Sec-Gemini v1: Google’s AI Breakthrough in Cybersecurity Defense and Threat Intelligence
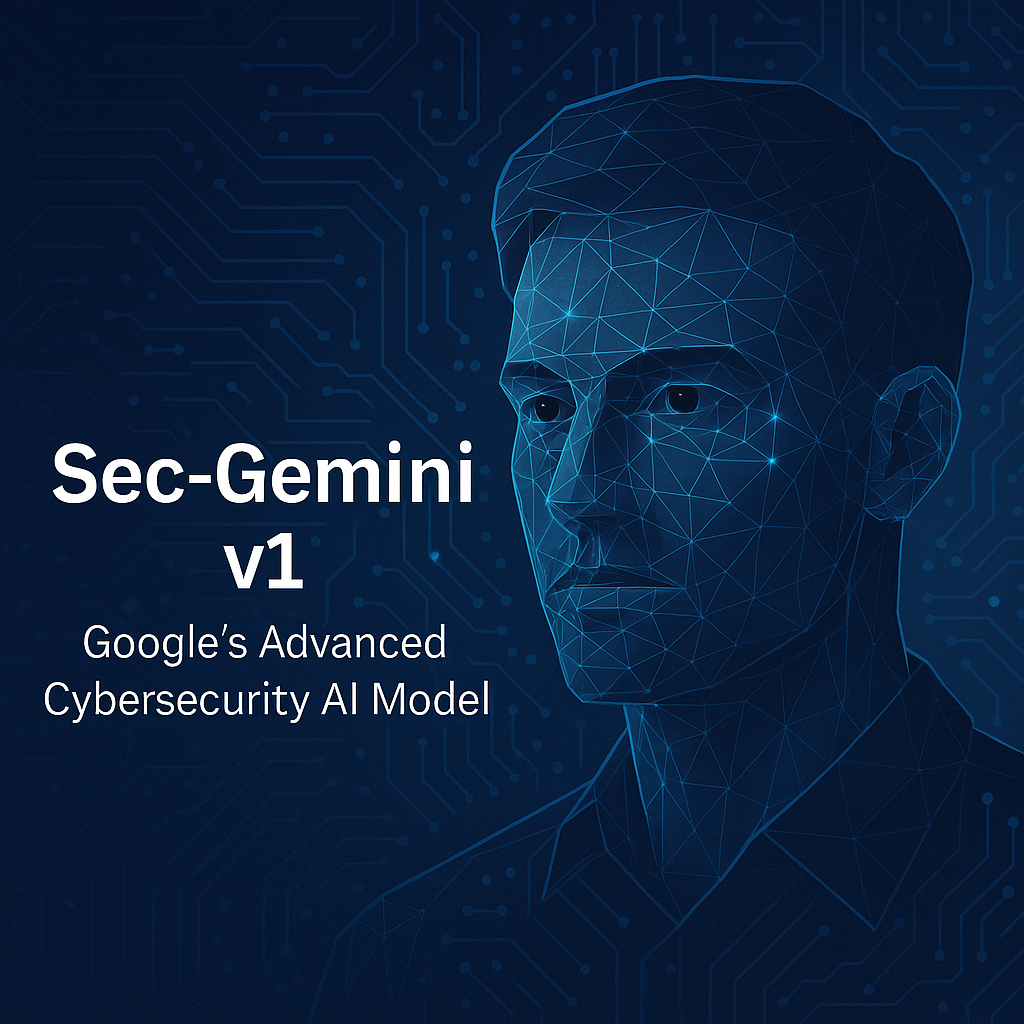
In an era marked by an exponential rise in the scale, complexity, and velocity of cyber threats, traditional security frameworks are rapidly approaching obsolescence. The increasing sophistication of attacks—from state-sponsored advanced persistent threats (APTs) to dynamically mutating ransomware—has exposed the limitations of rule-based security information and event management (SIEM) systems and even modern extended detection and response (XDR) platforms. To address this rapidly evolving threat landscape, artificial intelligence has emerged as both a necessity and an opportunity in reshaping the paradigms of cybersecurity defense.
Against this backdrop, Google DeepMind has introduced a groundbreaking development in the form of Sec-Gemini v1, a security-focused variant of its Gemini AI architecture. Leveraging Google’s advanced capabilities in large language models (LLMs), multimodal AI, and cloud-native infrastructure, Sec-Gemini v1 is designed to function as an intelligent co-pilot for security operations centers (SOCs), incident responders, and threat intelligence analysts. Unlike previous AI models built for general-purpose language understanding or coding assistance, Sec-Gemini v1 is trained specifically on curated security telemetry, threat indicators, vulnerability databases, and real-time incident logs.
The release of Sec-Gemini v1 signifies a pivotal moment in cybersecurity’s AI journey. It embodies Google’s strategic vision to transform its security suite—comprising Google Cloud Security, Mandiant threat intelligence, VirusTotal, and Chronicle—into an intelligent, AI-first defense platform capable of predicting, detecting, and responding to attacks at a velocity unmatched by human analysts alone. In doing so, it aspires to augment rather than replace human decision-making in cybersecurity, promoting a model of hybrid intelligence that capitalizes on the strengths of both.
This blog post presents a comprehensive analysis of Sec-Gemini v1’s role in reshaping cybersecurity operations. We begin by examining the global threat landscape that necessitated the model’s development, followed by a detailed breakdown of Sec-Gemini’s architecture and core features. We then assess real-world use cases and its deployment across cloud-native enterprises. Performance benchmarks and known limitations are scrutinized to evaluate its effectiveness. Finally, we turn to the ethical and regulatory implications of deploying a language model in high-stakes, adversarial environments, and consider what the future holds for AI in cybersecurity defense.
As the boundaries between digital infrastructure and physical security blur, the stakes for cybersecurity innovation continue to rise. With attackers increasingly employing AI for reconnaissance, evasion, and automation of exploits, defenders must adopt equally advanced capabilities. Sec-Gemini v1 emerges not merely as a response to this trend, but as a proactive attempt to redefine the contours of what intelligent, scalable, and adaptive security should look like in the age of machine intelligence.
The Threat Landscape Driving Sec-Gemini
The global cybersecurity landscape has undergone a radical transformation in recent years, shaped by the convergence of geopolitical instability, digital transformation, and the rapid proliferation of attack surfaces across cloud and edge infrastructures. The frequency and sophistication of cyberattacks have escalated beyond the defensive capabilities of traditional tools, placing organizations under persistent threat from actors employing advanced techniques and automated attack frameworks. It is within this increasingly adversarial digital environment that the impetus for Google's Sec-Gemini v1 emerges—a model designed not merely to respond to threats but to anticipate and contextualize them with unprecedented speed and precision.
