Mastering AI Pilot Programs: Strategies for Scalable and Responsible Implementation
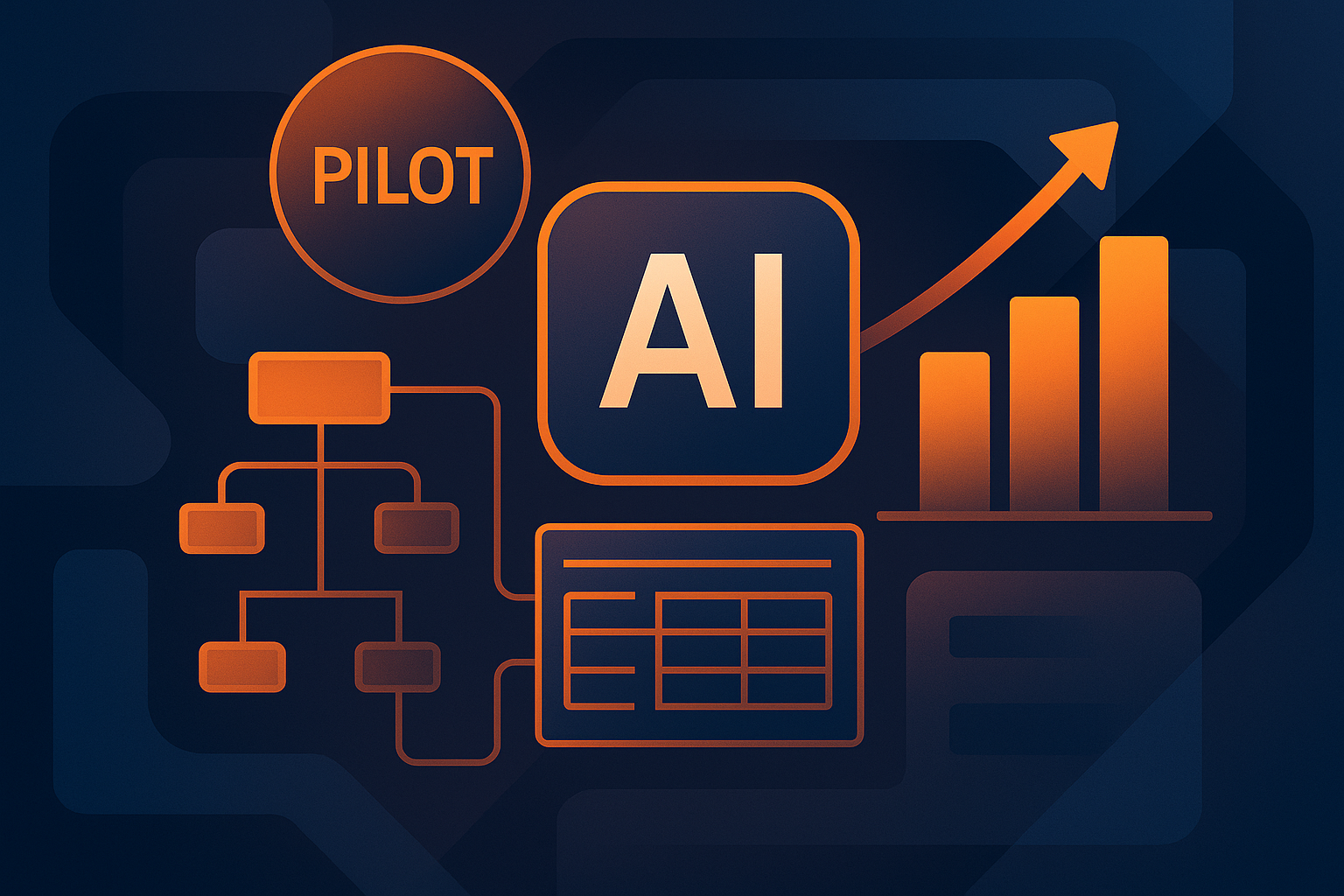
The integration of artificial intelligence (AI) into organizational workflows has evolved from experimental interest to strategic necessity. Across diverse industries—ranging from finance and manufacturing to healthcare and retail—AI is no longer a futuristic concept but a practical tool for enhancing efficiency, improving decision-making, and gaining competitive advantage. However, the path from theoretical promise to tangible business impact is fraught with complexity. Among the most critical yet often misunderstood phases of this journey is the pilot mode, a structured experimental deployment of AI systems intended to validate their effectiveness in controlled settings before wider rollout.
AI pilot projects function as a risk-mitigation mechanism and a crucial proving ground. They offer an opportunity to evaluate the technical, operational, and strategic feasibility of AI use cases within the unique context of an organization. Whether testing a chatbot to enhance customer service or implementing a predictive analytics tool for inventory management, pilot programs allow companies to gain insights, identify hidden constraints, and build confidence—without committing to full-scale deployment prematurely. Nevertheless, the success rate of AI pilots remains inconsistent. Many initiatives stall due to vague objectives, insufficient data readiness, poor stakeholder engagement, or lack of a cohesive strategy for scaling.
As AI systems become more capable and customizable, so too do the expectations from businesses looking to deploy them. Yet this heightened capability introduces new risks. Unlike traditional software pilots, AI pilots must grapple with issues such as algorithmic bias, interpretability, and ongoing learning from dynamic data streams. Moreover, deploying AI in pilot mode involves interdisciplinary collaboration, bridging gaps between data scientists, business strategists, operations personnel, and compliance teams. The multifaceted nature of AI technologies makes their pilot testing both more important and more complex than conventional software development pilots.
A well-executed AI pilot is not merely a proof-of-concept exercise; it is a foundational phase that should deliver measurable insights into the AI system’s utility, readiness for scale, and alignment with broader organizational goals. Pilot mode should enable enterprises to test technical feasibility and refine business logic while simultaneously identifying gaps in data infrastructure, workflows, and human oversight. Crucially, it must include feedback mechanisms to inform iteration, ensure regulatory alignment, and foster end-user trust. Unfortunately, in practice, AI pilots often fall short of their intended purpose. According to multiple industry surveys, fewer than 30% of AI pilot initiatives successfully transition into production environments, highlighting the need for a strategic, methodical approach.
