Is AI Powering Innovation or an Energy Crisis?
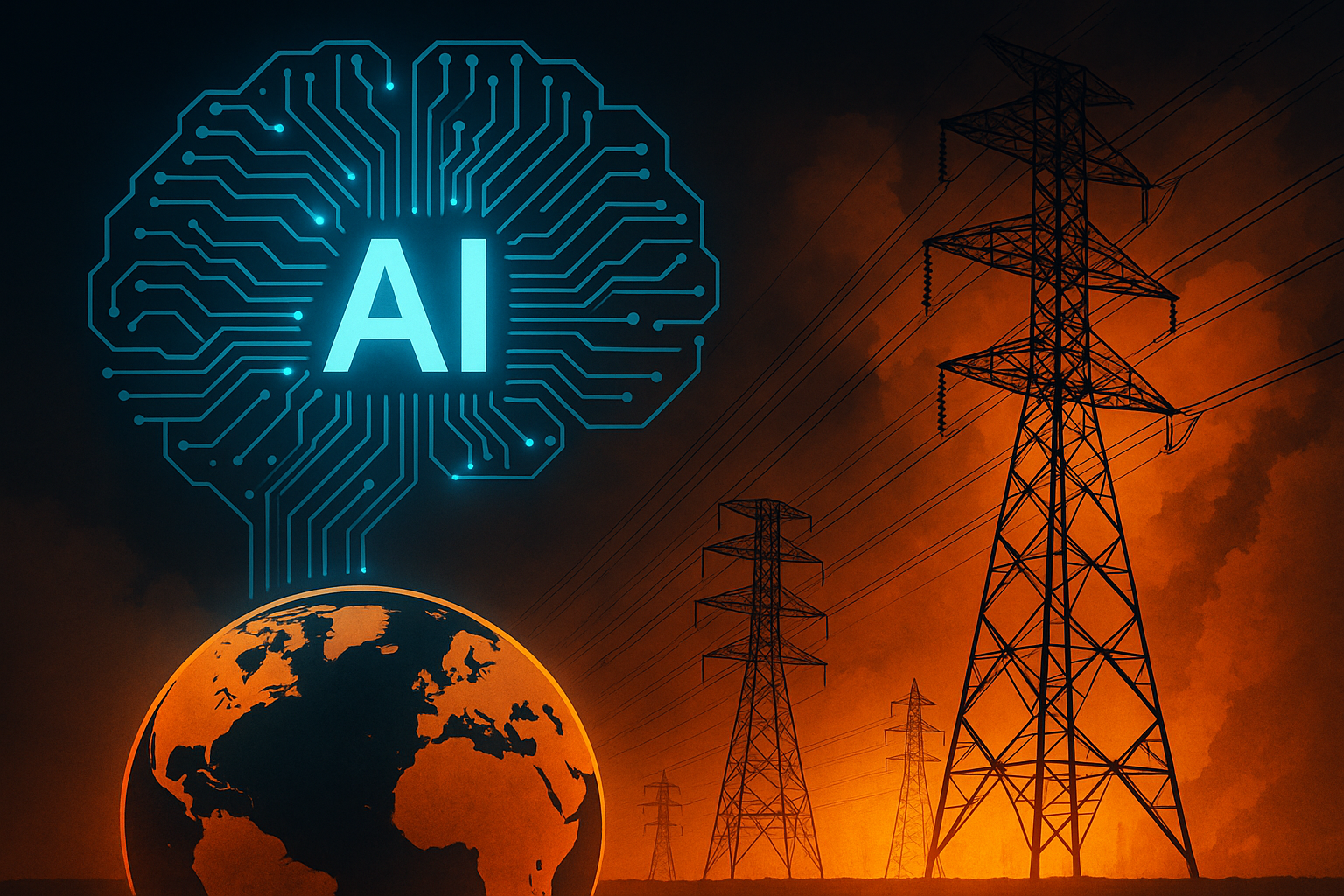
In recent years, the global technology landscape has undergone a seismic transformation driven by the rapid evolution and adoption of artificial intelligence (AI). From conversational agents like ChatGPT and Claude to advanced vision-language models powering robotics, AI has permeated virtually every industry. With this acceleration, however, comes an often-overlooked consequence: a dramatic surge in energy consumption. The training and operation of large-scale AI models require immense computational resources, typically delivered through energy-intensive data centers and specialized hardware such as GPUs and TPUs. As AI capabilities grow, so too does the demand for electricity — raising an increasingly urgent question: could the AI boom fuel a global energy crisis?
While AI promises productivity gains, economic growth, and scientific breakthroughs, it also introduces a paradox. On one hand, AI can optimize energy systems, forecast power usage, and facilitate the integration of renewables. On the other, the very operation of large models requires vast amounts of power, challenging the sustainability of their growth. The energy demands are not limited to model training alone; inference — the real-time application of AI models — occurs at massive scale across cloud servers, smart devices, and embedded systems. With enterprises and governments rushing to embrace AI, the infrastructure needed to support this transformation is rapidly expanding. Data centers, which house the backbone of AI computation, are now one of the fastest-growing electricity consumers worldwide.
Already, countries such as the United States, Ireland, and Singapore have raised alarms about the burden AI-powered data centers place on local grids. At the same time, the carbon footprint of AI, though difficult to quantify precisely, is believed to rival or exceed that of many traditional industrial processes. The pursuit of artificial general intelligence (AGI) and the deployment of foundation models with hundreds of billions of parameters suggest that energy consumption will continue its steep trajectory unless mitigated by technological or policy intervention.
This blog post explores whether the AI boom is pushing humanity toward a tipping point in energy sustainability. It examines the energy footprint of AI technologies, the stress they place on regional and global power infrastructures, the potential of renewables and efficiency gains to offset their consumption, and the long-term implications for global energy markets. By synthesizing technical data, industry trends, and policy developments, we aim to provide a comprehensive perspective on whether AI is on a collision course with the energy sector — or whether it could paradoxically become a catalyst for solving one of the most pressing challenges of our time.
