How AI is Slashing Drug Discovery and Development Costs: A New Era in Pharmaceutical Innovation
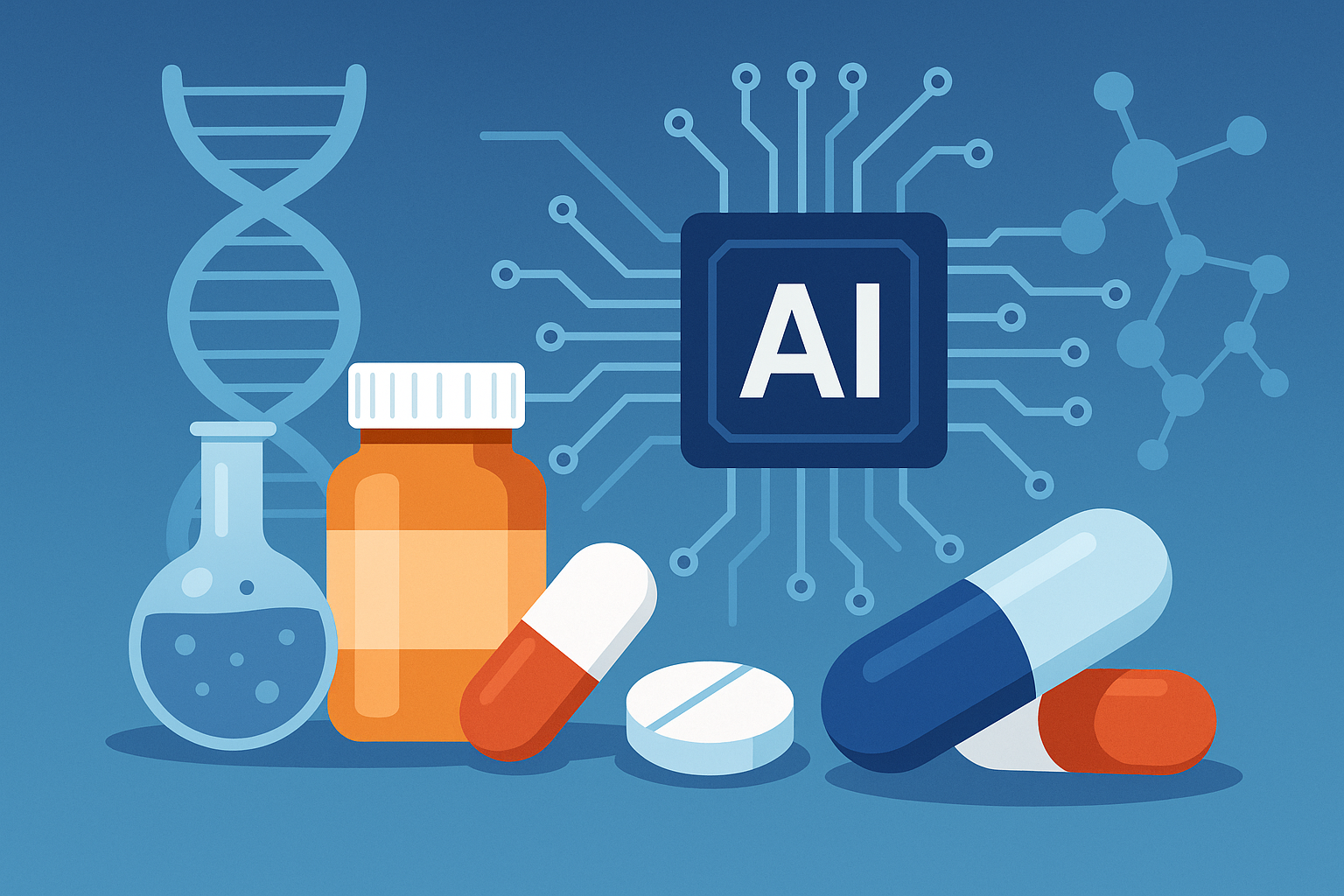
The pharmaceutical industry stands at a critical juncture, grappling with the dual challenges of escalating research and development (R&D) costs and the increasingly complex landscape of drug discovery. Traditionally, the path from initial compound identification to a market-ready therapeutic spans over a decade and can cost upwards of $2.6 billion per approved drug. This prolonged timeline and financial burden are exacerbated by high failure rates, regulatory complexities, and the unpredictability of clinical trials. The COVID-19 pandemic further exposed the vulnerabilities in global drug development pipelines, heightening the urgency for more agile, efficient, and cost-effective approaches.
In response to these mounting pressures, artificial intelligence (AI) has emerged as a transformative force in pharmaceutical R&D. By leveraging machine learning, natural language processing, and deep neural networks, AI technologies are reshaping the landscape of drug development. These innovations are not only accelerating the identification of viable drug candidates but also optimizing clinical trials, predicting molecular behavior, and streamlining regulatory processes. The promise of AI is clear: to dramatically reduce both the time and cost associated with bringing new drugs to market, while increasing the probability of success.
AI-driven drug discovery is no longer a speculative endeavor—it is a fast-growing sector backed by substantial investment, strategic partnerships, and early-stage clinical successes. From startups employing AI to discover novel compounds to multinational pharmaceutical companies integrating intelligent algorithms into their R&D ecosystems, the adoption curve is steepening. As the technology matures, AI is expected to deliver not just incremental efficiency gains but also foundational shifts in how therapeutics are conceived, validated, and approved.
This blog explores the multifaceted impact of AI on drug discovery and development costs. It begins by dissecting the current economic realities of pharmaceutical innovation and identifying the principal cost drivers. Subsequent sections delve into the technical applications of AI in preclinical research, clinical trials, and regulatory submissions. A data-driven analysis of AI’s financial impact is also presented, alongside a critical look at the challenges and ethical considerations that must be addressed to ensure sustainable adoption.
