Mastering Thematic Roles in Sentences with LLMs for Enhanced Language Understanding
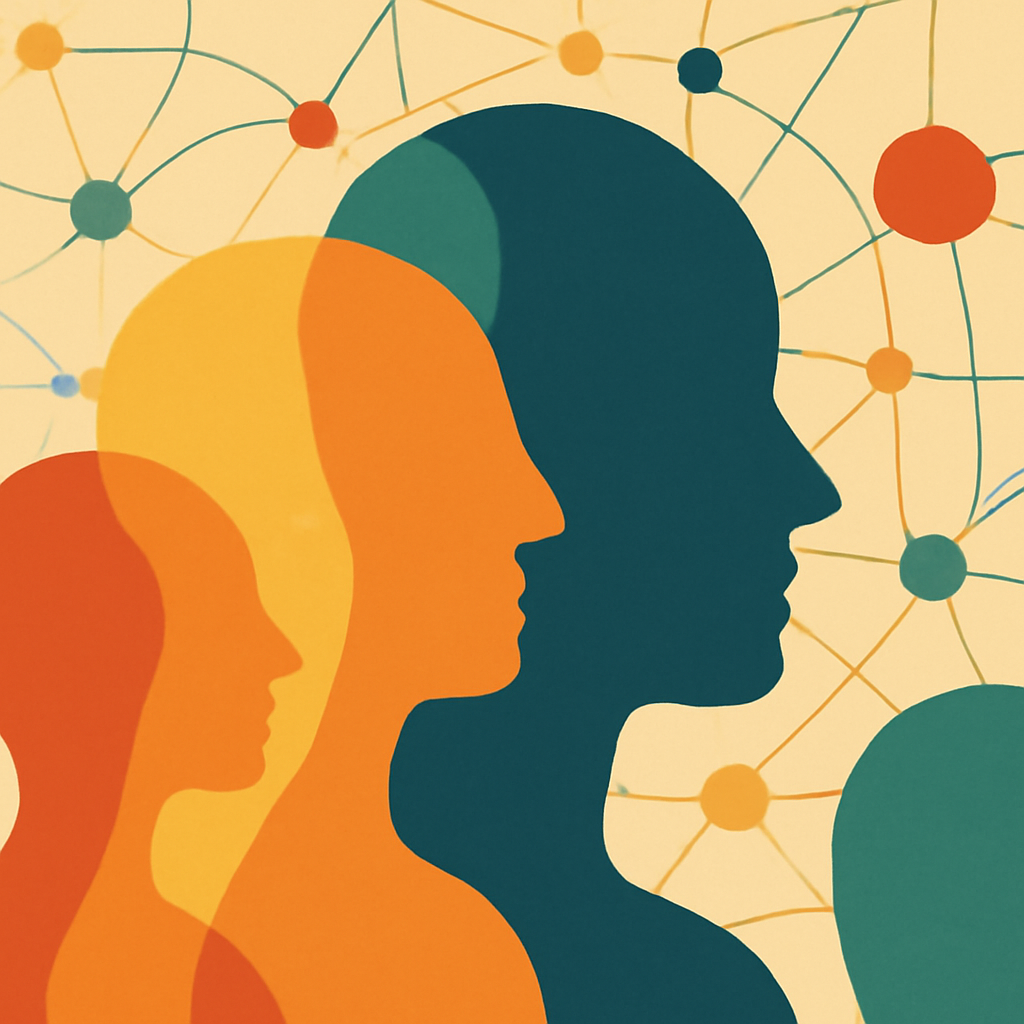
Understanding the complexities of human language has been a central goal in linguistics, computer science, and artificial intelligence (AI) for many decades. One key area that has gained significant attention in the development of natural language processing (NLP) systems is the concept of thematic roles. These roles refer to the various functions that different elements of a sentence take on, such as the agent (the doer of an action), the patient (the recipient of an action), or the experiencer (the one who perceives or experiences an action). Thematic roles play a critical role in how sentences are constructed and interpreted, as they directly impact the meaning conveyed by a sentence.
In recent years, large language models (LLMs) such as GPT-3, GPT-4, and BERT have become the go-to tools for various NLP tasks, including sentiment analysis, machine translation, and question answering. These models have shown remarkable proficiency in generating human-like text and understanding the nuances of language. However, the understanding of thematic roles is crucial for these models to achieve a deeper understanding of sentence structures and meanings. Thematic roles help LLMs parse complex sentence structures, resolve ambiguities, and produce more accurate responses to queries or tasks that involve language understanding.