The AI Energy Footprint – A Growing Concern
The widespread integration of artificial intelligence (AI) into modern infrastructure has precipitated a significant and often underestimated consequence: exponential growth in energy consumption. As AI systems become more powerful, particularly in the domains of large language models (LLMs), image generation, and multimodal learning, the computational resources required to develop and maintain these systems have scaled dramatically. This section provides an in-depth examination of how AI’s energy demands are escalating, explores the components driving that demand, and contextualizes the broader implications for sustainability.
The Computational Architecture Behind AI Energy Consumption
At the core of the AI energy footprint is the training and inference of large models. Training refers to the process of optimizing neural network parameters using vast datasets, a phase that can take weeks or months on thousands of GPU units operating continuously. Inference, on the other hand, refers to the real-time deployment of these models—whether generating text, analyzing video feeds, or synthesizing speech—and is often conducted across millions of user sessions daily.
The energy requirements of training state-of-the-art models are staggering. For example, the training of GPT-3, developed by OpenAI, is estimated to have consumed approximately 1.3 gigawatt-hours (GWh) of electricity—comparable to the annual energy consumption of over 120 U.S. households. With models like GPT-4, Google DeepMind’s Gemini, and Anthropic’s Claude continuing to scale in size and complexity, the energy footprint is expanding at an unprecedented rate. In parallel, inference workloads, though less energy-intensive on a per-query basis, occur at such a massive scale that their cumulative impact is immense. Millions of users interact with generative AI tools daily, making inference potentially even more consequential than training in the long run.
Hardware Intensity: GPUs, TPUs, and the Data Center Effect
A major factor in the AI energy equation is the underlying hardware. Most modern AI workloads are run on energy-hungry accelerators such as NVIDIA’s A100 or H100 GPUs, or Google’s custom-built TPUs. These chips are designed for high-throughput matrix operations but also generate substantial heat, necessitating robust cooling systems that further increase power usage.
Data centers housing these chips have proliferated globally to meet demand. These facilities not only require vast quantities of electricity to run AI hardware but also depend on cooling infrastructure to maintain optimal operating temperatures. According to the International Energy Agency (IEA), global data centers consumed around 460 terawatt-hours (TWh) in 2022, with AI and cryptocurrency mining contributing a significant share. Forecasts indicate that, without intervention, data center power demand could exceed 1,000 TWh by 2030—more than doubling in less than a decade.
The geographical distribution of AI infrastructure has led to localized power strains. Regions such as Northern Virginia, which hosts the highest concentration of data centers in the world, and areas in Ireland and the Netherlands, have reported rising energy stress and infrastructure bottlenecks due to AI-related compute loads. These localized effects hint at a broader pattern: the AI boom is not only a technological shift but also an infrastructural one, requiring continuous upgrades to national and regional electricity grids.
Energy Per Query: The Hidden Cost of Everyday AI Use
Another dimension of AI’s energy footprint is the energy required per user interaction or query. While early digital queries—such as traditional search engine lookups—consume relatively little energy, generative AI queries can be orders of magnitude more intensive. For instance, a single ChatGPT prompt can consume nearly 10 times more energy than a conventional Google search. The disparity becomes even more pronounced with tasks involving image generation, code synthesis, or real-time video analysis.
This seemingly trivial cost per interaction becomes significant when scaled to millions or billions of queries per day. OpenAI’s ChatGPT, Google’s Bard (now Gemini), and similar services are integrated into productivity tools, search engines, and mobile apps—substantially increasing the frequency of compute-intensive interactions. The result is an expanding “digital carbon shadow,” wherein user-facing convenience masks a deepening energy impact.
Benchmarking and Transparency Challenges
Despite the mounting concern, measuring the precise energy consumption of AI systems remains difficult. Few AI providers disclose the full lifecycle energy cost of their models. Metrics such as training FLOPs (floating point operations), PUE (power usage effectiveness), and data center emissions are not consistently reported or regulated. This lack of transparency hinders public understanding and policy formulation.
Nonetheless, some benchmarking efforts have been made. The MLCommons organization has introduced benchmarks for measuring energy and performance efficiency across AI workloads. Initiatives like Hugging Face’s "carbon emissions tracker" also attempt to estimate CO₂ footprints based on model architecture and training time. However, these are still voluntary and fragmented across providers, pointing to an urgent need for standardized energy reporting in AI development.
The Environmental Cost of Scale
As AI models increase in size—moving from millions to billions or even trillions of parameters—the marginal improvements in performance often come at disproportionate energy costs. The law of diminishing returns applies: doubling a model's size may result in only modest improvements in accuracy or fluency while doubling or tripling energy use. This raises important questions about the sustainability of the “bigger is better” paradigm in AI.
Moreover, many state-of-the-art models are trained multiple times as part of hyperparameter tuning and reinforcement learning processes. Fine-tuning for different languages, tasks, or regions further multiplies the total energy consumption. Companies developing open-source or domain-specific versions of foundational models may repeat these processes across multiple environments, adding to the cumulative impact.
Externalities and Global Inequities
AI’s energy demands also raise concerns about environmental externalities and global equity. Data centers are often located in developed economies where power infrastructure is resilient and cloud providers have preferential access to low-cost electricity. However, the environmental costs—such as carbon emissions and water usage for cooling—are often borne by local communities. In regions facing water scarcity or grid instability, the expansion of AI infrastructure can exacerbate existing socio-environmental tensions.
Additionally, nations with less access to high-performance compute (HPC) infrastructure are effectively locked out of the generative AI race, widening global inequality. As energy becomes a prerequisite for participation in the AI economy, the digital divide risks becoming an energy divide as well.
