Digital Twins in Action: How AI Is Revolutionizing Real-Time Monitoring Across Industries
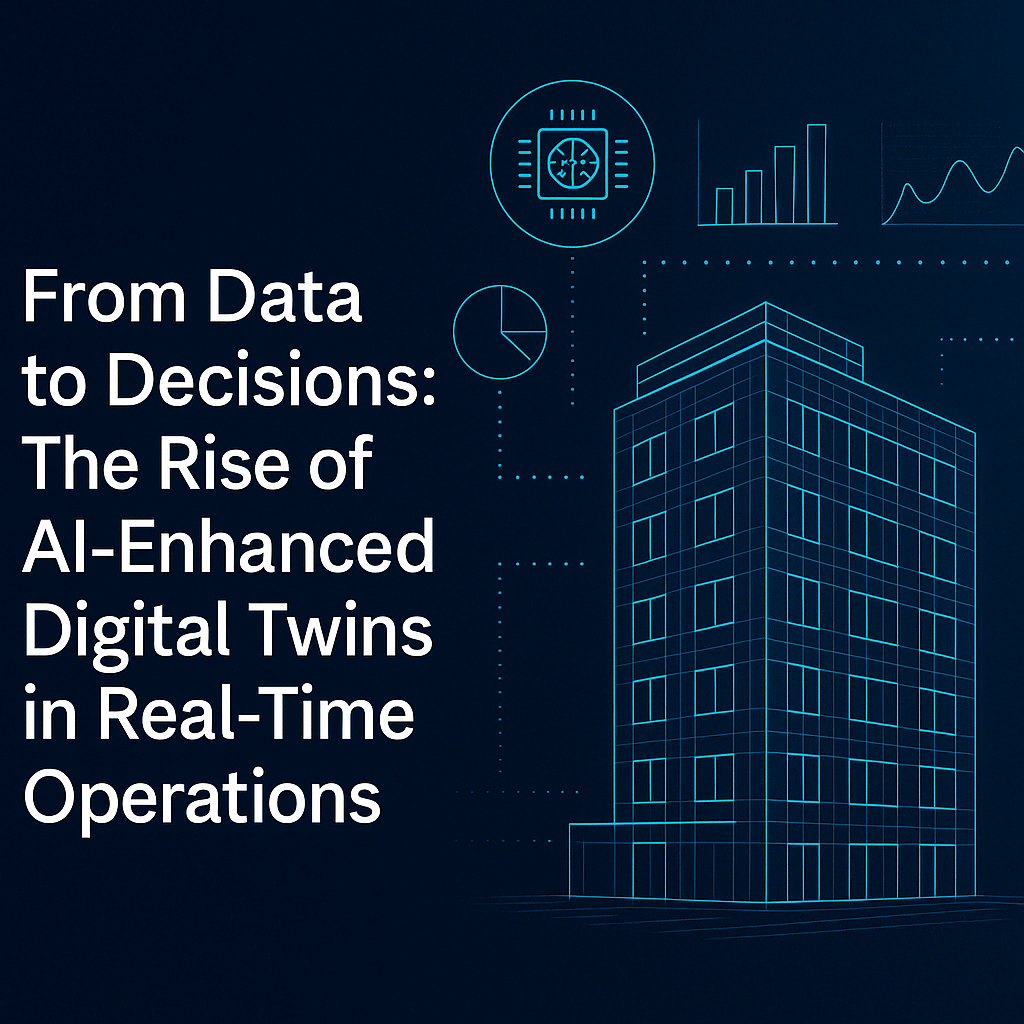
The increasing complexity and scale of modern systems—from manufacturing plants to smart cities—has placed an unprecedented demand on real-time monitoring capabilities. In today’s dynamic environments, traditional monitoring solutions often fall short, constrained by static models, delayed responses, and an inability to adapt to rapidly changing conditions. In this context, the concept of digital twins has emerged as a transformative technology, offering dynamic virtual representations of physical systems that can be used to observe, simulate, and optimize real-world processes. However, even digital twins in their original form lacked the real-time intelligence and adaptability required by next-generation applications.
Enter AI-enhanced digital twins, the next evolutionary step in the convergence of operational technology (OT) and artificial intelligence (AI). These advanced digital constructs go beyond static simulation, embedding real-time data ingestion, machine learning capabilities, and predictive analytics to create a living, learning replica of a system or asset. By integrating AI, digital twins become proactive agents capable of identifying anomalies, optimizing performance, forecasting outcomes, and even autonomously adjusting system parameters. This progression is not merely incremental—it redefines the entire landscape of real-time monitoring.
Organizations across sectors are recognizing the immense potential of AI-enhanced digital twins to drive efficiency, reduce risk, and unlock new forms of value. In manufacturing, these systems can monitor equipment health in real time, predict failures, and schedule preventive maintenance. In energy, they help operators balance grid loads, forecast demand, and optimize renewable asset performance. In healthcare, digital twins modeled on individual patients can support diagnostics, treatment planning, and post-operative monitoring. Across every use case, the combination of real-time monitoring and intelligent digital modeling holds the promise of reduced downtime, increased safety, and operational excellence.
Yet, the deployment of AI-enhanced digital twins is not without challenges. Organizations must navigate data integration complexities, infrastructure requirements, ethical implications, and evolving regulatory landscapes. As with any powerful technology, thoughtful implementation is essential to ensure reliability, trust, and long-term sustainability.