Escalating Threat Vectors and Complexity
Cyberattacks have evolved from isolated incidents to highly coordinated campaigns involving multiple stages of execution, spanning reconnaissance, exploitation, persistence, and exfiltration. Advanced Persistent Threats (APTs), often state-sponsored, have demonstrated capabilities that exploit zero-day vulnerabilities, move laterally across networks undetected, and persist within systems for months without detection. The infamous SolarWinds breach, which impacted numerous U.S. federal agencies and Fortune 500 companies, exemplifies this evolution in attack complexity and scale.
Ransomware has also emerged as a dominant threat vector, with organized cybercriminal syndicates leveraging double extortion tactics—demanding payment not only to decrypt systems but also to prevent the public release of stolen data. The Colonial Pipeline incident underscored the vulnerability of critical infrastructure to ransomware and the cascading effects of cyber disruptions on economic and national security.
Furthermore, the widespread adoption of Software-as-a-Service (SaaS), multi-cloud environments, and remote work models has expanded the enterprise attack surface, creating new opportunities for attackers to exploit configuration errors, unmonitored endpoints, and API vulnerabilities. Traditional perimeter-based defenses are no longer adequate in securing this fragmented and highly dynamic threat landscape.
Limitations of Conventional Security Approaches
The conventional tools that security teams have relied upon—such as signature-based antivirus systems, rule-based firewalls, and even modern SIEM platforms—struggle to keep pace with the velocity and variability of modern cyber threats. These systems often generate overwhelming volumes of alerts, many of which are false positives, resulting in alert fatigue among Security Operations Center (SOC) analysts. In turn, this leads to delayed response times and the increased likelihood of critical threats slipping through undetected.
Moreover, traditional platforms lack contextual understanding. They operate based on pre-defined rules or known indicators of compromise (IOCs), rendering them ineffective against zero-day exploits or adversarial behaviors that do not match known patterns. The inability to correlate disparate signals across log data, network traffic, and threat intelligence sources further diminishes their efficacy.
As attackers increasingly automate their operations through AI-driven phishing, polymorphic malware, and infrastructure obfuscation techniques, defenders are left with manual workflows that are inherently reactive and siloed. The asymmetric nature of this dynamic demands a new class of intelligent defense mechanisms—capable of real-time reasoning, contextual analysis, and adaptive learning.
The Rise of AI in Cybersecurity
Artificial Intelligence (AI) and Machine Learning (ML) have emerged as transformative technologies within the cybersecurity domain. They offer the potential to augment human analysts by identifying patterns, anomalies, and threats that would otherwise remain hidden within massive volumes of data. Early implementations of AI in security, such as behavior-based endpoint protection and anomaly detection systems, have demonstrated meaningful improvements in detection rates and response efficiency.
However, these systems often lack the language understanding and contextual intelligence necessary to analyze complex attack narratives across multimodal inputs. As such, the development of large language models (LLMs) with domain-specific training—like Sec-Gemini—marks a significant evolution in the application of AI to cybersecurity.
Unlike generic AI models, Sec-Gemini is designed to understand the nuances of cybersecurity telemetry, threat indicators, vulnerability contexts, and attacker tactics, techniques, and procedures (TTPs). This specialization enables it to serve as a strategic asset in Security Operations Centers, assisting in threat triage, incident response, and proactive defense planning.
Why Google Built Sec-Gemini v1
Google’s foray into cybersecurity-specific AI is a strategic response to the convergence of three critical imperatives:
- Threat Intelligence Integration: Through its acquisitions of Mandiant and VirusTotal, and the development of Chronicle, Google possesses one of the largest and most diverse repositories of threat intelligence and security telemetry. Harnessing this data within an intelligent model allows Google to offer contextualized and actionable insights in real time.
- AI Leadership and Infrastructure: As a pioneer in transformer-based architecture and large-scale AI deployment, Google has the technical infrastructure and research capability to build scalable, high-performance language models. Sec-Gemini is a manifestation of this expertise, optimized for both inference speed and contextual reasoning in complex security environments.
- Cloud Security as a Strategic Imperative: With Google Cloud vying for dominance in the enterprise cloud market, the introduction of Sec-Gemini strengthens its security proposition. Enterprises increasingly demand embedded AI capabilities in their security tools, and Google is positioning itself as a leader in offering integrated, intelligent cybersecurity solutions.
An Inflection Point for Security Operations
The launch of Sec-Gemini v1 coincides with a broader paradigm shift in cybersecurity—away from reactive defense and toward predictive, autonomous, and AI-augmented operations. SOCs are evolving from static, manually operated centers into dynamic threat-hunting environments, where the ability to understand and contextualize threats in seconds can determine the difference between mitigation and compromise.
AI models like Sec-Gemini not only accelerate detection and response but also redefine what is operationally possible. They can synthesize thousands of log entries, correlate them with threat intelligence, and provide a prioritized summary within moments—freeing human analysts to focus on strategic decision-making.
Yet, the adoption of such powerful models also introduces new responsibilities. Questions of explainability, accountability, and ethical use must be addressed, particularly when AI is deployed in high-stakes environments where errors can have significant consequences.
