How AI is Transforming Parkinson’s Care: Diagnosis, Treatment, and Daily Life Support
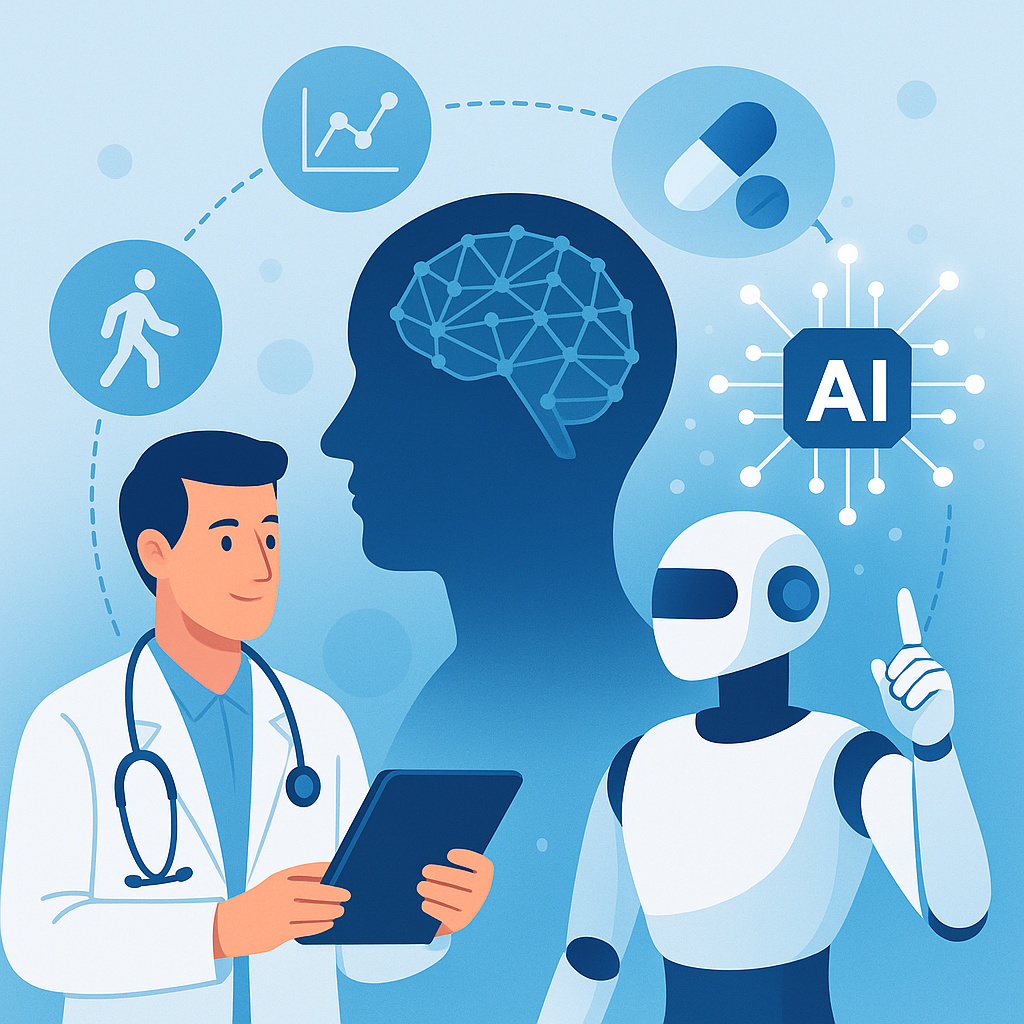
Parkinson’s disease (PD) is a progressive neurodegenerative disorder characterized primarily by motor symptoms such as tremors, rigidity, bradykinesia, and postural instability. However, the full spectrum of the disease extends beyond motor impairment, encompassing a wide range of non-motor symptoms including sleep disturbances, mood disorders, cognitive decline, and autonomic dysfunction. Affecting over 10 million people globally, Parkinson’s presents a profound and ongoing challenge for healthcare systems, patients, and caregivers alike. The burden is compounded by the complexity of its progression, the variability of symptoms, and the lack of a definitive cure. Despite decades of research, early diagnosis remains difficult, and treatment is largely symptomatic rather than disease-modifying.
The traditional paradigm of Parkinson’s care involves a combination of clinical observation, neurological examinations, patient-reported outcomes, and pharmacological interventions, most notably the administration of levodopa. While effective to an extent, these approaches are constrained by the limitations of subjective assessments and intermittent monitoring. Furthermore, variability in individual responses to medication often necessitates a trial-and-error approach to dosage adjustments, leading to suboptimal therapeutic outcomes. Delays in diagnosis, imprecise tracking of disease progression, and a lack of personalized treatment options continue to hinder effective management.
Amid these enduring challenges, artificial intelligence (AI) is emerging as a transformative force in modern healthcare. With the capability to process vast quantities of data, identify hidden patterns, and adapt to complex variables, AI offers new tools for enhancing diagnostic precision, optimizing treatment protocols, and improving quality of life for patients with Parkinson’s disease. Unlike conventional analytical methods, AI systems can integrate diverse data sources—ranging from medical imaging and electronic health records to wearable device outputs and genetic profiles—enabling a holistic and data-driven approach to care.
In the context of Parkinson’s, AI is showing promising potential in several critical areas. For instance, machine learning algorithms are being employed to detect the disease in its earliest stages, sometimes years before clinical symptoms become apparent. Wearable technologies powered by AI are facilitating continuous, real-time monitoring of motor and non-motor symptoms, enabling clinicians to make data-informed decisions. AI is also proving valuable in the realm of precision medicine, helping tailor treatments to individual patient needs by analyzing variables such as age, disease stage, medication response, and comorbidities. Moreover, artificial intelligence is playing a pivotal role in accelerating Parkinson’s research through its applications in biomarker discovery, drug repurposing, and the design of predictive models for disease progression.