The importance of thematic roles in natural language understanding cannot be overstated. When humans process language, we instinctively identify these roles, which allows us to understand not just the individual words in a sentence but also their relationships to each other and their contribution to the overall meaning. For instance, in the sentence "John (Agent) gave a book (Theme) to Mary (Recipient)," we intuitively recognize the roles each noun plays within the sentence structure. This is crucial in real-world applications such as machine translation or text summarization, where accurate understanding of roles ensures the output reflects the true meaning of the input.
LLMs, particularly those trained with vast amounts of linguistic data, have made significant strides in mimicking this human-like understanding of thematic roles. These models utilize deep learning techniques to process large volumes of text data, enabling them to recognize patterns and semantic relationships within sentences. However, while LLMs are often able to produce coherent text and engage in complex linguistic tasks, their ability to consistently and accurately assign thematic roles across diverse contexts remains an ongoing challenge.
This blog post aims to explore the concept of thematic roles in sentences, how large language models identify and use these roles, and why this understanding is pivotal for improving the capabilities of these models in real-world applications. In the following sections, we will delve into the various types of thematic roles, examine how LLMs process these roles, and discuss the practical implications of this understanding in the world of AI and NLP.
Thematic Roles in Linguistics
Thematic roles, also known as theta roles or semantic roles, are a foundational concept in linguistics. They describe the various participants and their functions within a sentence, essentially clarifying how different elements in a sentence relate to the main action or event. These roles are integral to understanding the meaning conveyed by the sentence, as they determine who is doing what to whom, when, where, and how. In the context of large language models (LLMs) like GPT and BERT, a nuanced understanding of thematic roles enables machines to interpret sentences with greater precision, mirroring human-like comprehension.
The most commonly discussed thematic roles in linguistics include Agent, Patient, Experiencer, Theme, Goal, and Instrument, among others. Each role is tied to a specific function within the sentence, and recognizing these roles is essential for both syntactic and semantic analysis. Let’s explore these roles in more detail:
1. Agent
The Agent is the entity that performs or initiates an action. This is typically the subject of the sentence, though not always. In the sentence "The dog chased the ball," the dog is the Agent, as it is the one carrying out the action of chasing. The Agent typically embodies the “doer” of the action in a sentence.
2. Patient
The Patient is the entity that undergoes or receives the action. It is often the object of the verb in the sentence. In the sentence "The dog chased the ball," the ball is the Patient because it is the entity being chased. The Patient does not actively participate in the action but is affected by it.
3. Experiencer
The Experiencer is the entity that perceives or experiences the action or state, usually without directly influencing the action itself. This role is often associated with mental or emotional experiences. For example, in the sentence "John felt happy," John is the Experiencer, as he is experiencing the state of happiness. The distinction between Experiencer and Agent is subtle, but critical; an Agent is active, while an Experiencer is passive in relation to the action.
4. Theme
The Theme refers to the entity that is involved in or affected by the action but is typically a passive participant. It is the “what” or “who” that is being discussed or described. In the sentence "She read the book," the book is the Theme, as it is the entity being read. The Theme is often confused with the Patient, but while the Patient typically undergoes change as a result of the action, the Theme is primarily the subject matter of the action.
5. Goal
The Goal is the entity toward which an action is directed or the endpoint of an action. This is often seen in sentences involving movement or transfer. For instance, in "She gave the book to John," John is the Goal, as he is the recipient of the book. The Goal typically answers the question "to whom?" or "where?"
6. Instrument
The Instrument refers to the means by which an action is performed. It is the tool or object used to carry out the action. For example, in the sentence "She cut the paper with scissors," scissors are the Instrument. This role is important in identifying the tools or methods involved in actions.
7. Source
The Source indicates the origin of an action or the starting point of a movement or transfer. In the sentence "She walked from the park," the park is the Source, as it is the point of departure. The Source is often contrasted with the Goal, which represents the destination or endpoint.
8. Location
The Location refers to the place or environment where an action takes place. It is the spatial context within which the action occurs. For example, in the sentence "She studied at the library," the library is the Location.