By examining the intersection of cutting-edge AI capabilities and the pressing needs of the pharmaceutical sector, this blog aims to offer a comprehensive view of how the industry is being reshaped. In doing so, it highlights the potential of AI to not only reduce costs but also accelerate the delivery of life-saving treatments to patients worldwide.
The Economics of Drug Development – A Crisis of Cost and Time
Drug discovery and development is a process mired in scientific uncertainty, regulatory complexity, and economic risk. Despite advances in biotechnology and data-driven modeling, the pharmaceutical industry continues to face daunting financial and temporal burdens associated with bringing new therapies to market. On average, it takes over a decade and an estimated $1.5 to $2.6 billion to develop a single FDA-approved drug. This investment accounts for a variety of operational phases—from early target discovery and preclinical validation to large-scale clinical trials and regulatory submission. Each stage introduces distinct challenges that compound costs, delay timelines, and contribute to the systemic inefficiencies that have plagued the sector for decades.
Rising Costs and Declining R&D Productivity
The traditional model of drug development is characterized by high attrition rates. According to industry data, only about 1 in 10 drug candidates that enter clinical trials ultimately secure regulatory approval. This low success rate is partially a consequence of the biological complexity of diseases, the unpredictability of patient responses, and the strict safety and efficacy standards imposed by regulatory agencies. However, the cost of failure is steep. Resources invested in compounds that are eventually discontinued are sunk costs that inflate the overall R&D expenditure.
The lion’s share of development costs is concentrated in clinical testing. Clinical trials—particularly Phase II and III—are resource-intensive endeavors requiring hundreds to thousands of participants, site monitoring, data collection, and long-term follow-ups. These trials often span several years and are prone to delays related to patient recruitment, protocol amendments, and regulatory review. Moreover, trials may need to be restarted or terminated early due to adverse events, lack of efficacy, or strategic realignments by sponsoring firms.
In addition to scientific and operational risks, economic factors have further complicated drug development. Inflation in healthcare services, stringent reimbursement environments, and competitive pressures demand that companies maximize their pipeline efficiency. Yet, despite increased investment in R&D—global pharmaceutical R&D expenditure exceeded $250 billion in recent years—productivity remains stagnant. This trend is often referred to as "Eroom’s Law," the inverse of Moore’s Law, which observes that drug discovery is becoming slower and more expensive over time.
Breakdown of Drug Development Phases and Cost Allocation
The process of bringing a drug from concept to market can be divided into five major stages: discovery, preclinical research, clinical trials (Phases I–III), regulatory approval, and post-marketing surveillance. Each stage bears unique scientific and financial considerations:
- Discovery Phase: This involves target identification, validation, and compound screening. Though costs are lower at this stage, inefficiencies here can lead to costly failures downstream.
- Preclinical Phase: Candidate molecules undergo in vitro and in vivo testing to assess toxicity, pharmacokinetics, and efficacy. Success here is necessary before transitioning to human trials.
- Clinical Trials: Spanning Phase I (safety and dosage), Phase II (efficacy and side effects), and Phase III (confirmation and comparison), this stage accounts for the highest share of total costs—often over 60%.
- Regulatory Approval: Submission of a New Drug Application (NDA) or Biologics License Application (BLA) involves preparing detailed data packages and responding to agency queries, often extending the time to market.
- Post-Marketing Surveillance: Even after approval, continued monitoring is essential for safety, which adds to lifecycle costs.
The figure below presents a visual representation of the average time and cost associated with each stage of drug development:
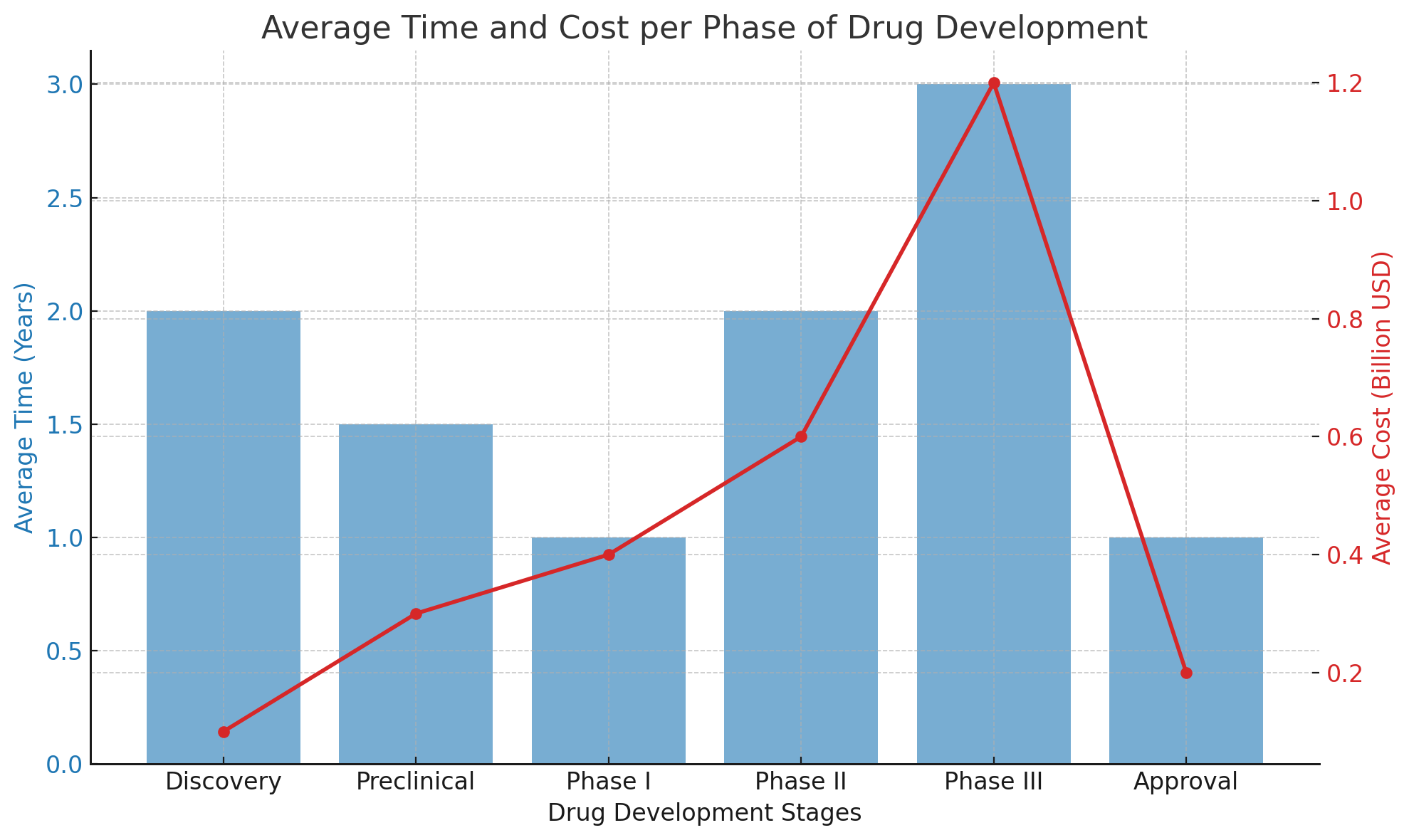
This chart illustrates how development costs escalate sharply during the clinical phase, peaking in Phase III, where large-scale, multicenter trials are conducted.