This blog aims to demystify the AI pilot mode and provide a comprehensive roadmap for its successful implementation. Drawing from industry best practices, real-world case studies, and enterprise AI research, the following sections will explore the key components required to navigate this critical stage effectively. From selecting the right use cases and ensuring data preparedness to building human-in-the-loop workflows and evaluating pilot outcomes, each phase demands rigorous planning and stakeholder alignment. Additionally, we will examine the underlying infrastructure and change management strategies that enable a seamless transition from pilot to production.
Through this lens, AI pilot mode is best understood not as a binary test of success or failure, but as a strategic learning cycle—a structured process for experimentation, evaluation, and refinement. Organizations that treat AI pilots as iterative, measurable, and aligned with business objectives are more likely to realize long-term value from their AI investments. The stakes are high: as AI becomes integral to digital transformation, the capability to execute and scale AI pilots effectively will increasingly differentiate industry leaders from laggards.
In the sections that follow, we will provide an in-depth analysis of the essential elements required for a successful AI pilot. These include defining precise objectives, ensuring data and infrastructure readiness, engaging human expertise throughout the process, establishing clear evaluation criteria, and securing organizational alignment. Together, these strategies form the backbone of an AI pilot model that not only validates innovation but sets the stage for scalable success.
Establishing a Clear Business Objective and Use Case
One of the most common pitfalls in AI pilot mode implementation is the absence of a clearly defined business objective. While the allure of adopting artificial intelligence is strong—fueled by promises of operational efficiency, predictive insights, and enhanced customer engagement—success is largely contingent upon anchoring the pilot to specific, measurable goals. A pilot that lacks a strategic purpose is prone to misalignment, resource waste, and eventual abandonment. Conversely, a well-scoped pilot, grounded in concrete business needs, sets the foundation for technical validation, stakeholder buy-in, and future scalability.
Aligning AI Pilots with Strategic Business Goals
The starting point for any successful AI pilot is understanding the why behind the initiative. Organizations must begin by articulating the broader business strategy that the pilot is intended to support. Whether the objective is to reduce customer churn, improve fraud detection accuracy, optimize supply chain operations, or enhance employee productivity, the AI pilot must serve as a means to a quantifiable end. This alignment ensures that the pilot is not perceived as a speculative experiment, but as a deliberate investment with clearly defined return-on-investment (ROI) parameters.
To this end, stakeholders must ask:
- What problem are we trying to solve?
- How will success be measured?
- What will the organization do differently if the pilot succeeds?
- Who benefits from the AI solution, and how?
By answering these questions early, teams can avoid the trap of deploying AI for AI’s sake—a mistake that often leads to pilots that are technologically interesting but operationally irrelevant.
Criteria for Selecting an Appropriate Use Case
Selecting the right use case is a balancing act between feasibility and impact. An ideal use case for AI pilot mode should meet the following criteria:
- Business Relevance: The use case should address a high-priority pain point or opportunity within the organization. This increases internal motivation to support and act upon the pilot results.
- Data Availability: AI systems require substantial volumes of relevant, clean, and labeled data. Pilots should be scoped to areas where this data already exists or can be gathered with minimal friction.
- Operational Containment: Pilot initiatives should be designed to operate within well-defined boundaries—such as a single team, department, or customer segment—to limit exposure and manage risk.
- Measurable Outcomes: The impact of the AI intervention should be measurable via clear KPIs or business metrics (e.g., accuracy improvement, cost reduction, processing time, customer satisfaction).
- Stakeholder Engagement: The use case should involve stakeholders who are committed to collaborating throughout the pilot, including business leads, data owners, technical teams, and end-users.
Common categories of pilot use cases include:
- Customer Support: Chatbots or AI assistants for automating FAQ responses or triaging support tickets.
- Finance and Risk: Predictive models for credit scoring, fraud detection, or expense categorization.
- Operations: Forecasting tools for inventory planning, demand prediction, or logistics optimization.
- Human Resources: Talent acquisition models for screening resumes or predicting employee turnover.
It is critical to avoid overly ambitious use cases in the pilot phase. While transformative applications are attractive, early pilots should focus on “low-hanging fruit” that can demonstrate tangible results within a 3–6 month timeframe. This allows organizations to test hypotheses, iterate quickly, and build confidence in the AI capabilities before scaling up to more complex or mission-critical domains.
Cross-Functional Collaboration in Use Case Definition
AI pilots should not be developed in isolation by the data science team. The most successful pilots are the result of cross-functional collaboration, where different parts of the organization come together to define the use case, establish goals, and ensure operational alignment. These typically include:
- Business Stakeholders: Define desired outcomes and ensure alignment with corporate strategy.
- Data Teams: Evaluate data availability, quality, and readiness for training and testing models.
- IT and Engineering: Ensure infrastructure compatibility and address integration points.
- Compliance and Legal: Review ethical, regulatory, and privacy considerations.
- End-Users: Provide feedback on the usability and impact of the AI system.
Such collaboration not only ensures a holistic understanding of the pilot’s requirements but also fosters ownership and trust—two elements that are indispensable when transitioning from pilot to production.
Setting Success Metrics and Baselines
Before the pilot begins, teams must establish what success looks like. These success metrics—often referred to as key performance indicators (KPIs)—should be quantitative, achievable, and time-bound. Depending on the use case, these may include:
- Model accuracy, precision, recall, or F1 score
- Time reduction for specific tasks
- Reduction in manual errors
- Improvement in customer satisfaction scores
- Cost savings or revenue growth
- Percentage increase in process automation
Baseline metrics must also be recorded, providing a benchmark against which to compare post-pilot performance. Without baselines, teams cannot definitively determine whether the AI system introduced any measurable improvement.