An Unsustainable Trajectory Without Mitigation
Without a concerted push toward energy efficiency and renewable integration, the trajectory of AI development appears unsustainable. The drive for faster, smarter, and more capable AI systems is fundamentally at odds with the pressing need to decarbonize global energy systems. In the worst-case scenario, AI’s energy appetite could undermine progress toward climate targets set by the Paris Agreement and national emissions reduction plans.
Yet, there is also reason for cautious optimism. Several leading AI developers are exploring low-power model architectures, neuromorphic computing, and other innovations to reduce energy use. Furthermore, the growing public and regulatory scrutiny around digital sustainability may compel organizations to prioritize greener practices in AI deployment.
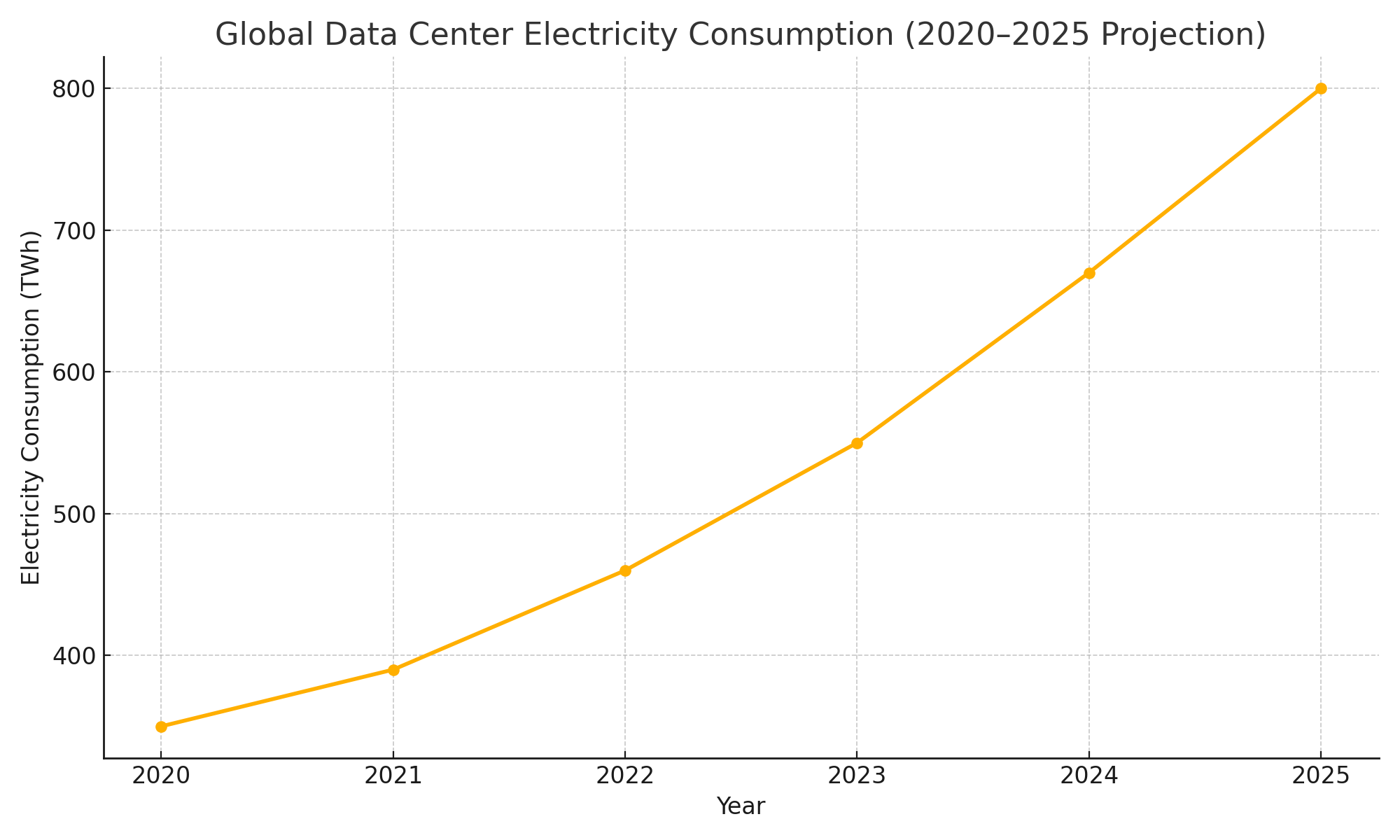
Infrastructure Strain and Regional Energy Implications
The unprecedented demand for artificial intelligence (AI) compute power has begun to exert pressure not only on global energy resources but also on national and regional infrastructure systems. The proliferation of AI-capable data centers, combined with the electrification of other sectors such as transportation and manufacturing, is accelerating the strain on electrical grids worldwide. As AI applications scale from experimental to ubiquitous, the implications for infrastructure readiness and regional stability have become impossible to ignore.
This section explores the impact of AI on energy infrastructure, the uneven distribution of strain across global regions, and the potential geopolitical and economic consequences of inadequate planning. It also examines governmental responses and introduces a comparative framework to understand how different regions are preparing—or failing to prepare—for the energy implications of AI proliferation.
Localized Infrastructure Overload
While AI is a global phenomenon, its physical footprint is often highly localized. Data centers tend to cluster in areas offering favorable tax regimes, high-speed fiber connectivity, abundant land, and most importantly, access to reliable and inexpensive electricity. These factors have created digital “hot zones” in regions like Northern Virginia in the United States, Frankfurt in Germany, Dublin in Ireland, and parts of Eastern China.
In Northern Virginia alone, home to the so-called “Data Center Alley,” data center electricity usage is projected to surpass 2,000 megawatts by 2026—more than twice the consumption of the entire city of Washington, D.C. This rapid growth has prompted Dominion Energy, the local utility, to delay new construction approvals due to concerns about overloading the regional grid. Similarly, in Ireland, the national grid operator EirGrid has placed a moratorium on new data center connections in the Dublin region to prevent blackouts and ensure service reliability for residential and industrial users.