Market Failures and Opportunity Costs
One often overlooked aspect of drug development economics is the opportunity cost associated with pipeline delays. Each year spent in development is a year lost in potential revenue, especially for drugs targeting high-prevalence or high-burden diseases. Additionally, patent lifespans—typically 20 years from the date of filing—begin ticking down long before a product reaches commercialization. Thus, delays compress the window of market exclusivity, undermining return on investment (ROI) and jeopardizing a company’s competitive positioning.
Market failures also occur when promising drugs are shelved not due to scientific invalidation, but because of market saturation, misaligned strategic priorities, or unfavorable reimbursement forecasts. These sunk costs ripple through company balance sheets and can deter innovation in niche therapeutic areas with limited commercial upside, such as rare diseases or antimicrobial resistance.
Pharma vs. Biotech: Divergent Cost Structures
While large pharmaceutical corporations typically manage a diversified pipeline and have the capital to absorb failures, smaller biotech firms operate under more constrained financial models. For biotech startups, even a single failed trial can be existential. These firms often rely on venture capital, public funding, or strategic partnerships with larger entities to finance development. As a result, cost containment and time-to-market become critical KPIs for biotech innovation. This asymmetry in financial resilience makes the efficient allocation of R&D resources even more vital across the ecosystem.
The Innovation Gap and Policy Pressures
Governments and regulatory bodies are increasingly aware of the unsustainable trajectory of drug development costs. In response, various policy instruments have been introduced to streamline approval processes and incentivize innovation, such as the FDA’s Breakthrough Therapy Designation or the European Medicines Agency’s (EMA) PRIME scheme. While these efforts provide temporary relief, they do not fundamentally address the underlying inefficiencies in the discovery and development pipeline.
Moreover, public expectations for rapid cures, particularly in oncology, rare diseases, and pandemics, have intensified pressure on both regulators and companies. Balancing patient safety with the urgency of therapeutic access is a delicate act that further complicates the economic calculus of drug development.
The Case for Disruption
These challenges have created a compelling case for technological disruption. Traditional methods—anchored in labor-intensive research, iterative testing, and empirical trial design—are no longer tenable in the face of mounting fiscal pressure and competitive urgency. AI, with its potential to identify patterns, generate hypotheses, and simulate biological systems, offers a radical departure from the status quo. It promises to reduce false starts, enhance predictive accuracy, and compress development timelines, all of which can translate into measurable cost savings.
The pharmaceutical industry is thus at an inflection point. Embracing AI not only offers a way to curb costs but also to fundamentally rethink how therapies are discovered, evaluated, and delivered. The following sections will explore how AI is being deployed across the drug development continuum—from target discovery and preclinical testing to clinical trials and regulatory approval—and the measurable impact it is already having on efficiency and cost-effectiveness.
AI’s Role in Transforming Drug Discovery Workflows
Artificial intelligence (AI) is rapidly becoming a linchpin in the pharmaceutical industry’s quest to streamline drug discovery and reduce associated costs. By replacing or augmenting traditional empirical methodologies with data-driven computational models, AI enables the rapid analysis of complex biological systems, prediction of molecular interactions, and acceleration of candidate selection. The adoption of AI technologies in drug discovery marks a significant paradigm shift—one that emphasizes speed, accuracy, and resource efficiency across early-stage research and development (R&D) pipelines.
Target Identification and Validation
One of the foundational steps in drug discovery is identifying a viable biological target—typically a protein, gene, or receptor—whose modulation is expected to yield therapeutic benefits. Historically, this process has been labor-intensive and hypothesis-driven, relying heavily on academic literature, biochemical assays, and expert intuition. AI dramatically accelerates this process by mining vast biomedical databases, including genomics, proteomics, and transcriptomics datasets.
Machine learning algorithms are capable of detecting patterns in high-dimensional data that would otherwise elude human researchers. For example, supervised learning models can be trained to predict disease-gene associations using labeled datasets, while unsupervised techniques like clustering can reveal novel biological pathways or biomarker profiles. More recently, transformer-based models—akin to those used in natural language processing—have been repurposed to interpret biological sequences, enabling the functional annotation of uncharacterized genes and proteins.
In practical terms, AI-driven target identification not only shortens the discovery timeline but also improves the quality of targets selected for subsequent drug development. By reducing the incidence of target failure—a leading cause of late-stage clinical trial discontinuation—AI contributes to substantial cost avoidance.
Compound Screening and Molecular Docking
Once a biological target has been validated, the next step involves identifying chemical compounds capable of modulating the target’s activity. This traditionally involves high-throughput screening (HTS) of massive chemical libraries, often numbering in the millions. HTS is costly, time-consuming, and prone to generating false positives that necessitate further experimental validation.