The integration of AI into Parkinson’s care does not merely represent a technological upgrade; it signifies a shift toward a more proactive, personalized, and preventive model of healthcare. This evolution aligns with the broader movement in medicine toward patient-centered care, wherein technology serves to augment, rather than replace, the expertise of clinicians. AI’s potential lies not only in computational power but in its ability to translate insights into actionable strategies that improve patient outcomes.
This blog explores the multifaceted role of artificial intelligence in improving Parkinson’s care, beginning with AI’s application in early detection and diagnosis, followed by its use in personalized treatment and medication management. It then delves into how AI-powered technologies are enhancing daily living and mobility for Parkinson’s patients, and concludes by examining the role of AI in research, clinical trials, and disease modeling. Through an evidence-based lens, this article aims to elucidate how the thoughtful and ethical deployment of AI technologies could fundamentally transform the Parkinson’s care landscape—offering hope where uncertainty has long prevailed.
Early Detection and Diagnosis with AI
Early and accurate diagnosis of Parkinson’s disease (PD) remains one of the most formidable challenges in clinical neurology. The disease often progresses insidiously, with motor symptoms appearing only after substantial neurodegeneration—typically the loss of more than 50% of dopaminergic neurons in the substantia nigra. Non-motor symptoms, which can manifest years before motor onset, are often subtle, varied, and nonspecific. Consequently, many patients receive a diagnosis only in the moderate stages of the disease, by which point significant functional impairment has already occurred. In this context, artificial intelligence (AI) emerges as a highly promising tool for addressing diagnostic delays and improving the accuracy and efficiency of early detection.
AI-Enabled Pattern Recognition in Parkinson’s Biomarkers
Traditional diagnostic approaches rely heavily on clinical observation and neurological assessments, often supported by imaging studies such as dopamine transporter (DaT) scans. However, these tools are subject to human interpretation and may not be effective in identifying prodromal or early-stage Parkinson’s. AI models, particularly those based on machine learning (ML) and deep learning (DL), offer a fundamentally different approach. By analyzing large datasets and recognizing complex patterns in multimodal data, AI can detect biomarkers and symptom clusters that might otherwise go unnoticed.
One of the most promising applications involves the analysis of voice recordings. Studies have shown that individuals with Parkinson’s often exhibit subtle changes in speech—such as reduced pitch variation, breathiness, and articulation difficulties—years before other symptoms become apparent. AI models trained on voice samples can achieve high classification accuracy by detecting these acoustic anomalies. Similarly, handwriting analysis has proven useful; patients with PD may develop micrographia, characterized by progressively smaller and cramped writing. Machine learning algorithms can quantify these changes using features such as stroke pressure, writing speed, and letter spacing.
Gait and Movement Analysis via Wearable Technologies
Wearable devices equipped with accelerometers, gyroscopes, and inertial sensors have introduced a new frontier in AI-assisted Parkinson’s diagnostics. These devices enable the continuous capture of movement data in real-world settings, far beyond the snapshot provided during clinical visits. When analyzed by AI algorithms, gait parameters—such as stride length, swing time, and postural sway—can serve as early indicators of Parkinsonian motor impairment.
Machine learning models, particularly supervised learning algorithms like support vector machines (SVM) and random forests, have been employed to classify movement patterns as normal or Parkinsonian with impressive accuracy. These models are capable of detecting variations so minute that they would be imperceptible to the human eye, enabling earlier interventions. Moreover, unsupervised learning approaches have been used to discover latent clusters in patient movement data, revealing novel subtypes of motor dysfunction that could inform more precise clinical management.
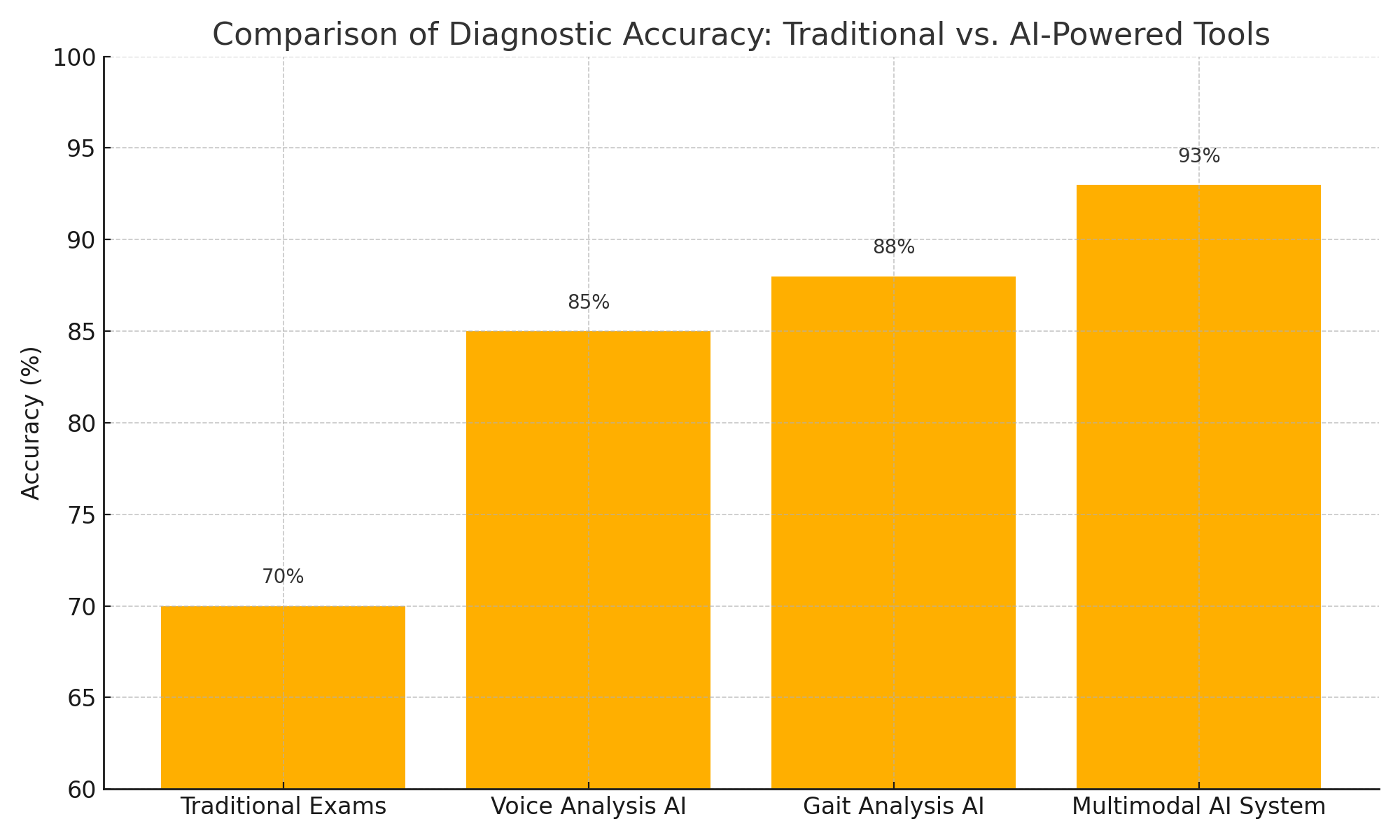
AI-powered tools such as voice analysis, gait recognition, and multimodal systems demonstrate significantly higher diagnostic accuracy for Parkinson’s disease compared to traditional neurological exams.