Equally important is defining thresholds for minimum viable success. This is not the ideal or aspirational target, but the minimum level of performance at which the AI solution would be deemed viable for further investment. This avoids the pitfall of continuing with a solution that offers negligible or negative returns.
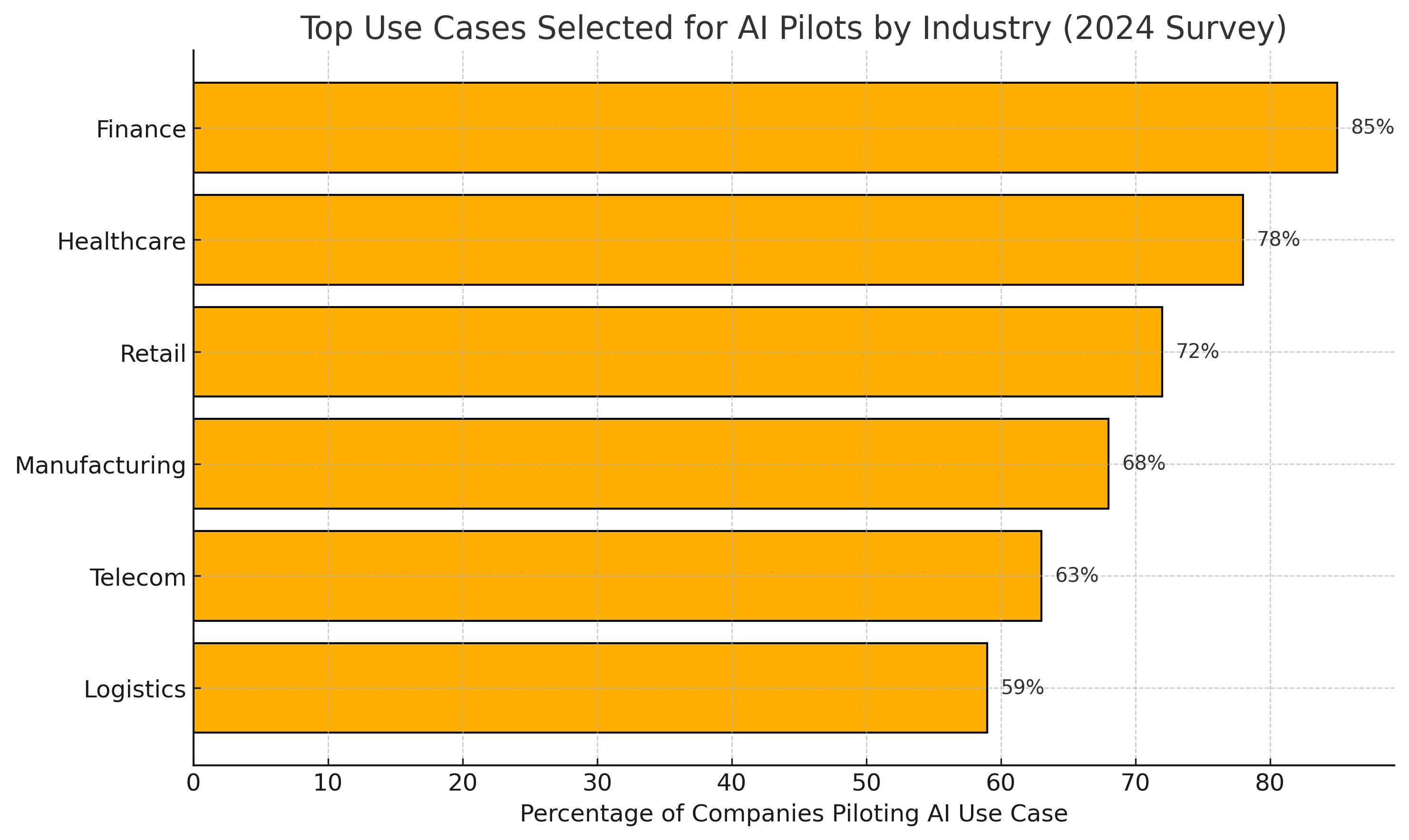
Establishing a clear business objective and selecting a suitable use case are not merely administrative steps—they are the strategic bedrock upon which the success of the AI pilot rests. Without this clarity, even the most advanced AI models can become disconnected from business value. By selecting a use case that is relevant, measurable, and achievable—and ensuring cross-functional alignment from the outset—organizations can lay the groundwork for an AI pilot that not only functions but flourishes.
Data Readiness and Infrastructure Considerations
The backbone of any successful AI pilot initiative lies not only in the strength of its algorithms but in the readiness of its data and supporting infrastructure. Despite the sophistication of contemporary machine learning models, their performance is only as robust as the data used to train and validate them. Moreover, the underlying infrastructure must be capable of handling the demands of AI workloads, including storage, processing power, integration capabilities, and security. Failure to prepare either domain adequately can compromise pilot outcomes, delay timelines, and erode stakeholder confidence.
This section explores the essential data and infrastructure elements that organizations must assess and address before and during AI pilot implementation. From ensuring data quality and governance compliance to leveraging scalable infrastructure and automated pipelines, preparation in these areas is not optional—it is foundational.
Assessing Data Quality and Availability
Data is the lifeblood of AI, and pilot projects are particularly sensitive to the quality, completeness, and relevance of the datasets they rely on. Before initiating any AI pilot, organizations must conduct a thorough audit of their data assets to answer key questions:
- Is the necessary data available, accessible, and well-structured?
- Are the datasets representative of the problem being addressed?
- Are there significant gaps, noise, or inconsistencies?
- Is the data annotated or labeled, where necessary, for supervised learning?
Poor data quality can render even the most advanced models ineffective. As such, data cleansing, normalization, and augmentation should be factored into the pilot's preparation phase. In many cases, data from disparate systems must be integrated, which can expose issues of duplication, latency, or misalignment in data schema.