AI-enabled virtual screening offers a more efficient alternative. Deep learning models, particularly convolutional neural networks (CNNs) and graph neural networks (GNNs), can model molecular structures and predict their binding affinities to target sites. These models are trained on large datasets of known ligand-receptor interactions, enabling the rapid in silico screening of chemical libraries.
Generative AI, which leverages variational autoencoders (VAEs) or generative adversarial networks (GANs), can even design entirely new molecules with desired physicochemical properties. These models are capable of optimizing for multiple objectives—such as potency, selectivity, and toxicity—simultaneously. Notable platforms like Insilico Medicine’s Chemistry42 and Atomwise’s AtomNet have demonstrated the power of AI to reduce compound screening costs while increasing the likelihood of identifying viable leads.
Predicting ADMET Properties
Absorption, distribution, metabolism, excretion, and toxicity (ADMET) properties are critical determinants of a drug’s viability. Traditionally, ADMET profiling involves in vitro and in vivo testing, which are not only expensive but also ethically contentious due to animal usage. AI models provide a cost-effective complement by predicting ADMET characteristics based on molecular structure alone.
For instance, support vector machines (SVMs), random forests, and deep learning architectures can be trained to classify compounds based on their potential for hepatotoxicity or blood-brain barrier permeability. By filtering out compounds with undesirable profiles early in the development process, AI reduces the financial burden of late-stage attrition and minimizes the reliance on animal testing.
Natural Language Processing in Biomedical Research
Natural language processing (NLP) has emerged as an indispensable tool in AI-driven drug discovery. The biomedical literature is vast, growing at an exponential rate, and contains valuable insights buried in unstructured text. NLP algorithms can extract relevant information from scientific articles, patents, clinical trial registries, and regulatory submissions.
Advanced models like BioBERT, SciSpacy, and PubMedGPT can perform named entity recognition (NER), relationship extraction, and semantic search tailored to the biomedical domain. This allows researchers to automatically identify known drug-disease interactions, adverse event profiles, and emerging therapeutic targets. Some AI platforms even integrate NLP outputs directly into hypothesis generation engines, enabling a dynamic and iterative discovery process.
By automating literature mining, NLP significantly reduces the manual labor associated with scientific review and hypothesis validation. It also ensures that research decisions are based on the most current and comprehensive evidence base, thereby improving the rigor and efficiency of discovery workflows.
AI Platforms and Ecosystem Examples
The growing ecosystem of AI-powered drug discovery platforms reflects both the maturity and diversity of this technological frontier. Several startups and established firms have developed end-to-end platforms that span the entire early R&D pipeline:
- BenevolentAI utilizes graph-based machine learning to identify novel drug targets and reposition existing compounds.
- Insilico Medicine integrates generative AI, target discovery, and molecular docking into a unified platform.
- Atomwise employs deep learning to predict binding affinity and prioritize compound candidates.
- Recursion Pharmaceuticals uses high-dimensional phenotypic screening combined with machine vision to model cellular responses.
These companies, often in collaboration with large pharmaceutical firms, are setting new benchmarks for time and cost efficiency in preclinical R&D. Case studies have shown that AI-enabled platforms can reduce target discovery time from months to weeks, and compound screening costs by as much as 70%.
This chart visualizes the integration of AI tools across the major stages of the drug discovery process—from initial target discovery through preclinical screening and optimization. It underscores how AI is not limited to a single function but is instead transforming every critical step in the workflow.
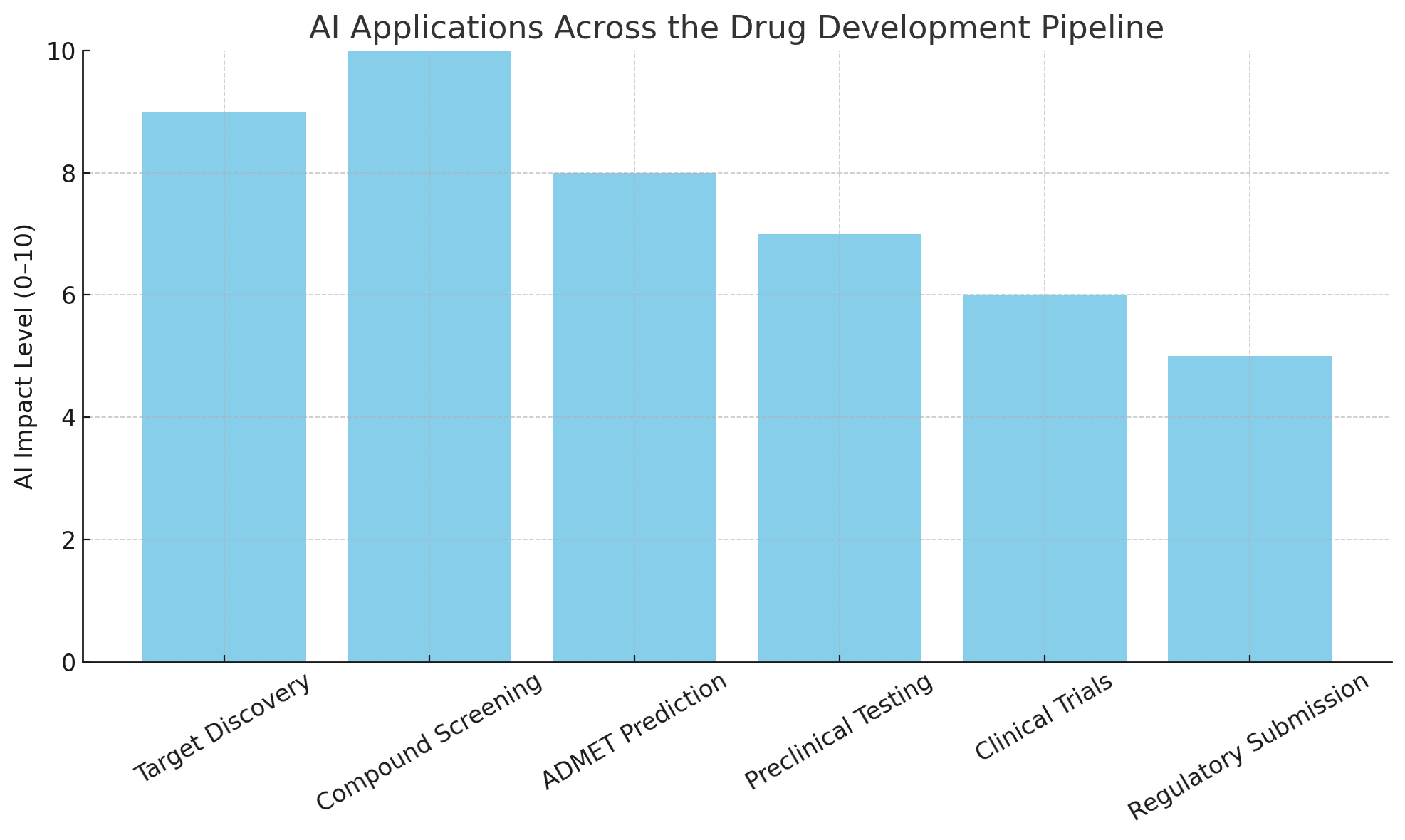
Integration with Laboratory Automation and Robotics
The benefits of AI are further amplified when combined with high-throughput laboratory automation. Robotic platforms can execute thousands of assays in parallel, and when guided by AI algorithms, the experimental search space can be intelligently reduced. This creates a feedback loop where AI models propose hypotheses, robotic systems test them, and results are fed back into the model for continuous improvement.
This iterative approach—often referred to as a “closed-loop” system—has been shown to significantly enhance the throughput and efficiency of early-stage R&D. In such systems, AI does not merely supplement human decision-making but serves as the primary driver of experimental design and optimization.
Reducing False Positives and Improving Reproducibility
