How AI-Powered SaaS Platforms Are Transforming Drug Discovery from Lab to Cloud
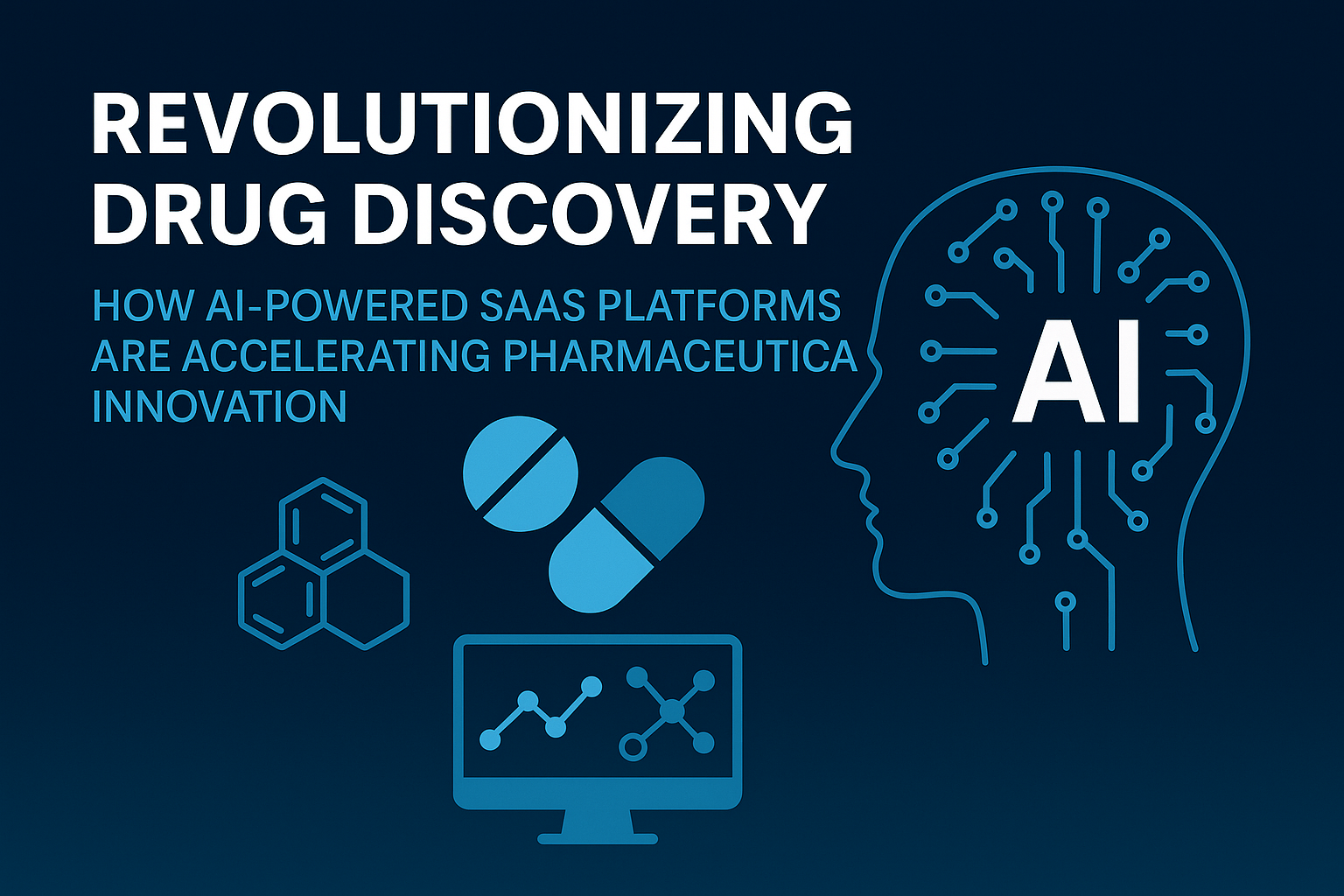
The pharmaceutical industry has long been characterized by its intricate, costly, and time-intensive drug discovery processes. Developing a new drug traditionally requires a decade or more and can cost upwards of $2 billion, with a high rate of attrition and regulatory hurdles compounding the complexity. Despite significant advancements in molecular biology, genetics, and high-throughput screening, many aspects of drug discovery remain locked in a pattern of inefficiency and unpredictability.
In recent years, however, the convergence of artificial intelligence (AI) and cloud-based software delivery—specifically the Software-as-a-Service (SaaS) model—has begun to transform this landscape. This shift represents more than just a technological upgrade; it marks a fundamental realignment of how scientific knowledge, computational tools, and biological data intersect to yield tangible medical solutions.
AI technologies now possess the capability to sift through vast molecular libraries, predict compound-target interactions with increasing accuracy, and simulate pharmacokinetics long before a drug reaches the preclinical trial phase. These capabilities are being seamlessly integrated into SaaS platforms that offer scalable, accessible, and continuously updated environments tailored for researchers, clinicians, and biopharmaceutical companies alike.
What distinguishes the SaaS model in this context is its democratizing effect. Previously, only well-capitalized pharmaceutical giants could afford the infrastructure and personnel necessary for AI-driven drug discovery. Today, SaaS platforms enable startups, academic labs, and medium-sized biotech firms to harness cutting-edge AI models through subscription-based services, thus leveling the playing field and accelerating innovation across the board.
