Smart Surveillance: How AI Is Redefining Nuclear Reactor Monitoring and Safety
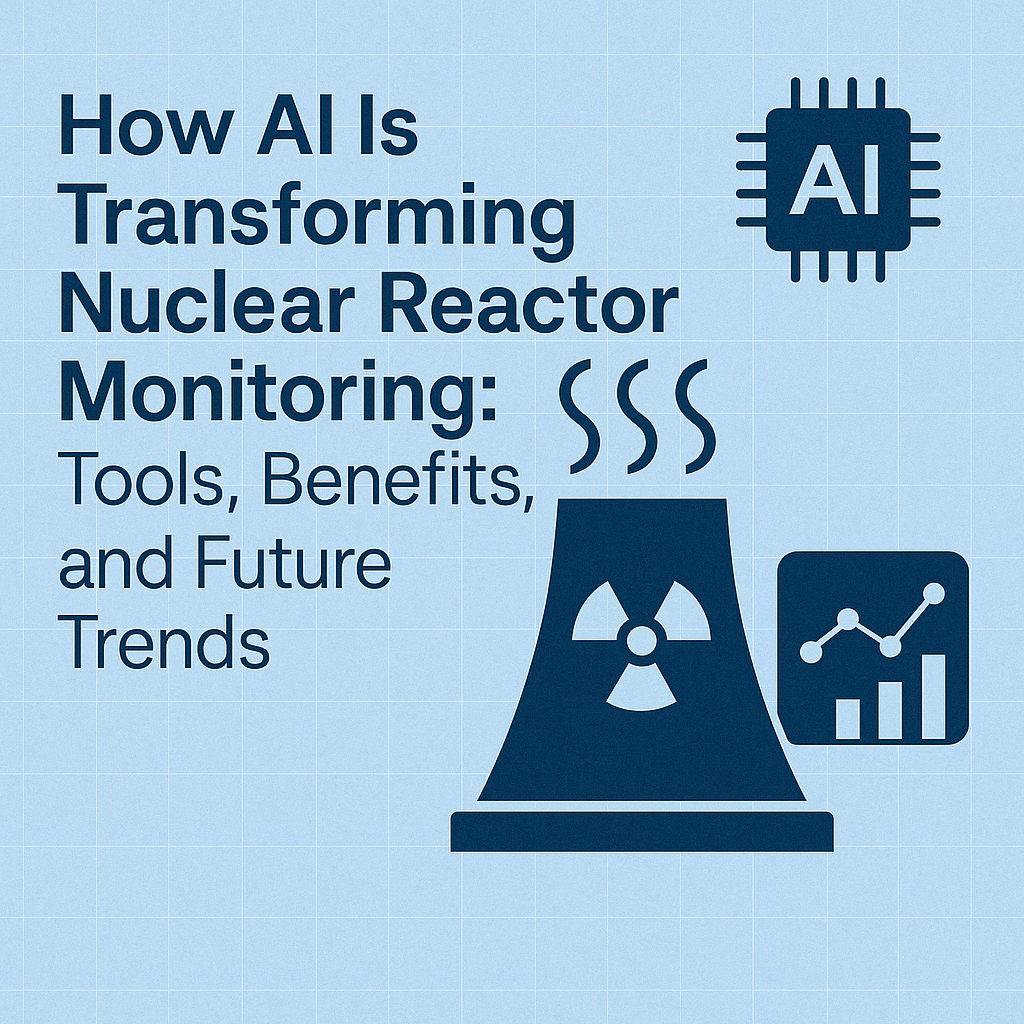
The global demand for reliable, low-carbon energy sources has positioned nuclear power as a vital component of the modern energy portfolio. With its ability to generate large amounts of electricity with minimal greenhouse gas emissions, nuclear energy stands as one of the most efficient alternatives to fossil fuels. However, the inherent risks associated with nuclear reactors—ranging from equipment malfunctions to catastrophic failures—necessitate rigorous safety protocols and continuous monitoring.
Historically, nuclear reactor monitoring has relied on a combination of analog sensors, supervisory control and data acquisition (SCADA) systems, and human oversight. While these traditional mechanisms have served the industry for decades, they are increasingly being challenged by the complexities of modern reactors and the growing expectations for zero-risk operation. Manual log reviews, delayed data processing, and limited predictive capabilities have exposed significant vulnerabilities, particularly in high-stakes environments where early detection and rapid response can prevent large-scale disasters.
Several nuclear incidents over the past half-century—most notably at Three Mile Island (1979), Chernobyl (1986), and Fukushima Daiichi (2011)—have underscored the consequences of inadequate reactor monitoring. In these cases, the inability to interpret complex signals quickly, recognize developing anomalies, or effectively communicate information contributed to tragic outcomes. While significant advancements in safety standards have been made since then, the nuclear industry remains under intense scrutiny from regulators, environmental advocates, and the public.
Against this backdrop, artificial intelligence (AI) has emerged as a transformative force capable of addressing many of the long-standing limitations in nuclear reactor monitoring. From anomaly detection to predictive maintenance and real-time data fusion, AI systems offer a level of speed, accuracy, and foresight that far exceeds the capabilities of legacy solutions. These technologies are particularly compelling in an era where operational efficiency must be balanced with uncompromising safety and where real-time decision-making is increasingly critical.
The integration of AI into nuclear safety frameworks is not merely aspirational—it is already underway. Research institutions, energy companies, and government agencies around the world are actively exploring and deploying AI tools to augment traditional monitoring systems. For example, deep learning models are being trained to identify subtle shifts in reactor performance that could signal future failures. Natural language processing (NLP) tools are being used to analyze thousands of pages of maintenance logs, extracting insights that would take human analysts weeks or months to uncover. Meanwhile, computer vision technologies are helping engineers detect visual signs of equipment wear or structural degradation that might otherwise go unnoticed.
AI does not replace the need for human judgment in the nuclear domain; rather, it enhances it. By reducing cognitive load, highlighting critical trends, and automating routine tasks, AI allows reactor operators and safety engineers to focus on strategic decision-making and high-risk scenarios. Importantly, the adoption of AI must be approached with caution. The black-box nature of some machine learning algorithms, the need for robust datasets, and the potential for cyber vulnerabilities all raise important questions about implementation, regulation, and oversight.