This blog post explores the transformative impact of AI-enhanced digital twins on real-time monitoring. We will begin by tracing the evolution of digital twin technologies and their convergence with AI. We then delve into the technical underpinnings of AI integration, followed by an examination of industry-specific applications. The post also addresses challenges and ethical considerations, and concludes with a forward-looking perspective on the strategic role of intelligent digital twins in shaping the future of enterprise operations.
Understanding Digital Twins and Their Evolution
Digital twins have steadily emerged as a cornerstone technology in the age of cyber-physical systems, serving as a bridge between the physical and digital realms. At their core, digital twins are virtual replicas of physical entities—be they products, processes, or entire systems—that are continuously updated with real-time data. Initially conceived as static models used for design validation, digital twins have evolved significantly over the past two decades, culminating in intelligent, autonomous systems that leverage artificial intelligence (AI) for decision-making and performance optimization.
Origins and Conceptual Foundation
The conceptual roots of digital twins can be traced back to aerospace engineering, where NASA pioneered the use of simulation models to support mission-critical operations. In the early 2000s, engineers began to formalize the digital twin concept as a framework for mirroring physical assets in a virtual space. The goal was to enable a continuous flow of information between the physical and digital domains, allowing for predictive modeling, diagnostics, and operational insight.
At this initial stage, digital twins were largely confined to basic modeling and simulation. These systems relied heavily on physics-based principles and were primarily used in design and engineering environments. Although they provided valuable insights during product development, their utility diminished once the product entered into operation, as these early models lacked real-time feedback loops.
The Shift Toward Sensor Integration
A significant inflection point occurred with the widespread adoption of sensor technology and embedded systems. This ushered in the second phase of digital twin evolution—sensor-integrated twins. Engineers began embedding sensors within machines, vehicles, and infrastructure to continuously capture operational data such as temperature, vibration, pressure, and motion.
This real-time data could then be fed back into the digital model, making it possible to monitor asset performance with a level of granularity and immediacy that was previously unattainable. These systems also supported condition-based maintenance strategies, allowing operators to move beyond calendar-based inspections to predictive interventions based on actual wear and usage patterns.
The addition of sensors enabled a dynamic feedback loop but still required human oversight to interpret the data and draw conclusions. The systems, though improved, remained essentially passive.
IoT and the Emergence of Real-Time Digital Twins
With the advent of the Internet of Things (IoT), the digital twin concept expanded significantly in scale and capability. IoT platforms enabled the seamless interconnection of thousands—sometimes millions—of sensors and devices. This advancement brought about the IoT-connected real-time twin, capable of ingesting vast amounts of data from distributed physical assets.
IoT-enhanced digital twins became central to operational monitoring in sectors such as manufacturing, energy, and transportation. Real-time visibility into system performance allowed for quicker incident response, improved quality control, and more effective resource utilization. Additionally, cloud computing platforms provided the necessary scalability to manage and process the large volumes of streaming data generated by IoT devices.
At this stage, digital twins began to move from being asset-centric to system-centric, modeling not just individual components but the interactions and dependencies among them. However, the ability to interpret complex patterns or forecast future states remained limited by the analytical capabilities of the underlying software.
The Infusion of Artificial Intelligence
The integration of AI represents the most transformative phase in the evolution of digital twins, giving rise to AI-enhanced autonomous twins. These systems are distinguished not merely by their capacity to monitor and simulate, but by their ability to learn, predict, and act autonomously.
AI models—particularly those leveraging machine learning, deep learning, and neural networks—enable digital twins to:
- Detect subtle anomalies in operational behavior that human analysts might overlook,
- Predict equipment failure before it occurs, based on historical and real-time data,
- Recommend and even initiate corrective actions without human intervention.
These capabilities shift digital twins from reactive tools to proactive agents of operational intelligence. For instance, a digital twin of a jet engine can predict when a turbine blade is likely to fail, initiate a maintenance ticket, and adjust flight schedules to minimize disruption—all before a human operator is even aware of the issue.
AI-enhanced twins also support the generation of synthetic data, which can be used to train more robust models in environments where real-world data is scarce, sensitive, or costly to obtain. This ability to simulate future scenarios based on learned behavior dramatically expands the strategic value of digital twins.
The Role of Edge and Cloud Architectures
The technical architecture supporting AI-enhanced digital twins has also evolved. Edge computing enables local data processing, reducing latency and ensuring real-time responsiveness in critical applications such as autonomous vehicles or industrial control systems. Simultaneously, cloud platforms offer the computational scale required for model training, data storage, and global coordination.
By deploying AI models across both edge and cloud environments, organizations achieve a hybrid architecture that maximizes efficiency, scalability, and resilience. This layered approach is particularly important in sectors with stringent uptime requirements and data governance constraints.
The Increasing Importance of Semantic Modeling
Another noteworthy advancement in the evolution of digital twins is the incorporation of semantic modeling. Traditional twins often represent physical states in numerical form, but semantic layers provide context to those numbers, enabling deeper machine understanding. For example, it is one thing for a twin to report that a pipeline’s temperature has exceeded 100°C; it is another for it to recognize that this threshold implies a risk of thermal expansion and notify operators of a potential structural hazard.
Semantic models, when combined with AI reasoning engines, can infer relationships, prioritize alerts, and support human decision-making with greater nuance and precision.