This blog post explores the multifaceted ways in which the SaaS model is revolutionizing drug discovery through AI. From examining the historical context and core technologies to showcasing real-world applications and future implications, we aim to provide a comprehensive understanding of this transformation. In doing so, we underscore how this convergence is not only enhancing R&D productivity but also paving the way for more targeted, efficient, and equitable healthcare solutions.
The Evolution of Drug Discovery and the Entry of AI
The process of drug discovery has historically been an endeavor of immense complexity, marked by a combination of scientific rigor, empirical trial-and-error, and often, serendipitous breakthroughs. From the isolation of penicillin in the early 20th century to the development of blockbuster small-molecule therapies in the 1990s, the evolution of pharmaceutical research has largely relied on laborious in vitro and in vivo experimentation. Despite incremental advancements in genomics and high-throughput screening, the underlying methodologies have remained relatively static in their reliance on manual hypothesis testing and biological validation.
Traditional Drug Discovery: Timelines, Bottlenecks, and Costs
At the core of traditional drug discovery lies a sequential pipeline comprising target identification, compound screening, hit-to-lead optimization, preclinical testing, and clinical trials. This linear process often spans 10 to 15 years and requires billions in investment. For every 10,000 compounds screened, only one might eventually be approved for therapeutic use. Attrition rates are particularly severe during the clinical trial phase, where failures due to efficacy, toxicity, or pharmacokinetics result in substantial financial and time losses.
Several systemic bottlenecks persist. First, the identification of viable biological targets remains a challenge due to incomplete knowledge of disease mechanisms and pathway interactions. Second, compound screening relies heavily on chemical libraries that may not comprehensively represent the vast chemical space. Finally, the optimization of lead compounds is a resource-intensive endeavor, requiring iterative cycles of synthesis, testing, and refinement. In aggregate, these limitations contribute to a high-risk, high-cost paradigm that hinders both innovation and access.
The Role of Informatics and Early Computational Tools
The late 20th century saw the gradual integration of computational tools into the drug discovery pipeline. Bioinformatics enabled more efficient genomic and proteomic analyses, while cheminformatics facilitated the digital representation and comparison of molecular structures. Quantitative structure-activity relationship (QSAR) models and molecular docking simulations allowed researchers to make preliminary predictions about drug-likeness and binding affinity, albeit with limited accuracy and scalability.
Despite these improvements, the majority of computational tools were fragmented and lacked the ability to generalize across diverse datasets. Moreover, the integration of these tools into research workflows required substantial expertise and bespoke infrastructure, limiting their accessibility and widespread adoption.
The Advent of Artificial Intelligence in Drug Discovery
The emergence of artificial intelligence in drug discovery represents a transformative leap rather than a mere extension of computational methodologies. AI, particularly in the form of machine learning (ML) and deep learning (DL), offers the ability to uncover complex, non-linear relationships in biological and chemical data that would otherwise remain obscured. These models are capable of ingesting heterogeneous data sources—ranging from molecular structures and omics data to clinical trial results—and generating actionable insights with unprecedented speed and precision.
Early applications of AI in drug discovery were largely exploratory. For instance, support vector machines and random forest algorithms were employed to classify compounds based on toxicity profiles or predict target binding affinity. However, with the advent of deep neural networks and attention-based architectures, the scope and efficacy of AI applications have grown exponentially. AI models can now generate novel molecular structures, predict polypharmacological effects, and even simulate disease progression in silico.
One of the most notable advancements is the use of generative models—such as variational autoencoders (VAEs), generative adversarial networks (GANs), and reinforcement learning agents—to design drug candidates from scratch. These models navigate the vast chemical space to identify molecules that satisfy predefined pharmacological and physicochemical constraints, thereby accelerating the hit identification and lead optimization stages of drug discovery.
Integration of AI with High-Throughput and Real-World Data
The utility of AI is further amplified when integrated with high-throughput screening technologies and real-world data (RWD). For example, high-content imaging platforms generate massive datasets capturing cellular responses to compounds. AI models can analyze these images to detect subtle phenotypic changes indicative of a compound's mechanism of action. Similarly, the availability of electronic health records (EHRs), patient registries, and pharmacovigilance data enables AI systems to refine predictions of drug efficacy and safety in diverse populations.
Moreover, AI facilitates the repurposing of existing drugs by identifying new therapeutic targets or indications based on shared molecular mechanisms or patient outcomes. This approach not only reduces development time and costs but also increases the likelihood of clinical success, given that the safety profiles of repurposed drugs are often well-established.
Democratization and Commercialization of AI Capabilities
Despite the transformative potential of AI, its adoption in drug discovery was initially limited to organizations with significant computational resources and data science expertise. However, this dynamic is rapidly changing with the rise of Software-as-a-Service (SaaS) models that package AI capabilities into user-friendly, scalable platforms. These platforms abstract away the complexity of model training, data integration, and algorithmic tuning, enabling researchers from diverse backgrounds to leverage state-of-the-art AI tools.
SaaS-based AI platforms are now providing access to pre-trained models, cloud-based computation, and collaborative research environments—all through subscription-based pricing structures. As a result, small biotech firms, academic institutions, and even non-profits can now participate meaningfully in the drug discovery process without the need for extensive capital investment.