This blog post delves into the evolving landscape of AI-powered nuclear reactor monitoring. It begins by examining the limitations of traditional systems and the motivations for change. It then explores how AI technologies—such as machine learning, digital twins, and edge computing—are currently being applied to monitor, predict, and prevent reactor issues. The discussion includes a critical assessment of the challenges faced by AI adoption in this sensitive field, including regulatory barriers, ethical concerns, and technical constraints. Real-world case studies, comparative performance data, and a forward-looking analysis of future developments will also be presented.
Through this comprehensive exploration, the goal is to provide readers with a deeper understanding of how AI is not only revolutionizing nuclear reactor monitoring but also reshaping the broader conversation around safety, accountability, and innovation in the energy sector. As the world continues to confront climate change and energy insecurity, the ability to deploy intelligent, adaptive, and trustworthy monitoring systems will play a crucial role in the sustainable expansion of nuclear power.
The Traditional Approach to Nuclear Reactor Monitoring
For much of the nuclear age, reactor monitoring has relied on a robust yet rigid framework of analog instruments, centralized control systems, and manual protocols. These systems were designed with redundancy and reliability in mind, reflecting a time when computational resources were limited and human oversight was paramount. While effective in their era, traditional nuclear monitoring systems are increasingly strained by the demands of modern reactors, which operate with heightened complexity and under more stringent safety expectations.
Historical Foundations and Core Components
The foundational monitoring systems in most nuclear power plants are built around Supervisory Control and Data Acquisition (SCADA) frameworks. These centralized systems collect data from various sensors located throughout the plant—measuring variables such as temperature, pressure, radiation levels, and flow rates—and transmit this data to control rooms where operators can observe, interpret, and respond. Early SCADA systems relied on analog signals, which were later upgraded to digital formats, but many facilities still operate on hybrid infrastructures.
In addition to SCADA, other components such as Programmable Logic Controllers (PLCs), manual inspection protocols, and alarm-based signaling remain critical to traditional monitoring regimes. Data from these systems are typically archived in historical logs, used for retrospective analysis and compliance reporting. Despite incremental modernization over time, the operational essence of these systems has not significantly changed over the past several decades.
Limitations and Operational Challenges
One of the most significant drawbacks of traditional nuclear monitoring systems is their limited ability to predict potential failures before they occur. These systems excel at detecting threshold breaches—such as when temperature exceeds a predefined safety limit—but struggle to recognize gradual patterns or trends that could signal future risks. Without the analytical capabilities to assess multivariate dependencies, traditional systems often fail to provide early warnings, especially in complex fault scenarios where multiple indicators may evolve subtly and simultaneously.
Latency is another critical limitation. Traditional systems operate on batch processing cycles and human interpretation, which introduces delays in the detection-to-response timeline. In high-stakes environments such as nuclear power plants, even small delays can have cascading effects on reactor stability and safety. In several historical cases, including the Three Mile Island incident, delayed understanding of abnormal signals contributed to escalation rather than mitigation of the problem.
Furthermore, the reliance on human operators introduces both strengths and weaknesses. While experienced personnel bring intuition and situational awareness, they are also susceptible to cognitive overload, fatigue, and procedural drift. Manual log reviews, alarm filtering, and decision-making under stress are inherently error-prone processes. According to various safety audits and post-incident analyses, a significant proportion of monitoring failures in nuclear plants have been traced to human factors.
Regulatory and Compliance Constraints
The regulatory environment governing nuclear power adds an additional layer of complexity to monitoring practices. Agencies such as the U.S. Nuclear Regulatory Commission (NRC), the International Atomic Energy Agency (IAEA), and national safety boards impose strict guidelines on what constitutes acceptable monitoring, reporting, and response procedures. These guidelines are typically rooted in legacy technologies and conservative safety philosophies, which can inhibit innovation or delay the adoption of newer tools.
Traditional systems, being well understood and documented, tend to align easily with regulatory frameworks. However, this regulatory inertia can become a double-edged sword. While it promotes reliability and consistency, it also discourages the adoption of more adaptive, dynamic systems—such as AI-driven monitoring platforms—that may not yet have a long track record of safety performance or standardization.
Case Study: Fukushima Daiichi and Monitoring Gaps
The Fukushima Daiichi nuclear disaster in 2011 provides a somber illustration of the shortcomings inherent in traditional monitoring systems. Following a massive earthquake and tsunami, reactor systems were severely damaged, and crucial monitoring instruments were rendered inoperative due to power loss. The inability to access real-time core temperature data, combined with ambiguous sensor readings and communication breakdowns, severely hindered the response effort.
Subsequent investigations highlighted how more advanced analytical systems—such as predictive modeling and real-time simulation—could have provided better insight into the evolving conditions inside the reactors. In this context, the disaster served as a catalyst for reevaluating the technological tools available to nuclear operators and prompted renewed interest in intelligent monitoring systems.
The Growing Complexity of Reactor Architectures
Today's reactors—whether pressurized water reactors (PWRs), boiling water reactors (BWRs), or next-generation small modular reactors (SMRs)—operate with an unprecedented degree of interconnectivity and data richness. Sensor density has increased, component lifespans are longer, and operating environments are more dynamic. However, traditional monitoring tools have not scaled proportionately. This gap has created a situation in which vast amounts of sensor data are underutilized or ignored simply because traditional systems are not equipped to analyze them in real time.
Additionally, emerging reactor designs include passive safety systems and non-linear operational dynamics that cannot be easily modeled or interpreted using legacy tools.