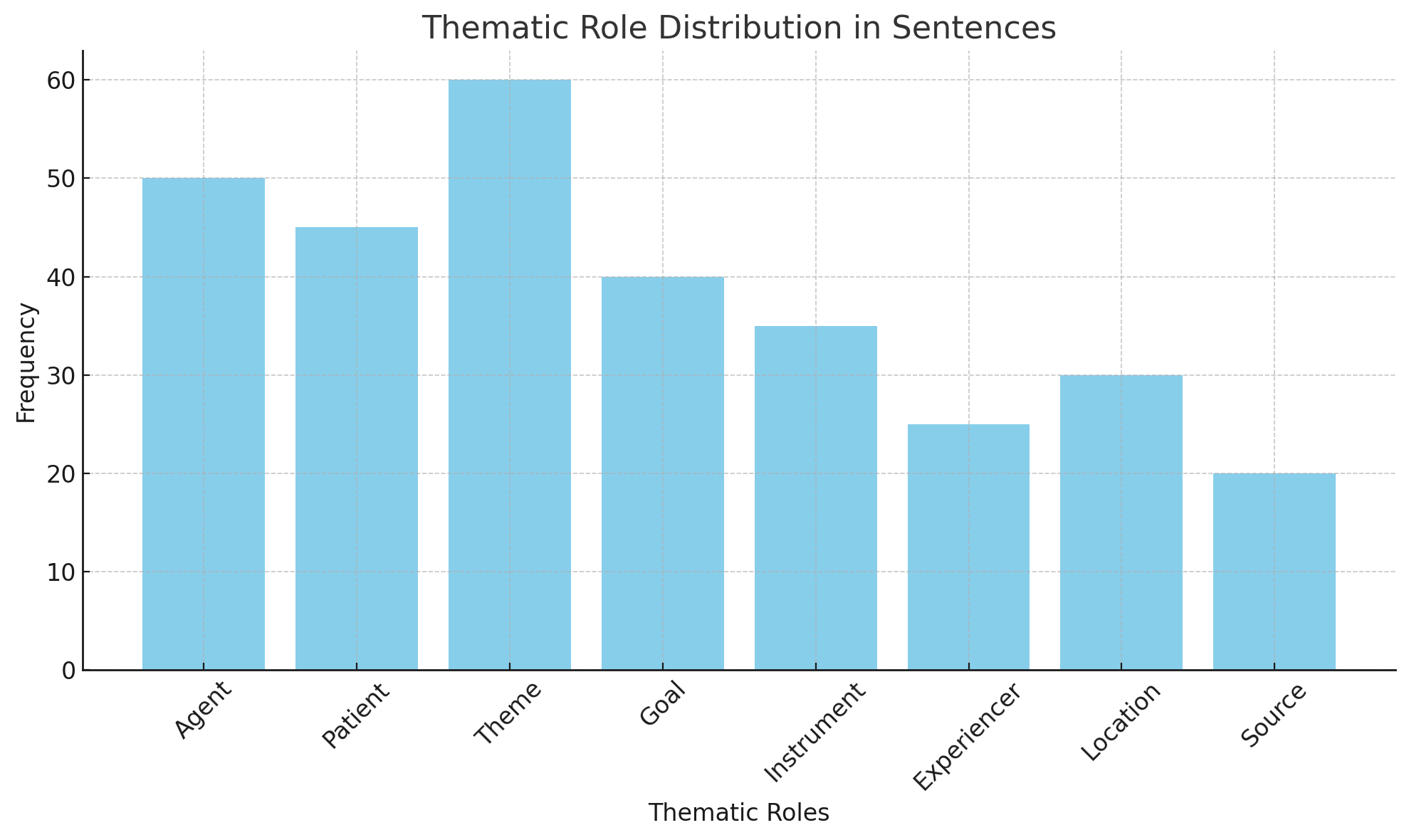
Examples of Thematic Roles in Sentences
To illustrate how these roles work in context, consider the following examples:
- Sentence 1: "John (Agent) sent a letter (Theme) to Mary (Goal)."
- In this case, John is the Agent, performing the action of sending. The letter is the Theme, as it is the object being sent, and Mary is the Goal, as she is the recipient of the letter.
- Sentence 2: "The teacher (Agent) taught the students (Experiencers) a lesson (Theme)."
- Here, the teacher is the Agent, performing the action of teaching. The students are the Experiencers, as they are the ones receiving the lesson. The lesson is the Theme, as it is the content being taught.
- Sentence 3: "She (Agent) cut the paper (Theme) with scissors (Instrument)."
- In this sentence, the Agent is the person performing the cutting action, the paper is the Theme, and the scissors are the Instrument used to carry out the action.
These examples demonstrate the variety of ways in which thematic roles can function within a sentence. Understanding these roles is not only important for linguistics but also crucial for computational models that aim to understand and generate natural language.
The Role of Thematic Roles in Sentence Structure
Thematic roles play a central role in sentence structure, as they dictate the relationships between different elements within a sentence. These relationships help define the meaning of the sentence and establish how the participants in an event or action interact with each other. For example, without the correct identification of thematic roles, the meaning of a sentence can become ambiguous or unclear.
Consider the following sentence: "The teacher gave the student a book."
- Without an understanding of thematic roles, a machine might struggle to correctly identify who is the giver and who is the recipient. However, once the thematic roles are identified—teacher as Agent, student as Goal, and book as Theme—the meaning becomes clear.