The advent of deep learning has further enhanced these capabilities. Convolutional neural networks (CNNs) and recurrent neural networks (RNNs) can process high-dimensional time-series data from wearables, capturing temporal dependencies and contextual nuances. This approach not only improves diagnostic accuracy but also facilitates real-time monitoring and dynamic risk assessment, which are critical in a progressive disorder like Parkinson’s.
Facial Recognition and Computer Vision in Symptom Detection
Another emerging avenue is the use of computer vision techniques to analyze facial expressions. Parkinson’s disease is often associated with facial masking, a reduction in spontaneous facial movement that can precede motor symptoms. Using video recordings, AI models can assess facial muscle rigidity, blinking frequency, and expression variability to detect early signs of PD. These tools can operate unobtrusively, potentially integrating into telehealth platforms for remote assessment.
Furthermore, computer vision has been applied to tremor analysis. By capturing video footage of a patient's hands or limbs, AI can quantify tremor frequency, amplitude, and periodicity. This data can then be used to distinguish between Parkinsonian tremors and those caused by other conditions such as essential tremor, thereby improving differential diagnosis.
Integrating Multimodal Data for Holistic Assessment
The true power of AI in early Parkinson’s diagnosis lies in its capacity to integrate and synthesize data from diverse modalities—voice, gait, handwriting, facial expressions, and genetic profiles—into a unified diagnostic framework. Multimodal machine learning models can cross-reference findings from different domains, enhancing diagnostic reliability. For instance, a patient’s subtle vocal anomalies, when combined with minor gait deviations and facial masking, may collectively indicate a high probability of prodromal PD.
Additionally, AI systems can incorporate data from electronic health records (EHRs) to evaluate comorbidities, medication history, and patient-reported outcomes. Natural language processing (NLP) techniques are used to extract relevant clinical insights from unstructured medical notes, further enriching the diagnostic dataset. By evaluating this complex interplay of factors, AI can generate individualized risk scores and recommend follow-up diagnostics or specialist referrals, enabling earlier and more targeted care.
Case Studies and Pilot Programs
Several real-world initiatives have demonstrated the effectiveness of AI in early Parkinson’s diagnosis. For instance, the mPower study conducted by Sage Bionetworks and the Michael J. Fox Foundation collected smartphone sensor data from thousands of participants performing daily tasks. AI models trained on this data were able to differentiate between PD patients and healthy controls with high sensitivity. Similarly, projects like Parkinson’s Voice Initiative and IBM’s Watson Health have explored the diagnostic potential of voice and sensor data, respectively, yielding encouraging results.
A recent pilot program in the United Kingdom integrated AI algorithms with general practitioner EHRs to flag patients at risk of Parkinson’s based on subtle patterns in visit frequency, symptom complaints, and prescription history. The system achieved significant success in identifying high-risk individuals who were subsequently referred for specialist evaluation, underscoring the value of AI-assisted screening at the primary care level.
Limitations and Ethical Considerations
Despite these advancements, several limitations must be acknowledged. Data quality and representativeness remain critical concerns. Many AI models are trained on datasets that may not reflect the full demographic and clinical diversity of the global Parkinson’s population, raising issues of bias and generalizability. Furthermore, over-reliance on AI could risk marginalizing human clinical judgment, especially in ambiguous cases.
Privacy and consent also merit careful attention. The use of continuous monitoring tools and biometric data collection necessitates robust data governance frameworks to ensure patient autonomy and data security. Ethical AI deployment in healthcare must be guided by principles of transparency, accountability, and equitable access.
Conclusion
Artificial intelligence holds transformative potential in the early detection and diagnosis of Parkinson’s disease. By leveraging advanced algorithms, wearable technologies, and multimodal data integration, AI can identify subtle, preclinical indicators of PD that elude conventional diagnostics. This enables proactive intervention, personalized treatment planning, and ultimately, a higher quality of life for patients. While challenges remain, the convergence of AI and neurology is paving the way for a new era of predictive, data-driven, and patient-centric Parkinson’s care.