Furthermore, data lineage—the ability to trace data’s origin and transformation history—is vital for transparency and auditability, especially in regulated industries. Pilots that do not maintain a clear view of data provenance often encounter trust and compliance issues that hinder production deployment.
Ensuring Data Governance, Privacy, and Compliance
In an era of heightened regulatory scrutiny, particularly surrounding data protection and ethical AI, data governance is a non-negotiable consideration in AI pilots. Enterprises must institute governance frameworks that define roles, responsibilities, policies, and procedures related to data usage. This includes:
- Ensuring adherence to regulations like GDPR, HIPAA, or CCPA
- Establishing data access controls and user permission levels
- Encrypting data both in transit and at rest
- Conducting data anonymization or pseudonymization, where applicable
- Maintaining audit trails for data queries and model predictions
Equally important is the concept of model accountability. Pilot initiatives should be capable of demonstrating how input data influences model decisions. Explainability mechanisms such as SHAP values, LIME, or saliency maps should be integrated early in pilot development, especially for models that may be used in high-stakes domains such as finance, healthcare, or legal advisory.
These practices not only ensure legal compliance but also cultivate trust with internal and external stakeholders, paving the way for smoother transitions into production environments.
Infrastructure: The Engine Behind Scalable Pilots
Beyond data, AI pilots demand robust and flexible technical infrastructure to manage model training, inference, deployment, and monitoring. Many pilot failures can be traced back to bottlenecks in compute resources, outdated architecture, or lack of integration capabilities. Key infrastructure considerations include:
- Cloud vs. On-Premises Deployment: Cloud infrastructure, such as AWS, Azure, or Google Cloud, offers scalability, elasticity, and access to managed services. For sensitive data or latency-critical applications, hybrid or on-premise solutions may be more appropriate.
- Data Storage and Retrieval: AI models require rapid access to large datasets. Using scalable and query-optimized storage systems—like data lakes, NoSQL databases, or distributed file systems—can accelerate pilot experimentation and testing.
- Processing Power: GPU and TPU acceleration may be necessary for training deep learning models. Pilot teams must assess whether their current compute environment can support parallel processing or whether they need to provision specialized hardware.
- DevOps and MLOps Integration: The adoption of Machine Learning Operations (MLOps) principles is essential even at the pilot stage. Tools such as MLflow, Kubeflow, or Amazon SageMaker help manage version control, automated testing, model registry, and deployment pipelines—ensuring that pilots are reproducible and scalable.
- API Integration: Pilots often need to interact with existing business systems such as CRMs, ERPs, or customer interfaces. Ensuring API compatibility and secure endpoints accelerates deployment and facilitates real-time inference.
Building a Scalable Testing Environment
AI pilot mode should not be viewed as a disposable testing phase but as a prelude to scalable implementation. Accordingly, organizations should adopt design principles that support modularity, reusability, and extensibility. These include:
- Containerization: Using containers (e.g., Docker) allows AI models and their dependencies to run consistently across different environments.
- CI/CD Pipelines: Establishing continuous integration and continuous deployment pipelines enables rapid iteration and controlled experimentation.
- Monitoring and Logging: Tracking model performance metrics, input/output data drift, and system errors during the pilot stage helps preempt scalability issues.
By establishing a resilient architecture upfront, enterprises significantly reduce the friction of moving from pilot to production. Moreover, the pilot becomes a learning platform that contributes to institutional knowledge and operational maturity.
Data readiness and infrastructure preparedness are not peripheral concerns—they are central determinants of AI pilot success. Regardless of the ambition or innovation behind a pilot project, it will fail to deliver meaningful insights or impact without structured, accessible data and a robust, scalable technical foundation. By proactively addressing data quality, governance, privacy, and computational infrastructure, organizations enhance the credibility, reliability, and longevity of their AI pilot initiatives. Moreover, these preparations accelerate the path to production, enabling enterprises to realize AI's transformative potential at scale.
Human-in-the-Loop Design and Feedback Integration
While the narrative surrounding artificial intelligence often emphasizes automation and independence, the most resilient and effective AI systems, particularly in pilot mode, are those that incorporate human oversight, judgment, and feedback. Human-in-the-loop (HITL) design is a methodology that deliberately integrates human input at critical stages of an AI system’s lifecycle—data labeling, model training, output validation, and continuous monitoring. In the context of AI pilot mode, HITL serves not only as a safeguard against errors but as a strategic asset for enhancing model accuracy, contextual understanding, and user trust.
This section examines the strategic rationale for embedding human interaction in AI pilot workflows, the operational models used to implement HITL systems, and the broader implications for organizational learning and trust building.
Why Human-in-the-Loop Matters in Pilot Mode
AI models—especially those based on machine learning and deep learning architectures—rely heavily on patterns derived from historical data. While this makes them powerful predictors and classifiers, it also renders them susceptible to various risks, including bias, overfitting, misinterpretation of edge cases, and unintended consequences. These vulnerabilities are particularly pronounced during the pilot phase when models are still being validated against real-world use cases.
By integrating human oversight, organizations can:
- Ensure Quality Control: Human reviewers can validate AI outputs, catch false positives or negatives, and correct errors that automated systems may overlook.
- Refine Model Behavior: Feedback loops from domain experts allow for real-time adjustments, especially in dynamic environments or ambiguous contexts.
- Maintain Compliance and Ethics: Human governance helps ensure AI decisions align with legal, ethical, and social norms, particularly in regulated sectors.
- Increase Stakeholder Confidence: Active human involvement demonstrates responsible AI practices and fosters trust among users and decision-makers.
The presence of humans in the decision-making loop acts as a buffer against unforeseen failures while also accelerating the model’s journey from experimental to production-ready.