A Pivotal Inflection Point
The integration of AI into drug discovery is not merely additive; it is catalytic. It shifts the focus from empirical observation to predictive modeling, from isolated experimentation to integrated ecosystems, and from siloed operations to collaborative innovation. When combined with the SaaS delivery model, AI becomes not just a tool, but a shared infrastructure that democratizes access, accelerates timelines, and ultimately enhances the probability of therapeutic breakthroughs.
As we move forward, understanding this inflection point is critical. It lays the foundation for a new era of drug development—one where innovation is no longer constrained by resource limitations, and where the power of intelligent computation is accessible to all who seek to solve some of the world’s most pressing health challenges.
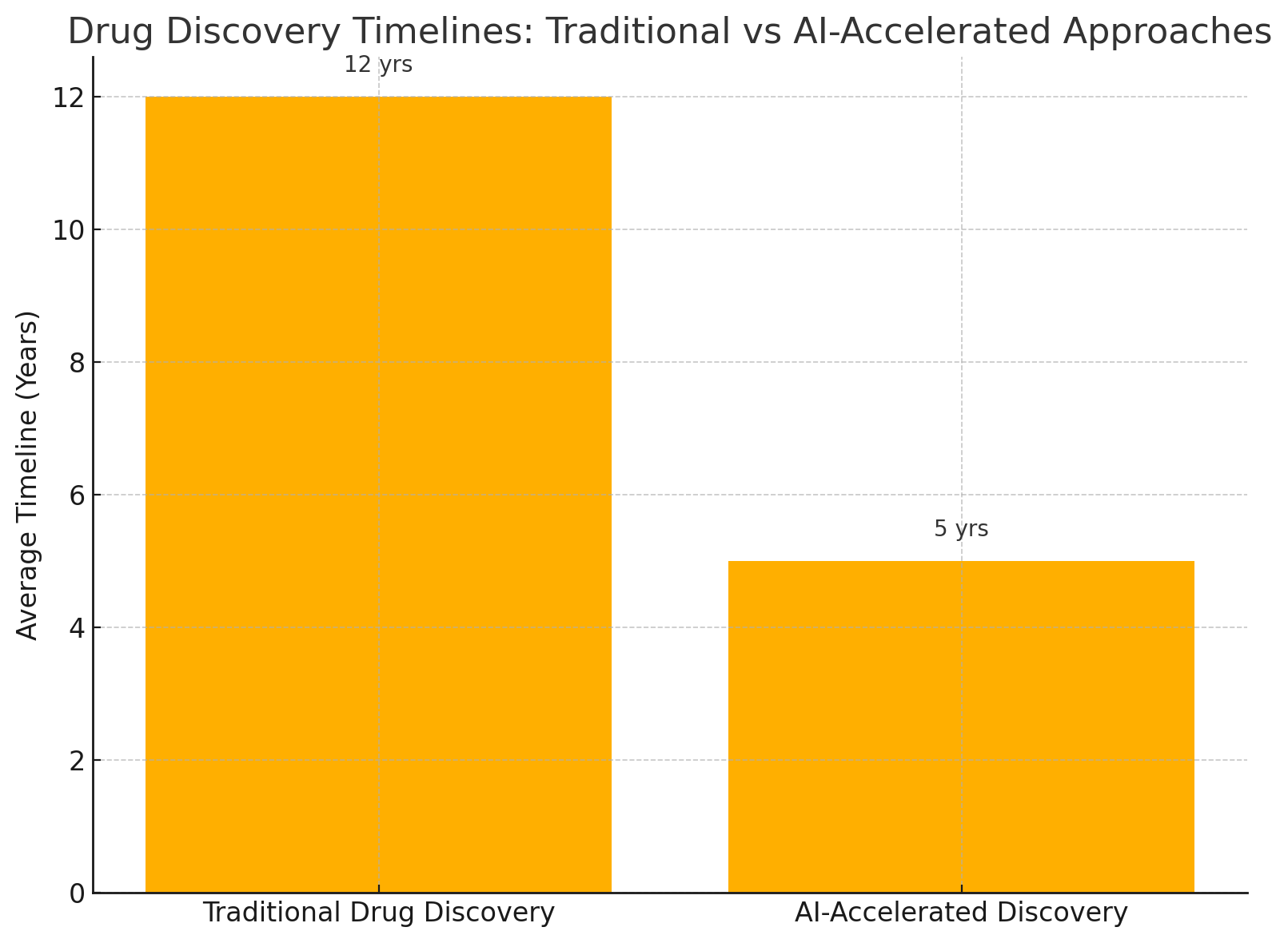
The SaaS Revolution in Life Sciences
The integration of Software-as-a-Service (SaaS) models into the life sciences industry marks a defining evolution in how pharmaceutical and biotech companies access, utilize, and scale computational resources. By transitioning from traditional, on-premises software installations to cloud-native, subscription-based platforms, SaaS has catalyzed a structural shift in the delivery of tools essential for modern drug discovery. When paired with artificial intelligence (AI), this model not only enhances R&D capabilities but also democratizes access to technologies previously available only to well-funded institutions.
What is SaaS and Why It Matters in Drug Discovery
SaaS refers to a method of software delivery in which applications are hosted on the cloud and accessed via the internet, typically through a subscription model. This paradigm offers several advantages over conventional software deployment methods, including scalability, continuous updates, reduced maintenance overhead, and accessibility from any location.