In large language models, understanding these relationships enables the model to better parse and understand sentence structures, ensuring more accurate predictions and more coherent responses. This ability to assign thematic roles is essential for tasks such as machine translation, text summarization, and information retrieval, where accurately understanding the roles of different elements in a sentence is crucial for delivering the intended meaning.
How LLMs Identify Thematic Roles
Large language models (LLMs), such as GPT, BERT, and T5, have revolutionized the field of natural language processing (NLP) by demonstrating an impressive ability to generate human-like text and understand complex language structures. One of the key aspects of understanding language involves the identification and interpretation of thematic roles—the roles that participants in a sentence play in relation to the action described. This section delves into how LLMs identify thematic roles, the mechanisms behind this identification, the challenges involved, and the advances that have been made in this area.
The Mechanisms Behind LLMs: Neural Networks and Attention
At the core of most large language models is a deep learning architecture known as the transformer model. Transformers use a mechanism called self-attention, which allows the model to weigh the importance of different words in a sentence relative to each other. This mechanism enables LLMs to capture long-range dependencies and relationships between words, which is crucial for tasks such as thematic role assignment.
When processing a sentence, the LLM breaks down the input into tokens (typically words or subword units), which are then passed through multiple layers of the model. Each token is embedded into a high-dimensional space that represents its semantic meaning. The self-attention mechanism then computes the relationships between all tokens, helping the model understand how words interact within the sentence and which words are most relevant to others.
For thematic role identification, the LLM must discern how different words in the sentence function within the action described by the verb. For example, in the sentence "John (Agent) gave a book (Theme) to Mary (Goal)," the model must recognize that "gave" is the main verb and that "John" is performing the action, while "book" is the object being transferred and "Mary" is the recipient.
The transformer’s self-attention mechanism is critical here, as it helps the model understand the syntactic and semantic relationships between the components of the sentence. These relationships enable the LLM to assign thematic roles to words based on their position in the sentence and their association with the main action.
Training on Large Datasets: Supervised and Unsupervised Learning
LLMs are typically trained on vast amounts of text data, which may include books, articles, websites, and other textual sources. This training process enables the model to learn the statistical patterns of language, including the assignment of thematic roles. There are two primary methods by which LLMs learn these patterns: supervised learning and unsupervised learning.
Supervised Learning
In supervised learning, LLMs are trained on datasets that contain labeled examples of thematic roles. For instance, in a corpus of sentences, each word or token is annotated with its corresponding thematic role (Agent, Patient, Goal, etc.). By learning from these labeled examples, the LLM can develop the ability to identify thematic roles in new, unseen sentences. Supervised learning typically requires a large, annotated corpus, which can be time-consuming and expensive to compile. However, it ensures that the model is explicitly taught how to identify thematic roles based on clear examples.
Unsupervised Learning
On the other hand, unsupervised learning allows LLMs to learn patterns in data without explicit annotations. Instead of relying on labeled training data, the model learns by processing vast amounts of text and recognizing patterns in how words tend to co-occur and interact in different contexts. Unsupervised learning methods, such as those used by transformer-based models, enable the LLM to infer underlying structures like thematic roles by identifying common word groupings and syntactic structures. Although unsupervised learning is less precise than supervised learning, it allows LLMs to generalize more broadly across different types of text without the need for extensive human annotation.
In practice, modern LLMs often use a combination of supervised and unsupervised learning, leveraging the strengths of both approaches. For example, models like GPT-3 are pre-trained in an unsupervised manner on massive text datasets, then fine-tuned using supervised methods on more specific tasks, including the identification of thematic roles.
Challenges in Thematic Role Identification
Despite the impressive capabilities of LLMs, identifying thematic roles accurately remains a challenging task, especially when dealing with complex or ambiguous sentences. Some of the key challenges include:
1. Ambiguity in Sentence Structure
Language is inherently ambiguous, and many sentences can be interpreted in multiple ways. For example, consider the sentence "The man saw the woman with a telescope." Here, it is unclear whether the man used a telescope to see the woman, or whether the woman had a telescope. Resolving such ambiguities requires the model to consider context, prior knowledge, and world knowledge, which can be difficult for LLMs to handle consistently.
2. Implicit Thematic Roles
Not all thematic roles are explicitly mentioned in every sentence. Some roles, such as the Agent or Patient, may be implied rather than directly stated. In the sentence "She opened the door," it is clear that "she" is the Agent and "door" is the Patient, but the sentence does not explicitly mention the action that caused the opening (such as “with a key”). LLMs must use contextual clues to infer these missing roles, which can be a difficult task in more complex situations.