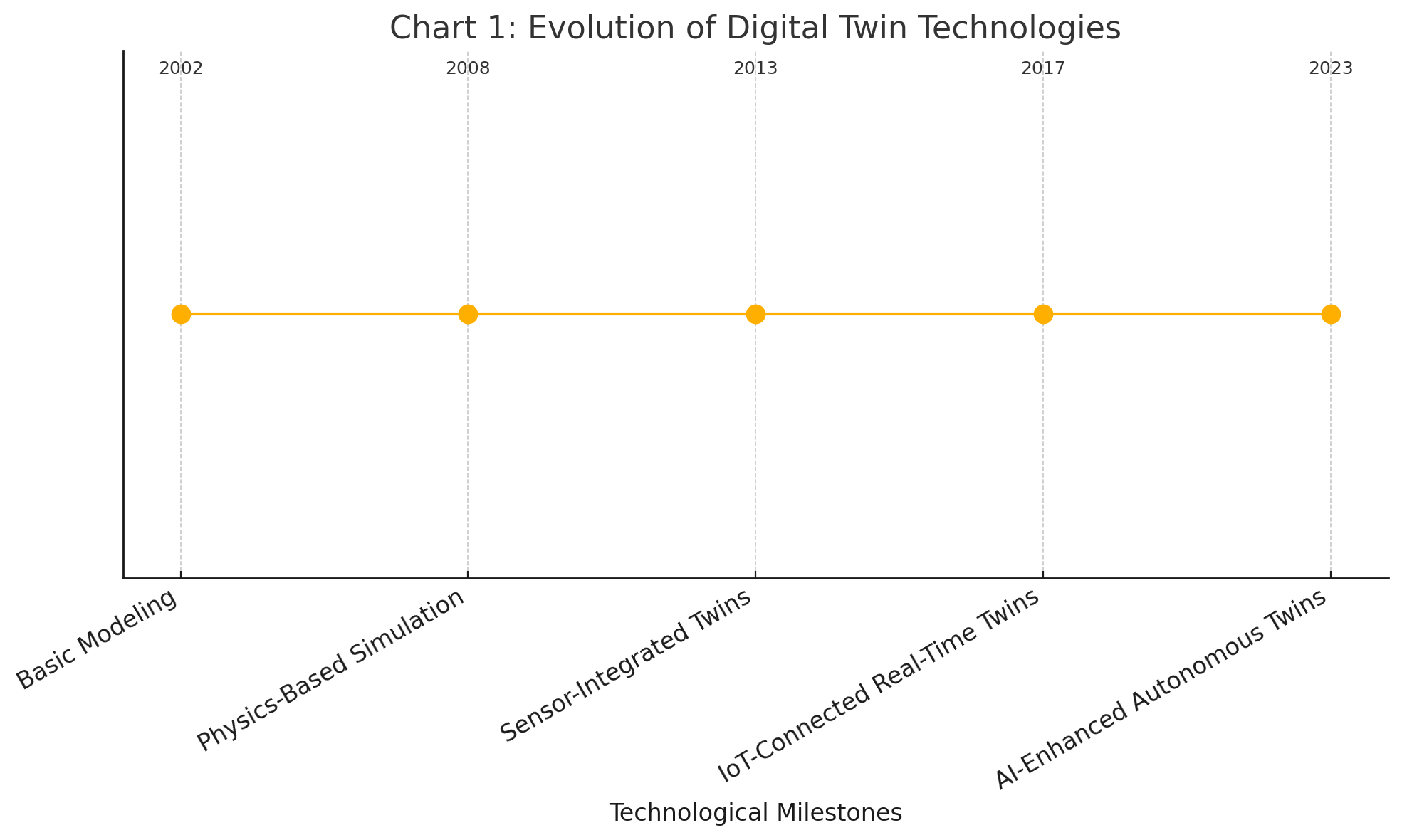
As illustrated in Chart 1, the evolution of digital twin technologies can be segmented into five distinct milestones: from basic modeling and simulation in the early 2000s, to physics-based simulation, sensor integration, IoT-driven real-time updates, and finally the current generation of AI-enhanced autonomous systems. Each phase represents not only a technical leap but also a shift in the conceptual role that digital twins play within enterprise ecosystems—from passive replicas to intelligent agents.
This progression highlights the increasing integration of disciplines, including systems engineering, data science, computer vision, and robotics. It underscores how digital twins have evolved from a product of engineering necessity to a foundational enabler of real-time operational intelligence.
AI Integration: Unlocking Intelligence in Real-Time Monitoring
The convergence of artificial intelligence (AI) and digital twin technology represents a watershed moment for real-time monitoring systems. While digital twins provide a structural framework for simulating and visualizing physical assets, AI imbues these systems with the capacity to understand, learn from, and autonomously respond to changing operational conditions. The result is an intelligent, data-driven architecture capable of elevating real-time monitoring from a reactive process to a predictive and adaptive one.
This section explores the transformative role of AI in digital twin environments. We will examine key AI techniques used in real-time monitoring, architectural considerations, data handling strategies, and the tangible benefits achieved through this integration.