In the context of drug discovery, these benefits are transformative. Traditional software solutions in life sciences often required high upfront capital expenditure, dedicated IT infrastructure, and skilled personnel for maintenance. In contrast, SaaS platforms offer out-of-the-box functionality, with intuitive user interfaces, pre-configured AI pipelines, and seamless integration with cloud-based databases. This allows researchers to focus on scientific inquiry rather than technical troubleshooting.
Moreover, the SaaS model enables real-time collaboration across geographically dispersed teams, facilitates version control in complex research environments, and ensures that users always have access to the latest analytical capabilities and scientific databases.
Core Features of AI-Enabled SaaS Platforms in Drug Discovery
AI-powered SaaS platforms designed for drug discovery typically encompass a suite of interconnected tools that address key stages in the drug development pipeline. These features include:
- Data Integration and Harmonization: SaaS platforms can ingest and normalize data from diverse sources such as omics datasets, clinical trial registries, high-throughput screening results, and literature databases. This integration is critical for constructing robust AI models capable of making biologically meaningful predictions.
- Predictive Modeling and Virtual Screening: Machine learning algorithms hosted on SaaS platforms can predict compound-target interactions, off-target effects, ADMET (absorption, distribution, metabolism, excretion, and toxicity) profiles, and optimal drug formulations. These predictions drastically reduce the need for physical assays during early discovery phases.
- Molecular Generation and Optimization: Generative models accessible via SaaS can design novel compounds tailored to specific biological targets, while reinforcement learning techniques iteratively refine these candidates to enhance efficacy and safety profiles.
- Automated Workflow Orchestration: From hypothesis generation to lead validation, SaaS platforms allow users to automate repetitive tasks, chain analytical operations, and set conditional triggers for experimentation, thereby accelerating research timelines.
- Collaboration and Version Control: With built-in tools for sharing notebooks, visualizing results, and managing datasets, these platforms support collaborative drug discovery initiatives across institutions and disciplines.
Leading SaaS Providers and Use Cases in the Biotech Sector
A growing ecosystem of SaaS providers is reshaping the biotech landscape. Companies such as Benchling, Schrödinger, and BioSymetrics offer AI-enhanced platforms that support drug development workflows from bench to bedside.
- Benchling provides a cloud-based informatics platform tailored for life sciences R&D. Its modular SaaS suite includes electronic lab notebooks, molecular biology tools, and inventory management systems. Benchling is widely used for managing experimental workflows and harmonizing data across departments.
- Schrödinger offers a physics-based modeling and machine learning platform, enabling molecular simulations and predictive analytics in drug design. Through its SaaS delivery, Schrödinger enables biopharma companies to reduce development cycles and increase the probability of identifying viable leads.
- BioSymetrics delivers a SaaS platform optimized for real-world data integration and ML model training. Its focus on structured and unstructured biomedical data makes it a critical tool for translational research and biomarker discovery.
These platforms exemplify how SaaS, when integrated with AI, enhances data interoperability, model reproducibility, and overall research efficiency.
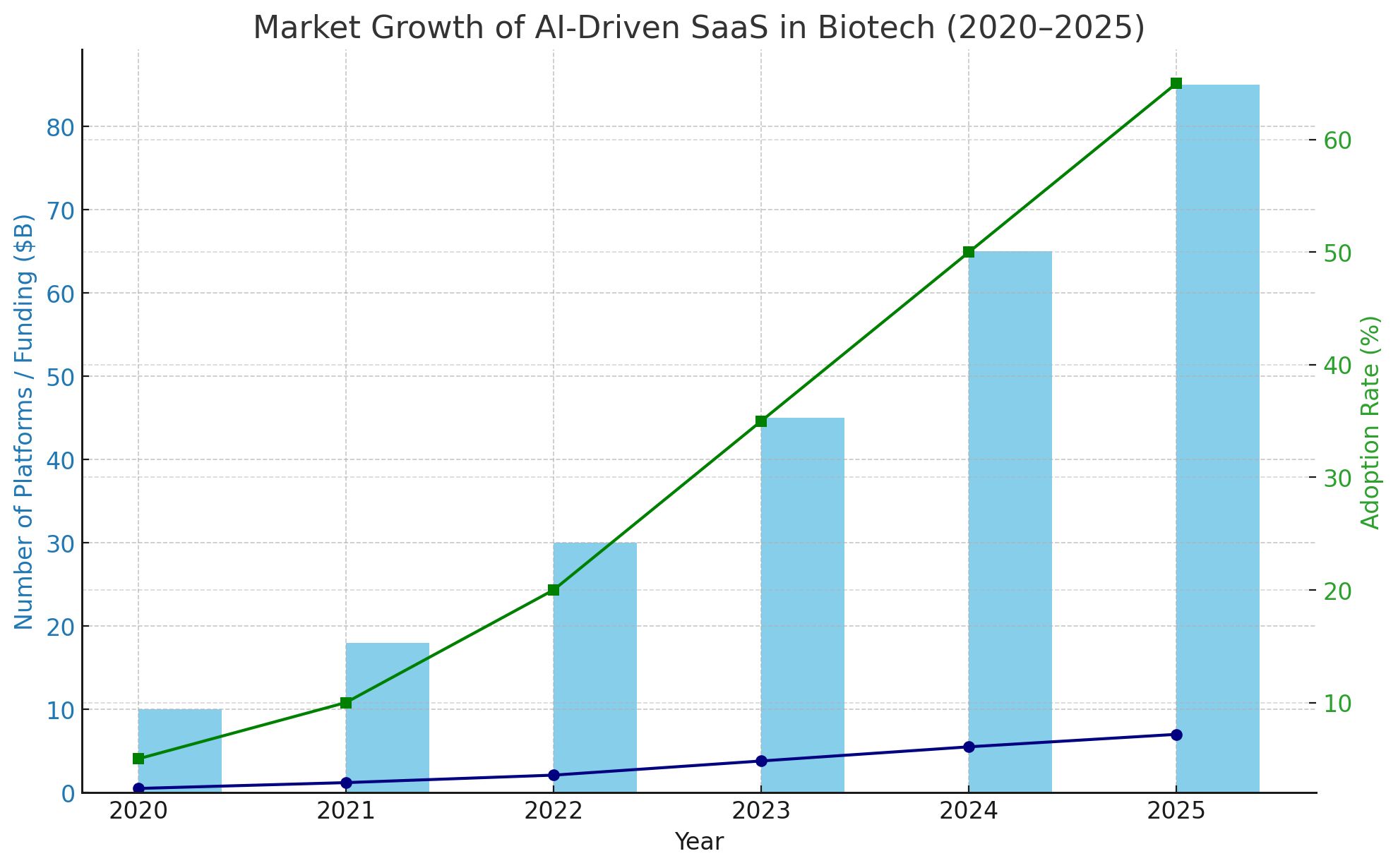
Democratizing Access to Advanced Technologies
One of the most profound impacts of SaaS in life sciences is its role in democratizing access to advanced computational technologies. In the past, only large pharmaceutical companies could afford the infrastructure necessary to host and run AI models internally. Today, SaaS platforms provide equivalent—if not superior—capabilities to a much broader array of users, including academic labs, government research agencies, and early-stage biotech startups.