Designing Effective HITL Workflows
Successful implementation of HITL within AI pilots requires more than simply assigning personnel to review outputs. It demands a structured design, where roles, responsibilities, and escalation protocols are clearly defined. Typical HITL workflows in pilot mode include the following components:
- Pre-Processing Stage:
- Human Role: Curating, cleaning, and labeling data.
- Tools: Annotation platforms like Labelbox, Prodigy, or Snorkel.
- Goal: Enhance training dataset quality and reduce bias in inputs.
- Model Inference Stage:
- Human Role: Reviewing real-time or batch predictions to assess accuracy and relevance.
- Use Cases: Reviewing flagged content, triaging support tickets, validating medical diagnostics.
- Goal: Intervene before incorrect or harmful outputs reach end-users.
- Post-Processing Stage:
- Human Role: Analyzing performance metrics, investigating anomalies, and suggesting retraining strategies.
- Collaboration: Data scientists, domain experts, compliance officers.
- Goal: Inform model retraining and continuous improvement.
- Feedback Loop Integration:
- Human Role: Rating model outputs, tagging corner cases, identifying unmet needs.
- Mechanism: In-app prompts, review panels, periodic evaluation sessions.
- Goal: Feed actionable insights into the next pilot iteration.
For instance, in a customer service AI pilot, agents might supervise chatbot responses during live sessions, correct misclassifications, and tag unrecognized intents. These interventions then inform retraining data for improved performance in subsequent cycles.
Leveraging Domain Experts for Contextual Intelligence
One of the most underutilized assets in AI pilot mode is the knowledge of subject matter experts (SMEs). Unlike generalist engineers or data scientists, SMEs bring deep contextual awareness that helps AI systems navigate ambiguity, nuance, and exceptions—areas where purely statistical models may falter.
Examples include:
- Radiologists verifying medical image diagnoses generated by AI
- Financial analysts validating risk assessments from fraud detection models
- Legal professionals interpreting sentiment analysis in compliance-related documents
Embedding SMEs in the pilot process ensures that the AI system does not merely deliver high-confidence predictions, but contextually appropriate and actionable outputs. Moreover, their involvement often reveals business logic errors, misaligned objectives, or data misinterpretations that could go unnoticed in purely technical evaluations.
Human Feedback as a Model Optimization Mechanism
AI systems thrive on data, but not all data is created equal. Human-generated feedback offers a rich source of high-signal input that accelerates model refinement. Unlike passive data collection, intentional human feedback is goal-directed and often highlights failure modes, rare events, and model blind spots.
Pilot teams should actively solicit and structure feedback in ways that maximize its value:
- Explicit Feedback: Direct evaluations, corrections, or ratings provided through dashboards or review tools.
- Implicit Feedback: Behavioral signals such as user corrections, response rejections, or re-routing decisions.
- Quantitative Feedback: Structured surveys, scoring systems, and agreement rates.
- Qualitative Feedback: Open-ended comments, interview transcripts, or annotated case studies.
For optimal effectiveness, this feedback must be logged, prioritized, and mapped to specific model adjustments. Modern MLOps pipelines should include mechanisms to integrate human feedback as labeled training data or to guide hyperparameter tuning.
Building Organizational Trust Through HITL
Beyond technical benefits, HITL serves as a trust-building mechanism across multiple organizational levels. In many cases, employees view AI systems with skepticism, fearing job displacement, dehumanization of processes, or loss of professional judgment. By explicitly embedding humans in the AI lifecycle, organizations can shift this narrative.
Some strategies for fostering trust through HITL include:
- Transparent Communication: Clarify the scope of AI systems and how human oversight is built-in.
- Role Augmentation, Not Replacement: Emphasize that AI assists, rather than replaces, human expertise.
- Training Programs: Equip employees with the skills to interpret AI outputs and provide meaningful input.
- Governance Inclusion: Involve frontline workers and SMEs in defining ethical guidelines and escalation policies.
When stakeholders across the organization see that their input is valued and that AI decisions are not final but reviewable, they are more likely to embrace the pilot and support its evolution.
Human-in-the-loop design is not a limitation of AI systems; it is a strategic advantage—especially during pilot mode. It ensures not only higher model fidelity but also fosters collaboration, contextual intelligence, and ethical integrity. By structuring HITL workflows, leveraging domain expertise, and systematically collecting feedback, organizations create AI pilots that are not only technically sound but socially and operationally robust. As the line between automation and augmentation continues to blur, the organizations that integrate humans into the AI loop from the outset will be best positioned to scale AI in a way that is trustworthy, efficient, and impactful.
Pilot Evaluation, Iteration, and Scaling Readiness
The transition from a controlled pilot to a fully integrated AI system is neither automatic nor guaranteed. Many promising AI pilots falter at this stage due to lack of robust evaluation, insufficient iteration, or premature scaling. To prevent these pitfalls, organizations must implement a rigorous, multi-dimensional framework for evaluating pilot outcomes, iteratively refining their approach, and planning for scale. This process ensures that only viable, value-generating solutions are promoted into production environments, while others are either recalibrated or consciously retired.
This section explores the key considerations in evaluating AI pilot performance, the mechanisms for iteration based on pilot learnings, and the strategic planning necessary to scale successfully.
Establishing Clear Evaluation Metrics
The foundation of effective pilot evaluation lies in predefined success metrics that align with both business objectives and technical feasibility. These metrics must be agreed upon by all relevant stakeholders—executives, data scientists, business users, and compliance officers—before the pilot begins.
Evaluation metrics typically fall into four categories:
Technical Performance:
- Model accuracy, precision, recall, F1-score
- False positive and false negative rates
- Latency and throughput for real-time systems
Business Impact:
- Cost savings or revenue improvement
- Reduction in cycle time or error rates
- Increased customer satisfaction (e.g., NPS, CSAT)
Operational Fit:
- Ease of integration with existing systems
- Alignment with current workflows
- Training and reskilling requirements for employees
Regulatory and Ethical Compliance:
- Transparency and explainability
- Adherence to data privacy laws and internal policies
- Human oversight and audit trail availability
Importantly, evaluation should be conducted continuously throughout the pilot, not only at the end. This allows teams to identify and address issues proactively, rather than retroactively. Dashboards, real-time alerts, and regular check-ins are essential tools in maintaining visibility throughout the pilot lifecycle.