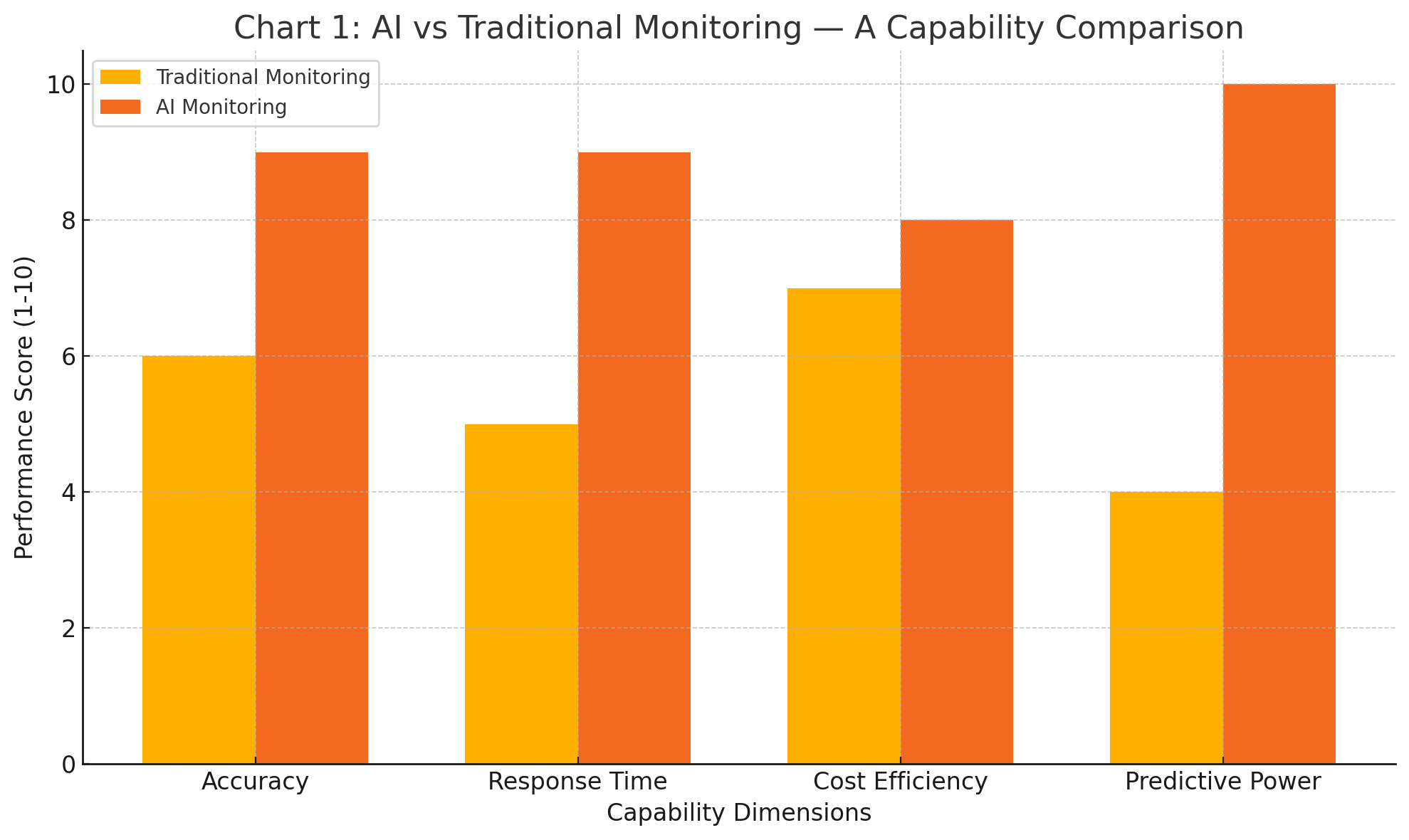
The growing complexity of these systems necessitates a new paradigm of monitoring—one that is adaptive, anticipatory, and capable of synthesizing disparate data streams into actionable insights.
Conclusion
While traditional nuclear reactor monitoring systems have served the industry well for decades, they are increasingly inadequate for meeting the demands of modern reactors and evolving safety standards. Limitations in predictive capabilities, latency, scalability, and human dependency expose vulnerabilities that can no longer be overlooked. The nuclear sector stands at a technological inflection point, where the risks of stagnation may outweigh the perceived safety of familiarity.
The Emergence of AI in Nuclear Safety and Monitoring
Artificial intelligence (AI) has begun to play a transformative role across many sectors of the global economy, from healthcare and manufacturing to finance and defense. In the context of nuclear energy, AI’s emergence is particularly significant due to the stringent safety requirements, complexity of reactor operations, and immense volume of operational data that must be monitored in real time. As nuclear reactors evolve in design and scale, traditional monitoring systems are increasingly inadequate. AI introduces a new era of intelligent automation, predictive insight, and enhanced decision-making that promises to redefine safety and efficiency in nuclear operations.
Drivers of AI Adoption in Nuclear Environments
The nuclear industry is inherently risk-averse, shaped by a history of high-profile accidents and subject to tight regulatory oversight. Nonetheless, several converging trends have accelerated the integration of AI technologies into reactor monitoring and safety frameworks.
First, the sheer volume and complexity of data generated by modern nuclear reactors—ranging from temperature and pressure readings to neutron flux and coolant flow—require computational methods beyond human capacity. AI excels at extracting patterns and anomalies from such large datasets in real time.
Second, the demand for predictive maintenance and reduced downtime in aging nuclear infrastructure has created an opportunity for machine learning algorithms to assess component health and anticipate failures before they occur. This shift from reactive to proactive maintenance is essential for extending reactor lifespans and maintaining safe operations.
Third, there is a growing international consensus around the role of AI in next-generation reactors, including small modular reactors (SMRs) and Generation IV systems, which emphasize modularity, passive safety, and digital control. AI technologies align naturally with these goals, providing autonomous capabilities and system-wide situational awareness.
Core Applications of AI in Reactor Monitoring
AI is currently being applied in several key domains of nuclear reactor monitoring. These include but are not limited to anomaly detection, predictive analytics, computer vision, natural language processing, and reinforcement learning for control system optimization.
Anomaly Detection and Pattern Recognition
AI systems, particularly those using deep learning and statistical modeling, are being deployed to identify patterns in reactor data that deviate from normal behavior. Unlike rule-based systems that rely on pre-programmed thresholds, AI models can learn from historical and real-time data to detect subtle, non-linear anomalies that may not trigger traditional alarms.