These decisions underscore a sobering reality: many regions are ill-equipped to support the explosive growth in AI-driven compute. Electrical grids, originally designed for static industrial use and predictable residential demand, are now being tested by dynamic, 24/7 loads driven by high-performance AI infrastructure.
Grid Resilience and Blackout Risk
The challenge of grid resilience is further complicated by the volatility of renewable energy sources. As many AI companies attempt to “green” their operations by shifting to solar, wind, or hydroelectric power, they also inherit the intermittency issues of those sources. Cloud providers must either overbuild renewable capacity or invest in energy storage solutions to maintain consistent availability—both of which are capital-intensive and logistically complex.
In California, for instance, several AI-focused data centers operate in parallel with the state’s aggressive renewable targets. However, record-breaking heatwaves in recent years have led to energy rationing, brownouts, and emergency alerts to avoid total grid collapse. If AI data centers are not load-balanced effectively, their round-the-clock demand could exacerbate such crises.
Likewise, in Texas, the Electric Reliability Council of Texas (ERCOT) has faced challenges in integrating massive new compute workloads into an already-fragile grid. The state’s independent grid, while offering regulatory flexibility for data center development, has been exposed during extreme weather events, revealing systemic vulnerabilities that AI expansion may amplify.
Energy and Water Interdependencies
Another dimension of infrastructure strain lies in the dual reliance on electricity and water. Most AI data centers use evaporative cooling systems, which consume significant quantities of water to dissipate heat from densely packed processors. This approach is particularly problematic in drought-prone areas where water is already a scarce resource.
For example, in Mesa, Arizona—home to multiple new data center construction projects—residents and officials have raised concerns about the sustainability of water-intensive cooling methods. A single hyperscale data center can consume millions of gallons of water per day, leading to potential conflicts with agricultural and municipal needs. In regions like the Middle East and parts of Africa, where water scarcity is a national security concern, the trade-offs become even more critical.
Regional Preparedness and Strategic Disparities
Not all regions are equally equipped to absorb the infrastructural demands of AI. While some countries have proactively invested in smart grid technologies, high-voltage transmission lines, and energy storage, others are lagging behind. The disparity in infrastructure readiness creates a digital divide not just in terms of AI development capacity but also in economic competitiveness and energy security.
The United States has seen fragmented responses due to its decentralized energy governance. While states like Virginia, California, and Texas have taken steps to manage data center loads, there is no national policy coordinating AI infrastructure development with grid expansion.
The European Union, through its Digital Decade initiative and Green Deal, has sought to align AI growth with carbon neutrality goals. However, member states vary widely in their infrastructure maturity. Germany and the Netherlands have faced significant opposition from local governments over land and energy use for AI infrastructure.
China, by contrast, has taken a more centralized approach. The government has designated “national computing hubs” and implemented energy quotas for data centers. These policies allow China to balance growth with infrastructural control, though transparency remains an issue.
The Middle East, particularly countries like the United Arab Emirates and Saudi Arabia, are positioning themselves as AI powerhouses by building purpose-driven smart cities like NEOM. These projects are designed with integrated energy infrastructure in mind, leveraging desalination and solar energy to address both electricity and water needs. However, their scalability and sustainability remain under scrutiny.
Public and Political Backlash
The growing awareness of infrastructure strain has led to rising public resistance against the unchecked expansion of AI-related facilities. In Ireland, local communities have protested new data center developments, citing energy hoarding, housing shortages due to real estate acquisition, and noise pollution from cooling systems.
Similarly, in parts of the United States, civic organizations have pushed back against data center sprawl, calling for environmental impact assessments and greater transparency in utility contracts. Politicians are increasingly being pressured to balance economic development with sustainability, leading to a patchwork of new regulations that may affect AI deployment speed.
In some jurisdictions, discussions are emerging about introducing “compute taxes” or “energy caps” on large AI operators to deter overconsumption and redistribute resources. While such measures remain controversial, they signal a shift in how governments may attempt to regulate AI through energy policy rather than through data or algorithmic constraints.
The Need for Policy Harmonization
The current policy environment is highly reactive. In most cases, regulations are being formulated only after infrastructure stress becomes visible. There is an urgent need for forward-looking, harmonized strategies that treat AI as a critical infrastructure domain—on par with transportation, telecommunications, and defense.
Key policy priorities should include:
- National compute inventories and demand forecasting
- Mandatory energy impact disclosures for large-scale AI projects
- Incentives for colocating AI infrastructure with renewable energy generation
- Investments in smart grids and microgrid architectures
- AI-specific environmental permitting procedures
Without such measures, the growth of AI could outpace the ability of infrastructure to support it, leading to grid instability, resource conflicts, and delays in broader digital transformation goals.