Pilot Review: Lessons from Success and Failure
A successful pilot is not defined solely by favorable metrics; it is also characterized by the depth of insight gained into technical and organizational readiness. Equally, a pilot that fails to meet its targets should not be automatically deemed unsuccessful. Often, these so-called "failures" provide critical feedback that informs future design and de-risks long-term strategy.
Effective pilot reviews should address questions such as:
- Were the objectives clear and realistic?
- Did the model behave as expected in different scenarios?
- What technical or process bottlenecks emerged?
- Did the human-in-the-loop framework function effectively?
- How did stakeholders perceive the pilot’s value?
These reviews should be formalized into a post-pilot assessment report that includes qualitative and quantitative insights. Organizations may use this report to determine one of three next steps:
- Proceed to Production: The pilot met success criteria and requires scaling.
- Iterate: Minor adjustments are needed before a second pilot cycle.
- Retire: The use case is deemed non-viable due to irreconcilable issues.
Making these decisions with clarity and documentation prevents resource drain and ensures that future AI initiatives are grounded in lessons learned.
Iteration: The Engine of AI Pilot Improvement
AI pilots, by their experimental nature, should be iterative and adaptive. Rarely does the first version of a model fully meet all performance, usability, and integration expectations. Structured iteration allows teams to systematically improve the system while still operating within a controlled environment.
Areas commonly addressed during iteration include:
- Data Enrichment: Incorporating new or corrected data to reduce model bias or enhance coverage.
- Model Tuning: Adjusting hyperparameters, switching architectures, or retraining with updated data.
- User Interface Enhancements: Modifying how model outputs are presented to end-users.
- Workflow Integration: Adjusting the AI system’s role within business processes to better match human workflows.
- Security and Compliance: Reinforcing safeguards, expanding audit logging, or refining consent mechanisms.
Each iteration cycle should be time-bound and goal-oriented. Agile methodologies, such as sprint reviews and retrospective meetings, are effective for managing the cadence of iteration and ensuring that refinements are targeted and impactful.
Readiness to Scale: Technical and Organizational Prerequisites
Once a pilot has demonstrated its value, the next logical step is scaling—replicating or expanding the AI system across departments, geographies, or customer segments. However, scaling without readiness can jeopardize performance and erode stakeholder confidence. Therefore, organizations must conduct a scaling readiness assessment, evaluating the following key dimensions:
Model Robustness:
- Is the model performant across diverse datasets and conditions?
- Can it handle increased data volume or complexity?
Infrastructure Scalability:
- Are cloud and on-premise systems capable of supporting scaled deployment?
- Are APIs, data pipelines, and compute environments production-grade?
Governance and Risk Management:
- Are mechanisms in place to monitor drift, bias, and performance degradation at scale?
- Are legal and compliance requirements addressed for wider deployment?
Stakeholder Alignment:
- Is there buy-in from leadership, business units, and IT?
- Are there resources allocated for scaling, including budget and personnel?
Training and Change Management:
- Are end-users equipped to interact with the AI system?
- Are support structures, documentation, and escalation paths established?
Without proper scaling readiness, the strengths of a pilot can become liabilities in production. As such, organizations must plan for scale from the pilot's inception, ensuring architectural and procedural flexibility.
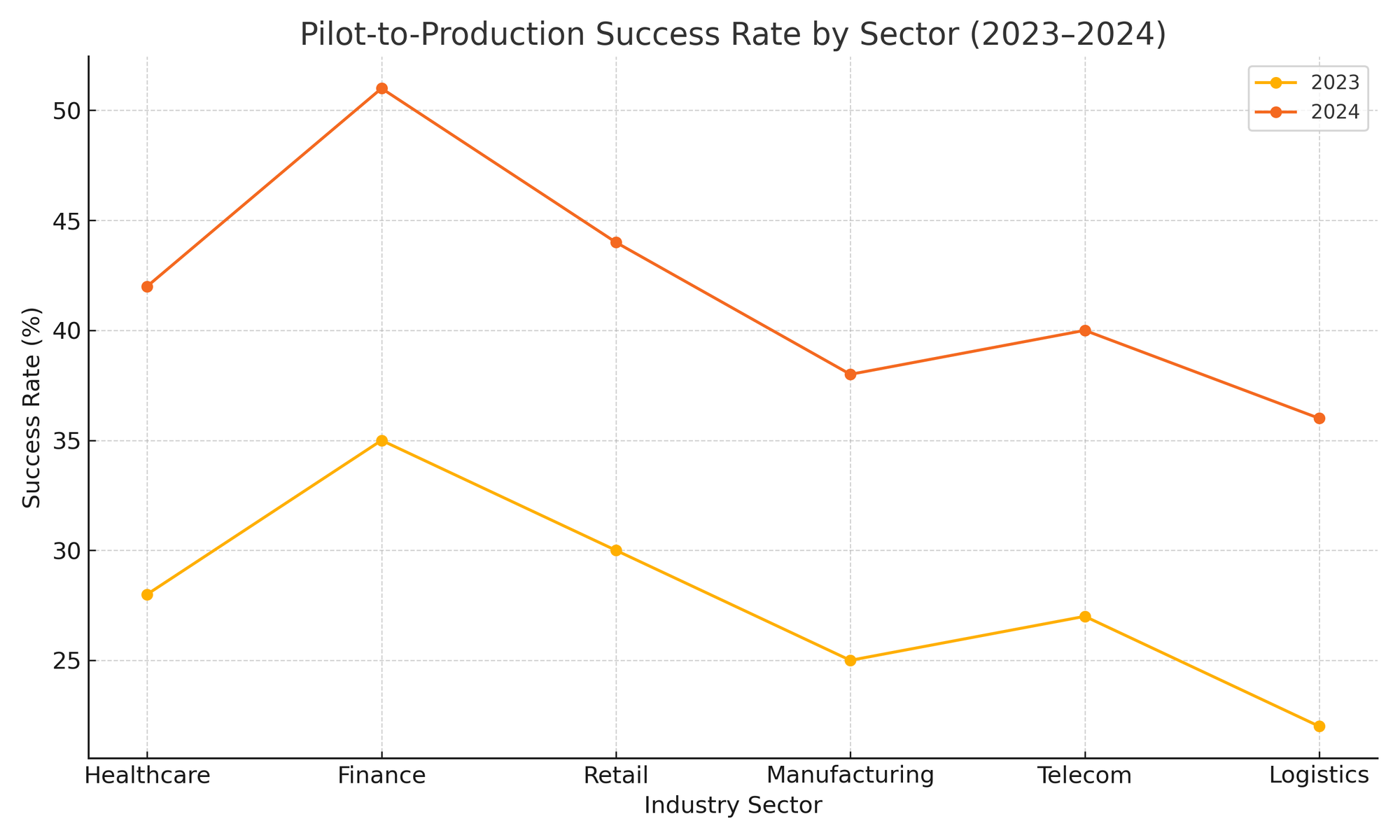
Pilot evaluation, iteration, and scaling readiness are not sequential checkboxes—they form an interconnected feedback loop that distinguishes transient AI experimentation from sustainable transformation. By defining precise evaluation metrics, embracing a culture of structured iteration, and building the technical and organizational capacity to scale, enterprises can convert pilot insights into production success. Ultimately, the ability to translate AI innovation into real-world value hinges not just on experimentation, but on disciplined execution and strategic foresight.
Organizational Alignment and Change Management