Another key advantage of AI in drug discovery is its ability to reduce the rate of false positives and improve experimental reproducibility. Traditional screening methods often generate hits that cannot be replicated under different conditions or in downstream studies. AI models trained on diverse datasets are better equipped to account for confounding variables and predict which candidates are likely to translate into successful outcomes.
Reproducibility is particularly critical in pharmaceutical R&D, where regulatory scrutiny demands high levels of confidence in preclinical results. AI-driven models, by virtue of their data-driven nature, can standardize screening procedures and eliminate sources of human error, thereby enhancing the reliability of the discovery process.
AI as an Enabler of Personalized Discovery
The advent of personalized medicine requires a more nuanced approach to drug discovery—one that takes into account individual genetic, epigenetic, and environmental factors. AI excels in integrating multimodal datasets to uncover subpopulation-specific targets and therapeutic strategies.
For example, clustering algorithms can segment patient data into biologically relevant subtypes, enabling the identification of targets that would be missed in aggregated analyses. This facilitates the design of targeted therapies with improved efficacy and reduced side effects, especially in oncology, rare diseases, and immunological disorders.
AI is not merely optimizing existing drug discovery workflows—it is reimagining them. From target identification and molecule design to ADMET prediction and literature mining, AI technologies are enabling pharmaceutical companies to move faster, spend less, and discover more. The next section will explore how these AI innovations extend into the clinical and regulatory phases, further transforming the cost structure and success probability of drug development pipelines.
Accelerating Clinical Trials and Regulatory Review with AI
While artificial intelligence (AI) is revolutionizing early-stage drug discovery, its impact is equally transformative in the realm of clinical development and regulatory submission. Clinical trials—comprising Phase I through Phase III—are widely recognized as the most costly, complex, and time-consuming components of pharmaceutical research. On average, they account for more than 60% of total drug development expenses and often extend over a period of six to seven years. AI’s integration into clinical trial design, patient recruitment, real-time monitoring, and regulatory documentation is reshaping this phase, offering unprecedented opportunities to enhance efficiency, reduce attrition, and ensure data integrity.
AI in Clinical Trial Design and Simulation
Designing effective clinical trials is a balancing act between scientific rigor, ethical responsibility, and economic feasibility. Poorly designed trials can result in inconclusive outcomes, safety risks, and substantial financial losses. AI algorithms now assist in optimizing trial protocols by simulating outcomes based on historical trial data and patient population characteristics.
Advanced machine learning models are capable of predicting trial outcomes under different design parameters—such as dosage levels, treatment durations, and stratification strategies—before any participant is enrolled. These predictive simulations improve statistical power while minimizing risks, leading to better-designed studies that are more likely to achieve their endpoints.
Furthermore, digital twin technology—a novel AI-based approach—enables the creation of virtual representations of patient profiles. These digital avatars can be used to forecast responses to experimental treatments, allowing sponsors to identify ideal inclusion and exclusion criteria. This simulation-based optimization reduces the likelihood of mid-trial amendments, which are a major source of delays and increased costs.
Patient Recruitment and Stratification
Recruiting eligible participants remains one of the most significant bottlenecks in clinical research. Studies indicate that nearly 80% of clinical trials fail to meet enrollment deadlines, and some are terminated entirely due to insufficient recruitment. AI is helping to resolve this issue through the use of real-world data (RWD) derived from electronic health records (EHRs), insurance claims, wearable devices, and genomic databases.
Natural language processing (NLP) tools extract structured and unstructured information from clinical narratives, enabling more accurate patient matching based on eligibility criteria. Additionally, AI algorithms can identify subpopulations most likely to respond to a given treatment—an essential function in the era of precision medicine. This targeted recruitment not only accelerates enrollment but also increases the probability of demonstrating efficacy.
Moreover, patient retention is being enhanced through AI-powered engagement platforms. These tools can predict when participants are likely to drop out and intervene with tailored communications, reminders, or support services. In doing so, AI contributes directly to maintaining trial continuity and data quality.