3. Non-Canonical Word Order
Many LLMs, particularly those trained on English text, are optimized for canonical word order, where the subject generally precedes the verb, and the object follows the verb. However, many languages have non-canonical word orders, and even in English, variations such as passive constructions ("The book was read by John") or topicalization ("As for John, he gave a book to Mary") can disrupt the model’s ability to easily identify thematic roles.
4. Multiple Participants with Overlapping Roles
Some sentences feature multiple participants performing similar roles, which can confuse LLMs. For example, in the sentence "John and Mary (Agents) both gave a book (Theme) to Tom (Goal)," the LLM must correctly interpret both John and Mary as Agents. Without the ability to effectively resolve these multiple participants, the model might struggle to assign roles accurately.
Advances in Thematic Role Identification
Recent advancements in transformer models have improved the ability of LLMs to handle these challenges. One such advancement is the development of pre-trained models with contextual embeddings. These embeddings enable the model to consider the surrounding context when identifying thematic roles, helping to resolve ambiguities and improve accuracy in complex sentences.
Additionally, models like BERT have been trained using a masked language modeling technique, where parts of a sentence are hidden, and the model must predict the missing words based on the context. This technique improves the model's understanding of sentence structure and its ability to infer thematic roles even in sentences with incomplete information.
Furthermore, transfer learning has allowed LLMs to adapt more quickly to tasks involving thematic roles. By fine-tuning a pre-trained model on a specific task, such as thematic role labeling, the model can better learn how to assign roles in a wide variety of sentence structures.
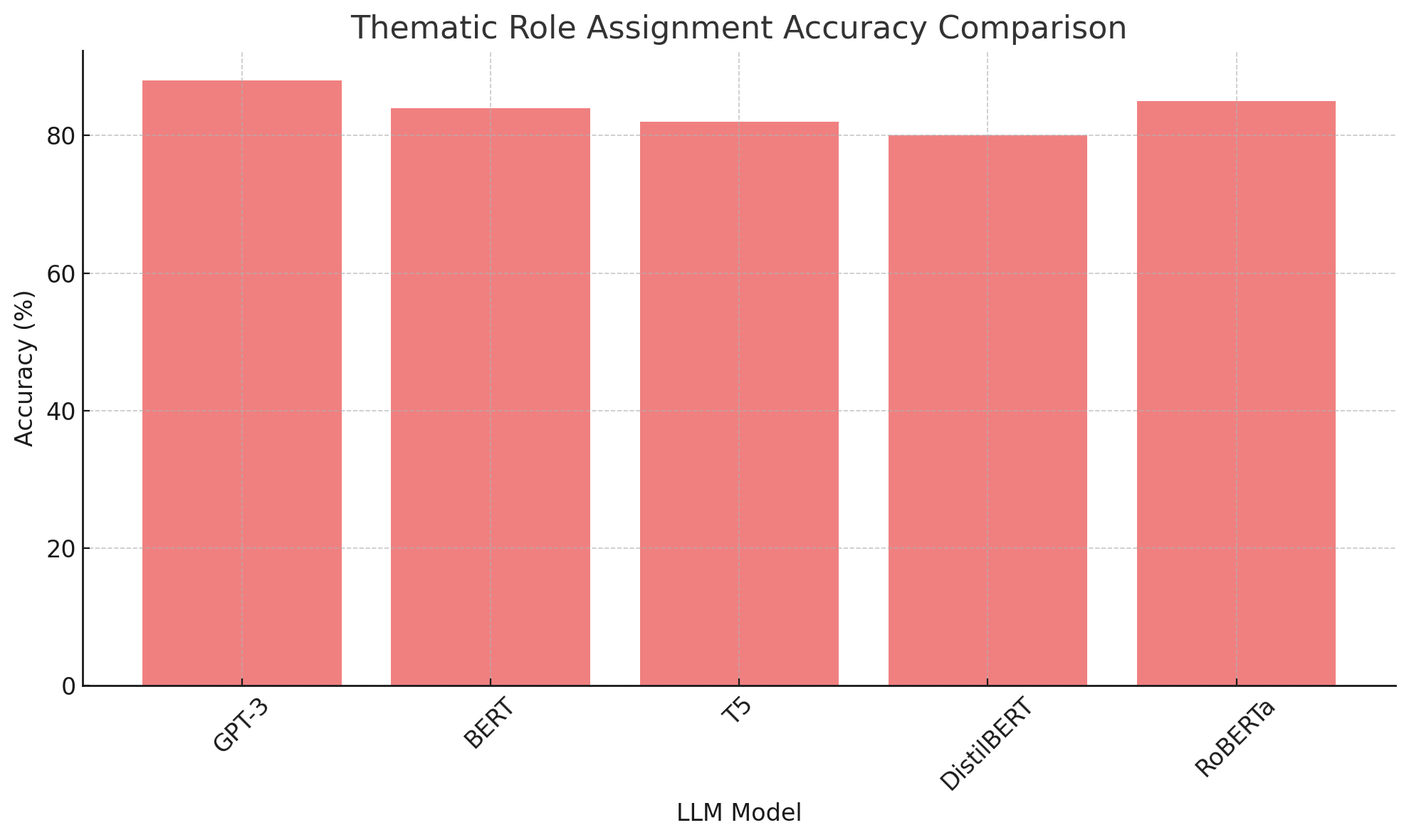
Practical Applications of Thematic Roles in LLMs
Thematic roles are not just a theoretical concept in linguistics; they have significant practical applications in the real world, particularly in the context of large language models (LLMs). The ability to correctly identify and assign thematic roles enhances the performance of LLMs in a wide array of natural language processing (NLP) tasks. From improving machine translation to enhancing content generation and automating complex information retrieval, thematic role identification plays a pivotal role in ensuring that LLMs accurately interpret and generate meaningful text. In this section, we explore several key areas where understanding and identifying thematic roles directly contribute to the effectiveness of LLMs in real-world applications.