AI-Enhanced Digital Twins: Defining Characteristics
To understand the integration of AI within digital twins, it is important to first clarify what distinguishes an AI-enhanced digital twin from its traditional counterpart. Whereas conventional digital twins are capable of mirroring a system’s current state and simulating future scenarios based on pre-configured rules or physics-based models, AI-enhanced twins go further by:
- Learning from historical and real-time data to improve model accuracy.
- Identifying patterns and anomalies without explicit programming.
- Predicting failures and performance bottlenecks based on probabilistic models.
- Recommending or initiating actions based on contextual understanding.
These capabilities are enabled by a broad range of AI techniques, including supervised and unsupervised machine learning, deep learning, computer vision, and natural language processing (NLP), all of which contribute to a more holistic, dynamic view of system behavior.
Key AI Techniques and Their Roles
- Machine Learning (ML):
ML algorithms allow digital twins to identify correlations within large volumes of structured and unstructured data. These models are particularly effective in predictive maintenance applications, where they analyze sensor data to forecast component failures with high precision. - Deep Learning (DL):
DL models, especially convolutional neural networks (CNNs), are used in scenarios requiring image or video interpretation. In industrial settings, digital twins augmented with DL can detect defects in real-time using visual data from cameras and infrared sensors. - Reinforcement Learning:
In autonomous operations, reinforcement learning enables the digital twin to improve decision-making by receiving feedback from its environment. For example, a twin simulating an HVAC system can optimize energy consumption by adjusting airflow patterns and learning from outcomes over time. - Natural Language Processing (NLP):
NLP is particularly useful in customer service and documentation-heavy environments, allowing digital twins to process maintenance logs, incident reports, or operator comments to enrich their contextual understanding.
Real-Time Data Ingestion and Sensor Fusion
Real-time monitoring demands not only speed but also accuracy in interpreting a complex array of data sources. AI-enhanced digital twins employ sensor fusion—the process of integrating data from multiple heterogeneous sensors to produce more accurate and comprehensive insights than any single source alone.
For instance, a digital twin of a smart building might combine data from:
- Environmental sensors (temperature, humidity, CO2)
- Structural health monitors (vibration, load)
- Behavioral tracking (occupancy, movement)
- Visual inputs (CCTV and thermal imaging)
By fusing these inputs, the AI model can detect inconsistencies, resolve conflicting signals, and generate a coherent view of building performance. More importantly, it can continuously refine its accuracy through retraining on fresh data, thereby minimizing drift and improving operational confidence.
Predictive Analytics and Anomaly Detection
A cornerstone of AI-enhanced digital twins is their predictive capability. Machine learning models are trained to recognize operational baselines and forecast future states based on ongoing trends. These capabilities support a range of monitoring tasks, including:
- Predictive Maintenance:
Detecting early indicators of equipment wear or failure allows organizations to intervene before costly breakdowns occur, significantly reducing unplanned downtime. - Process Optimization:
Digital twins can simulate various control strategies and identify optimal configurations, which are then tested and deployed in real time to enhance productivity or efficiency. - Anomaly Detection:
AI models excel at recognizing patterns that deviate from the norm. This is particularly valuable in industries like finance, manufacturing, and healthcare, where early detection of anomalies can prevent cascading failures or safety incidents.
The sophistication of these predictions is continually improving through techniques such as transfer learning, ensemble modeling, and federated learning, which allow models to be trained across multiple sites without compromising data privacy.
Edge vs. Cloud Architectures
Deploying AI-enhanced digital twins requires an infrastructure capable of processing large volumes of data with minimal latency. Two complementary approaches are typically used:
- Edge Computing:
Edge nodes handle localized processing tasks, minimizing latency and bandwidth requirements. In time-sensitive applications—such as autonomous vehicles or industrial automation—AI models are deployed directly on edge devices to enable real-time decision-making. - Cloud Computing:
The cloud provides elastic computational resources, ideal for training complex models, running long-term analytics, and storing historical data. AI models trained in the cloud can then be pushed to the edge for execution.
This hybrid architecture ensures that organizations benefit from both scalability and responsiveness. Furthermore, containerization and orchestration tools such as Kubernetes enable seamless deployment of AI workloads across distributed environments.
System Feedback and Autonomous Action
Perhaps the most transformative capability of AI-enhanced digital twins is their potential to autonomously close the loop between monitoring and control. By embedding decision-making logic into the twin, systems can respond to events without human intervention.
Examples include:
- A smart grid digital twin adjusting transformer loads based on energy demand forecasts.
- A twin of an autonomous drone recalibrating flight paths in response to changing weather conditions.
- A manufacturing system reassigning robotic arms based on real-time production throughput.
This capacity for autonomous adaptation significantly reduces human workload while improving response times and system resilience.
As shown in Chart 2, AI-enhanced digital twins outperform traditional monitoring systems across several key performance indicators. These improvements result in increased system reliability, reduced operational costs, and enhanced strategic decision-making.