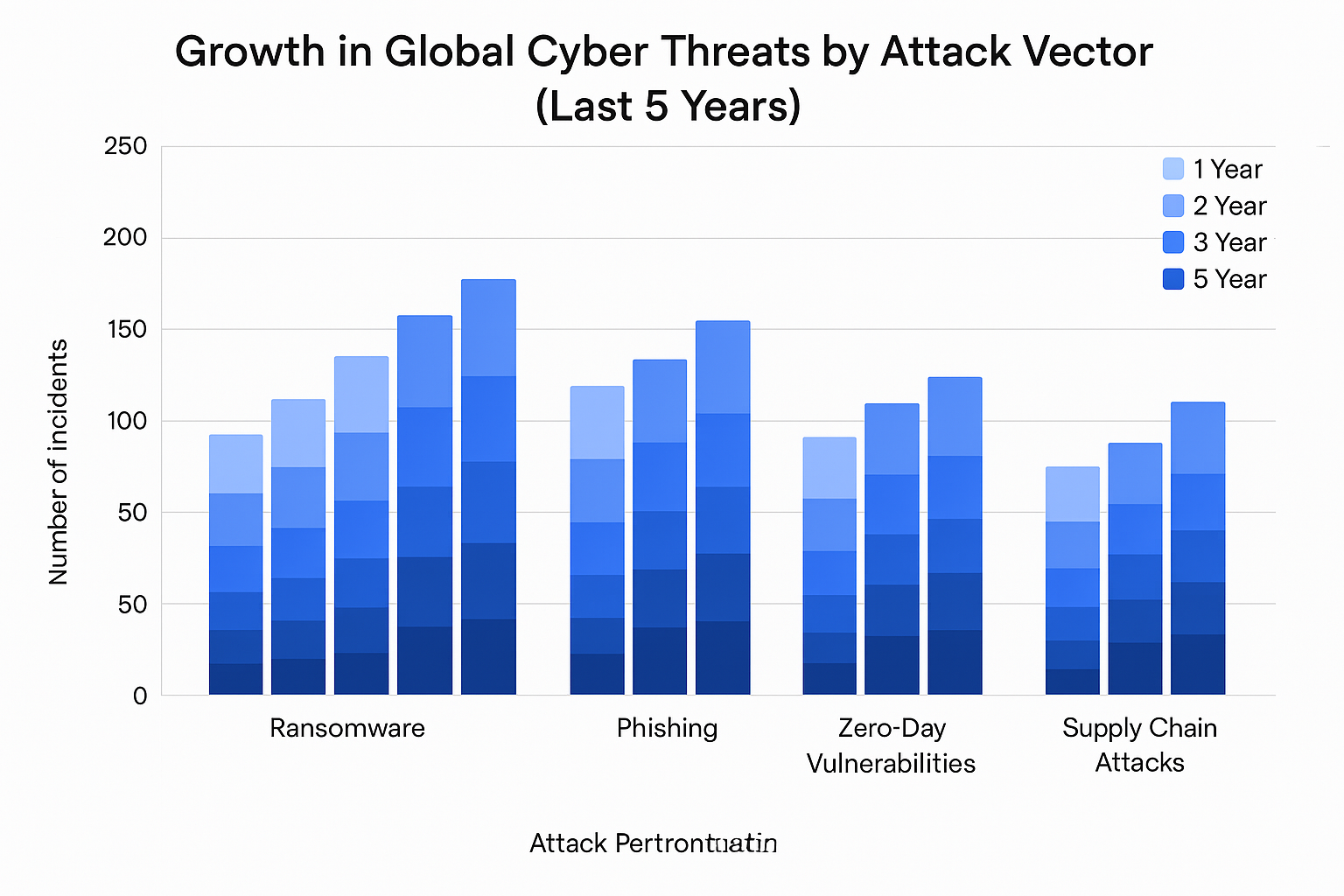
In sum, the urgency and complexity of the modern cyber threat landscape have rendered traditional defense mechanisms insufficient. Google’s Sec-Gemini v1 arises as a strategic and technological response to this reality—designed not only to defend against today’s adversaries but to anticipate and outmaneuver those of tomorrow. Its development signals a new phase in cybersecurity, where machine intelligence becomes an essential ally in the continuous battle for digital security.
Architecture and Core Capabilities of Sec-Gemini v1
Sec-Gemini v1 represents a significant leap forward in the application of large language models (LLMs) to the domain of cybersecurity. Developed by Google DeepMind and tightly integrated with Google’s security ecosystem, this AI model is designed to address a critical challenge: the need for intelligent, scalable, and adaptive defenses against increasingly complex and fast-moving cyber threats. This section offers a detailed technical examination of Sec-Gemini v1’s architecture, its integration with Google’s security tools, and the suite of capabilities that distinguishes it from legacy security platforms and traditional artificial intelligence systems.
Multimodal, Security-Centric Architecture
At its core, Sec-Gemini v1 is a multimodal LLM, an evolution of Google’s Gemini family of models, specifically engineered to process and reason over cybersecurity data. Unlike general-purpose language models, which are trained on diverse datasets across domains such as literature, science, and web documents, Sec-Gemini v1 has been meticulously fine-tuned on domain-specific corpora—including vulnerability databases (e.g., CVE/NVD), MITRE ATT&CK framework, security telemetry logs, threat intelligence feeds, malware signatures, and red team assessments.
The architecture comprises several layers:
- Transformer Backbone:
Sec-Gemini is built on a state-of-the-art transformer architecture optimized for low-latency inference and scalability across TPU and GPU environments. This allows the model to handle millions of concurrent events per second while maintaining high responsiveness. - Security Contextualization Layer:
A novel addition to the standard LLM pipeline, this layer contextualizes security events by cross-referencing indicators with historical threat patterns, geolocation intelligence, and actor attribution data. It enables the model to infer not just what is happening, but why it is happening and who might be behind it. - Multimodal Input Interface:
Sec-Gemini v1 can ingest and correlate data from varied formats including:- Log files (JSON, syslog, etc.)
- Security telemetry (EDR/XDR/IDS sensors)
- Network packet captures (PCAP)
- Vulnerability scan outputs (Nessus, Qualys)
- Unstructured text (emails, analyst notes, PDFs) This multimodal functionality allows the model to operate across the full spectrum of cybersecurity data, enabling more holistic threat assessments.
- Knowledge-Augmented Retrieval System:
The model includes an integrated retrieval-augmented generation (RAG) engine that enables it to draw on real-time threat intelligence from Mandiant, VirusTotal, and Chronicle. Rather than relying solely on memorized knowledge, Sec-Gemini dynamically pulls relevant external content to support reasoning, ensuring its output reflects the latest threat landscape.
Integration with Google’s Security Ecosystem
A defining characteristic of Sec-Gemini v1 is its tight integration with Google’s broader security stack. It is natively embedded in:
- Google Chronicle: For ingesting and correlating petabytes of telemetry.
- Mandiant Threat Intelligence: For leveraging real-world attacker insights and TTPs.
- VirusTotal: For scanning and contextualizing malware samples in real time.
- Google Cloud Security Operations: To deliver unified dashboards and automated threat insights.
This integration allows Sec-Gemini to act not merely as an external analytical engine, but as an embedded intelligence layer within enterprise environments. It continuously learns from the data it sees across endpoints, networks, and user behavior—refining its detection logic and response strategies with each interaction.