Personalized Treatment and Medication Management
Effective management of Parkinson’s disease (PD) hinges not only on accurate diagnosis but also on the continuous customization of treatment regimens in response to the patient's evolving needs. Parkinson’s manifests in highly individualized ways; symptom progression, medication response, and comorbidity profiles vary significantly from person to person. Consequently, a uniform treatment approach often fails to yield optimal outcomes. In this context, artificial intelligence (AI) has emerged as a pivotal enabler of personalized medicine, offering clinicians powerful tools to tailor treatment strategies, predict therapeutic efficacy, and dynamically adjust regimens based on real-time data.
Challenges in Traditional Treatment Approaches
The standard pharmacological treatment for PD is the administration of dopaminergic agents, particularly levodopa. While initially effective, long-term use often leads to complications such as motor fluctuations and dyskinesia. Moreover, achieving the correct dosage is an intricate task; underdosing can lead to symptom undercontrol, while overdosing may cause debilitating side effects. These challenges are exacerbated by the disease’s fluctuating nature, which means that patients may require different dosages at different times of the day or stages of disease progression.
Traditionally, clinicians rely on intermittent patient visits, subjective symptom reporting, and trial-and-error methodologies to manage medication schedules. This reactive framework often results in delayed response to symptom changes and fails to account for the nuanced daily variations in patient condition. AI, with its capacity for data integration, pattern recognition, and predictive modeling, offers a paradigm shift toward proactive and precision-driven care.
AI-Driven Optimization of Medication Schedules
One of the most promising applications of AI in Parkinson’s treatment is the optimization of medication timing and dosage. By analyzing historical patient data, wearable sensor outputs, and electronic health records (EHRs), AI models can generate individualized dosing schedules that align with each patient's symptom patterns and physiological rhythms. Reinforcement learning algorithms, in particular, have shown success in this domain. These models learn by simulating different treatment strategies and receiving feedback in the form of symptom relief or side effects, ultimately identifying the regimen that maximizes therapeutic benefit.
An example is the use of deep reinforcement learning to manage “on-off” motor fluctuations. By modeling the patient’s symptom-response cycle, AI can forecast when the effects of medication will wane and preemptively adjust dosages or suggest behavioral interventions, such as rest or exercise. Such real-time adaptability enhances patient comfort and minimizes periods of immobility or dyskinesia, leading to a marked improvement in quality of life.
Integration with Wearable Devices for Continuous Feedback
Wearable technologies further amplify the impact of AI in personalized treatment. Devices equipped with accelerometers, gyroscopes, and biosensors can continuously monitor motor function, tremor intensity, and even autonomic indicators like heart rate and skin conductance. When coupled with AI algorithms, this data provides a granular view of patient status throughout the day, enabling dynamic treatment adjustments.
For instance, if a wearable device detects a rise in tremor amplitude or gait instability, the AI system can cross-reference the medication schedule to determine if a dosage adjustment is warranted. This continuous loop of monitoring and response moves PD care away from static prescriptions and toward real-time therapeutic modulation. Additionally, AI-powered platforms can send alerts to both patients and healthcare providers, promoting adherence to medication plans and facilitating timely interventions.
Predictive Modeling of Treatment Response
Another critical capability of AI in this domain is its ability to predict how a patient will respond to a given treatment plan. By analyzing large datasets encompassing demographic information, genetic markers, previous medication outcomes, and clinical biomarkers, machine learning models can identify patterns that correlate with positive or negative treatment responses. This enables clinicians to make data-driven decisions when selecting pharmacological agents or adjusting dosages, reducing the reliance on empirical guesswork.
Furthermore, AI can support drug repurposing initiatives by identifying existing medications that may be effective in treating specific subtypes of PD. For example, unsupervised clustering algorithms have been used to segment patients into phenotypic subgroups based on symptom presentation and progression rate. These insights can guide the selection of adjunctive therapies, such as MAO-B inhibitors, COMT inhibitors, or non-dopaminergic agents, for tailored combination treatment plans.
Personalized Non-Pharmacological Interventions
AI is not limited to pharmacotherapy management; it also plays a significant role in personalizing non-drug interventions. Physical therapy, cognitive exercises, and dietary adjustments are all integral to comprehensive PD care. AI systems can evaluate patient data to recommend individualized exercise regimens aimed at improving balance, flexibility, and strength. For example, AI-guided virtual coaching platforms have been developed to deliver real-time feedback during physiotherapy sessions, ensuring that patients perform movements correctly and safely.
Similarly, natural language processing (NLP) algorithms can analyze speech patterns to identify emerging dysarthria and suggest targeted speech therapy. AI can even monitor sleep patterns and mood fluctuations using data from smart devices, enabling the recommendation of behavioral therapies or adjustments to nighttime medication to improve rest and psychological well-being.
Clinical Decision Support Systems
AI-driven clinical decision support systems (CDSS) are becoming an invaluable resource for neurologists treating Parkinson’s patients. These platforms synthesize multimodal data and generate actionable insights, such as suggesting dosage changes, flagging abnormal symptom patterns, or proposing new interventions based on real-time analytics. Importantly, these systems function as augmentative tools, empowering clinicians rather than replacing their expertise.
For example, an AI-enabled CDSS might alert a physician that a patient’s walking speed has declined by 15% over the past week, coinciding with a reduced response to morning levodopa doses. This insight could prompt the clinician to consider modifying the medication regimen or ordering additional tests. By offering such real-time, evidence-backed recommendations, AI contributes to more responsive and individualized care.