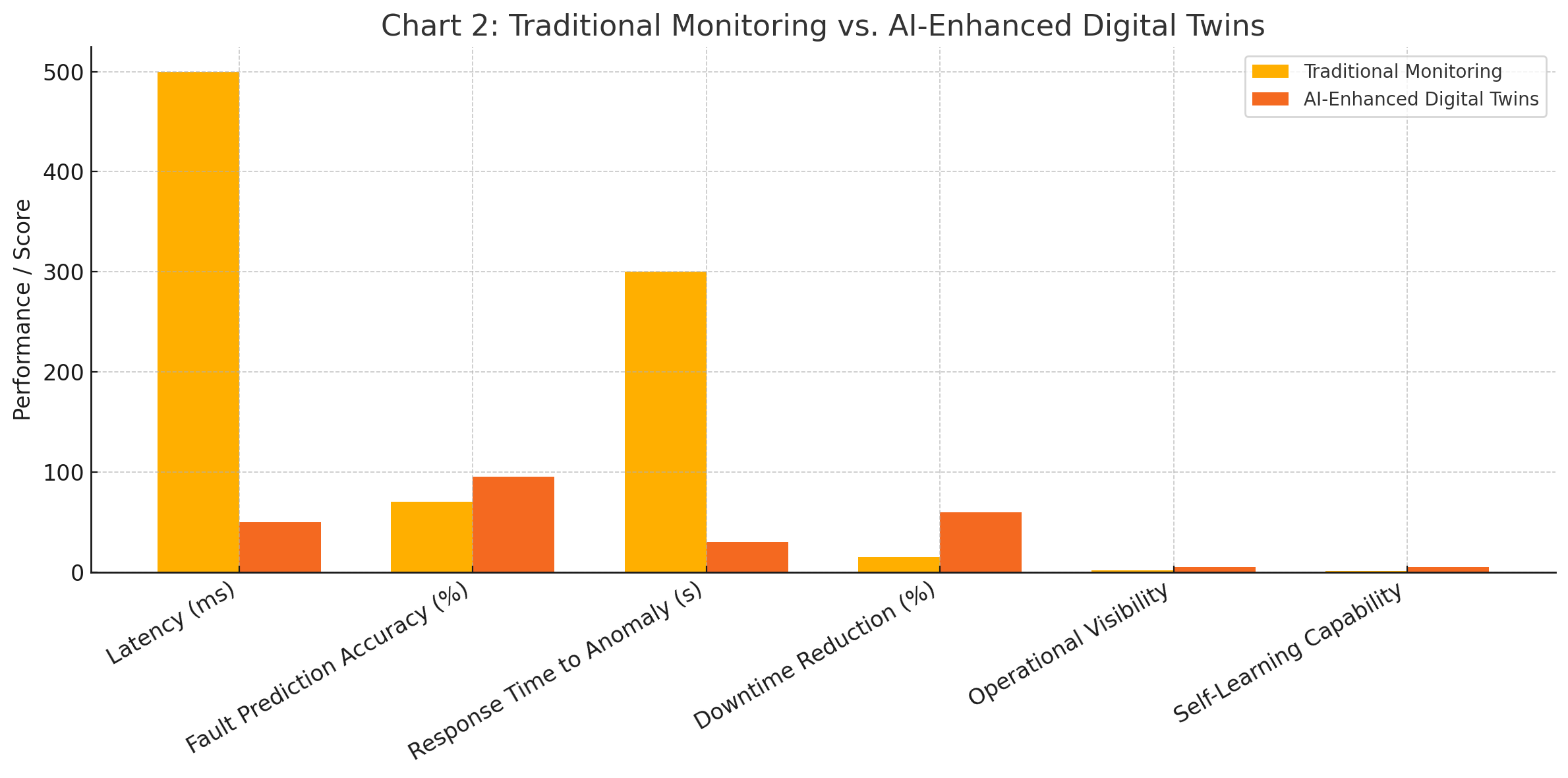
Security and Model Governance
While AI integration offers many benefits, it also introduces new challenges. Models must be continuously validated to avoid drift and ensure trustworthiness. Moreover, securing AI pipelines—particularly those running at the edge—is critical. Organizations must adopt secure model update mechanisms, encrypted data streams, and access control protocols to maintain system integrity.
In parallel, model explainability tools are becoming increasingly important, particularly in regulated industries where operators must understand and justify automated decisions.
AI-enhanced digital twins offer a powerful synthesis of real-time monitoring, simulation, and autonomous decision-making. By incorporating advanced AI techniques, organizations are not only observing the present but actively shaping the future of their operations.
Cross-Industry Applications and Outcomes
The transformative potential of AI-enhanced digital twins lies not merely in their technical sophistication, but in their remarkable adaptability across diverse industries. These intelligent replicas of physical assets, processes, and systems are being deployed to address real-time operational challenges, reduce inefficiencies, enhance safety, and optimize performance across sectors ranging from manufacturing and energy to healthcare, logistics, and urban infrastructure.
This section explores how AI-augmented digital twins are delivering tangible outcomes in varied operational contexts. The applications detailed below demonstrate that digital twins are no longer experimental; they are integral to the next generation of intelligent enterprise solutions.
Manufacturing: Real-Time Optimization and Predictive Quality Control
Manufacturing has been at the forefront of digital twin adoption due to the complexity and interconnectivity of shop floor processes. In this domain, AI-enhanced digital twins are revolutionizing how factories operate by enabling real-time equipment monitoring, predictive maintenance, and autonomous process control.
For example, a digital twin of a CNC (Computer Numerical Control) milling machine can continuously monitor vibration, torque, and temperature sensors to detect tool wear before failure. Using AI models trained on historical performance data, the system predicts when recalibration or part replacement is needed, minimizing unplanned downtime. Furthermore, the twin can simulate production scenarios to identify bottlenecks and test corrective actions before implementing them in the physical environment.