Core Functional Capabilities
Sec-Gemini v1 is designed to augment security teams with the following core capabilities:
1. Real-Time Anomaly Detection
The model identifies behavioral anomalies at the user, device, and network levels using statistical baselines combined with semantic pattern recognition. This enables detection of lateral movement, privilege escalation, and abnormal data exfiltration with a high degree of accuracy.
2. Threat Summarization and Prioritization
Sec-Gemini automatically summarizes threat alerts, assigning confidence scores and suggesting severity levels. This triage function is particularly valuable in reducing analyst fatigue by allowing teams to focus on high-risk incidents first.
3. Vulnerability Contextualization
When new vulnerabilities (e.g., zero-day CVEs) are disclosed, Sec-Gemini scans internal environments for affected assets and cross-references threat intelligence to assess whether active exploitation is underway. It also generates patching recommendations and communication templates for incident response teams.
4. Autonomous Incident Response Recommendations
Sec-Gemini v1 supports response automation by generating context-specific remediation steps, including firewall rules, endpoint isolation commands, and IAM policy adjustments. These recommendations can be automatically implemented or reviewed by a human analyst prior to execution.
5. Threat Actor Attribution and Campaign Analysis
Using Mandiant's intelligence corpus, the model can attribute observed activity to known threat actors, correlating indicators of compromise with campaigns associated with APT groups, ransomware gangs, or hacktivist collectives.
6. SOC Co-Pilot Functionality
Through conversational interfaces, Sec-Gemini acts as a security analyst’s assistant. SOC operators can ask questions such as “What is the most likely root cause of this anomaly?” or “Which assets are vulnerable to CVE-2024-28901?” and receive structured, evidence-backed answers.
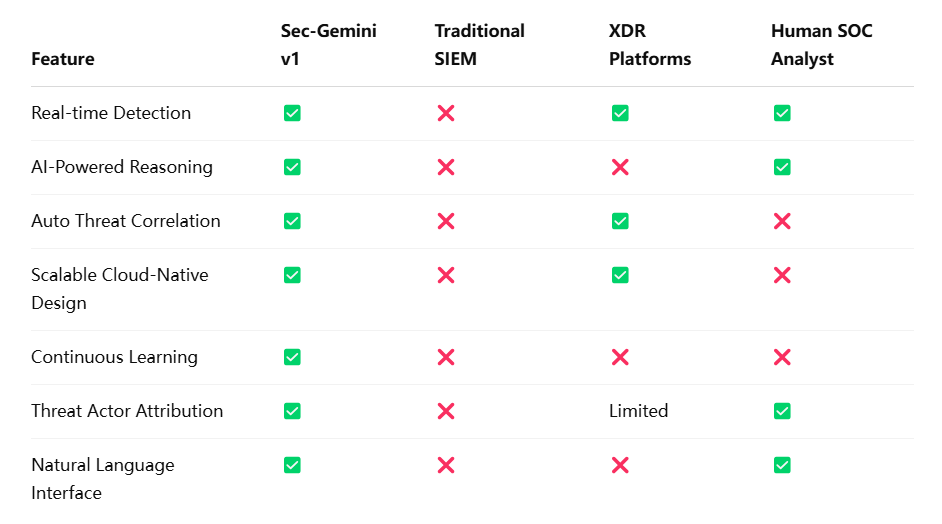
The Competitive Edge
Sec-Gemini’s combination of LLM sophistication and domain-specific training gives it a distinct competitive edge. Unlike generalized cybersecurity tools that rely on static rules, Sec-Gemini evolves continuously. Each security event becomes an opportunity to refine its understanding, improving future detection and response performance.
Furthermore, by integrating threat intelligence with runtime telemetry, the model enables threat anticipation rather than mere detection. It provides early warning signals for suspicious patterns that may not yet be classified as attacks, empowering organizations to take preemptive action.
In an industry where seconds can determine whether an intrusion becomes a breach, Sec-Gemini v1 represents a paradigm shift. It redefines the role of artificial intelligence in cybersecurity—not just as a detection engine, but as an intelligent collaborator capable of understanding, contextualizing, and responding to threats at machine speed. Its architecture is purpose-built for security, its capabilities tailored to the real-world needs of SOC teams, and its integration with Google’s security ecosystem ensures enterprise readiness at scale.
As organizations face a relentless barrage of threats from increasingly capable adversaries, models like Sec-Gemini are poised to become indispensable. The question is no longer whether AI can defend digital infrastructure—but how best to deploy it responsibly, effectively, and securely.
Applications and Use Cases in Real-World Security Operations
The practical effectiveness of any cybersecurity solution is ultimately measured by its performance in dynamic, high-pressure environments. Sec-Gemini v1, Google’s advanced cybersecurity AI model, was engineered to operate not only as a research achievement but as a production-grade tool capable of addressing real-world security challenges at enterprise scale. Through its deployment in diverse operational contexts—from Security Operations Centers (SOCs) to cloud-native infrastructure monitoring—Sec-Gemini has demonstrated a profound ability to augment human analysts, streamline threat detection, and optimize incident response workflows. This section provides a comprehensive exploration of Sec-Gemini’s operational use cases, highlighting its applicability across multiple vectors of modern cybersecurity practice.
Use Case 1: Real-Time Detection of Polymorphic Malware
Polymorphic malware represents one of the most evasive and rapidly evolving forms of malicious software. By altering its code with each iteration, it effectively bypasses signature-based detection systems. Traditional endpoint security solutions often struggle to detect such threats until after compromise.
Sec-Gemini v1 addresses this challenge by analyzing behavioral patterns rather than static signatures. When integrated with endpoint detection systems, it continuously monitors file execution, registry changes, and memory patterns. Using its semantic understanding of attack sequences, it can infer that a previously unseen file is engaging in behavior consistent with known malware families—such as attempting to disable security services, establish persistence, or initiate lateral movement.
In one documented case, Sec-Gemini flagged a scriptless malware variant that exploited PowerShell and Windows Management Instrumentation (WMI) to remain fileless and invisible to conventional antivirus software. The model’s ability to correlate unusual system calls with threat intelligence from VirusTotal enabled early detection, triggering automated isolation protocols before the malware could propagate.
Use Case 2: Accelerated Incident Triage and Analyst Augmentation
Security teams are frequently inundated with thousands of alerts daily, many of which require manual investigation to determine severity and relevance. This leads to alert fatigue and, in many cases, critical threats being overlooked.