Machine Translation
Machine translation (MT) has been one of the most transformative applications of LLMs in recent years. By enabling seamless communication across different languages, MT systems powered by LLMs have revolutionized industries such as e-commerce, customer support, and content localization. The accurate identification of thematic roles is crucial to ensuring that translations maintain the intended meaning, especially in languages with different syntactic structures.
In machine translation, understanding the thematic roles of sentence components allows LLMs to preserve the underlying relationships between words across languages. For instance, in the sentence "She gave him a book," the thematic roles of "She" as the Agent, "him" as the Recipient (or Goal), and "book" as the Theme need to be preserved when translating this sentence into languages with different word orders, such as Japanese or German. Without accurate role identification, a machine translation system might struggle to assign the correct roles to the words in the target language, leading to awkward or incorrect translations.
Thematic roles help LLMs maintain syntactic and semantic consistency in translated sentences, particularly in cases where word order differs between languages. In languages like German, where the verb is often placed at the end of a sentence, recognizing the roles of participants and their relationships to the verb becomes even more critical for preserving the sentence's meaning.
Sentiment Analysis and Opinion Mining
Sentiment analysis is the task of determining the emotional tone or opinion expressed in a piece of text, and it is widely used in applications ranging from brand monitoring to customer feedback analysis. Accurate sentiment analysis requires not only detecting words with positive or negative connotations but also understanding the roles played by different entities in the sentence. Thematic roles enable LLMs to differentiate between the subject expressing the sentiment and the object being commented on.
For example, in the sentence "I love the new iPhone," sentiment analysis would need to recognize that "I" is the experiencer of the emotion (Agent), while "the new iPhone" is the object of affection (Theme). This distinction is crucial, as it allows sentiment analysis models to correctly interpret the polarity of the sentence and to identify which entity the sentiment is directed toward.
Similarly, in opinion mining tasks, thematic role identification enables the LLM to extract relevant opinions about specific entities. In a sentence like "The customer criticized the service for being slow," understanding that "the customer" is the Agent (the one providing the criticism) and "the service" is the Patient (the entity being criticized) allows the LLM to accurately map the sentiment to the correct target.
Question Answering and Information Retrieval
In question answering (QA) systems and information retrieval, understanding thematic roles is essential for extracting precise and relevant answers from a given body of text. Whether the question is fact-based or open-ended, LLMs must identify the thematic roles of both the query and the answer to ensure that the response is contextually accurate and aligned with the user's intent.
For example, in a simple fact-based question like "Who wrote 'To Kill a Mockingbird'?" a QA system needs to recognize that "who" is the entity performing the action (Agent), and "To Kill a Mockingbird" is the Theme (the work being written). Understanding these thematic roles allows the system to identify the correct answer, "Harper Lee."
In more complex queries, such as "What is the impact of artificial intelligence on job markets?" thematic roles help LLMs identify the key entities—"artificial intelligence" (Agent), "impact" (Theme), and "job markets" (Goal). By recognizing the roles of these components, the model can search its knowledge base and provide a response that reflects a coherent and accurate understanding of the question's intent.
In information retrieval, thematic role identification enables LLMs to improve search results by matching queries with documents that contain relevant information about the correct entities and their relationships. For instance, a search query like "How did the company’s new product affect sales?" requires the system to correctly identify "company’s new product" as the Agent, "sales" as the Goal, and "affect" as the Action. This understanding helps retrieve documents that address the effects of the product on sales, as opposed to unrelated information.