Quality assurance also benefits significantly. Digital twins integrated with computer vision can detect defects in materials or finished goods in real time, ensuring that issues are flagged immediately rather than post-inspection. This real-time feedback loop enhances product consistency, reduces waste, and streamlines compliance with industry standards such as ISO 9001 or Six Sigma.
Energy: Predictive Maintenance and Grid Optimization
The energy sector—particularly utilities and renewable energy providers—has embraced AI-powered digital twins to address the challenges of system reliability, demand forecasting, and asset management.
For instance, wind farms use digital twins to model turbine behavior, integrating real-time SCADA (Supervisory Control and Data Acquisition) data with historical performance and environmental variables. AI models detect early-stage anomalies in gearboxes or blades, which are difficult to observe manually or with traditional analytics. Predictive maintenance based on these insights reduces repair costs, increases turbine availability, and extends asset lifespan.
In power grid management, AI-enhanced digital twins provide utilities with the ability to balance loads in real time. By simulating various generation and consumption patterns—accounting for solar, wind, and demand-side fluctuations—grids can dynamically redistribute power, reduce congestion, and prevent blackouts. These models also help operators test resilience strategies against cyber-attacks or extreme weather events, reinforcing infrastructure robustness.
Healthcare: Personalized Care and Clinical Decision Support
In healthcare, digital twins are being applied to human physiology itself. Patient-specific digital twins, powered by AI, simulate organs, systems, or entire bodies to support personalized diagnostics, treatment planning, and post-procedural care.
For instance, digital twins of the cardiovascular system can be calibrated using MRI scans, blood pressure data, and genetic profiles to simulate how a patient’s heart responds to different medication dosages or surgical interventions. These simulations, enhanced by AI-driven pattern recognition, help clinicians predict complications and optimize therapeutic strategies.
In hospitals, digital twins of operating rooms or intensive care units integrate IoT and environmental sensors with AI to ensure compliance with safety protocols, predict equipment failures, and streamline workflows. Predictive models can alert staff to upcoming equipment maintenance needs or forecast patient influx trends to optimize staffing levels.
Additionally, AI-powered twins are playing a vital role in remote patient monitoring. For example, wearable devices feeding real-time data into a patient’s twin can trigger alerts when metrics deviate from safe baselines, allowing for proactive intervention without a hospital visit.
Smart Cities: Infrastructure Intelligence and Public Safety
Urban infrastructure is rapidly becoming more intelligent through the deployment of digital twins that represent everything from buildings and bridges to roadways and public transit systems. These city-scale twins enable municipalities to make data-informed decisions that improve efficiency, sustainability, and citizen safety.
Consider the use of AI-enhanced digital twins in transportation. Traffic signals, road sensors, and GPS data from vehicles are integrated into a city-wide twin, which uses machine learning to simulate and optimize traffic flow. These simulations predict congestion before it happens and adjust signal timings in real time to improve mobility and reduce emissions.
Structural monitoring of infrastructure such as bridges or tunnels is another critical application. Digital twins enhanced with vibration, stress, and temperature sensors can detect early signs of material fatigue or structural failure. AI models identify patterns that suggest risk, prompting maintenance or structural reinforcements before safety is compromised.
Public safety also benefits. City twins can integrate feeds from surveillance cameras, emergency response systems, and environmental sensors to simulate emergency scenarios, allocate resources effectively, and coordinate responses to real-world incidents such as fires, floods, or public disturbances.
Logistics and Supply Chain: Operational Efficiency and Fleet Performance
In logistics, AI-enhanced digital twins are being used to track the performance of entire fleets, optimize routes, and streamline warehouse operations. These systems provide end-to-end visibility, connecting vehicle telemetry, driver behavior, cargo conditions, and route planning data into a unified digital model.
A fleet management twin, for instance, can use AI to analyze historical delivery data alongside real-time traffic, weather, and driver fatigue indicators. This enables dynamic route optimization, fuel efficiency improvements, and compliance with regulatory mandates such as HOS (Hours of Service).
In warehouse management, digital twins simulate inventory flow, shelf life, and pick-pack-ship operations. AI-driven insights reveal bottlenecks in picking routes, underutilized storage zones, or misaligned labor schedules. These insights allow logistics providers to reconfigure operations without disrupting live workflows.
The combination of AI and digital twins in this sector supports just-in-time delivery models, enhances asset utilization, and minimizes costs associated with fuel, labor, and equipment downtime.
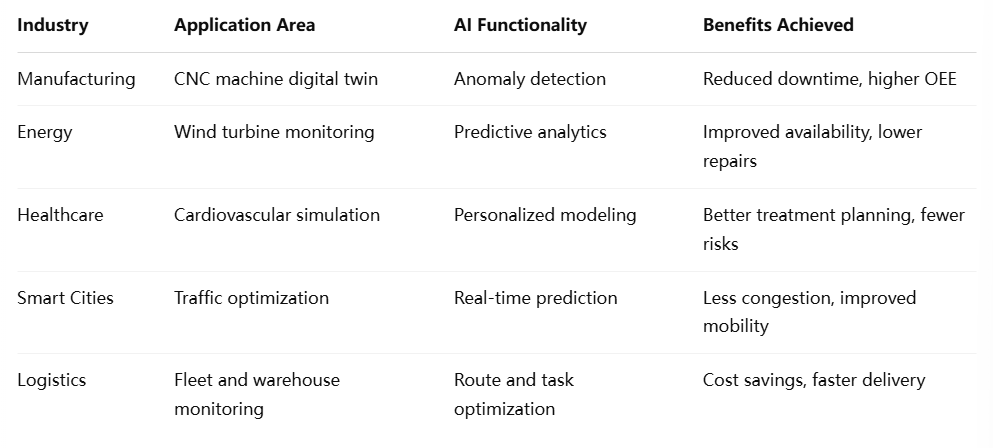
Broader Strategic Outcomes
Beyond the specific operational gains, the adoption of AI-enhanced digital twins across industries is yielding broader strategic benefits. Organizations report:
- Improved agility, as real-time insights allow faster adaptation to changing market or environmental conditions.