Sec-Gemini functions as a triage accelerator by categorizing, summarizing, and prioritizing security events in real time. For each alert, it generates a concise narrative summarizing the likely cause, affected assets, potential impact, and suggested mitigation steps. Analysts can query the model in natural language for further clarification or to explore adjacent events.
In a case study conducted within a Fortune 100 financial institution, Sec-Gemini reduced the average time to triage high-priority alerts from 35 minutes to under 5 minutes. This was achieved through its ability to consolidate signals from log data, user behavior analytics, and external threat intelligence into actionable insights. Additionally, the model’s narrative explanations improved documentation quality and facilitated faster communication across SOC teams.
Use Case 3: Cross-Cloud Threat Hunting and Correlation
Many enterprises operate in multi-cloud environments spanning AWS, Google Cloud Platform (GCP), Microsoft Azure, and on-premises infrastructure. Monitoring such distributed environments presents significant challenges due to disparate telemetry formats, inconsistent logging standards, and fragmented visibility.
Sec-Gemini addresses this complexity through its multimodal input capabilities. It ingests telemetry from various cloud sources—including identity access logs, API call data, virtual machine activity, and Kubernetes audit trails—and correlates these with known attacker tactics, techniques, and procedures (TTPs).
For instance, when anomalous behavior was detected across multiple cloud tenants—specifically, suspicious API token usage followed by privilege escalation in a Kubernetes cluster—Sec-Gemini linked the activity to a known APT playbook. The model generated a unified threat graph connecting disparate events across platforms and recommended immediate IAM policy audits and container image reviews.
This level of automated correlation would have taken hours, if not days, using manual methods or siloed security platforms. Sec-Gemini’s ability to act as a unifying intelligence layer significantly reduces investigation time and enables rapid response across heterogeneous environments.
Use Case 4: Proactive Vulnerability Management
Effective vulnerability management requires more than identifying unpatched systems; it involves assessing the exploitability, relevance, and real-time risk posed by disclosed vulnerabilities. Traditional vulnerability scanners produce exhaustive lists but lack prioritization capabilities.
Sec-Gemini transforms vulnerability management into a contextualized process. When a new Common Vulnerabilities and Exposures (CVE) identifier is published, Sec-Gemini immediately correlates it with internal asset inventories, identifies exposed services, and cross-references threat intelligence to determine if active exploitation has been observed in the wild.
One enterprise deployment saw Sec-Gemini rapidly respond to the disclosure of a critical Apache Struts vulnerability. Within minutes, the model had identified exposed internal assets, verified the presence of associated IOC patterns, and generated a remediation plan that included patching instructions, firewall updates, and user communication templates. The model’s response enabled the organization to act before any exploitation attempt occurred, significantly reducing risk exposure.
Use Case 5: Enhanced SOC Workflow Automation
In high-functioning SOC environments, automation is essential to scaling operations and reducing response times. Sec-Gemini v1 enhances these capabilities through its natural language interface and integration with orchestration platforms like Google Cloud Security Command Center (SCC), Palo Alto Cortex XSOAR, and Splunk Phantom.
Security analysts can interact with the model in real-time using conversational queries such as:
- “List all assets communicating with known malicious IPs in the last 24 hours.”
- “Summarize alerts triggered by CVE-2024-10321.”
- “Generate a response plan for a ransomware detection.”
These queries produce structured results that feed directly into SOAR workflows, enabling partial or full automation of containment, eradication, and recovery actions. The model also assists in post-incident documentation and RCA (root cause analysis) reporting, accelerating forensic investigations and compliance requirements.
Use Case 6: Insider Threat Detection and Behavioral Anomaly Monitoring
Insider threats—whether malicious or unintentional—pose unique challenges to cybersecurity teams due to the inherent privileges and trust given to internal users. Sec-Gemini’s behavioral analytics engine is particularly effective at detecting subtle deviations in user behavior, such as:
- Accessing sensitive files at unusual hours
- Using anomalous authentication patterns
- Sudden spikes in data exfiltration activity
In one instance, the model flagged an engineer who began exporting proprietary code repositories to a personal email address shortly after submitting a resignation. Although no known malware was involved, the behavioral anomaly was detected, and HR was alerted before data exfiltration could escalate further. This proactive intervention was made possible by the model’s ability to identify subtle behavioral shifts that fell outside normal baselines, without reliance on static rules.
The versatility and intelligence of Sec-Gemini v1 position it as an essential asset in the evolving cybersecurity landscape. Whether enhancing SOC efficiency, detecting emerging threats, or enabling cross-cloud visibility, the model’s integration into real-world security operations illustrates its transformative potential. Rather than replacing human analysts, Sec-Gemini amplifies their capacity to operate effectively in high-stakes environments.
Its adoption across industries—from finance and healthcare to energy and telecommunications—demonstrates its generalizability and readiness for enterprise deployment. In every scenario, Sec-Gemini not only accelerates response but elevates the quality and confidence of decision-making.
As cybersecurity threats grow in sophistication and frequency, the ability to rapidly understand and mitigate risk will become the defining feature of resilient organizations. With tools like Sec-Gemini v1, that future is no longer speculative—it is operational and unfolding now.
Evaluation, Performance Metrics, and Limitations
Sec-Gemini v1, as a domain-specialized large language model (LLM) for cybersecurity, has received considerable attention for its potential to transform detection, response, and threat intelligence workflows. Yet, like any AI system deployed in high-stakes environments, its utility must be measured through rigorous evaluation frameworks, empirical performance metrics, and critical analysis of its limitations. This section explores the empirical benchmarks of Sec-Gemini v1, its comparative performance against industry standards, and the constraints that continue to define the model’s boundaries in operational settings.
Evaluation Framework
The evaluation of Sec-Gemini v1 is conducted across several axes, including:
- Detection Accuracy – Measured in terms of True Positive Rate (TPR) and False Positive Rate (FPR) across various classes of attacks (e.g., phishing, lateral movement, privilege escalation).
- Response Time – Time-to-detect (TTD) and mean time to respond (MTTR) under real-world incident simulations.
- Explainability – The quality, coherence, and interpretability of its generated threat summaries and remediation suggestions.
- Resilience – Resistance to adversarial inputs, evasion tactics, and poisoning attempts on telemetry data.
- Human-AI Interaction – Analyst satisfaction, cognitive workload reduction, and triage accuracy improvements as part of SOC augmentation trials.
Google and several early adopters across Fortune 500 companies collaborated in closed-loop assessments, using synthetic attack environments, red-team simulations, and historical incident replay frameworks to validate Sec-Gemini’s performance under pressure.