Below is a comparison that outlines the fundamental differences between AI-driven and traditional approaches to Parkinson’s treatment planning:
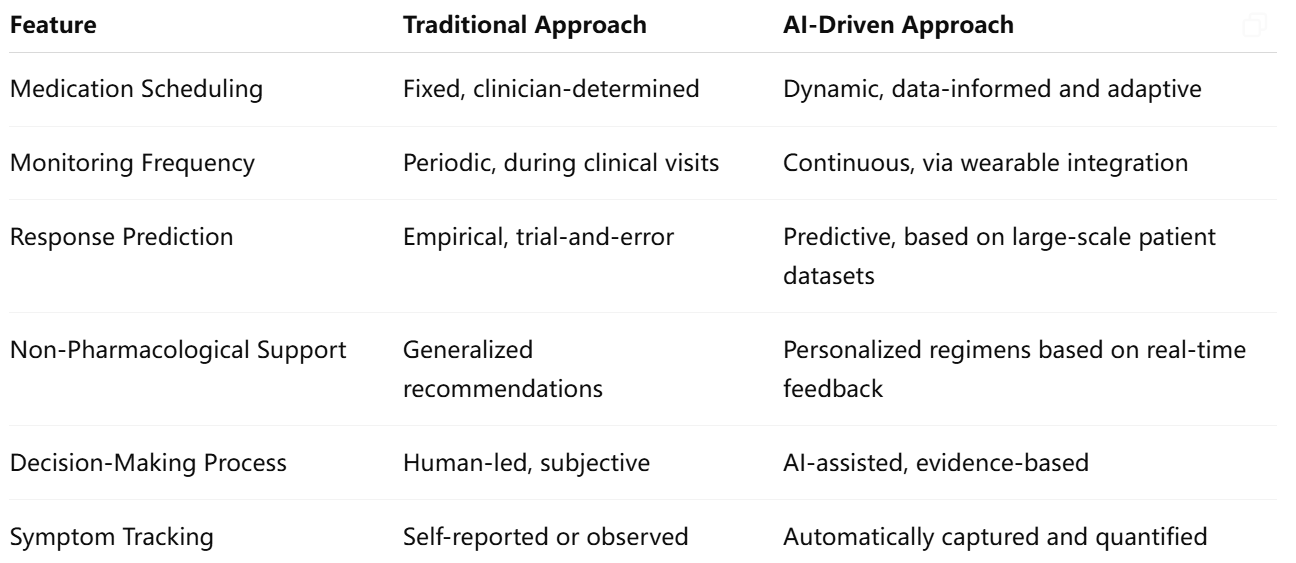
This table underscores the significant advantages of AI in enhancing the granularity, responsiveness, and precision of Parkinson’s care.
Barriers and Considerations
While the benefits of AI in personalized PD management are evident, several barriers must be addressed to ensure successful implementation. Interoperability between devices, data privacy regulations, and the integration of AI tools into existing clinical workflows require thoughtful planning. Additionally, disparities in access to wearable technology and high-speed internet could widen the treatment gap between different socioeconomic groups.
Moreover, algorithmic transparency is essential. Clinicians must understand the rationale behind AI-generated recommendations to maintain trust in the system. Collaborative development between data scientists, neurologists, and patient advocacy groups is crucial to ensure these tools are safe, inclusive, and clinically meaningful.
Conclusion
AI is redefining the landscape of Parkinson’s treatment by enabling a shift from reactive to proactive and personalized care. Through intelligent medication scheduling, real-time monitoring, predictive analytics, and tailored therapeutic interventions, AI empowers clinicians and enhances patient outcomes. While challenges related to implementation and equity remain, the trajectory of innovation suggests that AI will be a cornerstone of next-generation Parkinson’s disease management—delivering more effective, compassionate, and individualized care.
Enhancing Mobility and Daily Living with Smart Systems
Parkinson’s disease (PD) progressively impairs a patient's ability to perform everyday tasks, significantly diminishing their independence and quality of life. While pharmacological interventions offer symptomatic relief, they do not fully address the daily challenges that patients encounter—such as difficulty with walking, tremors that affect dexterity, or speech issues that hinder communication. In this context, artificial intelligence (AI)-enabled smart systems are increasingly being deployed to complement medical treatment by improving functional mobility, ensuring safety at home, and enabling more autonomous living. These innovations represent a critical evolution in care delivery, extending therapeutic support beyond clinical settings into the lived environment of Parkinson’s patients.
The Role of AI in Assistive Technologies
AI-powered assistive devices are designed to compensate for motor deficits, guide movement, and reduce the risk of accidents. These devices often leverage real-time data from embedded sensors to make adaptive decisions tailored to the user’s immediate needs. Among the most common are smart canes and walkers, which use AI algorithms to monitor gait parameters and provide haptic or visual feedback to correct posture or alert users when their gait becomes unstable.
For example, some AI-integrated smart walkers are equipped with obstacle detection and pathfinding capabilities. Using computer vision and sensor fusion techniques, these walkers can autonomously navigate around furniture or uneven surfaces, reducing fall risks. In addition, they can track stride length, walking speed, and freezing of gait episodes, enabling clinicians to fine-tune treatments or recommend physical therapy exercises based on objective data.
AI-enhanced exoskeletons, though still in developmental stages for Parkinson’s, also offer promise. These wearable robotic systems use predictive algorithms to support the lower limbs, making it easier for patients to initiate movement and maintain balance. By adapting to a user's walking patterns and fatigue levels, they can deliver the right amount of assistance at the right time, promoting mobility without inducing dependence.
Smart Home Integration for Parkinson’s Patients
Beyond mobility aids, AI is increasingly being integrated into home automation systems to support daily routines and reduce the cognitive burden associated with Parkinson’s. Smart home platforms can be programmed to respond to voice commands, automate repetitive tasks, and monitor environmental factors such as lighting and temperature to create a safe and comfortable living space.
For instance, AI-driven voice assistants (like Amazon Alexa or Google Assistant) can be tailored to remind patients to take medications, schedule appointments, or perform exercises. These systems can be integrated with smart pill dispensers that alert caregivers if a dose is missed, improving adherence to treatment plans.