Synthetic Control Arms and Real-World Evidence
Traditional clinical trials rely on randomized control groups, which can be ethically and logistically challenging, especially in rare diseases or life-threatening conditions. AI enables the development of synthetic control arms—constructed from historical patient data and real-world evidence (RWE)—to serve as comparators.
By aggregating and analyzing anonymized data from past clinical trials, patient registries, and EHRs, AI can simulate outcomes for a control group that closely mirrors the treatment cohort. Regulatory agencies such as the U.S. Food and Drug Administration (FDA) and the European Medicines Agency (EMA) have shown growing openness to synthetic control methodologies, provided the underlying data is robust and appropriately modeled.
The benefits of synthetic controls are manifold: fewer patients are required to be randomized to placebo or standard-of-care treatments, timelines are shortened, and patient recruitment becomes more ethical and efficient. Furthermore, the approach enables more rapid iteration of trial designs based on emergent RWE insights.
Real-Time Monitoring and Adaptive Trial Management
Clinical trials generate enormous volumes of data, including vital signs, lab results, adverse events, and adherence metrics. Traditionally, this data has been reviewed manually at predefined intervals, creating latency in detecting deviations or anomalies. AI addresses this challenge through real-time data ingestion and monitoring systems.
Machine learning models can flag safety signals, protocol violations, and data integrity issues as they occur. These capabilities allow for adaptive trial management, where trial parameters can be adjusted dynamically in response to interim findings. For instance, dosing regimens may be modified, or recruitment criteria refined, to optimize outcomes without compromising scientific validity.
Wearable health technologies and remote monitoring devices further augment AI’s potential. When paired with AI analytics, these devices provide continuous, high-resolution health data that enriches the trial dataset and enhances the detection of subtle treatment effects or adverse events.
AI in Regulatory Documentation and Compliance
The regulatory submission process is a documentation-intensive endeavor that requires the compilation of detailed data from across all stages of drug development. Preparing a New Drug Application (NDA) or Biologics License Application (BLA) can take several months and involves hundreds of individual documents, ranging from clinical study reports to manufacturing protocols.
AI is being deployed to automate and accelerate the drafting, review, and submission of regulatory documents. NLP and document intelligence tools can extract relevant data points from clinical trial databases and populate submission templates. These systems can also cross-reference data for consistency, flag discrepancies, and ensure compliance with regulatory standards.
In addition, AI chatbots and virtual assistants are being developed to facilitate real-time communication with regulatory bodies, enabling faster resolution of queries and requests for additional information. As agencies become more technologically agile, the regulatory ecosystem is increasingly receptive to the integration of AI-driven tools in submissions.
Key Industry Players and Solutions
Numerous technology providers and pharmaceutical companies are now leveraging AI to streamline clinical development and regulatory workflows. The following table highlights some of the most prominent examples:
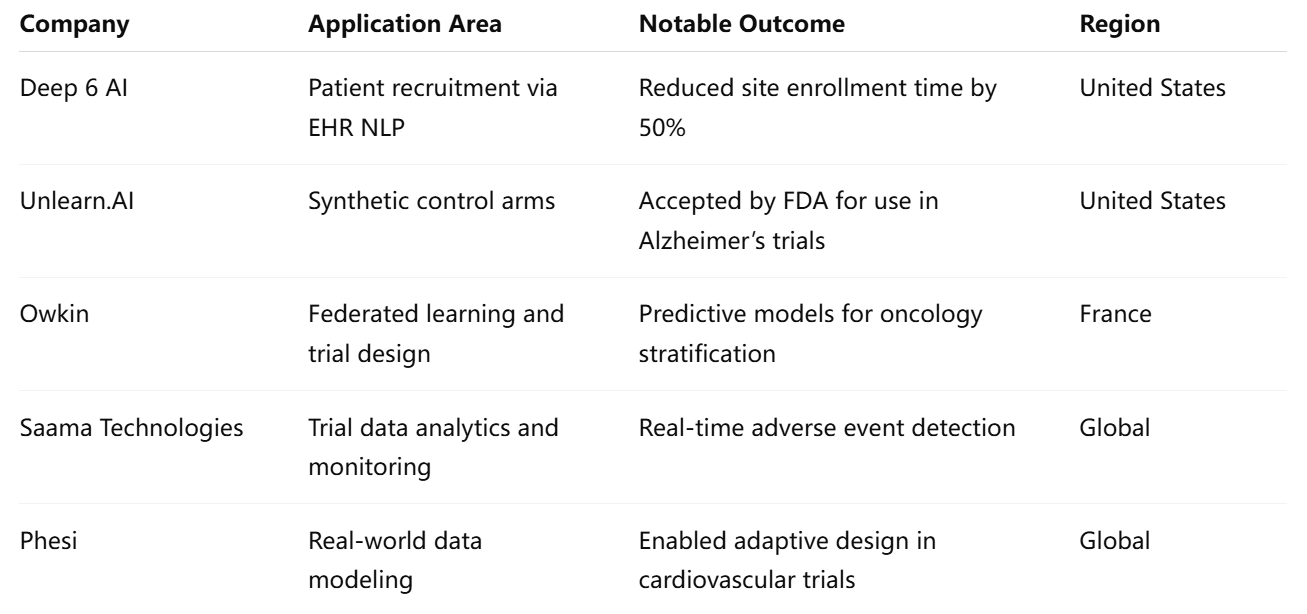
These organizations represent a growing class of AI innovators working in tandem with clinical researchers and regulatory professionals to transform the development lifecycle.
Regulatory Acceptance and Framework Evolution
AI’s increasing role in clinical development has prompted regulators to adapt their frameworks accordingly. Both the FDA and EMA have launched pilot programs to evaluate AI-assisted trials and promote transparency in algorithmic decision-making.