Comparative Analysis of Regional Readiness
To synthesize the global landscape, the following table provides a comparative snapshot of major regions based on their AI compute growth and infrastructure readiness:
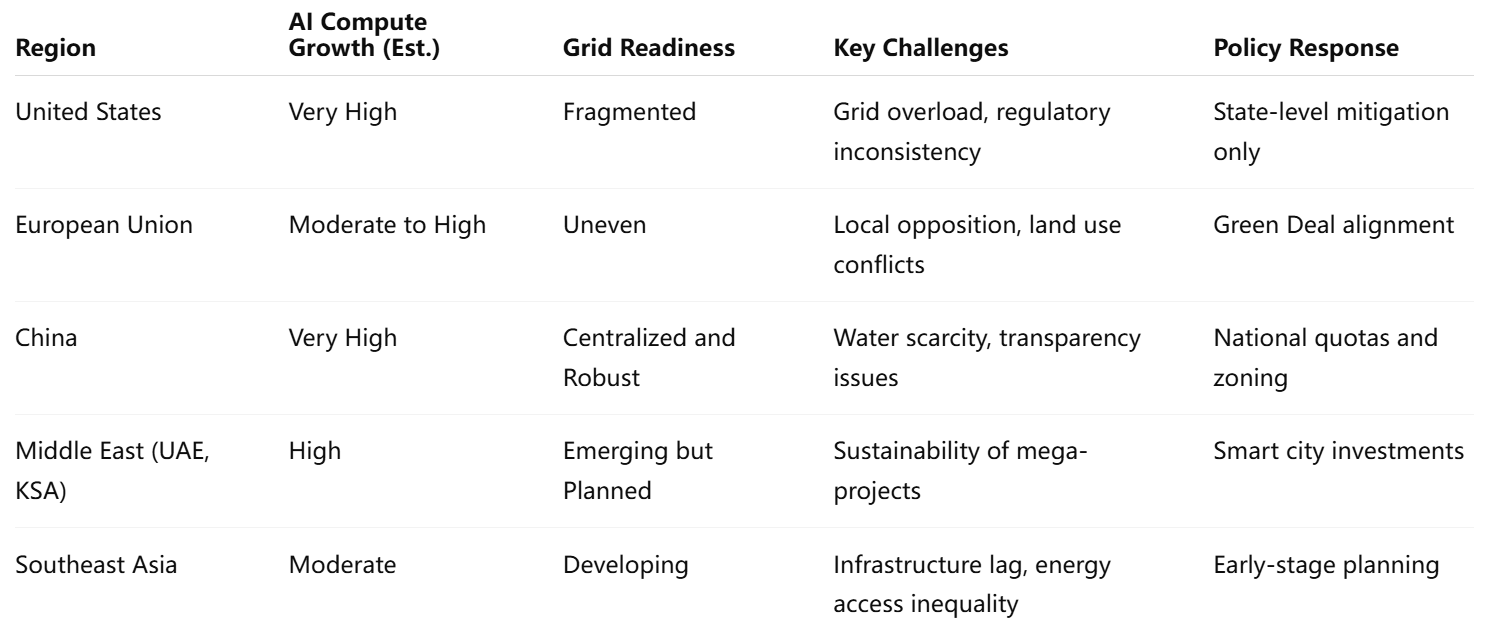
This table underscores a key theme: while AI has a universal trajectory, its infrastructural feasibility remains deeply region-specific. The divergence in readiness and regulatory response is likely to define competitive advantages and limitations in the next wave of AI-driven innovation.
Can Renewables and Efficiency Catch Up?
As the energy footprint of artificial intelligence (AI) continues to expand, the question of whether renewable energy sources and computational efficiency improvements can offset its growing demand has moved to the forefront of technological and policy discussions. While the deployment of AI systems offers immense potential for productivity and innovation, their rising power requirements risk undermining global decarbonization goals unless effectively addressed through sustainable energy strategies and model optimization.
This section critically examines the capacity of renewable energy sources to meet AI’s power needs, the challenges in scaling sustainable infrastructure, and the progress being made in AI model efficiency and hardware innovations. Together, these factors will determine whether the industry can maintain its current trajectory without triggering unsustainable energy consumption.
Renewable Energy Integration in AI Infrastructure
Several major AI stakeholders, including Microsoft, Google, Amazon, and Meta, have publicly committed to powering their operations with 100% renewable energy. These commitments are often cited as a key counterbalance to growing energy use, with companies pledging to source energy from solar farms, wind parks, hydroelectric stations, and even emerging technologies like geothermal and green hydrogen.
However, a closer inspection reveals that renewable adoption, while commendable, is not without significant constraints. One major challenge is intermittency. Solar and wind energy are weather-dependent and time-bound, making them less reliable for continuous 24/7 data center operations, which require consistent and predictable power loads. To bridge this gap, operators must either invest in large-scale battery storage—an expensive and technically complex undertaking—or rely on backup fossil-fuel generators, which undermine carbon neutrality goals.
Moreover, the physical geographical disconnect between renewable generation sites and data centers poses logistical barriers. Renewable energy is often most abundant in rural or remote areas, whereas data centers are typically located near urban centers or network interconnection points. This mismatch requires substantial investment in long-distance high-voltage transmission lines, which face regulatory, land-use, and political hurdles.
Despite these barriers, some companies are experimenting with colocation strategies, building data centers adjacent to renewable energy sources. For example, Google’s data center in Hamina, Finland, is powered by a nearby wind farm and cooled using seawater. Similarly, Amazon Web Services (AWS) has invested in multiple solar-powered facilities in Virginia and Texas. Yet, these remain exceptions rather than the rule, and replicating such models globally involves overcoming significant scalability challenges.
Power Usage Effectiveness and Sustainable Design
In addition to sourcing cleaner energy, AI infrastructure developers have made progress in reducing energy waste through innovations in data center design and cooling systems. The industry-standard metric, Power Usage Effectiveness (PUE)—which measures the ratio of total facility energy to energy used by computing equipment—has improved substantially in recent years.
Legacy data centers often had PUE ratings above 2.0, indicating that for every watt of computing power used, another watt was consumed by cooling and overhead systems. Modern hyperscale facilities now target PUE values as low as 1.1 or 1.2, approaching theoretical efficiency limits. Innovations contributing to this improvement include:
- Liquid cooling systems that use chilled water or dielectric fluids to dissipate heat more effectively than air-based systems.
- Free cooling, which utilizes ambient outdoor air in cold climates to cool equipment passively.
- AI-driven energy management, which optimizes server loads, cooling cycles, and power routing in real-time to reduce wastage.
These efficiency gains, while impressive, are being outpaced by the rapid increase in AI compute demand. As model size and usage scale, even highly efficient data centers are consuming more total energy year-over-year.
AI Model Efficiency: Architectural Advances and Trade-offs
Beyond infrastructure, another key avenue for reducing AI’s energy consumption lies in optimizing the models themselves. Traditionally, larger models have been equated with better performance, but this has come at the cost of exponential increases in training time, hardware utilization, and ultimately, energy consumption.