Fall detection is another critical application. AI-powered home monitoring systems use computer vision and motion sensors to identify sudden movements or abnormal patterns indicative of a fall. Unlike traditional emergency alert systems that rely on manual activation, these systems can autonomously detect incidents and notify caregivers or emergency services. Some platforms also utilize anomaly detection algorithms to identify gradual declines in physical activity or behavioral changes, signaling early signs of health deterioration.
Enhancing Dexterity and Fine Motor Skills
Patients with PD often experience tremors and bradykinesia that affect fine motor control, making tasks like buttoning a shirt, writing, or eating increasingly difficult. AI-powered wearable devices are being developed to mitigate these challenges. Tremor-suppression gloves, for example, use sensors to detect involuntary movements and apply counteracting forces through actuators in real time. These gloves are trained using machine learning algorithms to distinguish between intentional and unintentional movements, enabling smoother execution of daily tasks.
In addition, AI-enabled utensils and eating aids can adjust to a user’s tremor patterns by stabilizing the handle using gyroscopic technology. These tools have shown promise in improving nutritional intake and reducing frustration during meals—an area of growing concern as PD progresses.
For digital interaction, voice-to-text and gesture-recognition systems driven by natural language processing (NLP) and AI vision models allow users with limited hand control to navigate computers, smartphones, and smart TVs with ease. These technologies not only enhance communication but also facilitate access to virtual consultations, social media, and online communities, reducing isolation and enhancing psychological well-being.
Cognitive Assistance and Daily Scheduling
Cognitive decline is a frequent non-motor symptom of Parkinson’s, manifesting as memory lapses, decreased attention span, and impaired executive function. AI systems can provide cognitive support through scheduling tools, reminders, and interactive applications that engage the brain in stimulating activities.
For example, AI-based mobile apps can offer personalized mental exercises, adapting in difficulty based on the user's performance. These apps use reinforcement learning to refine cognitive tasks and promote neuroplasticity. Additionally, calendar management tools with intelligent prompts can help patients plan and recall daily activities, attend appointments, and maintain social connections.
Some AI-driven platforms also feature conversational agents that simulate human dialogue. These virtual companions provide both cognitive stimulation and emotional support by engaging users in meaningful conversations, storytelling, or games. Research indicates that such systems can help slow cognitive decline and improve mood in neurodegenerative disorders, including PD.
AI for Caregiver Support and Remote Monitoring
Caregivers play a vital role in Parkinson’s management, yet they often face burnout due to the demands of constant supervision. AI-powered monitoring tools can relieve this burden by providing caregivers with real-time updates and risk alerts through mobile applications. For instance, AI can track deviations in movement patterns or nighttime activity and flag potential issues before they escalate.
Some platforms use predictive analytics to estimate care needs and recommend interventions. For example, if the system detects increased nighttime agitation, it might suggest altering evening medication or introducing a calming routine. AI can also facilitate communication between multiple caregivers, ensuring continuity of care and coordination among family members and healthcare providers.
These smart systems not only extend the reach of clinical oversight but also empower caregivers to make informed decisions, ultimately improving outcomes for both patients and support networks.
To better illustrate the benefits, the following chart (Chart 2) presents the outcomes associated with various AI-assisted interventions based on recent pilot studies and research trials. These include improvements in mobility, reduction in fall incidents, adherence to treatment plans, and patient satisfaction levels.
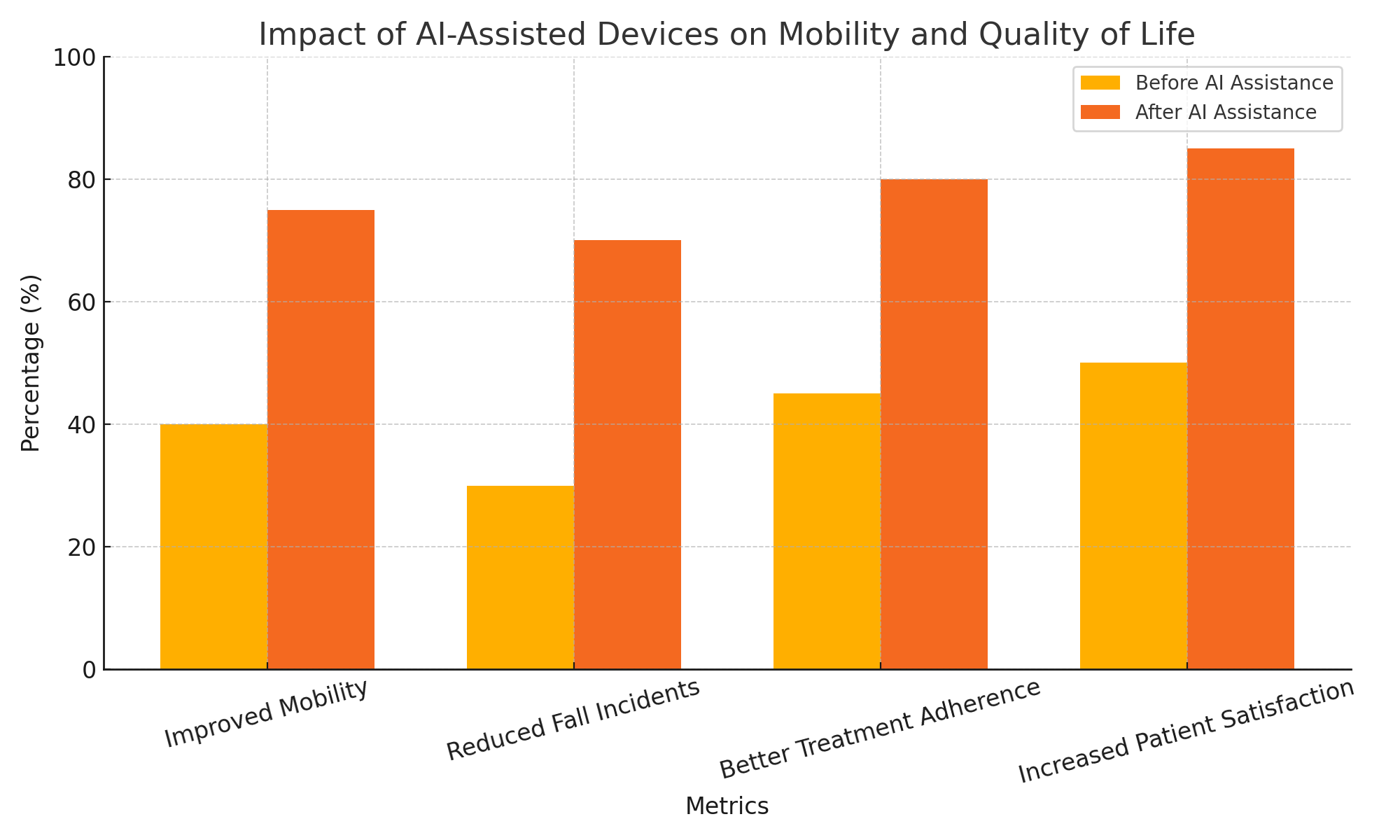
Conclusion
The integration of AI into assistive technologies and smart systems is transforming the way Parkinson’s patients navigate daily life. From intelligent mobility aids and home automation to tremor-dampening devices and cognitive assistants, these innovations are not just augmenting physical function—they are restoring autonomy, dignity, and safety. As these technologies mature and become more accessible, they promise to redefine the standard of supportive care in Parkinson’s, extending therapeutic impact well beyond the clinic. Moving forward, the convergence of AI, robotics, and personalized user experience design will be central to empowering individuals with Parkinson’s to live more independently and with greater confidence.