This democratization has significant implications for global health equity. By reducing the barriers to entry, SaaS allows resource-constrained institutions to participate in cutting-edge drug discovery efforts, including those targeting neglected diseases or rare conditions that may not be prioritized by profit-driven entities.
Furthermore, SaaS platforms often support multi-language interfaces and are accessible via low-bandwidth environments, thereby enabling participation from researchers in low- and middle-income countries (LMICs). This inclusivity fosters a more diverse and globally distributed research community, accelerating innovation through cross-border collaboration.
Cloud-Native Architecture and Security Considerations
The underlying cloud-native architecture of SaaS platforms is instrumental to their scalability and performance. These platforms are typically built using microservices, containerization (e.g., Docker), and orchestration tools (e.g., Kubernetes), enabling elastic scalability based on computational demand.
Security and compliance are paramount in life sciences SaaS applications. Reputable platforms adhere to industry standards such as HIPAA, GDPR, and GxP. Data encryption, audit trails, and access controls are implemented to protect sensitive patient data and proprietary research findings. Additionally, cloud service providers offer robust disaster recovery and business continuity plans, ensuring that research activities can proceed uninterrupted even in the face of localized disruptions.
Interoperability with Laboratory Automation and IoT
An emerging trend is the integration of SaaS platforms with laboratory automation systems and the Internet of Things (IoT). By connecting AI-enabled SaaS tools with automated lab instruments, organizations can create closed-loop experimental systems in which hypotheses are generated, tested, and refined with minimal human intervention. This integration paves the way for real-time optimization of experiments, adaptive research pipelines, and the acceleration of discovery cycles.
In parallel, IoT devices embedded in laboratory equipment can feed environmental and operational data into SaaS platforms for real-time monitoring and quality control. Such capabilities are particularly beneficial in maintaining regulatory compliance and enhancing reproducibility.
Case Studies of AI-SaaS Success in Drug Discovery
The practical impact of AI-enabled Software-as-a-Service (SaaS) platforms in drug discovery is most vividly illustrated through real-world case studies. Several pioneering companies have successfully deployed SaaS models to deliver accelerated timelines, reduce development costs, and improve hit identification accuracy. This section highlights key players—Insilico Medicine, Atomwise, and Recursion Pharmaceuticals—that exemplify the integration of AI and SaaS to reimagine traditional drug discovery paradigms. These case studies underscore how cloud-native, algorithm-driven approaches are reshaping pharmaceutical innovation.
Insilico Medicine: An End-to-End AI Drug Discovery Platform
Founded in 2014, Insilico Medicine has emerged as a leader in AI-driven drug discovery by combining deep generative models with a cloud-based SaaS infrastructure. The company’s end-to-end Pharma.AI platform integrates three core modules: PandaOmics (target discovery), Chemistry42 (molecule generation), and InClinico (clinical trial prediction). Through a unified interface, users can perform target identification, compound design, and clinical viability assessment—processes that traditionally occur in silos over many years.
One of Insilico’s most notable achievements occurred in 2021 when it announced the discovery of a novel preclinical drug candidate for idiopathic pulmonary fibrosis (IPF) in under 18 months, with a development cost of less than $3 million. This milestone was made possible through the application of generative reinforcement learning algorithms hosted via their SaaS platform, which generated and validated molecules with optimal drug-likeness, synthetic accessibility, and ADMET properties.
In 2022, Insilico advanced this candidate into Phase I clinical trials—a rare feat for an AI-designed drug. The significance lies not only in the algorithmic design but in the collaborative nature of its SaaS platform, which allowed interdisciplinary teams to interact across data layers and iteratively refine compound profiles based on real-time analytics.