To counteract this trend, researchers and developers are focusing on several promising strategies:
Model Compression and Quantization
These techniques reduce the size and complexity of models without significantly compromising performance. Quantization lowers the precision of model parameters (e.g., from 32-bit to 8-bit), enabling faster inference and reduced memory usage. Compression methods like pruning eliminate redundant neurons or layers, slimming down the model.
Distillation
Knowledge distillation involves training a smaller “student” model to replicate the behavior of a larger “teacher” model. This results in more efficient deployments, especially for edge devices and mobile applications, without retraining from scratch.
Sparse Architectures
Rather than activating all nodes in a neural network simultaneously, sparse models compute only the necessary paths based on the input context. Google’s Mixture of Experts (MoE) model, for instance, activates only a subset of its massive parameter space for each task, significantly improving energy efficiency per query.
Low-Rank Adaptation (LoRA) and Fine-Tuning
Instead of retraining full models, techniques like LoRA introduce minimal trainable parameters that adapt pre-trained models to new tasks, reducing both time and energy consumption during customization.
While these methods are highly effective, they often involve trade-offs in performance fidelity or adaptability. Moreover, deploying them at scale across a fragmented landscape of AI applications and platforms requires significant coordination and tooling support.
Hardware Innovations and Specialized Accelerators
Complementing software-level efficiency improvements are advancements in AI hardware accelerators. Companies are developing chips designed specifically for AI workloads, with an emphasis on energy-efficient matrix computations, memory bandwidth optimization, and intelligent power scaling.
- Google TPUs (Tensor Processing Units) have evolved to deliver higher performance per watt compared to general-purpose GPUs, with custom silicon tuned for inference and training.
- NVIDIA’s Grace Hopper Superchip and H100 architecture feature advanced power management, high-throughput interconnects, and software-defined control planes that optimize resource allocation.
- Graphcore, Cerebras, and Tenstorrent are pushing the boundaries of low-power AI compute, focusing on edge deployment and minimal latency with reduced energy overhead.
Moreover, emerging paradigms such as neuromorphic computing—which mimics brain-like architectures—and optical computing promise quantum leaps in energy efficiency, although these remain largely experimental.
Market Incentives and Sustainability Pressure
Corporate and regulatory pressure is further accelerating the move toward greener AI. Investors are increasingly prioritizing Environmental, Social, and Governance (ESG) metrics, compelling public companies to disclose energy usage, emissions, and sustainability plans.
Cloud providers are being scrutinized for “greenwashing”—the practice of overstating the environmental friendliness of their operations. Independent watchdogs and NGOs are calling for standardized energy reporting, lifecycle emissions accounting, and carbon-offset verification. As scrutiny intensifies, many enterprises are integrating carbon-aware scheduling, which delays non-urgent tasks until renewable energy is most available, as part of their AI workload strategies.
The Efficiency vs. Scale Paradox
Despite the encouraging progress in renewables and efficiency, a fundamental tension remains: efficiency gains are being outpaced by the scale of AI adoption. While each model or data center may become more efficient, the total volume of compute is expanding faster than energy savings can compensate.
This phenomenon—known as the Jevons Paradox—suggests that increased efficiency may lead to higher overall consumption due to lower operational costs and greater accessibility. As AI tools become more embedded in daily operations across industries, the baseline demand for compute will likely rise, even if per-operation energy use declines.
Looking Ahead
To truly align AI with climate resilience, a multi-pronged strategy is required:
- Policy alignment to ensure that AI infrastructure is colocated with clean energy sources.
- Standards for energy reporting and emissions transparency from all major AI developers.
- Investment in frontier technologies like carbon-negative data centers and grid-integrated AI platforms.
- Public-private partnerships that scale infrastructure intelligently while preserving ecological integrity.
The AI revolution need not be at odds with environmental sustainability, but achieving this balance demands intentional design, regulatory foresight, and technological discipline. The next section explores whether these efforts will be enough to avert an energy crisis—or if a deeper systemic recalibration is required.