- Data democratization, enabling cross-functional teams to make informed decisions using the same digital infrastructure.
- Innovation acceleration, as simulation and testing can occur in the virtual domain, reducing physical trial-and-error.
- Sustainability gains, due to optimized resource usage and waste reduction through predictive control mechanisms.
As these examples illustrate, the AI-enhanced digital twin is not a one-size-fits-all solution, but a highly adaptable toolset capable of being tailored to the nuances of any domain. Its flexibility and intelligence make it a foundational element of enterprise digital transformation strategies.
Challenges, Risks, and Ethical Considerations
While AI-enhanced digital twins present significant opportunities across a wide array of industries, their deployment is not without considerable challenges. As with any transformative technology, the integration of AI into real-time digital replicas raises technical, organizational, and ethical concerns that must be addressed to ensure robust, secure, and responsible adoption. Understanding these constraints is essential for enterprises seeking to leverage digital twins not only as innovation tools, but also as components of critical infrastructure.
This section explores the primary limitations and risks associated with the implementation of AI-powered digital twins, including system complexity, data integrity, model reliability, cybersecurity, ethical deployment, and regulatory compliance.
Technical Complexity and System Integration
The development and deployment of AI-enhanced digital twins require sophisticated integration across a diverse technological stack. Real-time monitoring demands that digital twins interface seamlessly with numerous sources, including IoT devices, enterprise resource planning (ERP) systems, manufacturing execution systems (MES), and cloud-based analytics platforms. This level of interoperability can be difficult to achieve, particularly in environments that contain legacy systems with proprietary protocols or inconsistent data formats.
Further complicating integration is the need for real-time data processing at scale, often involving high-frequency sensor inputs, video streams, or transactional logs. These inputs must be normalized, synchronized, and transmitted across distributed computing environments—ranging from edge devices to cloud servers—without introducing latency or data loss. Orchestrating such a dynamic infrastructure requires not only investment in compute resources, but also highly skilled personnel with cross-disciplinary expertise in AI, systems engineering, and networking.
Moreover, enterprises must grapple with platform fragmentation. The absence of universally accepted standards for digital twin modeling and deployment means that solutions are often vendor-specific or require significant customization. This reduces portability and increases the risk of vendor lock-in, limiting long-term flexibility.
Data Privacy, Security, and Governance
Given the data-intensive nature of digital twins, cybersecurity and data governance become critical concerns. These systems often process sensitive operational data, personally identifiable information (PII), or regulated health records. Unauthorized access, data leakage, or malicious model manipulation can lead to financial loss, legal consequences, and reputational damage.
AI models embedded within digital twins are also vulnerable to adversarial attacks, in which attackers exploit model weaknesses to induce incorrect predictions or recommendations. For example, in a smart grid scenario, tampering with input data streams could cause a digital twin to misallocate loads, leading to instability or outages.
Securing a digital twin environment therefore requires a multilayered approach that includes:
- Encrypted data transmission and secure APIs.
- Role-based access controls and user authentication.
- Continuous monitoring for anomalous activity.
- Data anonymization where appropriate.
Equally important is the implementation of robust data governance policies. Organizations must define who owns the data, how it is stored, how long it is retained, and how it can be used or shared. In multi-stakeholder environments—such as healthcare or transportation—data governance must also account for inter-organizational agreements and legal boundaries.
Model Accuracy, Drift, and Transparency
The reliability of AI-enhanced digital twins is inherently dependent on the quality and consistency of the underlying AI models. Poorly trained or improperly maintained models can result in inaccurate simulations, flawed forecasts, and suboptimal decision-making. This is particularly problematic in safety-critical domains such as aerospace, energy, or healthcare, where inaccurate predictions may result in catastrophic consequences.
One key issue is model drift, which occurs when a model’s performance degrades over time due to changes in the environment, system behavior, or data patterns. For example, a model trained on past sensor data from a machine may become outdated as the machine ages or is reconfigured. Without ongoing retraining and validation, the digital twin may fail to detect emerging anomalies or misrepresent the system state.
To mitigate this risk, organizations must implement:
- Continuous learning pipelines that update models as new data becomes available.
- Validation protocols that test model predictions against real-world outcomes.
- Model explainability tools that allow users to understand how conclusions were reached.
The latter is especially important in regulated industries. Black-box models that cannot justify their outputs may not be permissible under data protection laws such as the European Union’s General Data Protection Regulation (GDPR), which grants individuals the right to explanation for automated decisions.