The company’s growing customer base spans academic institutions, mid-sized biotech firms, and global pharmaceutical corporations, all of whom leverage Insilico’s subscription-based platform to reduce R&D costs and improve clinical success rates.
Atomwise: Democratizing Virtual Screening through SaaS
Atomwise specializes in the application of deep learning to structure-based drug design, with a strong emphasis on virtual screening. Its core technology, AtomNet, employs convolutional neural networks to predict the binding affinity between small molecules and protein targets based on 3D structural data. This predictive capability is deployed through an enterprise-grade SaaS platform that allows researchers to screen millions of compounds virtually before any wet-lab validation.
The scalability of Atomwise’s SaaS model is particularly noteworthy. As of 2024, the company has engaged in over 750 partnerships with academic laboratories, biotechnology startups, and pharmaceutical companies. These collaborations span therapeutic areas including oncology, neurology, infectious diseases, and autoimmune disorders.
One compelling example is Atomwise’s partnership with Baylor College of Medicine, in which its SaaS platform was used to identify a novel candidate for treating Ebola virus infections. Within weeks, the platform screened over 10 million compounds and prioritized a short list of 60 candidates for in vitro validation, significantly compressing what would typically be a months-long process.
Atomwise’s pay-per-project licensing model makes its platform attractive to smaller organizations that may lack in-house screening infrastructure. Additionally, the platform’s user-centric interface allows researchers without extensive AI expertise to conduct large-scale computational experiments, thereby expanding the reach and impact of AI in biomedical research.
Recursion: Merging AI with Automated Labs for Phenotypic Drug Discovery
Recursion Pharmaceuticals offers a distinct approach to AI-SaaS integration by combining high-throughput cellular imaging with deep learning and robotic lab automation. Its Recursion OS platform generates terabytes of high-content biological data daily by perturbing cells with thousands of small molecules and capturing resultant phenotypic changes via microscopy.
These images are analyzed using proprietary convolutional neural networks to identify phenotypic “fingerprints” that suggest therapeutic potential. The cloud-based SaaS interface allows users to query this vast data lake for compounds that exhibit desired biological effects across different disease models.
Recursion’s capabilities have led to a portfolio of over 20 internal and partnered drug programs, with a particular focus on rare genetic diseases. In one notable case, the company identified a candidate for cerebral cavernous malformation (CCM) through phenotypic screening and AI prioritization, moving the compound into clinical trials in record time.
The SaaS model plays a critical role in enabling external partners to access Recursion’s data and models securely. Partners can interact with the platform’s AI tools, visualize phenotypic maps, and conduct virtual experiments without requiring on-site access to Recursion’s lab infrastructure. This approach not only optimizes collaboration but also allows for secure data partitioning and real-time model updates.
Comparative Overview of AI-SaaS Drug Discovery Platforms
The following table presents a comparative summary of the aforementioned platforms, highlighting their core competencies, technological differentiators, and operational models.
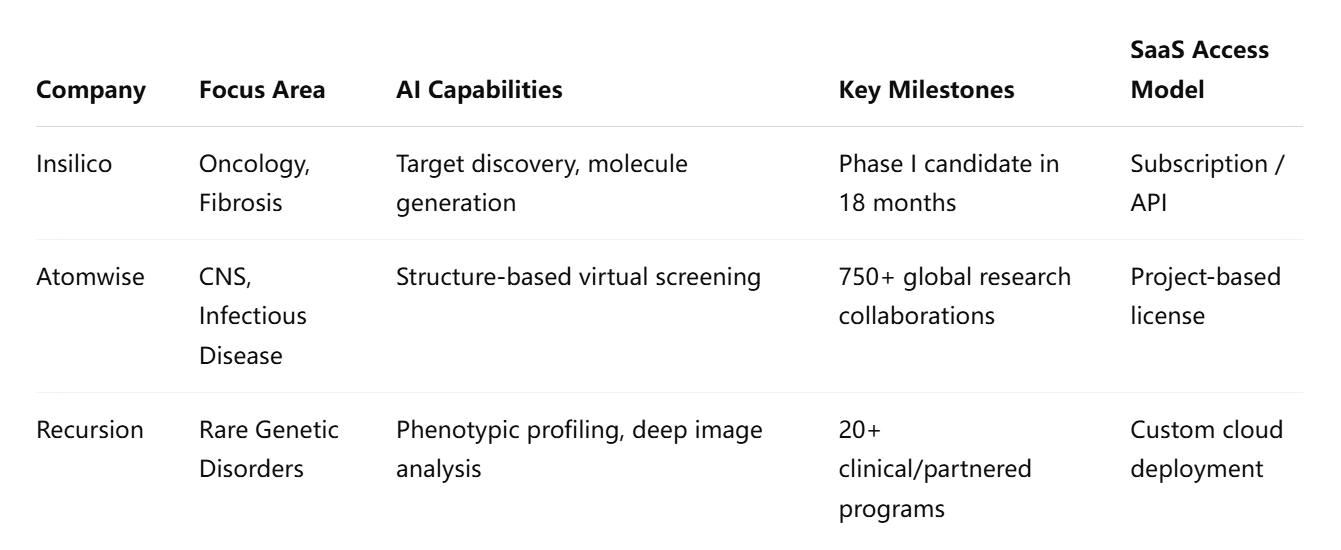
This comparative overview reflects a shared commitment to transforming drug discovery into a more agile, cost-effective, and accessible endeavor. While each company’s platform addresses distinct stages of the R&D pipeline, they all leverage SaaS to provide scalable, user-friendly, and collaborative environments that transcend the limitations of traditional infrastructure.