As technical and infrastructural elements of an AI pilot evolve, the often-overlooked but equally critical dimension of success lies in organizational alignment and change management. No AI initiative, no matter how advanced or accurate, can deliver transformative value without active support from the humans who plan, manage, and interact with the system. In pilot mode, aligning organizational goals, workflows, and cultures is as vital as aligning data and algorithms. Change management, in this context, is the strategic orchestration of people, processes, and priorities to foster AI adoption and mitigate resistance.
This section delves into the foundational elements of organizational alignment, effective change management practices, and the cultural shifts necessary to ensure AI pilot initiatives thrive and scale successfully.
Aligning Leadership and Stakeholder Expectations
The journey toward AI maturity begins at the top. Executive alignment is fundamental to securing funding, cross-departmental collaboration, and long-term integration pathways for AI initiatives. Leaders must articulate a clear vision for the AI pilot, including its business relevance, anticipated impact, and boundaries of experimentation.
Key strategies to secure executive and stakeholder alignment include:
- Vision Communication: Present the AI pilot not merely as a technical trial but as a strategic enabler of business transformation.
- Tangible Value Framing: Link the pilot’s goals to key performance indicators that matter to each stakeholder group (e.g., efficiency gains, cost savings, improved compliance).
- Transparent Governance: Establish decision-making committees or steering groups that oversee progress, resolve conflicts, and adjust direction when needed.
Equally important is managing expectations regarding what AI can and cannot do. Unrealistic beliefs about autonomy or precision often lead to disillusionment when pilot systems encounter inevitable limitations. Leaders must set a tone of measured optimism, reinforcing that AI pilots are experimental, iterative, and subject to refinement.
Cross-Functional Coordination
AI pilots span multiple disciplines—data science, engineering, operations, legal, compliance, and user experience. Effective execution requires cross-functional coordination to align objectives, share resources, and break down silos that impede progress.
Tactics for enhancing coordination include:
- Integrated Teams: Create pilot teams composed of representatives from all key functions, each with defined roles and collaborative responsibilities.
- Agile Ceremonies: Employ agile methodologies such as daily standups, sprint planning, and retrospectives to foster real-time communication.
- Knowledge Repositories: Maintain shared documentation platforms (e.g., Confluence, Notion, or SharePoint) where learnings, metrics, and feedback are continuously updated.
In practice, one of the most common coordination failures arises when data scientists build models in isolation from business teams, only to find their outputs misaligned with operational workflows or compliance requirements. Coordinated pilot teams prevent such disconnects and accelerate time-to-insight.
Addressing Employee Concerns and Building Trust
AI systems often trigger anxiety among employees, who may perceive automation as a threat to job security, autonomy, or identity. Ignoring these concerns can create cultural resistance, undermine pilot adoption, and sabotage broader AI strategy. Addressing such sentiments is not simply an HR function—it is a strategic imperative.
Organizations must actively manage employee concerns through:
- Education and Transparency: Conduct workshops and briefings that explain the AI system’s purpose, scope, and impact.
- Inclusion and Participation: Involve employees in testing, feedback, and governance activities to foster ownership and relevance.
- Role Augmentation Narratives: Highlight how AI will enhance human capabilities rather than replace them, such as by offloading repetitive tasks or enhancing decision support.
Moreover, deploying AI ethics guidelines and publishing internal AI use charters can strengthen the perception of responsible innovation. These documents should outline principles such as fairness, accountability, transparency, and non-maleficence, reinforcing that employee well-being is a core consideration.
Training and Upskilling for AI Readiness
Even the most intuitive AI system requires a level of fluency from its users to achieve optimal performance. AI pilots often introduce new processes, interfaces, and decision-making paradigms that differ from traditional methods. Hence, preparing employees through structured learning interventions is crucial.
Training efforts during pilot mode should be tailored by user group:
- End Users: Train on how to interpret AI outputs, flag anomalies, and understand the system’s limitations.
- Managers: Educate on oversight practices, performance evaluation metrics, and change facilitation.