For instance, a deep learning model trained on years of reactor operation data may identify a rare vibration pattern in a coolant pump that correlates with future mechanical failure. Such insights enable early intervention and prevent escalation into critical failures.
Predictive Analytics and Maintenance Forecasting
One of the most valuable contributions of AI in the nuclear sector is its ability to predict equipment degradation before it becomes a threat to safety. Predictive analytics uses time-series data to estimate the remaining useful life of reactor components, such as valves, turbines, and heat exchangers.
This capability supports condition-based maintenance strategies, replacing the conventional approach of scheduled inspections that often lead to either premature replacement or undetected failure. The result is a significant improvement in cost efficiency and operational reliability.
Computer Vision for Remote Inspection and Surveillance
AI-powered computer vision is increasingly used to automate the visual inspection of nuclear infrastructure. This includes monitoring reactor cores, piping systems, cooling towers, and spent fuel storage facilities. Cameras and drones equipped with AI algorithms can detect corrosion, leaks, structural deformities, and other hazards without placing human workers in dangerous environments.
In high-radiation areas, where manual inspection is not feasible, robotic systems paired with computer vision provide continuous monitoring and instant alerts for visual anomalies. This improves both safety and accuracy in condition assessments.
Natural Language Processing for Document Analysis
Nuclear power plants generate vast amounts of unstructured textual data, including maintenance logs, inspection reports, safety audits, and regulatory filings. Natural language processing (NLP) enables AI systems to interpret and analyze this information, extracting relevant insights and detecting recurring themes or risks.
For example, NLP can identify patterns in incident reports that indicate systemic issues with a particular subsystem or vendor-supplied component. It can also support compliance efforts by cross-referencing operational data with regulatory standards, ensuring that safety documentation is both accurate and complete.
Reinforcement Learning and Control Optimization
Although still in its early stages of adoption in nuclear environments, reinforcement learning (RL) shows promise for optimizing reactor control systems. RL algorithms learn optimal actions through trial-and-error interactions with a simulated environment, making them well suited to optimizing complex, multi-variable control processes.
In simulation environments, RL agents can be trained to manage reactor startup, shutdown, and load adjustments with high precision, potentially reducing operator burden and minimizing the risk of human error. Future applications may include real-time optimization of fuel efficiency and thermal output in dynamic grid conditions.
Case Studies of AI Integration in Nuclear Monitoring
Several institutions and energy providers have initiated pilot programs or full-scale implementations of AI tools in nuclear settings. For instance, the Korea Hydro & Nuclear Power (KHNP) Corporation has developed AI systems for early fault detection in reactor coolant systems, using neural networks to analyze pressure and flow data in real time. Similarly, France’s EDF Group is exploring AI for predictive maintenance and digital twin modeling in its fleet of nuclear reactors.
In the United States, the Department of Energy’s Idaho National Laboratory (INL) has launched initiatives to integrate machine learning and AI into reactor control systems and cybersecurity. INL's work includes using AI to monitor reactor behavior during transient events and to detect potential cyber threats to digital instrumentation and control (I&C) systems.
These case studies demonstrate the growing confidence in AI’s ability to complement and enhance human oversight in high-consequence environments.
Benefits and Strategic Impact
The strategic benefits of AI integration into nuclear monitoring are multifold. They include:
- Enhanced safety through early anomaly detection and fault prevention.
- Increased efficiency by optimizing maintenance schedules and reducing downtime.
- Reduced human risk by minimizing the need for manual inspections in hazardous zones.
- Improved data utilization through real-time analysis and multi-source integration.
- Regulatory support via transparent, auditable decision processes driven by AI insights.
Moreover, AI’s adaptability ensures that it can evolve alongside reactor technology, offering a future-proof layer of intelligence in a domain where operational continuity over decades is common.
Conclusion
The emergence of AI in nuclear reactor monitoring marks a decisive evolution from static, rule-based oversight to dynamic, data-driven safety management. By enhancing predictive capabilities, streamlining inspections, and enabling real-time response to complex operational scenarios, AI is positioning itself as an indispensable asset in the modern nuclear toolkit.
While challenges related to implementation, trust, and governance remain, the momentum behind AI adoption is accelerating. Governments, research bodies, and private operators are recognizing that the future of nuclear safety and monitoring will be shaped not just by engineering excellence but by intelligent systems capable of learning, adapting, and anticipating the unpredictable.
Core AI Technologies Transforming Reactor Monitoring
The advent of artificial intelligence in the nuclear sector signifies a paradigm shift in how reactors are monitored, maintained, and optimized for safety. Unlike traditional systems, which rely on static rules and threshold alerts, AI technologies bring a dynamic and adaptive intelligence layer capable of contextual understanding, pattern recognition, and predictive reasoning. As the operational complexity of nuclear reactors increases—especially with the rise of advanced reactor designs—AI’s role becomes not only valuable but indispensable.
This section explores the core AI technologies currently reshaping reactor monitoring, with particular emphasis on their real-time applications, technical foundations, and transformative impact on nuclear safety practices.
Machine Learning and Predictive Maintenance
At the heart of AI deployment in reactor monitoring lies machine learning (ML)—a suite of algorithms capable of learning patterns from data and making forecasts based on those insights. Predictive maintenance is one of the most impactful applications of ML in nuclear settings. Instead of relying on fixed inspection intervals or reacting to component failures after they occur, predictive maintenance models analyze historical and real-time data to estimate the remaining useful life of key components.
For instance, machine learning algorithms can analyze vibration signatures, temperature deviations, pressure trends, and acoustic emissions to detect early signs of wear in turbines, pumps, or valves. These systems continuously adjust their forecasts based on new incoming data, offering reactor operators timely alerts for proactive servicing. The result is a significant reduction in unscheduled downtime, lower maintenance costs, and enhanced operational safety.
Moreover, ML models can be trained using supervised, unsupervised, or reinforcement learning frameworks. Supervised learning is particularly useful for classification tasks, such as identifying whether a reactor subsystem is operating within normal parameters. Unsupervised learning helps detect anomalies without prior labeling, and reinforcement learning—though still in experimental stages—offers promise in adaptive control optimization and policy learning.