Broader Implications and Industry Validation
The success of these companies has not gone unnoticed by the broader biopharmaceutical industry. Strategic partnerships, licensing agreements, and venture investments have surged, signaling institutional confidence in the SaaS model. Furthermore, regulatory agencies such as the U.S. Food and Drug Administration (FDA) and the European Medicines Agency (EMA) have begun issuing guidance on the use of AI and real-world evidence in drug development, paving the way for broader adoption of these technologies.
AI-SaaS platforms are also being integrated into academic curricula and publicly funded research initiatives, further embedding them into the scientific ecosystem. The transition from isolated, computational experiments to shared, cloud-enabled platforms is enabling more reproducible research and fostering cross-disciplinary innovation.
Challenges, Ethics, and the Future Landscape
While the convergence of artificial intelligence (AI) and Software-as-a-Service (SaaS) platforms has introduced a new paradigm in drug discovery, this transformation is not without its complexities. Technical limitations, ethical concerns, and evolving regulatory frameworks continue to pose significant challenges. As the biopharmaceutical industry increasingly relies on AI-enabled SaaS models, it must address issues surrounding data quality, algorithmic transparency, model validation, and equitable access. Simultaneously, stakeholders must prepare for a rapidly changing landscape shaped by emerging technologies and a shifting regulatory and societal context.
Data Quality, Standardization, and FAIR Principles
High-quality data is the bedrock of effective AI modeling. In drug discovery, this includes omics datasets, chemical structures, high-content imaging, clinical trial records, and real-world evidence (RWE) such as electronic health records (EHRs). However, such data are often fragmented, incomplete, or biased. Legacy systems within pharmaceutical companies can store data in proprietary formats, and integration across platforms or institutions remains a non-trivial challenge.
SaaS platforms aim to mitigate this issue through standardized data ingestion protocols and adherence to the FAIR principles—Findability, Accessibility, Interoperability, and Reusability. Nevertheless, inconsistencies in metadata annotation, differences in nomenclature, and data silos can significantly reduce model accuracy and generalizability. Ensuring data quality, provenance tracking, and rigorous validation pipelines is essential to maintain the reliability of AI predictions within SaaS ecosystems.
Furthermore, the challenge of “data drift”—where underlying patterns in data change over time due to shifts in clinical practice or biological understanding—necessitates continuous model retraining and validation. SaaS platforms must incorporate feedback loops to monitor data relevance and model performance in real-time.
Transparency, Interpretability, and Trust in AI Models
AI models, particularly deep learning architectures, are often criticized as “black boxes” due to their lack of interpretability. In the context of drug discovery, this opacity raises critical concerns regarding trust, reproducibility, and regulatory acceptance. Researchers, clinicians, and regulatory bodies require explanations for why a specific compound was prioritized or how a model inferred a target-disease relationship.
SaaS providers are responding by incorporating Explainable AI (XAI) features into their platforms. These include feature attribution methods, saliency maps for imaging data, and model auditing tools that trace decision-making pathways. Despite these efforts, interpretability remains an open research challenge, especially as models grow in complexity.
The tension between model performance and transparency must be carefully managed. In high-stakes applications such as drug development, stakeholders may favor slightly less accurate but interpretable models over opaque architectures with marginally higher predictive power. SaaS platforms must provide users with the flexibility to select models based on their specific risk tolerance and regulatory requirements.
Model Bias, Reproducibility, and Overfitting
Bias in AI models can arise from unbalanced datasets, flawed assumptions, or poorly specified objectives. In drug discovery, this can result in algorithms that favor compounds active against well-studied targets or that underperform in underrepresented populations. For instance, training a model exclusively on data from one ethnic group may compromise its utility in global health applications.
SaaS platforms must incorporate mechanisms to detect and mitigate bias. This includes demographic stratification, fairness audits, and diverse dataset augmentation. Moreover, reproducibility—a cornerstone of scientific validity—must be preserved by providing users with standardized pipelines, version-controlled datasets, and transparent model training protocols.
Overfitting is another prevalent issue, especially when models are trained on limited or noisy data. SaaS platforms must implement cross-validation, regularization techniques, and robust performance metrics to ensure that models generalize well to novel inputs. Additionally, reproducibility and peer-reviewed validation should be encouraged to maintain scientific rigor.
Ethical Considerations in Algorithm-Driven Drug Design
As AI assumes a greater role in shaping therapeutic pipelines, ethical questions come to the fore. Who is accountable if an AI-designed compound leads to adverse outcomes in clinical trials? What safeguards are in place to prevent dual-use applications, such as the design of harmful biological agents? How can stakeholders ensure that algorithmic innovations do not exacerbate healthcare disparities?
One pressing concern is informed consent in the context of real-world data used for model training. Patients may not be fully aware that their anonymized health records are being used to train drug discovery algorithms, raising questions about autonomy, data ownership, and secondary use.
SaaS platforms must uphold stringent ethical standards, including transparent data governance policies, ethical review boards, and opt-out mechanisms for data contributors. Moreover, public engagement is vital to foster societal trust in AI-driven drug development. Stakeholders should strive to cultivate a dialogue that includes ethicists, patients, clinicians, and technologists.