Text Summarization
Text summarization is the process of reducing a long piece of text into a concise version that retains the essential information. Thematic role identification enhances the performance of both extractive and abstractive summarization systems by helping them identify the key participants and actions in the text. A model that understands thematic roles can extract or generate summaries that accurately reflect the main subjects, actions, and objects of the original content.
In an extractive summarization task, thematic roles enable the model to pick sentences that contain crucial actions and participants. For example, from a news article about a company launching a new product, the system needs to recognize that "company" is the Agent, "new product" is the Theme, and "launch" is the Action. It can then extract the most relevant sentences about these participants to create a coherent summary.
In abstractive summarization, where the system generates a new summary based on the original content, thematic roles guide the model in constructing sentences that maintain the relationships between the key elements of the text. For instance, when summarizing a scientific paper, the system needs to identify the Agent (the researcher), the Action (conducting an experiment), and the Theme (the hypothesis or subject being studied) to create a concise yet accurate summary.
Dialogue Systems and Conversational AI
In dialogue systems, such as chatbots or virtual assistants, understanding thematic roles allows the system to generate more natural and contextually appropriate responses. By identifying the roles of various entities in the conversation, LLMs can engage in a way that accurately reflects the ongoing dialogue and provides relevant responses.
For instance, in the dialogue exchange "User: Can you recommend a movie? Assistant: Sure! How about 'Inception'?" the assistant understands that "User" is the Agent, asking for a recommendation, and "Inception" is the Theme, being proposed as a movie. Identifying these roles helps the assistant determine the focus of the query (the user's request for a movie recommendation) and offer a suitable response.
Moreover, understanding thematic roles is essential for maintaining coherence in multi-turn conversations, where participants shift between different topics or tasks. By identifying the Agent, Patient, Theme, and other roles in ongoing exchanges, the system can track the flow of conversation and generate appropriate follow-up responses, ensuring the interaction feels natural and relevant.
Conclusion and Future Directions
Thematic roles are a critical element of natural language understanding, providing the structure necessary for large language models (LLMs) to interpret and generate meaning from text. By identifying and assigning roles such as Agent, Patient, Theme, Goal, and others, LLMs are better equipped to understand the underlying relationships between words, which in turn enhances their performance across a variety of natural language processing (NLP) tasks. The ability of LLMs to accurately assign these roles has profound implications for applications ranging from machine translation and sentiment analysis to question answering and dialogue systems. As the field of AI and NLP continues to advance, the role of thematic role identification will undoubtedly remain at the forefront of efforts to improve language models' performance and their ability to handle increasingly complex language structures.
Thematic roles not only support the syntactic and semantic analysis of language but also enable more intuitive and human-like interaction with machines. For example, in machine translation, understanding the thematic roles of sentence components ensures that translations maintain their intended meaning, even when word order or grammar structures differ between languages. In sentiment analysis, recognizing which entity the sentiment is directed toward is crucial for understanding customer feedback, social media posts, or product reviews. Similarly, in question answering and information retrieval, identifying thematic roles helps LLMs better understand user intent and provide more relevant, contextually appropriate answers.
However, despite the remarkable progress made in LLMs, there remain significant challenges in thematic role identification that need to be addressed. Ambiguities in sentence structure, implicit thematic roles, non-canonical word orders, and multiple participants performing overlapping roles can all complicate the task of correctly assigning thematic roles. As we have seen, many of these challenges stem from the inherent complexity of human language, which is often full of subtleties, contradictions, and contextual dependencies.
Challenges to Overcome
Although the use of deep learning models like transformers has significantly advanced the field, LLMs still struggle in certain contexts. One major challenge is the ambiguity that arises in natural language. Ambiguous sentences—such as "The man saw the woman with a telescope"—require models to rely heavily on context to disambiguate the roles of words. While LLMs can often resolve such ambiguities when provided with enough context, they still struggle with edge cases where the context is not explicit enough or when multiple plausible interpretations exist.
Another challenge lies in non-canonical sentence structures. While LLMs are generally trained on data with a subject-verb-object (SVO) structure, real-world language often presents sentences with different syntaxes, such as passive constructions ("The book was read by John") or topicalized phrases ("As for John, he gave a book to Mary"). These variations can confuse the model if it has not been adequately trained to handle them.
Moreover, many sentences contain implicit thematic roles—roles that are understood from context but are not explicitly stated in the sentence. For example, in a sentence like "She smiled," the subject "She" is understood to be the Experiencer of the action, but there is no direct object or other role mentioned. The challenge lies in having LLMs infer these missing roles from minimal or indirect cues.