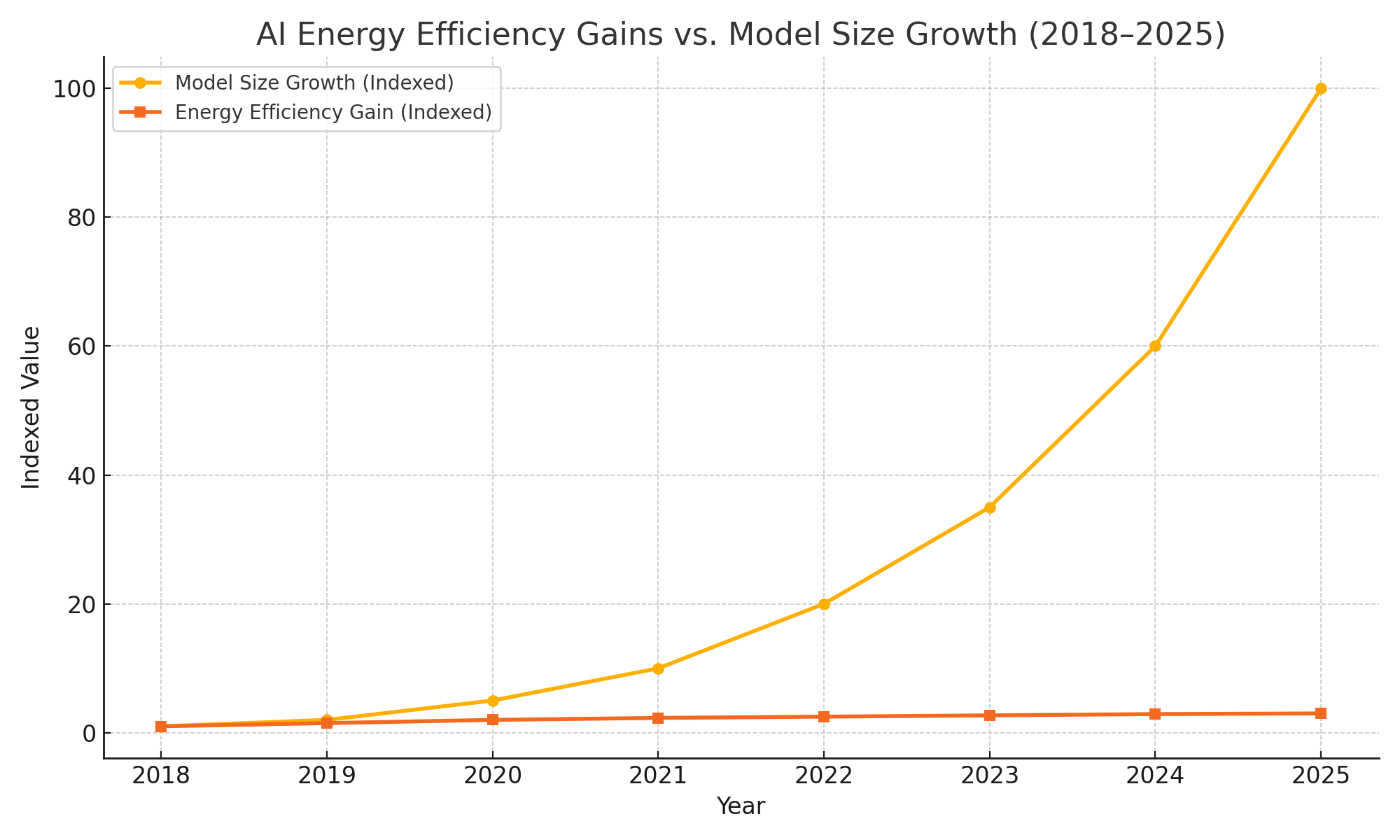
Long-Term Outlook – Energy Crisis or Transformation?
As artificial intelligence (AI) continues its rapid integration into virtually every domain—from finance and manufacturing to education and healthcare—the long-term implications of its energy consumption have become a focal point of global discourse. The dual trajectory of AI advancement and intensifying climate challenges raises a pivotal question: will the AI revolution culminate in a deepening global energy crisis, or could it catalyze a transformation in energy innovation and sustainable development?
This section explores potential future scenarios based on current trends, policy initiatives, and technological developments. It considers the role of regulatory frameworks, ethical imperatives, market dynamics, and scientific innovation in shaping whether AI becomes a liability to the planet—or a lever for its preservation.
Diverging Scenarios: Crisis vs. Transformation
The future of AI’s energy impact can be broadly categorized into two divergent scenarios: one where growth is unchecked and outpaces sustainable controls, and another where innovation and policy converge to enable a greener, more resilient digital economy.
Scenario A: The Crisis Pathway
In this trajectory, AI expansion proceeds largely unregulated, driven by commercial competition and national security imperatives. Large language models, multimodal architectures, and autonomous systems scale at exponential rates, fueled by global demand for intelligent automation. However, the infrastructure required to support this boom—compute clusters, cloud platforms, and edge devices—grows faster than the energy systems that underpin them.
As data centers proliferate, they exacerbate strain on aging electrical grids, increase peak-load vulnerabilities, and accelerate the consumption of fossil fuels, especially in regions lacking robust renewable infrastructure. Countries with limited energy resilience may face rolling blackouts, inflationary energy prices, and resource conflicts as digital systems outcompete traditional sectors for electricity. In this scenario, AI becomes a driver of environmental degradation, potentially undermining progress on emissions targets and deepening global inequities.
The public response could lead to regulatory backlash, moratoriums on new data center construction, and social resistance to further AI deployment. These reactive policies, while well-intentioned, may hamper innovation without addressing the structural inefficiencies that underlie the crisis. In short, unchecked AI growth without parallel investment in sustainable energy risks triggering a systemic crisis that reverberates far beyond the tech sector.
Scenario B: The Transformation Pathway
In the alternative scenario, AI acts as a catalyst for sustainability. Here, nations and corporations recognize early the risk-reward calculus of AI’s energy appetite and implement strategic interventions to harmonize growth with climate goals. This transformation is driven by four primary pillars:
- Energy-Aware AI Development
Developers begin to treat energy efficiency not as an afterthought but as a core design principle. Model architecture is optimized not only for performance but also for carbon impact, with incentives tied to energy-efficient innovations. Organizations adopt standards that mandate the publication of training emissions and power usage per inference, fostering transparency and competition around sustainability. - Carbon-Conscious Regulation
Governments introduce compute-based carbon taxes, green AI certifications, and energy allocation frameworks for large-scale model deployments. These policies reward sustainability leadership and penalize excessive or wasteful compute usage. A global alliance on sustainable AI emerges, promoting cross-border best practices and emissions accounting mechanisms. - Grid-Smart AI Infrastructure
Data centers evolve into grid-responsive entities, equipped with AI systems that manage their own power consumption in alignment with supply availability. Carbon-aware scheduling becomes a standard practice, dynamically shifting workloads to regions and time periods with surplus renewable energy. Modular microgrids and on-site generation become common, decoupling AI infrastructure from centralized grid vulnerabilities. - Positive Feedback Loop for Clean Energy
AI is increasingly deployed to optimize renewable generation, battery storage, and demand-side management. Predictive algorithms stabilize power flows, reduce waste, and accelerate the decarbonization of broader energy systems. In this vision, the very tools that once threatened sustainability become instrumental in securing it.
This transformative scenario requires visionary leadership, robust public-private partnerships, and sustained investment in frontier technologies. However, it offers the most promising path toward a symbiotic relationship between AI and energy.