AI in Clinical Trials, Research, and Disease Modeling
The pursuit of more effective therapies and a deeper understanding of Parkinson’s disease (PD) remains an urgent global priority. Traditional research paradigms, while rigorous, often encounter limitations in scale, speed, and predictive capability. Clinical trials for PD are complex, expensive, and frequently hampered by recruitment inefficiencies, patient heterogeneity, and the slow progression of the disease. Artificial intelligence (AI) offers transformative potential to accelerate this landscape—enhancing clinical trial design, improving patient stratification, and enabling sophisticated disease modeling that was previously unattainable. Through its integration into research workflows, AI is not only optimizing existing methods but also unlocking novel pathways in the study and treatment of Parkinson’s.
Accelerating Drug Discovery and Repurposing
One of the most promising applications of AI in PD research is the acceleration of drug discovery. Traditionally, the development of new therapeutics spans over a decade and involves substantial financial investment. AI-driven platforms are capable of dramatically shortening this timeline by identifying candidate molecules, predicting pharmacological interactions, and simulating biological responses.
Deep learning models can analyze vast biochemical datasets to predict how compounds will interact with target proteins implicated in Parkinson’s pathology, such as alpha-synuclein and LRRK2. These models use pattern recognition to evaluate compound binding affinity and selectivity, allowing researchers to prioritize the most promising candidates for laboratory testing. Moreover, AI techniques like generative adversarial networks (GANs) are being employed to design entirely new molecules that meet predefined pharmacodynamic profiles.
In parallel, AI is facilitating drug repurposing—identifying existing FDA-approved medications that could be used to treat PD. By mining electronic health records, biomedical literature, and genomic databases, AI models can uncover associations between non-PD drugs and Parkinsonian symptom relief or disease modification. Such insights not only save development costs but also fast-track regulatory approval since the safety profiles of repurposed drugs are already well-established.
Enhancing Patient Recruitment and Stratification in Clinical Trials
Recruitment is a critical bottleneck in clinical research, especially in diseases like Parkinson’s that present with considerable heterogeneity. AI tools can enhance recruitment by analyzing electronic medical records and social determinants of health to identify suitable candidates with a high degree of specificity. Natural language processing (NLP) algorithms extract relevant clinical features from unstructured physician notes, while machine learning classifiers can score patients based on trial inclusion criteria, thus streamlining participant selection.
Furthermore, AI supports patient stratification by clustering individuals into biologically meaningful subgroups based on multi-omic data (genomic, proteomic, metabolomic), imaging biomarkers, and longitudinal clinical profiles. Such precision stratification enables targeted interventions and reduces variability in treatment response, ultimately increasing the likelihood of statistically significant outcomes. Stratification is particularly vital in Parkinson’s trials, where disease progression and symptom expression vary widely among patients.
Some trials have begun to incorporate AI to adaptively modify trial parameters in response to emerging data—a concept known as adaptive trial design. AI algorithms analyze interim results to recalibrate dosing arms, reassign participants, or discontinue ineffective treatment groups, optimizing both trial efficacy and ethical standards.
Digital Twins and Predictive Disease Modeling
Beyond trial logistics, AI is at the forefront of predictive disease modeling through the development of digital twins—virtual representations of patients that simulate disease progression and treatment outcomes. These models ingest real-world data from wearables, imaging, lab results, and clinical notes to create a dynamic and individualized forecast of the patient’s condition over time.
In Parkinson’s care, digital twins can predict symptom trajectory, medication response, and risk of complications such as dyskinesia or falls. Clinicians can test different therapeutic strategies within the virtual model, enabling personalized treatment planning without exposing the patient to unnecessary risk. For researchers, digital twins offer a scalable method to test interventions across thousands of simulated scenarios, providing insights that would otherwise require years of longitudinal studies.
AI-enhanced predictive modeling also extends to population-level forecasts. Epidemiological models powered by AI can predict trends in Parkinson’s incidence and prevalence, aiding in resource allocation and public health planning. These models account for demographic shifts, environmental factors, and genetic predispositions, allowing governments and healthcare organizations to prepare for future burdens of the disease.