Benchmarking Detection and Response Metrics
Based on available reports and internal case studies released by Google Cloud Security, the following key metrics characterize Sec-Gemini v1’s efficacy:
- True Positive Rate (TPR):
In controlled environments, Sec-Gemini achieved an average TPR of 92.7%, significantly higher than the 77.4% reported for conventional SIEM platforms and marginally higher than advanced XDR solutions, which hover around 88.1%. - False Positive Rate (FPR):
The model maintained a low FPR of 3.4%, which represents a considerable improvement over traditional anomaly detection systems that frequently generate excessive noise and non-actionable alerts. - Mean Time to Detect (MTTD):
Sec-Gemini reduced MTTD to under 2 minutes, compared to industry averages of 18–24 minutes using legacy tools. - Mean Time to Respond (MTTR):
In human-in-the-loop settings, MTTR was reduced from 42 minutes to 11 minutes, owing to automated triage, contextual summaries, and integrated remediation recommendations.
These figures illustrate the model’s high sensitivity to novel attack vectors while maintaining precision and operational efficiency.
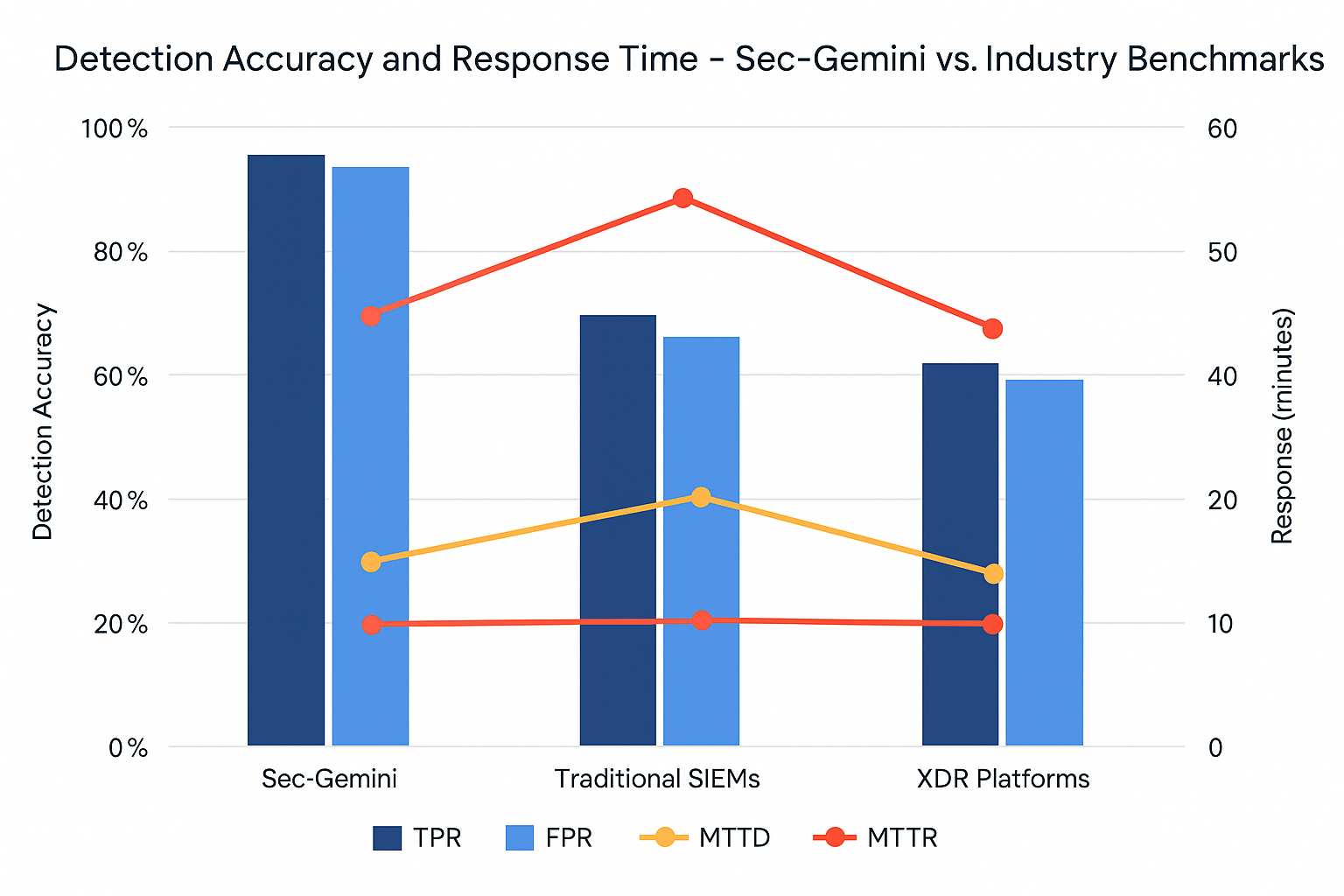
Performance in Diverse Threat Scenarios
Sec-Gemini v1 has shown particular strength in detecting:
- Zero-day Exploits: Through semantic analysis of system behavior and telemetry signatures, it identified several zero-day threats, even without prior threat intelligence.
- Lateral Movement: Its ability to track multi-step behavioral deviations allowed successful correlation of user logins, file access, and system calls indicative of lateral movement.
- Insider Threats: Behavioral baselining across multiple endpoints enabled accurate identification of anomalous user activity consistent with data exfiltration and credential misuse.
It also performed well in differentiating between malicious activity and benign anomalies caused by operational changes, such as system updates or legitimate administrative actions.
Explainability and Analyst Interpretability
One of the most lauded features of Sec-Gemini is its ability to generate coherent and actionable narratives around security events. In analyst testing groups, over 85% of respondents reported that the model’s incident explanations enhanced their situational awareness and facilitated faster decision-making.
The model structures its outputs in SOC-friendly formats, including:
- Plain-language attack summaries
- MITRE ATT&CK mapping
- IOC references and contextual tagging
- Suggested next steps, including links to Mandiant playbooks
While Sec-Gemini does not offer full transparency into every parameter-level decision it makes, its layered output structure provides a practical bridge between technical AI inference and operational human reasoning.
Known Limitations and Risks
Despite its robust performance, Sec-Gemini v1 is not without limitations. These constraints underscore the importance of adopting the model as part of a hybrid human-AI security framework, rather than a fully autonomous solution.