Digital Twins and Real-Time Simulation
A transformative AI-enabled concept gaining traction in the nuclear sector is the digital twin—a real-time, virtual replica of a physical system that mirrors its performance, status, and health. In the context of reactor monitoring, digital twins use live sensor data to simulate core functions such as coolant circulation, neutron flux distribution, and heat exchanger performance.
Digital twins not only allow for scenario-based forecasting—such as simulating how a small coolant leak might escalate under different conditions—but also serve as sandboxes for training operators and testing emergency protocols without real-world risk. They integrate with AI-driven optimization algorithms to provide what-if analyses that inform safety decisions and regulatory reporting.
These models rely heavily on deep learning and physics-informed neural networks (PINNs), which blend data-driven learning with physical laws governing nuclear processes. The convergence of AI and physics ensures both high-fidelity simulation and robust interpretability, a critical factor in high-risk environments.
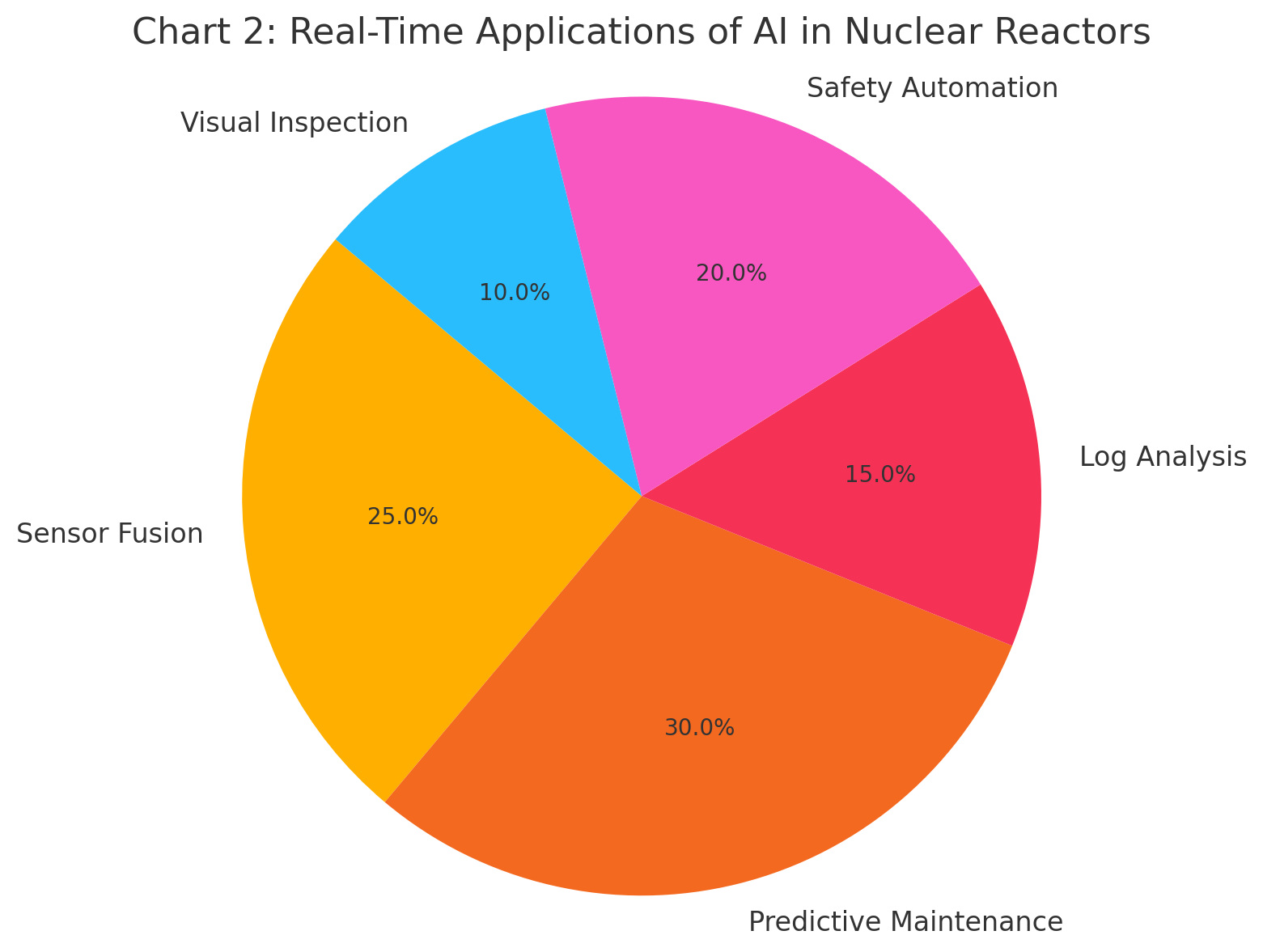
Edge AI and On-Site Intelligence
As nuclear facilities increasingly require ultra-low-latency decision-making, edge AI has emerged as a compelling solution. Edge AI involves deploying machine learning models on localized hardware—such as microcontrollers or edge servers—within the reactor facility itself. This approach minimizes reliance on external networks or centralized servers, thereby reducing latency, enhancing reliability, and improving cybersecurity.
Edge devices are particularly useful in monitoring high-risk systems where milliseconds matter. For example, AI models embedded in control rods or coolant pumps can instantly detect deviations and issue corrective commands without requiring input from centralized systems. This distributed intelligence architecture enhances both responsiveness and fault tolerance.
Another benefit of edge AI is data sovereignty. In a sector where confidentiality and national security are paramount, retaining sensitive sensor data within the facility—while still benefiting from AI analysis—addresses many compliance and governance concerns.
Computer Vision for Visual Inspection
Routine inspections of reactor components such as piping systems, containment structures, and heat exchangers are labor-intensive, high-risk, and prone to human error. Computer vision, powered by convolutional neural networks (CNNs), offers a safer and more efficient alternative by enabling AI to "see" and interpret images or video streams.
