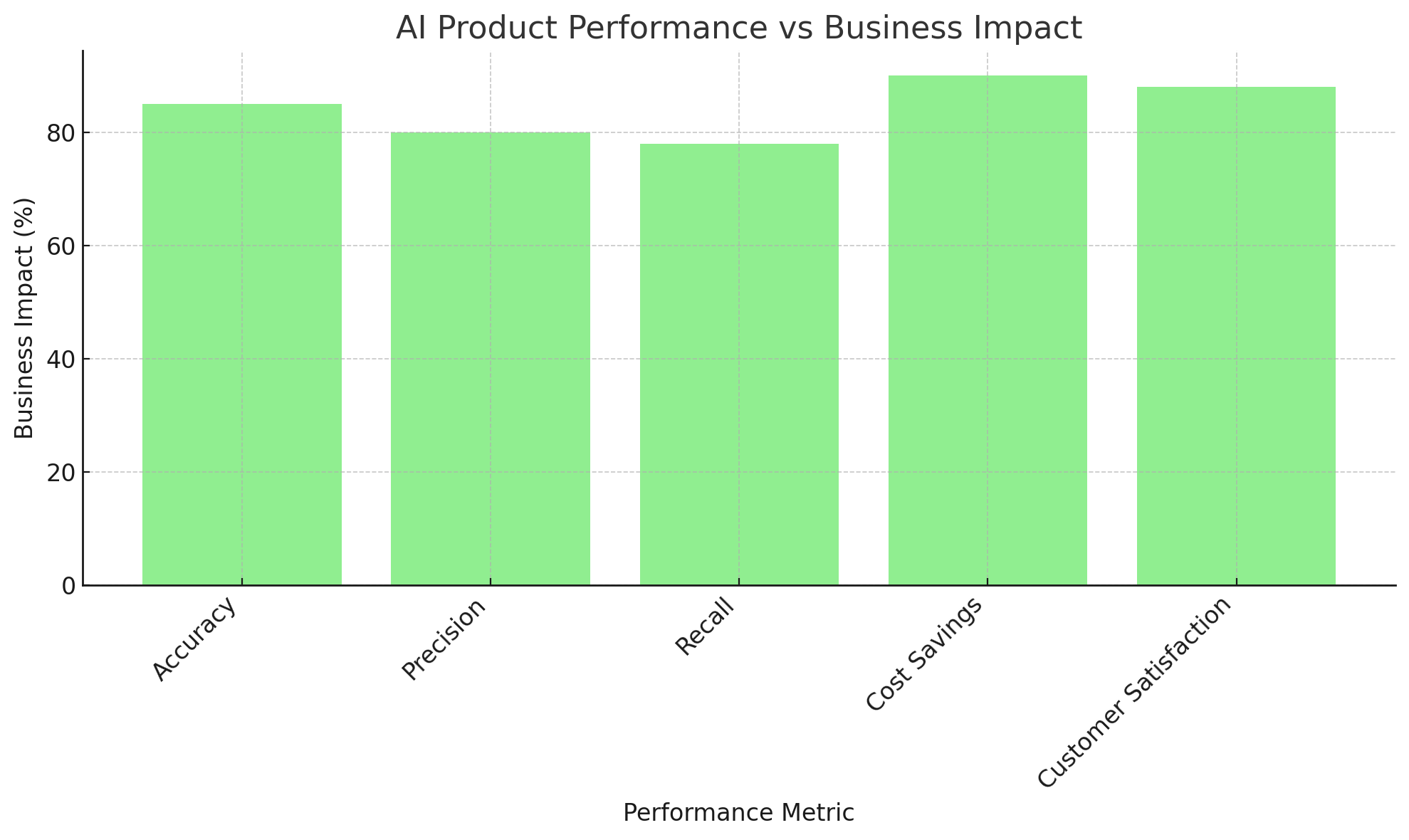
Conclusion
Aligning AI product metrics with business goals is essential for ensuring that AI solutions deliver measurable value to organizations. By defining clear business objectives, establishing relevant KPIs, fostering cross-functional collaboration, focusing on customer-centric outcomes, and promoting continuous iteration, organizations can create AI products that not only perform well technically but also drive long-term business success. AI products must be viewed as integral parts of broader organizational strategies, with their success evaluated through a combination of technical, operational, and business metrics. By embedding this alignment into the AI development process, organizations can maximize the impact of their AI initiatives and stay competitive in an increasingly AI-driven world.
Ensuring Long-Term Success in AI Product Development
In conclusion, defining and evaluating the success of AI products is a multifaceted process that requires careful consideration of various metrics and frameworks. While technical performance metrics like accuracy and precision are crucial, they must be complemented by business-focused KPIs, customer-centric outcomes, and an ethical framework that ensures fairness and societal responsibility. The integration of AI into a business strategy is not a one-time event but an ongoing process of refinement, iteration, and alignment with organizational goals.
Through the use of structured frameworks such as CRISP-DM, the OODA Loop, and the AI Maturity Model, businesses can guide the lifecycle of AI products from ideation to deployment, ensuring that they evolve to meet changing market demands and user expectations. These frameworks not only help assess the technical viability of AI products but also support continuous adaptation to maximize business value.
Moreover, aligning AI product metrics with business objectives is vital for demonstrating the tangible impact of AI investments. Organizations must define clear business goals, develop KPIs that directly link AI performance to these goals, and ensure that cross-functional teams collaborate throughout the AI development process. By focusing on customer satisfaction, operational efficiency, and innovation, organizations can ensure that AI products contribute to long-term growth and success.
However, the journey toward AI product success is not without its challenges. Issues such as data quality, model bias, and the dynamic nature of AI models require careful management to ensure that AI products are not only effective but also ethical and responsible. The constant evolution of AI technologies demands that organizations maintain flexibility and a commitment to continuous improvement through regular monitoring and iteration.
In the rapidly changing landscape of AI, the ability to align AI products with business goals will be a defining factor in organizational success. By leveraging best practices for metric development, ensuring continuous feedback loops, and fostering an environment of cross-disciplinary collaboration, businesses can unlock the full potential of AI products and achieve sustained success. As AI technologies continue to advance, the alignment of AI products with strategic business priorities will be key to maintaining competitive advantage and delivering value to users and stakeholders alike.
References
- Understanding AI in Business https://www.techradar.com/understanding-ai-business
- Key Metrics for AI Success in Enterprises https://www.forbes.com/key-metrics-ai-success
- How to Measure AI Performance https://www.dataversity.net/measure-ai-performance
- AI Maturity Models Explained https://www.simplilearn.com/ai-maturity-model-article
- Effective AI Product Metrics https://www.analyticsvidhya.com/ai-product-metrics
- Why AI Alignment with Business Goals is Crucial https://www.mckinsey.com/ai-alignment-business-goals
- Building AI Success Metrics https://www.cio.com/building-ai-success-metrics
- The Importance of Data Quality in AI Products https://www.datafloq.com/importance-of-data-quality-ai-products
- AI Frameworks to Accelerate Business https://www.businessinsider.com/ai-frameworks-accelerate-business
- Aligning AI Projects with Strategic Goals https://www.gartner.com/aligning-ai-projects-strategic-goals