The Role of Global Policy and Coordination
Whether the future unfolds as crisis or transformation will largely depend on global policy harmonization and the willingness of key actors to embed sustainability into AI governance. To date, energy policy has rarely intersected meaningfully with digital regulation. AI is often viewed through the lens of ethics, fairness, and safety—but its material footprint has been underestimated in regulatory frameworks.
This must change. International organizations such as the United Nations, International Energy Agency (IEA), and the World Economic Forum have begun issuing warnings about the unsustainable trajectory of digital infrastructure. Still, binding mechanisms remain elusive.
A global protocol on compute and emissions governance, similar in ambition to the Paris Agreement, could establish guardrails for responsible AI growth. Such a framework would include:
- Mandatory emissions disclosure for training and deployment phases
- Caps or quotas on high-energy model development
- International benchmarking for AI energy efficiency
- Support mechanisms for low-income nations to access sustainable AI infrastructure
Without such coordination, the geopolitical fragmentation of AI development may foster resource hoarding, cross-border energy arbitrage, and technological monopolies that undermine equitable progress.
Ethical Considerations and Societal Prioritization
The long-term energy impact of AI also raises profound ethical questions. Should society permit the unrestricted use of computationally expensive models when they offer marginal utility? Is it acceptable for entertainment applications—such as AI-generated art or synthetic video—to consume the same energy as vital medical simulations or climate modeling?
A broader societal discourse on computational prioritization is needed. This includes reevaluating the value of digital abundance versus sustainability, and exploring whether certain applications should face usage restrictions based on their carbon cost per benefit ratio. Much like industrial zoning or emission permits, AI deployment could be triaged according to societal impact and resource availability.
Moreover, the ethics of digital consumption must be democratized. End-users rarely see the energy implications of their queries, image generations, or chatbot conversations. Including carbon cost indicators in user interfaces—similar to calorie counts on food packaging—could raise awareness and foster more responsible consumption.
Reframing the Narrative: From Risk to Opportunity
While the risks of an AI-driven energy crisis are real and pressing, they must be weighed against the opportunity for proactive transformation. AI, if governed wisely, has the potential to revolutionize not only information processing but also how humanity generates, distributes, and consumes energy.
By reframing the challenge as an opportunity—AI as a force multiplier for sustainability—governments and corporations can galvanize support for climate-aligned innovation. Rather than resisting AI growth, the goal should be to embed ecological intelligence into its very DNA.
The stakes are high. AI may be the defining technology of the 21st century, but its legacy will not be measured solely in capability—it will also be judged by its cost to the planet and its contribution to humanity’s resilience.
Conclusion
The intersection of artificial intelligence and energy infrastructure presents one of the most consequential challenges of our era. As AI systems scale in scope, sophistication, and societal impact, their energy requirements are becoming increasingly difficult to ignore. What was once a niche computational task performed in academic settings has evolved into a global industrial-scale operation, underpinned by high-density data centers, energy-intensive hardware, and always-on service models. The implications of this transformation extend far beyond the technology sector, touching upon climate policy, infrastructure planning, economic development, and ethical responsibility.
This blog has explored the multifaceted nature of AI’s energy footprint—from the rising demands of model training and inference to the physical strain placed on regional power grids. It has examined the disparity in regional infrastructure readiness, highlighting how some countries are moving decisively to manage AI-related energy consumption while others are struggling to cope with the pace of digital expansion. Furthermore, it has considered whether renewable energy adoption and advances in model and hardware efficiency can realistically mitigate the projected demand curve. While signs of progress are evident, they are often outpaced by the scale of AI adoption and the competitive pressures driving ever-larger models.
Looking to the future, two divergent paths emerge. One leads toward escalating energy crises, grid instability, and growing resistance to AI deployment. The other charts a course toward transformation, wherein AI is leveraged not only to optimize its own efficiency but also to act as a key enabler of global energy transition strategies. The determining factors will be proactive policy-making, corporate accountability, transparent benchmarking, and a global consensus on responsible innovation.
Crucially, the energy cost of AI must no longer be viewed as a secondary concern. It is an integral part of the technology's lifecycle and societal impact. As stakeholders—from developers and investors to regulators and end-users—contemplate the future of AI, sustainability must be embedded into the core design and deployment philosophy. This requires a shift from short-term performance metrics to long-term ecological stewardship.
AI holds the potential to accelerate solutions for climate change, healthcare, logistics, education, and beyond. Yet, if left unchecked, its development could simultaneously hinder progress in these very domains by exacerbating energy imbalances and environmental degradation. The path we choose now will shape not only the trajectory of artificial intelligence but also the resilience of the world it seeks to improve.
In the final analysis, the AI boom need not fuel a global energy crisis. It can instead be the catalyst that compels society to reimagine the relationship between intelligence, innovation, and sustainability. The imperative is clear: build smarter—and greener.
References
- OpenAI – Energy and compute considerations for AI:
https://openai.com/blog/ai-and-compute - International Energy Agency – Data centers and energy demand:
https://www.iea.org/reports/data-centres-and-data-transmission-networks - Microsoft Sustainability – AI and carbon footprint strategy:
https://www.microsoft.com/sustainability - Google – Climate commitments and data center energy practices:
https://sustainability.google/projects/clean-energy - MIT Technology Review – The hidden cost of AI:
https://www.technologyreview.com/2022/04/05/1048742/ai-climate-change-carbon-footprint - Hugging Face – Carbon emissions tracking for ML models:
https://huggingface.co/blog/emissions - Nature – The environmental costs of artificial intelligence:
https://www.nature.com/articles/d41586-019-03875-5 - The Verge – Data centers, AI, and grid strain:
https://www.theverge.com/2023/03/06/data-centers-ai-energy-consumption - World Economic Forum – Making AI more sustainable:
https://www.weforum.org/agenda/2023/01/how-to-make-ai-sustainable - MLCommons – Benchmarking efficiency in machine learning:
https://mlcommons.org/en