Computer vision models are trained to detect corrosion, surface cracks, leakages, and mechanical distortions from high-resolution imagery captured by cameras or drones. In one notable deployment, robotic crawlers equipped with AI vision systems were used to inspect reactor cooling pipes in a confined area that would be hazardous for human workers.
The application of computer vision extends beyond structural integrity. It also enables real-time surveillance of restricted zones, detection of unauthorized access, and verification of operator compliance with safety protocols. Integrating visual data with sensor readings enhances situational awareness and operational transparency.
Natural Language Processing (NLP) and Log Analysis
An often-overlooked aspect of nuclear monitoring is the vast repository of unstructured text data generated in the form of maintenance logs, incident reports, inspection records, and operator notes. Traditionally, this wealth of information has been underutilized due to the manual effort required for analysis. Natural language processing (NLP), however, is changing that paradigm.
NLP models can automatically extract entities, detect sentiment, identify causal chains, and highlight anomalies within thousands of documents in minutes. For example, an NLP engine might flag recurring issues across separate logs that suggest a systemic problem with a heat exchanger model. It can also summarize long records into actionable insights, enabling decision-makers to rapidly assess operational history or regulatory compliance.
Recent advancements in large language models (LLMs) further enhance NLP capabilities. These models can understand domain-specific jargon, infer complex relationships between entities, and even suggest remedial actions based on past precedent. As a result, NLP not only accelerates information retrieval but also contributes directly to safety planning and risk mitigation.
Integration and Holistic Monitoring Systems
While each of these AI technologies offers standalone benefits, their combined integration into holistic monitoring platforms is where the most profound impact is realized. Such platforms consolidate data from sensors, cameras, logs, and simulations into unified dashboards with advanced visualization, automated alerts, and decision-support modules.
This comprehensive approach fosters a feedback loop where insights from one subsystem (e.g., computer vision) inform actions in another (e.g., predictive maintenance), resulting in a synergistic system that continually learns, adapts, and optimizes itself. These integrated platforms are currently being piloted in several next-generation nuclear facilities across Europe, Asia, and North America.
Conclusion
AI technologies are rapidly redefining the landscape of nuclear reactor monitoring. Machine learning enables predictive maintenance, reducing failures and costs. Digital twins provide real-time simulations for informed decision-making. Edge AI facilitates instant, on-site analytics with minimal latency. Computer vision streamlines inspections and enhances safety compliance. NLP unlocks the power of unstructured text for systemic insight.
Together, these technologies represent a shift from reactive monitoring to proactive, intelligent oversight. As adoption accelerates, the nuclear sector is poised to achieve higher levels of operational efficiency, safety assurance, and regulatory transparency than ever before.
Implementation Challenges and Risk Considerations
The integration of artificial intelligence into nuclear reactor monitoring systems presents a compelling opportunity to enhance safety, efficiency, and operational insight. However, deploying AI in such a high-stakes environment is not without its complexities. The transition from traditional to AI-enhanced monitoring introduces several challenges that must be carefully managed to ensure reliability, transparency, and regulatory compliance. These challenges span technical, organizational, regulatory, and ethical dimensions and must be addressed holistically to realize the full benefits of AI in the nuclear sector.
1. Data Quality, Availability, and Standardization
AI systems thrive on high-quality, comprehensive datasets for training and operation. In nuclear reactor environments, however, acquiring such datasets can be a formidable task. Many nuclear facilities operate under strict confidentiality due to national security concerns, making it difficult to share operational data across institutions or with third-party AI developers.
Moreover, legacy systems often store data in inconsistent formats or silos, lacking the interoperability needed for effective AI integration. In some cases, sensor data may be incomplete, imprecise, or subject to signal noise, limiting the accuracy of AI models trained on these inputs. Historical records—particularly for older reactors—may be sparse or contain errors that hinder model development.
To address these limitations, significant investment in data infrastructure is required, including digitization of analog records, harmonization of data formats, and the deployment of high-fidelity sensors. Additionally, collaborative frameworks between reactor operators, regulators, and AI researchers can help create anonymized data repositories that support safer and more accurate model training.
2. Algorithmic Transparency and Explainability
In the context of nuclear safety, decisions made by AI systems must be explainable and interpretable by human operators and regulators. However, many advanced machine learning techniques—especially those based on deep learning—are often considered “black boxes,” meaning their internal decision processes are not easily understood.
This lack of transparency poses a significant challenge in safety-critical applications. Operators need to trust and verify the reasoning behind AI-driven alerts or recommendations, especially in emergency scenarios where split-second decisions may impact reactor integrity or public safety. Moreover, regulators require comprehensive documentation and validation to certify any automated system used within a nuclear plant.
Explainable AI (XAI) is an emerging field aimed at addressing this issue by providing tools and methods to make AI decisions interpretable. Techniques such as feature attribution, surrogate models, and visual diagnostics can help elucidate how an AI system arrived at a particular conclusion. Incorporating these methods into AI frameworks for nuclear applications is essential for achieving operational transparency and regulatory acceptance.
3. Cybersecurity Risks
As AI systems become increasingly integrated into nuclear infrastructure—often connected to cloud services, edge devices, and remote monitoring platforms—they expand the cyber-attack surface of these facilities. Malicious actors targeting AI-based control systems could potentially alter sensor inputs, manipulate model outputs, or exploit software vulnerabilities to disrupt reactor operations.
The deployment of AI must therefore be accompanied by robust cybersecurity protocols, including network segmentation, end-to-end encryption, real-time anomaly detection, and fail-safe redundancies. AI models themselves should be tested against adversarial inputs—intentionally crafted data designed to mislead or corrupt predictions—to assess their resilience under hostile conditions.
Cybersecurity in the AI era also requires coordination between multiple stakeholders, including reactor operators, government agencies, AI vendors, and national security entities. A comprehensive threat modeling approach must account for both conventional IT risks and novel threats specific to AI systems.
4. Regulatory and Compliance Barriers
Nuclear power is one of the most heavily regulated industries in the world, with safety standards enforced by national and international agencies such as the U.S. Nuclear Regulatory Commission (NRC), the International Atomic Energy Agency (IAEA), and the European Nuclear Safety Regulators Group (ENSREG). While these bodies have well-established frameworks for evaluating traditional systems, the rapid evolution of AI presents regulatory challenges.