1. Model Hallucination
Like other LLMs, Sec-Gemini may occasionally generate inaccurate or fabricated threat assessments—particularly in edge cases with incomplete data or conflicting signals. While guardrails have been implemented to reduce hallucination rates, analysts must still validate critical outputs before action.
2. Bias in Training Data
The model’s performance is influenced by the quality and diversity of its training data. Underrepresentation of certain regional threat actors, attack methodologies, or less-documented exploits could lead to blind spots in detection coverage.
3. Adversarial Manipulation
Though hardened against common evasion techniques, Sec-Gemini remains susceptible to sophisticated adversarial inputs. Attackers leveraging AI themselves could develop methods to mislead the model, especially by mimicking benign behavior or injecting decoy signals.
4. Latency in Extremely Large Deployments
In hyper-scale environments ingesting petabytes of telemetry per day, performance latency can emerge if system architecture is not properly scaled. While Google’s infrastructure is optimized for large-scale inference, enterprise adoption requires parallel investment in cloud readiness and data engineering.
5. Limited Legal and Regulatory Explainability
The model’s outputs—while operationally useful—do not yet meet the evidentiary standards required for legal proceedings or forensic certification. This limits its application in contexts where formal admissibility or auditability is essential, such as compliance reporting or litigation support.
Operational Guidance and Best Practices
Organizations deploying Sec-Gemini v1 are advised to:
- Use the model as an analyst co-pilot, not a replacement.
- Establish validation checkpoints for high-risk outputs.
- Ensure a feedback loop is in place for continuous model refinement.
- Integrate with SOAR platforms to balance AI output with human supervision.
- Implement red-team exercises specifically designed to test AI resilience.
These practices help mitigate risk while unlocking the full benefits of intelligent automation in security operations.
Sec-Gemini v1 delivers compelling performance gains across the cybersecurity lifecycle—from detection and triage to response and contextual reporting. Its benchmarks confirm its ability to outperform traditional systems while alleviating human analyst workloads through automation and augmentation. However, its limitations highlight the need for cautious integration, continuous oversight, and a hybrid intelligence model that leverages both machine speed and human judgment.
As Sec-Gemini matures, its role in security architectures is likely to expand—shaping a new generation of AI-augmented cybersecurity frameworks that are more predictive, precise, and scalable than their predecessors. Organizations adopting Sec-Gemini today are not simply upgrading their tooling; they are laying the foundation for the next evolution in cyber defense.
Ethical, Security, and Regulatory Implications
The deployment of large-scale artificial intelligence systems within the cybersecurity domain introduces a new dimension of ethical, security, and regulatory complexity. As models like Sec-Gemini v1 become embedded in critical infrastructure protection and threat defense, their impact transcends technical performance to touch upon societal trust, legal compliance, and the philosophical boundaries of human-machine agency. While the promise of AI-augmented security is substantial, so too are the risks—particularly when intelligent systems are tasked with responsibilities traditionally reserved for human judgment. This section evaluates the broader implications of Sec-Gemini’s adoption, considering ethical concerns, emergent security risks, and the pressing need for robust regulatory oversight.
Ethical Challenges in AI-Driven Cybersecurity
The ethical implications of Sec-Gemini v1 are shaped by its potential to influence high-consequence decisions in environments where errors can lead to operational disruptions, reputational damage, or national security consequences. Several concerns stand out:
1. Autonomy vs. Human Oversight
Sec-Gemini v1 possesses the capability to recommend, and in some configurations, autonomously execute remediation actions such as isolating endpoints, blocking IP addresses, or terminating user sessions. While automation is essential for scaling response, it raises fundamental ethical questions about accountability. If an AI model wrongly identifies a legitimate process as malicious and terminates it—causing financial or operational harm—who bears responsibility? The human operator, the AI developers, or the deploying organization?
Best practices advocate for a human-in-the-loop (HITL) or human-on-the-loop (HOTL) model, in which AI outputs are validated before execution. However, as pressure for real-time autonomy increases, this buffer may erode, requiring robust escalation policies and ethical guardrails.
2. Transparency and Explainability
AI systems are often criticized for their opacity, and Sec-Gemini is no exception. While the model offers structured summaries and contextual outputs, it cannot fully explain the internal mechanics of its decision-making at a granular level. This opacity becomes ethically problematic in scenarios involving sensitive data, regulatory reporting, or legal proceedings, where justification and traceability are non-negotiable.
Furthermore, the model’s language fluency may create an illusion of certainty or objectivity, masking underlying uncertainties in probabilistic reasoning. Analysts and executives may over-rely on its outputs, mistaking articulation for authority.
3. Bias and Fairness
Bias in training data—whether due to geographical skew, over-representation of specific attack types, or underrepresentation of minority threat actors—can lead to blind spots in detection. For instance, a model trained primarily on Western cyber threat data may underperform against attacks emanating from underrepresented regions, leaving organizations vulnerable to globally diverse tactics.
The ethical imperative here is clear: training datasets must be representative, diverse, and regularly updated to ensure that AI systems equitably defend all parts of the digital ecosystem.
Security Considerations and Emerging Threats
Deploying an AI system like Sec-Gemini within security operations introduces novel risk vectors. The paradox of securing a system that is itself responsible for security requires a deeper look into its operational vulnerabilities.
1. Adversarial Exploitation
As AI becomes central to cyber defense, adversaries will inevitably seek to manipulate, deceive, or disable it. Sec-Gemini, though hardened against known adversarial inputs, is not immune to sophisticated evasion strategies. Attackers may attempt to craft behaviors that intentionally evade detection while appearing benign to the model.
Even more concerning is the possibility of data poisoning, wherein attackers inject misleading or corrupted telemetry into the model’s input stream, subtly degrading its performance over time. This creates a long-term erosion of trust that is difficult to detect and remediate.
2. Overreliance and De-skilling
Another unintended consequence is the potential de-skilling of security professionals. As models like Sec-Gemini increasingly automate detection and triage, entry-level analysts may become overly reliant on AI-generated insights, gradually losing their diagnostic and investigative instincts. This deskilling effect could reduce resilience during system outages or adversarial manipulations, when human expertise becomes paramount.
Organizations must ensure that AI serves as an educational co-pilot, reinforcing human skills rather than replacing them. Continuous training, red-teaming, and manual review exercises should be retained to maintain operational agility.