Future Directions
As AI and NLP technologies continue to evolve, the future of thematic role identification in LLMs looks promising. Several avenues hold potential for improving the ability of LLMs to accurately assign and interpret thematic roles, leading to more effective and human-like language understanding.
1. Integration of World Knowledge and Contextual Awareness
One of the most promising directions for improvement is the integration of world knowledge and contextual awareness. Thematic roles often depend on external knowledge beyond the sentence itself. For example, understanding that a "doctor" is likely to be an Agent in a sentence about treating a patient requires access to general world knowledge. Incorporating large-scale knowledge bases, like Wikidata or conceptually rich databases, into LLMs could help resolve ambiguities and provide models with the broader context needed to interpret sentences more accurately.
Similarly, fine-tuning models to better incorporate contextual cues would allow them to adapt more dynamically to different sentence structures and contexts. As conversational agents and dialogue systems become more sophisticated, the ability to maintain context over extended dialogues will be essential for more accurate thematic role identification. Leveraging techniques such as long-term memory or contextual embeddings could improve the consistency of the model’s understanding across longer conversations or multi-turn interactions.
2. Multilingual and Cross-Lingual Thematic Role Recognition
Another important area for future development is the ability to identify and assign thematic roles across different languages. While English-based LLMs have shown substantial success, their performance is often less effective when applied to languages with distinct syntactic structures. Developing multilingual models that can automatically identify thematic roles in various languages—particularly those with non-SVO word orders, such as Japanese, Arabic, or Hindi—will be a key milestone. Cross-lingual thematic role recognition will enable LLMs to operate more effectively in a global context, making them valuable tools for translation, international customer support, and cross-cultural communication.
3. Enhanced Pre-training Techniques
The pre-training phase of LLM development will also continue to evolve. Recent advancements in transfer learning, where models are pre-trained on vast amounts of text data and then fine-tuned on specific tasks, have already contributed to significant gains in thematic role identification. Future pre-training techniques could focus more heavily on specific tasks related to thematic role identification, such as explicitly annotating thematic roles in large corpora or using reinforcement learning techniques to improve the model’s ability to assign roles in more complex sentence structures.
Another avenue for future research involves improving the understanding of implicit and missing roles in sentences. By incorporating techniques such as unsupervised learning or zero-shot learning, where models learn from data without explicit role annotations, LLMs could become better at inferring missing or implied thematic roles. This would improve their performance in situations where roles are left unstated but still crucial for understanding the action or event being described.
4. Addressing Ethical and Bias Concerns
As LLMs become more integrated into real-world applications, it is essential to address potential ethical concerns and biases related to thematic role assignment. LLMs trained on large, diverse datasets may inadvertently learn and perpetuate biases present in the data, such as gender stereotypes or racial biases. Ensuring that models assign thematic roles in a fair and unbiased manner will require ongoing research into bias detection and mitigation methods. Additionally, improving transparency in how LLMs make decisions about role assignment will be crucial for gaining trust and ensuring ethical AI practices.
Conclusion
Thematic roles are a crucial aspect of language understanding, serving as the foundation for many tasks in natural language processing. By enabling LLMs to accurately identify the roles that different participants play in a sentence, we are improving the model's ability to engage with language in a more human-like way. From machine translation and sentiment analysis to question answering and dialogue systems, the practical applications of thematic roles are vast and impactful.
As the field continues to advance, the challenges related to ambiguity, implicit roles, and non-canonical sentence structures will require innovative solutions. However, the integration of world knowledge, multilingual capabilities, and enhanced training techniques promises a future where LLMs are even more effective at understanding and generating natural language. With these advancements, we can expect LLMs to become more powerful tools for solving complex language tasks and driving forward the next wave of AI-powered applications.
References
- Introduction to Thematic Roles in Linguistics https://www.linguisticsociety.org/resource/thematic-roles
- Large Language Models and Natural Language Understanding https://www.ai-knowledge.com/large-language-models-nlu
- Transformers and Thematic Roles in NLP https://www.researchgate.net/publication/transformers-nlp-theory
- Understanding Thematic Roles and Their Application in Machine Translation https://www.language-tech.com/machine-translation-thematic-roles
- Sentiment Analysis and Its Importance in Modern AI Systems https://www.sentimentai.com/sentiment-analysis-importance
- The Future of Question Answering Systems https://www.qa-ai.com/future-of-question-answering
- Semantic Role Labeling for Text Summarization https://www.nltu-summarization.com/semantic-role-labeling
- Practical Applications of Large Language Models in NLP https://www.aiinsights.com/practical-applications-llms
- Challenges in Thematic Role Identification for LLMs https://www.research-papers-ai.com/thematic-role-identification
- The Role of Context in Language Model Performance https://www.ai-performance.com/contextual-language-models