Current certification processes are often ill-suited for assessing adaptive systems that learn and evolve over time. Regulators may require static documentation and deterministic behavior, which are difficult to provide with AI systems that operate probabilistically or incorporate continuous learning mechanisms.
To bridge this gap, new regulatory paradigms are needed. These may include dynamic certification models, performance-based testing, and sandbox environments where AI systems can be evaluated under simulated conditions. Close collaboration between developers, operators, and regulators will be critical to ensure that safety and innovation move forward in tandem.
5. Workforce Training and Cultural Resistance
The successful implementation of AI in nuclear monitoring also depends on human factors, including the preparedness and willingness of the existing workforce to engage with new technologies. Nuclear engineers and plant operators may be skeptical of AI systems, particularly if they perceive them as opaque, unreliable, or threatening to their professional autonomy.
Moreover, many employees in the nuclear sector have been trained to operate within highly procedural, deterministic frameworks. Introducing probabilistic tools that generate dynamic outputs requires a significant shift in mindset, as well as new competencies in data science, model interpretation, and digital system oversight.
Comprehensive training programs, participatory design processes, and transparent communication about AI capabilities and limitations can help ease the cultural transition. Importantly, AI should be framed not as a replacement for human expertise but as a tool to augment decision-making, reduce cognitive load, and enhance operational safety.
6. Ethical Considerations and Accountability
AI deployment in nuclear monitoring raises broader ethical questions related to accountability, bias, and unintended consequences. For example, if an AI system fails to detect a critical anomaly, who bears responsibility—the model developer, the plant operator, or the regulator who approved the system?
Furthermore, bias in training data—such as an overrepresentation of certain reactor types or operational scenarios—could lead to skewed model performance and unequal safety outcomes across different facilities. Ethical frameworks must be established to guide the responsible development, deployment, and oversight of AI in nuclear settings.
These frameworks should include principles of fairness, transparency, accountability, and human-in-the-loop decision-making. By embedding ethics into the AI lifecycle, stakeholders can build public trust and ensure that safety remains the paramount objective.
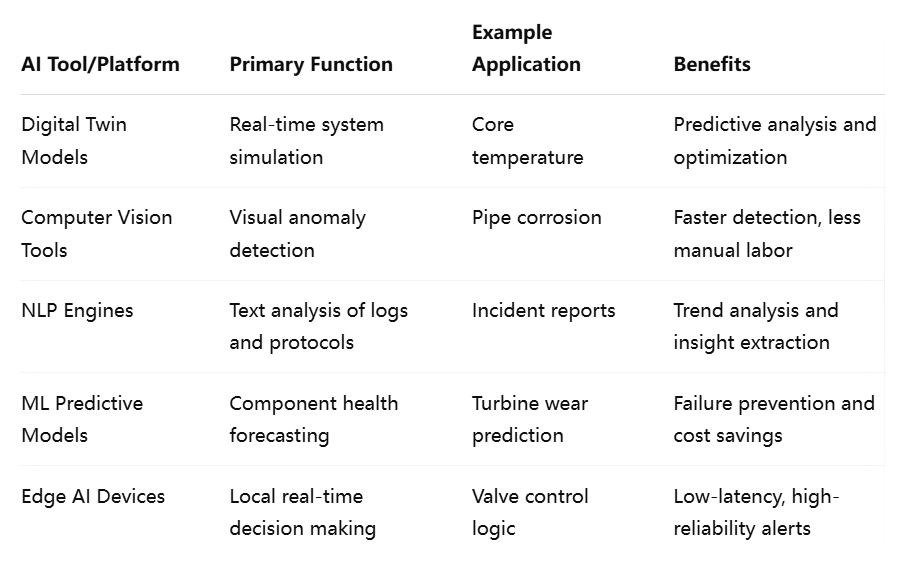
Conclusion
While the integration of AI into nuclear reactor monitoring offers profound advantages, it must be approached with caution, discipline, and strategic foresight. The challenges—ranging from data quality and algorithm transparency to cybersecurity and regulatory compliance—are significant but not insurmountable. With collaborative governance, ongoing technical refinement, and a strong commitment to ethical principles, the nuclear industry can leverage AI not only to improve safety but also to pave the way for a smarter, more resilient energy future.
The Future of AI in Nuclear Safety
The integration of artificial intelligence into nuclear monitoring systems is not merely a transient technological trend—it represents a structural transformation of how nuclear energy facilities will be designed, operated, and regulated in the coming decades. As AI technologies continue to evolve in sophistication and accessibility, their role in nuclear safety is expected to expand dramatically. This final section explores the likely trajectory of AI in the nuclear sector, examining its role in next-generation reactor designs, potential for full autonomy, international collaboration, and the implications for governance and human oversight.
1. AI and the Next Generation of Reactor Architectures
The future of nuclear energy lies increasingly in advanced reactors such as Small Modular Reactors (SMRs) and Generation IV designs. These systems are being developed with the express intent of improving safety, scalability, and cost-efficiency while minimizing environmental impact and nuclear waste. Many of these reactors are conceived as inherently safer and more digitally integrated, making them well-suited for AI-driven monitoring systems.
AI will likely be embedded in the core design of such facilities from the outset, rather than retrofitted as is the case with older installations. This allows for seamless integration of smart sensors, real-time control algorithms, and adaptive analytics that can learn from operational history and environmental data. In SMRs, which may operate in remote or unmanned locations, AI could enable semi-autonomous or fully autonomous monitoring and control, supported by cloud-based diagnostics and predictive maintenance platforms.
The modularity and repeatability of SMRs also support scalable AI training, where data from one reactor can be used to refine models deployed across multiple units. This type of federated learning can accelerate model maturity and safety validation, while minimizing risks associated with data siloing.
2. Toward Autonomous Monitoring and Control
While current AI applications in nuclear plants are primarily decision-support tools, there is growing interest in the concept of autonomous reactor operation, where AI systems not only monitor but also make real-time control decisions without direct human intervention. Such autonomy could significantly improve response times in emergency situations, optimize fuel usage, and dynamically balance power output in response to grid demand.