- Technical Teams: Provide advanced training on model explainability, debugging, and system integration.
Digital learning platforms, live demonstrations, simulation environments, and job shadowing are effective mechanisms for delivering training. Upskilling should be framed not only as a requirement but as a career growth opportunity that future-proofs employee capabilities in an AI-driven economy.
Change Management Frameworks for AI Pilots
Successful AI pilots are as much about people as they are about technology. Therefore, leveraging formal change management frameworks can provide structure and consistency during pilot deployment. Two commonly used models include:
ADKAR Model (Awareness, Desire, Knowledge, Ability, Reinforcement):
- Raise awareness of the AI initiative.
- Cultivate desire to support it.
- Provide knowledge to engage with it.
- Build ability through hands-on learning.
- Reinforce changes with recognition and follow-up.
Kotter’s 8-Step Change Model:
- Create urgency.
- Form a powerful coalition.
- Develop a vision and strategy.
- Communicate the vision.
- Remove obstacles.
- Generate short-term wins.
- Consolidate gains.
- Anchor new approaches in the culture.
By applying such frameworks, organizations can navigate the emotional, behavioral, and procedural changes that AI pilots often introduce. Change leaders should be visible, accessible, and equipped with the authority to respond quickly to resistance or confusion.
Fostering a Culture of Experimentation and Innovation
At a higher level, AI pilot success is closely tied to organizational culture. Companies that embrace experimentation, tolerate failure, and encourage innovation are better positioned to capitalize on the iterative nature of AI pilots.
Cultural attributes that support AI adoption include:
- Psychological Safety: Employees feel safe to critique models, suggest improvements, or question assumptions.
- Learning Orientation: Pilot results are viewed as data points, not verdicts, and failures are treated as sources of insight.
- Recognition Systems: Teams and individuals who contribute to pilot development and refinement are celebrated and rewarded.
Leaders can foster this culture by modeling openness to feedback, de-stigmatizing pilot failure, and celebrating learning outcomes—even when they reveal limitations or require significant rework.
Organizational alignment and change management are not peripheral to AI pilot success—they are essential enablers. Without coordinated leadership, cross-functional teamwork, trust-building, and structured adaptation, even technically robust pilots can collapse under the weight of cultural inertia. By addressing human factors proactively, training users effectively, and embedding change management practices from the outset, organizations pave the way for AI pilots that are embraced, sustained, and scaled. As enterprises continue to experiment with AI technologies, those that invest in people—not just platforms—will lead the next wave of responsible and impactful AI transformation.
Conclusion
As organizations accelerate their pursuit of artificial intelligence to drive efficiency, innovation, and competitive differentiation, the pilot phase has emerged as a critical juncture in the AI adoption lifecycle. Far from being a preliminary or experimental exercise, AI pilot mode represents a structured opportunity to validate technical viability, measure strategic impact, and establish foundational capabilities for scalable deployment. The success of this phase is not determined by model complexity or novelty alone, but by the convergence of business alignment, data readiness, human-centered design, rigorous evaluation, and cultural adaptability.
This blog has outlined the essential strategies required to navigate AI pilot mode effectively:
- First, aligning pilot efforts with clear business objectives and selecting a feasible, high-impact use case ensures relevance and accountability from the outset.
- Second, ensuring data integrity and infrastructure readiness—both technical and regulatory—is fundamental to pilot credibility and performance.
- Third, integrating human-in-the-loop design principles enhances accuracy, contextual intelligence, and stakeholder trust, thereby reinforcing the model’s real-world utility.
- Fourth, establishing robust evaluation criteria and embracing iterative refinement enables organizations to learn quickly, adapt responsibly, and determine true readiness for scale.
- Fifth, organizational alignment and change management are indispensable for securing buy-in, minimizing resistance, and cultivating a workforce that is equipped and motivated to collaborate with AI systems.
The cumulative effect of these strategies is a resilient AI pilot model—one that treats experimentation not as a gamble, but as a methodical, measurable step toward enterprise transformation.
It is also important to view AI pilot initiatives through the lens of long-term value creation. Too often, pilots are evaluated in isolation, without regard to their potential to shape enterprise AI architecture, data governance protocols, and cross-functional collaboration models. When executed with foresight and discipline, even modest pilot projects can produce lasting institutional knowledge, process innovation, and cultural momentum that pave the way for broader AI integration.
Equally, organizations must resist the temptation to scale prematurely. Scaling AI without appropriate evaluation, user readiness, or infrastructural maturity can magnify risks and undermine stakeholder confidence. A cautious, iterative approach—guided by continuous feedback and evidence-based refinement—will always outperform impulsive deployment.
Ultimately, the hallmark of a successful AI pilot is not merely the generation of impressive metrics, but the activation of learning, trust, and alignment across the organization. As AI capabilities evolve, so too must the frameworks through which businesses test, adopt, and govern these systems. The pilot phase, when handled strategically, becomes the fulcrum that balances innovation with responsibility, and ambition with sustainability.
In a business landscape increasingly shaped by intelligent systems, those enterprises that learn how to pilot AI with purpose, precision, and people at the center will not only achieve technological success—they will lead the future of digital transformation.
References
- McKinsey on AI Transformation – https://www.mckinsey.com/capabilities/mckinsey-digital
- Microsoft Azure AI Guide – https://azure.microsoft.com/en-us/solutions/ai
- Gartner: AI Strategy Insights – https://www.gartner.com/en/information-technology/insights/artificial-intelligence
- IBM: AI Maturity Model – https://www.ibm.com/artificial-intelligence
- AWS AI & ML Services – https://aws.amazon.com/machine-learning
- Deloitte AI Institute – https://www2.deloitte.com/us/en/pages/deloitte-analytics/articles/artificial-intelligence.html
- Towards Data Science: AI Use Cases – https://towardsdatascience.com
- World Economic Forum on AI Ethics – https://www.weforum.org/agenda/archive/artificial-intelligence