For example, the FDA’s 2021 draft guidance on “Artificial Intelligence and Machine Learning in Software as a Medical Device” outlines best practices for validation, data governance, and real-time updates of AI models. Similarly, the EMA has initiated consultations on the use of AI in clinical documentation and pharmacovigilance.
While regulatory bodies are not yet fully standardized in their approach to AI, the general trend is toward conditional acceptance with rigorous oversight. To gain regulatory approval, AI tools must be explainable, validated, and compliant with data privacy regulations such as HIPAA and GDPR. As AI technologies continue to mature, clearer regulatory pathways are expected to emerge.
Risks, Challenges, and Ethical Considerations
Despite its advantages, the use of AI in clinical trials and regulatory affairs is not without risks. Algorithmic bias, data quality issues, and lack of model interpretability can jeopardize trial outcomes and patient safety. Moreover, over-reliance on historical data may inadvertently reinforce health disparities or obscure novel treatment responses.
Ethical concerns also arise regarding informed consent when AI is involved in participant selection or monitoring. Transparency with trial participants about the use of AI tools and data handling practices is essential to maintaining trust and compliance.
To mitigate these risks, companies are investing in explainable AI (XAI) frameworks, rigorous validation protocols, and cross-disciplinary governance teams. Ethical AI design, stakeholder transparency, and continuous auditability are becoming non-negotiable elements in the clinical AI landscape.
AI is fundamentally reshaping the clinical development and regulatory landscape of pharmaceuticals. By enabling smarter trial design, enhancing patient recruitment, supporting adaptive management, and expediting regulatory submissions, AI delivers measurable efficiencies that reduce both time and cost. These capabilities, once considered aspirational, are now becoming operational realities for forward-thinking companies.
Financial Impact, ROI, and Market Adoption
As artificial intelligence (AI) gains traction across all stages of drug discovery and development, the conversation has increasingly shifted from theoretical benefits to measurable financial outcomes. The integration of AI into pharmaceutical research is not merely an exercise in technological sophistication; it is a strategic imperative aimed at reshaping the return on investment (ROI) landscape in one of the most capital-intensive sectors of the global economy. By reducing R&D costs, accelerating time-to-market, and improving success rates, AI offers a compelling value proposition that has begun to resonate with investors, executives, and policymakers alike.
Quantifying AI’s Economic Impact
The cost of developing a new drug traditionally ranges from $1.5 billion to $2.6 billion, with the lion’s share devoted to lengthy preclinical and clinical trials. AI intervenes at multiple points in this pipeline to produce incremental savings that compound over time. According to a study by Deloitte, biopharmaceutical companies that adopt AI-based platforms for molecule screening and predictive analytics have reported a reduction in preclinical development costs of up to 40%. Additionally, McKinsey & Company estimates that AI could help the industry save between $50 billion and $70 billion annually by improving R&D efficiency alone.
These projections are supported by real-world case studies. For example, Insilico Medicine leveraged its AI-powered drug discovery platform to identify a novel fibrosis candidate in just 18 months—less than half the typical time required. Similarly, Atomwise’s deep learning algorithms have enabled pharmaceutical partners to screen billions of compounds virtually, eliminating the need for costly high-throughput physical screening. Such outcomes demonstrate AI’s potential not only to reduce expenditures but also to unlock new market opportunities that would otherwise be cost-prohibitive.
Accelerated Time-to-Market and Its Financial Implications
Time is a critical variable in pharmaceutical economics. The faster a drug reaches the market, the sooner it can generate revenue and recover sunk costs. AI shortens the timeline across multiple stages—from early discovery to clinical validation—thereby enhancing the net present value (NPV) of development projects.
For blockbuster drugs, even a few months’ acceleration can yield significant financial benefits. Consider a cancer therapy projected to earn $1 billion annually: shaving six months off its development timeline translates to an additional $500 million in early revenue. These time savings also extend the effective patent life of the product, allowing developers to maximize profits before generic competition enters the market.
Moreover, AI-driven efficiencies in clinical trials reduce the need for rework and redundant testing, which are major contributors to timeline inflation. When paired with automation and real-time data analytics, AI ensures that development milestones are met more predictably, reducing the overall volatility of drug R&D investments.
Improved Probability of Success and Portfolio Optimization
Traditional drug development is fraught with risk, characterized by high attrition rates at every stage. A common metric used to evaluate R&D pipelines is the probability of technical and regulatory success (PTRS). AI enhances PTRS by improving target validation, refining candidate selection, and enabling more precise patient stratification in clinical trials.
Pharmaceutical companies are increasingly using AI to model the expected commercial and clinical success of each compound in their pipeline. These models incorporate variables such as biomarker prevalence, competitive landscape, anticipated pricing, and regulatory pathway complexity. By simulating multiple development scenarios, AI provides decision-makers with a probabilistic understanding of expected returns, enabling better allocation of resources and capital.
This level of strategic foresight is especially valuable in portfolio management. With the help of AI, firms can identify underperforming assets early and reallocate funds to more promising candidates, thereby optimizing the overall ROI of the R&D program. This data-driven approach stands in stark contrast to the historically intuition-based methods of pipeline prioritization.
Market Adoption and Industry Investment Trends
The value of AI in pharma is now reflected in investment patterns, strategic alliances, and M&A activity. Venture capital funding for AI-driven drug discovery startups has surged over the past five years, with deals exceeding $5 billion globally in 2023 alone. Leading players such as Recursion Pharmaceuticals, BenevolentAI, and XtalPi have secured multi-million dollar rounds, often led by prominent investors like SoftBank, BlackRock, and Temasek.
Large pharmaceutical corporations are also entering into strategic partnerships with AI firms to access proprietary platforms and expertise. For instance, Sanofi has committed over $5.2 billion in milestone payments to Exscientia for AI-designed drug candidates. Similarly, Pfizer has partnered with Iktos to leverage generative AI in its medicinal chemistry programs. These collaborations not only accelerate innovation but also serve as validation of the commercial viability of AI technologies.