AI in Biomarker Discovery
Biomarker discovery is central to understanding Parkinson’s pathology and developing disease-modifying therapies. However, identifying reliable and specific biomarkers has proven difficult due to the complexity of PD’s etiology. AI offers powerful capabilities for high-dimensional data analysis, uncovering subtle patterns that may serve as early indicators of disease or therapeutic efficacy.
For example, machine learning algorithms have been applied to genomic and transcriptomic datasets to identify gene expression signatures associated with PD subtypes. AI has also been used to analyze cerebrospinal fluid composition, imaging markers from PET and MRI scans, and even skin biopsies to detect misfolded alpha-synuclein proteins.
In addition, AI-based voice and movement analytics are being evaluated as potential digital biomarkers. Continuous data from smartphones and wearable devices provide a non-invasive and scalable source of information. AI algorithms trained on this data can track disease severity and detect fluctuations in real-time, providing feedback that can inform both research and clinical practice.
Case Studies and Ongoing Initiatives
Several large-scale initiatives illustrate the growing role of AI in Parkinson’s research. The Accelerating Medicines Partnership (AMP) for Parkinson’s Disease, a collaboration among NIH, FDA, industry, and non-profit organizations, is leveraging AI to identify new biomarkers and therapeutic targets by analyzing massive datasets from clinical cohorts.
Another notable example is the Parkinson’s Progression Markers Initiative (PPMI), which uses AI to process imaging, biosample, and clinical data from thousands of participants worldwide. These AI models aim to characterize disease heterogeneity and predict progression more accurately than traditional statistical approaches.
Startups and academic institutions are also developing proprietary AI platforms for PD research. Companies like BenevolentAI and Insilico Medicine are using AI to map the molecular pathways of Parkinson’s and discover novel treatment opportunities. Meanwhile, academic research centers are integrating AI into translational studies, validating models with clinical data, and contributing to the broader scientific literature on AI applications in neurology.
Ethical Considerations and Future Directions
As AI becomes more deeply embedded in clinical research, ethical considerations must remain at the forefront. Data privacy, informed consent, and algorithmic transparency are essential to maintaining public trust and research integrity. Furthermore, efforts must be made to ensure that AI models are trained on diverse datasets to avoid biases that could affect patient outcomes or generalizability of findings.
Looking ahead, the convergence of AI with other emerging technologies—such as quantum computing, CRISPR gene editing, and nanotechnology—may further enhance the power and scope of Parkinson’s research. Interdisciplinary collaborations among data scientists, neurologists, pharmacologists, and patient advocates will be critical in driving these innovations forward.
Conclusion
AI is revolutionizing the research and clinical trial ecosystem for Parkinson’s disease. From accelerating drug discovery and refining trial design to enabling digital twins and biomarker discovery, AI provides unprecedented capabilities that are reshaping the pace and precision of scientific inquiry. As these tools continue to evolve, they promise to deliver more personalized, efficient, and effective solutions for the millions affected by Parkinson’s disease worldwide.
Conclusion and Future Outlook
Parkinson’s disease continues to pose immense challenges for patients, caregivers, and healthcare systems worldwide. Its progressive nature, symptom heterogeneity, and lack of curative treatment necessitate a care model that is not only responsive but also anticipatory and personalized. Artificial intelligence has emerged as a powerful ally in addressing these complexities—redefining diagnostic precision, treatment personalization, daily living support, and clinical research paradigms.
From early detection via voice, gait, and facial analysis, to personalized dosing supported by reinforcement learning and real-time symptom monitoring, AI is transforming the way clinicians manage Parkinson’s. The integration of AI into smart devices and home systems extends this care model into everyday environments, offering patients increased safety, autonomy, and quality of life. Simultaneously, AI is streamlining the research pipeline—from accelerating drug discovery to enabling predictive modeling through digital twins and biomarker analytics.
Yet, the path to widespread adoption is not without hurdles. Data privacy, interoperability, ethical governance, and equitable access remain critical issues that must be addressed. Clinicians must be supported in learning to interpret and trust AI-generated insights, while researchers must continue validating these systems across diverse populations to ensure fairness and generalizability.
The future of Parkinson’s care is undeniably intertwined with technological progress. As AI capabilities mature and become more integrated across healthcare domains, the standard of care will shift from episodic interventions to continuous, predictive, and patient-centric support. With robust interdisciplinary collaboration and ethical deployment, artificial intelligence has the potential not just to improve Parkinson’s care—but to redefine it entirely.
References
- Parkinson’s Foundation – What is Parkinson’s?
https://www.parkinson.org/Understanding-Parkinsons - Michael J. Fox Foundation – AI in Parkinson’s Research
https://www.michaeljfox.org/news/using-artificial-intelligence-advance-parkinsons-research - NIH – Accelerating Medicines Partnership for Parkinson’s Disease
https://www.ninds.nih.gov/current-research/accelerating-medicines-partnership-parkinsons-disease - IEEE Spectrum – AI Helps Spot Parkinson’s Earlier
https://spectrum.ieee.org/artificial-intelligence-diagnosis-parkinsons - Nature – Wearables and AI in Neurological Disorders
https://www.nature.com/articles/d41586-technology-neuro-wearables - IBM Research – AI for Parkinson’s Patient Monitoring
https://research.ibm.com/blog/ai-parkinsons - Lancet Digital Health – AI in Neurology
https://www.thelancet.com/journals/landig/article/ai-in-neurology-overview - Mayo Clinic – Parkinson’s Disease: Diagnosis and Treatment
https://www.mayoclinic.org/diseases-conditions/parkinsons-disease/diagnosis-treatment - Stanford Medicine – AI in Precision Neurology
https://med.stanford.edu/news/all-news/ai-neurodegenerative-disease-tools - Harvard Health – AI and the Future of Medicine
https://www.health.harvard.edu/technology/ai-and-the-future-of-medicine