Regulatory and Legal Landscape
Regulatory bodies worldwide are grappling with the implications of AI in life sciences. While agencies such as the FDA and EMA have issued preliminary guidelines on the use of AI and real-world data, a comprehensive regulatory framework is still evolving. SaaS providers must design their platforms to align with existing regulations while remaining adaptable to future changes.
Key regulatory considerations include:
- Model traceability and documentation: All decision-making pathways must be auditable.
- Validation requirements: AI predictions used to advance drug candidates must be experimentally validated through independent replication.
- Cybersecurity and data privacy: Platforms must comply with HIPAA, GDPR, and other relevant standards.
In anticipation of forthcoming regulations, some SaaS providers have begun offering built-in compliance modules that assist users in preparing documentation for regulatory submissions. This proactive approach reduces barriers to clinical adoption and fosters trust among stakeholders.
Future Outlook: Towards AI-Native Drug Discovery
Looking forward, the fusion of AI and SaaS is expected to deepen, giving rise to what may be termed “AI-native drug discovery.” In this paradigm, drug candidates will be conceived, optimized, and validated in silico before any laboratory interaction occurs. Advances in quantum computing, neuromorphic chips, and foundation models will further augment the predictive capabilities of SaaS platforms.
Emerging trends include:
- Multimodal modeling: Integrating genomics, proteomics, imaging, and EHRs into unified models for more comprehensive predictions.
- Synthetic biology and AI: SaaS platforms will increasingly incorporate gene-editing simulations, pathway engineering, and synthetic circuit design.
- Digital twins: Personalized virtual replicas of patients will be used to simulate drug responses, enabling tailored therapeutics.
Moreover, the industry is likely to witness a convergence of AI-SaaS platforms with decentralized clinical trial platforms, real-time biosensors, and blockchain-based data exchange protocols. These integrations will enable end-to-end digital ecosystems that support the full drug development lifecycle—from hypothesis generation to market access.
A Transformative, Yet Cautious Path Forward
Despite its promise, the AI-SaaS model for drug discovery must be approached with both optimism and prudence. It offers immense potential to accelerate timelines, reduce costs, and improve health outcomes. Yet it also demands careful stewardship to ensure that its deployment is ethical, equitable, and scientifically sound.
Stakeholders—including biotech firms, regulators, academia, and patient advocacy groups—must collaborate to develop best practices, establish shared governance models, and foster innovation ecosystems that prioritize public good. In doing so, they can ensure that the next generation of therapeutics is not only more effective but also more responsibly developed and equitably distributed.
Enabling the Future of Drug Discovery through AI and SaaS
The drug discovery process, long hindered by inefficiencies, protracted timelines, and high costs, is undergoing a profound transformation. At the heart of this shift lies the convergence of artificial intelligence (AI) and Software-as-a-Service (SaaS), two technological forces that, when combined, have the capacity to fundamentally redefine how new therapies are discovered, developed, and delivered.
AI brings to the table unparalleled analytical power—identifying patterns in complex biological data, optimizing molecular structures, and predicting clinical outcomes with increasing accuracy. Meanwhile, the SaaS model operationalizes this intelligence, delivering scalable, cloud-native platforms that offer accessibility, real-time collaboration, and ongoing innovation without the overhead of traditional software infrastructures. Together, these technologies are democratizing access to advanced drug discovery tools, empowering researchers across geographies and institutional scales to participate in cutting-edge pharmaceutical innovation.
The case studies of Insilico Medicine, Atomwise, and Recursion illustrate the practical impact of AI-SaaS integration. These platforms are not theoretical constructs but operational solutions already yielding viable drug candidates, accelerating timelines, and enabling broader participation in the biomedical sciences. They also highlight the importance of interdisciplinary collaboration and shared digital infrastructure in driving meaningful scientific outcomes.
Nonetheless, challenges persist. Issues related to data quality, model transparency, ethical governance, and regulatory compliance must be navigated with care. The development of robust frameworks for ethical AI use, standardized data practices, and transparent validation protocols will be essential to ensure that the benefits of these technologies are equitably realized.
Looking ahead, the path is clear: the fusion of AI and SaaS is not a fleeting trend, but a structural evolution in how life sciences operate. As platforms become increasingly sophisticated, and as stakeholders embrace open innovation, the dream of faster, safer, and more personalized medicine moves closer to reality. By harnessing the power of intelligent automation and cloud-based delivery, the industry is laying the foundation for a more responsive and inclusive era of drug discovery—one in which scientific excellence and global health priorities can be more closely aligned than ever before.
References
- Recursion Pharmaceuticals – https://www.recursion.com
- Benchling Life Sciences R&D Platform – https://www.benchling.com
- Schrödinger Drug Discovery Platform – https://www.schrodinger.com
- FDA Guidance on Artificial Intelligence in Drug Development – https://www.fda.gov/media/167973/download
- EMA Regulatory Reflection Paper on AI in Medicinal Products – https://www.ema.europa.eu/en/documents/other/reflection-paper-artificial-intelligence-ai-medicinal-products_en.pdf
- BioSymetrics Platform Overview – https://www.biosymetrics.com
- Nature Reviews Drug Discovery: AI and Machine Learning – https://www.nature.com/nrd/articles?searchType=journalSearch&sort=PubDate&type=review
- Harvard Data Science Review – AI in Drug Discovery – https://hdsr.mitpress.mit.edu/pub/ai-drug-discovery