Ethical Considerations in Deployment
The ethical use of AI-powered digital twins is an increasingly pressing issue, particularly as these systems begin to influence autonomous decisions in areas that impact human lives and societal outcomes. Ethical concerns arise in several forms:
- Bias and Fairness:
AI models may reflect or amplify existing biases in the data used to train them. In healthcare, for example, models trained predominantly on data from one demographic group may fail to provide accurate predictions for others, leading to inequitable care. - Surveillance and Consent:
In smart city deployments, digital twins that incorporate video analytics, motion tracking, or biometric sensors may infringe on personal privacy if implemented without adequate transparency or consent mechanisms. - Autonomy and Accountability:
As digital twins take on greater decision-making responsibilities, questions arise regarding accountability in the event of failure. If a digital twin controlling a fleet of autonomous vehicles makes a suboptimal decision leading to harm, who is responsible—the software vendor, the system integrator, or the end-user?
To address these concerns, enterprises should adhere to AI ethics frameworks such as those proposed by the IEEE, the World Economic Forum, or national AI commissions. These typically emphasize principles such as fairness, accountability, transparency, and human oversight. Establishing Ethics Review Boards and conducting impact assessments before deployment can also help ensure responsible innovation.
Economic and Organizational Challenges
Beyond technical and ethical considerations, there are practical concerns related to cost, change management, and workforce readiness. Implementing AI-enhanced digital twins can entail substantial upfront investment in hardware, software licenses, integration services, and training. For small- to medium-sized enterprises, these costs may be prohibitive unless offset by clear ROI projections.
Additionally, organizational resistance can hinder adoption. Employees may be skeptical of automation initiatives that appear to threaten their roles or require them to adopt unfamiliar technologies. Overcoming such resistance requires transparent communication, inclusive planning, and the development of reskilling programs that empower employees to work alongside intelligent systems rather than feel displaced by them.
A further complication is the shortage of skilled professionals. Developing, deploying, and maintaining AI-driven digital twins demands expertise in AI/ML, systems architecture, cybersecurity, and domain-specific operations—an intersection of skills that remains scarce in the global labor market.
Conclusion and Strategic Outlook
AI-enhanced digital twins represent one of the most consequential advancements in the evolution of real-time monitoring and intelligent operations. By combining the fidelity of digital replicas with the predictive and autonomous capabilities of artificial intelligence, organizations can achieve unprecedented levels of situational awareness, operational efficiency, and strategic agility. What began as a tool for simulation and diagnostics has now matured into an intelligent, adaptive framework capable of driving real-time decision-making across critical infrastructures and complex systems.
The adoption of these intelligent twins is already reshaping industries. In manufacturing, they are improving productivity and product quality while reducing downtime. In energy, they are optimizing grid stability and asset longevity. In healthcare, they are supporting personalized care models and enhancing patient safety. In cities and logistics, they are contributing to safer, more sustainable, and more responsive environments. As these applications continue to mature, they will not only deliver operational benefits but also enable entirely new business models centered around predictive services, outcome-based contracts, and dynamic asset management.
Looking forward, the strategic role of AI-enhanced digital twins will continue to expand. They are expected to form the backbone of the next-generation enterprise architecture—integrating with enterprise resource planning (ERP) systems, feeding data into strategic dashboards, and acting as key nodes in a broader network of cyber-physical intelligence. The increasing convergence of digital twins with technologies such as 5G, blockchain, extended reality (XR), and generative AI will further amplify their capabilities and unlock synergies previously considered unattainable.
However, to fully realize this potential, organizations must approach implementation with a balanced perspective. Technical excellence must be accompanied by ethical rigor, data security must be prioritized alongside scalability, and stakeholder inclusion must be embedded within innovation strategies. Investing in robust governance frameworks, continuous learning systems, and cross-functional talent will be essential to sustaining the benefits and mitigating the risks of these powerful technologies.
In the final analysis, AI-enhanced digital twins are not merely an upgrade to traditional monitoring systems—they are a paradigm shift. They move organizations from reactive surveillance to anticipatory intelligence, from static insights to dynamic optimization, and from human-led control to machine-assisted autonomy. As the global economy continues its digital transformation, these intelligent twins will play a defining role in shaping the infrastructure, resilience, and adaptability of the enterprises and societies of tomorrow.
References
- What are digital twins?
https://www.ibm.com/topics/what-is-a-digital-twin - Digital Twin Technology
https://www.ge.com/digital/solutions/digital-twin - Digital Twins: Bridging the Physical and Digital
https://new.siemens.com/global/en/company/stories/industry/digital-twins.html - Azure Digital Twins Overview
https://azure.microsoft.com/en-us/services/digital-twins/ - Smart operations with AI-powered digital twins
https://www2.deloitte.com/global/en/pages/consulting/articles/ai-digital-twin.html - Digital twins: Driving value beyond the buzz
https://www.mckinsey.com/business-functions/mckinsey-digital/our-insights/digital-twins - The Power of AI in Digital Twins
https://www.ptc.com/en/blogs/iiot/ai-digital-twins - Emerging Tech Impact Radar: Digital Twins
https://www.gartner.com/en/articles/emerging-technologies-digital-twin - Building AI Digital Twins with Omniverse
https://www.nvidia.com/en-us/omniverse/digital-twin/ - How AI and digital twins are reshaping infrastructure
https://www.weforum.org/agenda/2023/06/ai-digital-twins-infrastructure-sustainability/