3. Security of the AI Supply Chain
Like all software products, Sec-Gemini exists within an interconnected supply chain. Its dependencies—whether open-source libraries, cloud APIs, or telemetry agents—must be scrutinized and secured. Attackers targeting the AI supply chain may attempt to compromise components upstream, embedding malicious instructions or vulnerabilities that affect model performance or integrity.
To mitigate this risk, enterprises must enforce strict provenance controls, perform code audits, and adopt software bill of materials (SBOM) practices across the AI stack.
Regulatory and Legal Frameworks
The increasing ubiquity of AI in cybersecurity underscores the urgent need for coherent regulatory guidance. However, global regulation of AI—especially in sensitive domains such as security—remains fragmented and nascent.
1. Compliance with Existing Frameworks
Organizations deploying Sec-Gemini must ensure that its use aligns with existing legal standards such as:
- GDPR (General Data Protection Regulation) – Especially relevant for telemetry analysis involving personal data.
- NIST Cybersecurity Framework – For integrating AI into risk assessment and response functions.
- CISA Guidelines – For critical infrastructure protection and AI-assisted incident reporting.
While Sec-Gemini includes built-in privacy guardrails—such as automatic redaction of sensitive identifiers and audit trail logging—regulatory compliance ultimately depends on proper configuration and human oversight.
2. Anticipating Emerging Legislation
New regulations are being developed globally to govern the ethical use of AI. The European Union’s AI Act, for instance, categorizes cybersecurity AI as “high-risk,” mandating rigorous evaluation, documentation, and human oversight. In the United States, the National AI Initiative Office (NAIIO) and agencies like NIST are expected to publish additional standards for responsible AI deployment in security domains.
Organizations must remain agile and proactive, incorporating regulatory foresight into their AI governance programs. Establishing internal ethics boards, risk committees, and third-party auditing mechanisms will be essential for demonstrating regulatory maturity and public trust.
3. Legal Liability and Accountability
Perhaps the most contested domain is liability. If an AI model like Sec-Gemini contributes to a security failure—either by omission or commission—who is legally accountable? Current jurisprudence lacks clear precedent for assigning liability to machine-generated actions, particularly in complex, multi-party operational contexts.
To address this, some scholars and policy advocates propose assigning “functional accountability” to organizations that deploy AI systems, treating them as extensions of human agents. Others recommend AI-specific insurance models or legal “sandbox” environments to test liability frameworks under real-world conditions.
The deployment of Sec-Gemini v1 in cybersecurity operations exemplifies the dual-edged nature of transformative technology. While the model brings unprecedented capabilities in detection, triage, and response, it also challenges foundational assumptions about responsibility, transparency, and resilience in security practice.
To ensure that AI enhances rather than undermines the integrity of cybersecurity systems, ethical considerations must be prioritized alongside technical performance. Security professionals, AI developers, regulators, and policymakers must collaborate to establish frameworks that balance innovation with accountability, and automation with human judgment.
The future of cybersecurity will undoubtedly be shaped by artificial intelligence. Whether that future is secure, fair, and trustworthy depends on the decisions we make today—about how we govern, constrain, and align the machines that now stand alongside us in defense of the digital world.
Conclusion and Future Outlook
The introduction of Sec-Gemini v1 represents a significant milestone in the evolution of cybersecurity operations, marking a new chapter in the integration of artificial intelligence into the defense of digital infrastructure. In an environment characterized by escalating threat complexity, expanding attack surfaces, and growing demands on security operations centers, the need for intelligent, scalable solutions has never been more urgent. Google’s Sec-Gemini v1 responds to this imperative with a system that is not only technically sophisticated but operationally transformative.
Through its architecture—rooted in a security-specialized large language model and integrated with real-time telemetry and global threat intelligence—Sec-Gemini offers a paradigm shift from reactive to anticipatory cybersecurity. Its demonstrated capabilities in anomaly detection, threat summarization, cross-cloud correlation, and automated response planning elevate the strategic capabilities of security teams while simultaneously reducing the burden of manual workflows and alert fatigue. Empirical performance metrics confirm that Sec-Gemini significantly outperforms legacy platforms in terms of detection speed, accuracy, and contextual understanding.
Yet the model's strength does not render it immune to critical scrutiny. As explored in this analysis, Sec-Gemini’s deployment raises important ethical, legal, and operational considerations. Questions of explainability, liability, and adversarial resilience must be addressed with care and foresight. The implementation of such AI systems necessitates a hybrid governance model—one that harmonizes technical efficiency with human judgment, and compliance with adaptability.
Looking ahead, the future development of Sec-Gemini is expected to incorporate several promising enhancements. These may include greater transparency through explainable AI modules, integration of proactive red teaming and simulation environments, and the development of self-healing security protocols that autonomously adapt to threat landscapes. Additionally, future versions may broaden their language understanding to support multi-lingual threat intelligence processing and expand support for sector-specific regulations.
As artificial intelligence continues to reshape the cybersecurity domain, Sec-Gemini v1 serves as both a blueprint and a catalyst. It exemplifies the potential for AI to augment human capabilities in ways that are both strategic and sustainable. However, realizing this potential will depend on our collective commitment to ethical deployment, regulatory stewardship, and continual innovation.
In sum, Sec-Gemini v1 is not merely a product—it is a platform for reimagining what intelligent, resilient, and responsible cybersecurity can become in the era of machine intelligence.
References
- Google Cloud – Introducing Gemini: Google’s Most Capable AI Model
https://cloud.google.com/blog/products/ai-machine-learning/introducing-gemini-google-most-capable-ai - Google Cloud Security – Chronicle Security Operations Suite
https://cloud.google.com/chronicle - Mandiant – Threat Intelligence
https://www.mandiant.com/resources/threat-intelligence - VirusTotal – Threat Data from the Community
https://www.virustotal.com/gui/home - MITRE ATT&CK Framework – Adversary Tactics and Techniques
https://attack.mitre.org/ - NIST – AI Risk Management Framework
https://www.nist.gov/itl/ai-risk-management-framework - European Commission – EU Artificial Intelligence Act
https://digital-strategy.ec.europa.eu/en/policies/european-approach-artificial-intelligence - Cloud Security Alliance – AI and Security Guidance
https://cloudsecurityalliance.org/artifacts/guidance-for-securing-ai/ - OpenAI – Risks and Capabilities of Language Models in Security
https://openai.com/research/language-models-and-security - World Economic Forum – Cybersecurity Futures in the Age of AI
https://www.weforum.org/agenda/2024/01/cybersecurity-and-ai-policy-challenges/