However, the path to autonomy is fraught with technical and ethical challenges. Fully autonomous systems must demonstrate an exceptionally high degree of reliability, explainability, and resilience. Fail-safes must be rigorously designed to ensure that AI systems revert control to human operators under abnormal conditions or system failures.
Autonomy will likely emerge in phases, beginning with automated subsystems such as coolant loop optimization, radiation leakage detection, and secondary system management. Over time, as trust in AI performance grows and regulatory frameworks adapt, more critical control functions may be entrusted to AI, potentially culminating in autonomous microreactors designed for off-grid or emergency deployment.
3. Digital Twins as Central Nervous Systems
Looking ahead, the use of digital twins—already in deployment in some forward-thinking facilities—is expected to become the standard for all nuclear installations. These AI-driven virtual replicas will serve as the central nervous system of plant operations, enabling continuous simulation of reactor dynamics, scenario testing, and anomaly detection in real time.
The convergence of digital twins with edge computing and AI-enhanced robotics could give rise to cognitive nuclear infrastructure—intelligent systems capable of sensing, reasoning, and acting based on real-time environmental inputs. These systems could facilitate unprecedented levels of situational awareness, automate regulatory reporting, and continuously refine operational models to match physical conditions.
In addition, the integration of natural language interfaces with digital twins may allow operators to query the virtual model in real time using conversational AI, thereby improving accessibility and democratizing complex insights across teams.
4. Global Collaboration and Policy Harmonization
The future of AI in nuclear safety will also be shaped by the extent to which international cooperation can be achieved. As nations deploy different reactor designs and AI platforms, the risk of fragmentation in safety standards, data interoperability, and regulatory interpretations increases. To address this, global nuclear bodies such as the International Atomic Energy Agency (IAEA) are beginning to engage with AI-related initiatives, seeking to develop frameworks that promote consistency, safety, and trust.
Cross-border collaboration will be essential in developing shared benchmarks, data models, and validation protocols. This is especially important for ensuring that AI systems behave reliably under a variety of reactor types, environmental conditions, and stress scenarios. Multi-country simulation datasets and joint safety drills involving AI decision engines may become standard practice.
Additionally, international governance must address dual-use risks, where AI technologies developed for civilian reactors could be repurposed for military applications. Clear norms and oversight mechanisms will be required to ensure AI is deployed exclusively for peaceful and safe nuclear operations.
5. The Role of Human Oversight in an AI-Enhanced Future
Despite advances in automation, human oversight will remain an essential pillar of nuclear safety for the foreseeable future. The future is not one in which human operators are eliminated, but rather one in which their roles evolve. As AI systems assume more routine monitoring and optimization tasks, human expertise will increasingly focus on strategic oversight, exception handling, and ethical governance.
The integration of AI into nuclear operations should thus be guided by a "human-in-the-loop" philosophy—one that ensures human operators can intervene, audit, and override AI decisions when necessary. This includes developing intuitive user interfaces, integrating explainable AI techniques, and promoting transparency in model decision-making.
Training and professional development will also need to evolve. The nuclear workforce of the future will include data-literate engineers, AI ethics specialists, and cross-disciplinary system analysts, all working together to steward the safe deployment of AI.
6. Ethical Foresight and Responsible Innovation
As AI becomes more deeply embedded in nuclear infrastructure, the importance of ethical foresight cannot be overstated. Emerging technologies bring unintended consequences, and the risks of system bias, model drift, and unforeseen failure modes must be proactively managed. Ethics-by-design principles—such as accountability, fairness, transparency, and resilience—should be codified into all stages of AI system development, from initial modeling to operational deployment.
Public trust will also play a critical role. AI in nuclear power may raise concerns among stakeholders who are wary of entrusting machines with responsibilities in such a high-risk domain. Transparency in deployment, robust risk communication strategies, and meaningful stakeholder engagement will be key to building acceptance and confidence.
Conclusion
The future of AI in nuclear safety is both promising and complex. As reactor technologies advance and data systems mature, AI will increasingly serve as a cornerstone of proactive, intelligent, and adaptive safety management. From autonomous monitoring to predictive control and cognitive infrastructure, AI is poised to usher in a new era of nuclear operational excellence.
Yet the path forward must be tread carefully. The challenges of technical robustness, regulatory compliance, cybersecurity, and human trust demand a multidisciplinary approach—one that aligns innovation with responsibility. By embracing this vision, the global nuclear sector can leverage AI not just as a tool, but as a transformational partner in the pursuit of safe, sustainable energy.
References
- International Atomic Energy Agency (IAEA) – AI for Nuclear Applications
https://www.iaea.org/topics/artificial-intelligence - U.S. Department of Energy – AI and the Nuclear Sector
https://www.energy.gov/ne/articles/ai-and-nuclear-energy-future - World Nuclear Association – Advanced Nuclear Power Reactors
https://world-nuclear.org/information-library/current-and-future-generation/advanced-nuclear-power-reactors.aspx - MIT Energy Initiative – Artificial Intelligence for Nuclear Energy
https://energy.mit.edu/research/artificial-intelligence-for-nuclear-energy - Idaho National Laboratory – Digital Twin Technology
https://inl.gov/research-program/digital-twins-for-reactor-safety - OECD Nuclear Energy Agency – Trustworthy AI in Nuclear Safety
https://www.oecd-nea.org/science/trustworthy-ai-nuclear-safety - EDF Group – AI for Predictive Maintenance in Nuclear Reactors
https://www.edf.fr/en/the-edf-group/industrial-innovation/artificial-intelligence-and-nuclear - International Journal of Nuclear Energy Science and Technology – AI Applications in Nuclear Reactor Monitoring
https://www.inderscience.com/jhome.php?jcode=ijnest - Korea Atomic Energy Research Institute (KAERI) – Smart AI-Based Nuclear Monitoring
https://www.kaeri.re.kr/english - Nuclear Regulatory Commission (NRC) – Digital Systems and AI in Nuclear Facilities
https://www.nrc.gov/about-nrc/regulatory/digital-systems.html