Furthermore, AI’s integration into the pharmaceutical value chain is being catalyzed by institutional support. Governments and regulatory bodies are funding public-private partnerships aimed at digitizing drug development. Initiatives such as the U.S. National Institutes of Health’s (NIH) Accelerating Medicines Partnership (AMP) and the European Union’s Innovative Health Initiative (IHI) explicitly prioritize AI as a cornerstone of next-generation biomedical research.
Commercial Models and Monetization Strategies
AI companies in the pharmaceutical sector operate under a variety of commercial models. These include:
- Platform-as-a-Service (PaaS): Offering subscription-based access to AI platforms for target discovery or lead optimization.
- Milestone and Royalty Agreements: Structuring deals with biopharma partners that include upfront payments, development milestones, and post-commercialization royalties.
- Joint Ventures and Co-Development: Collaborating on specific drug programs where risk and reward are shared.
- Licensing and IP Sales: Monetizing proprietary molecules or predictive models developed through AI platforms.
These models provide flexibility in monetization and align incentives between AI providers and their pharmaceutical partners. For biopharma firms, outsourcing AI functions also reduces the need for internal technical capabilities, thereby improving operational agility.
Barriers to Broader Financial Realization
Despite its promise, the financial realization of AI’s potential in drug development is not without obstacles. Integration challenges, data silos, and regulatory uncertainties can impede implementation and delay ROI. Furthermore, the upfront costs of deploying AI systems—including talent acquisition, infrastructure investment, and model training—can be substantial.
There are also concerns about the scalability and generalizability of AI models. Tools that perform well in one therapeutic domain may require extensive retraining to function effectively in another. This can lead to fragmented deployments and inconsistent value capture across portfolios.
Additionally, cultural resistance within organizations—particularly in legacy pharmaceutical firms—can slow adoption. R&D teams accustomed to traditional methodologies may be hesitant to rely on algorithmic decision-making, especially in high-stakes clinical scenarios. Overcoming these barriers requires not only technical excellence but also change management and executive leadership.
The Future of AI-Driven Pharma Economics
Looking ahead, AI is expected to play a central role in reshaping pharmaceutical economics. As technologies mature and evidence accumulates, AI-driven drug development may evolve from a competitive differentiator to an industry standard. Emerging trends such as federated learning, explainable AI (XAI), and multimodal modeling are poised to further enhance value delivery.
Moreover, the convergence of AI with other digital health technologies—such as digital twins, blockchain, and IoT-based diagnostics—will unlock synergistic efficiencies across the drug development lifecycle. In this integrated ecosystem, the marginal cost of developing and testing a new drug could decline substantially, democratizing innovation and expanding access to underserved populations.
From a market standpoint, analysts project that the global market for AI in drug discovery and development will exceed $15 billion by 2030, growing at a compound annual growth rate (CAGR) of over 25%. This growth trajectory reflects not only expanding adoption but also the deepening financial integration of AI within pharmaceutical business models.
The financial impact of AI on drug discovery and development is profound, measurable, and rapidly evolving. By reducing R&D costs, accelerating time-to-market, enhancing success probabilities, and enabling strategic portfolio management, AI is redefining the ROI calculus for the entire pharmaceutical sector. Buoyed by robust investment, strategic alliances, and institutional support, AI adoption is set to transform drug economics from cost-prohibitive to capital-efficient.
Challenges, Ethics, and the Future Outlook
While artificial intelligence (AI) has introduced a transformative paradigm in drug discovery and development, its integration is not without substantial challenges. The technology’s promise of accelerated timelines, reduced costs, and enhanced scientific accuracy is tempered by concerns related to data quality, algorithmic bias, regulatory uncertainty, and ethical implications. As AI systems increasingly influence high-stakes decisions in the pharmaceutical sector, it is imperative to scrutinize their limitations, anticipate risks, and construct a governance framework that ensures their safe, equitable, and effective deployment.
Data Integrity and Model Generalizability
AI algorithms are only as robust as the data on which they are trained. One of the fundamental challenges in pharmaceutical AI is the heterogeneity, incompleteness, and bias inherent in biomedical datasets. Whether sourced from clinical trials, electronic health records (EHRs), or omics platforms, data often suffer from inconsistencies in format, demographic representation, and outcome reporting. These limitations can compromise the reliability and generalizability of AI models, particularly when applied to diverse patient populations or rare diseases.
The scarcity of high-quality annotated datasets in specific therapeutic areas further exacerbates the issue. In such scenarios, models trained on narrow or homogenous datasets may fail to predict outcomes accurately in real-world settings. This problem is especially pronounced in global health contexts, where underrepresented populations in training data may be disadvantaged by algorithmic decision-making.
Addressing these challenges requires the development of standardized data protocols, robust data curation strategies, and techniques such as transfer learning and federated learning, which enable the use of distributed data without compromising privacy.
Algorithmic Transparency and Explainability
A critical barrier to widespread AI adoption in pharma is the opaque nature of many machine learning models—commonly referred to as “black boxes.” Stakeholders including clinicians, regulators, and patients need to understand how an AI system arrives at a particular recommendation or decision. The lack of interpretability raises legitimate concerns about accountability and trust, particularly in clinical trial design, patient recruitment, and regulatory filings.
Explainable AI (XAI) frameworks are emerging as a solution, offering mechanisms to interpret, audit, and validate AI outputs. Techniques such as SHAP (SHapley Additive exPlanations), LIME (Local Interpretable Model-agnostic Explanations), and attention maps for neural networks enable users to gain insight into the inner workings of AI models. Regulatory agencies are increasingly emphasizing the importance of interpretability, particularly for AI applications involved in patient safety and trial management.
Nonetheless, achieving a balance between model complexity and transparency remains a technical and philosophical challenge. Simplified models may offer greater explainability but at the cost of predictive accuracy, while complex models may be more powerful but harder to scrutinize.
Ethical Considerations in AI-Driven Drug Development
The ethical dimensions of AI in drug discovery span a broad spectrum—from informed consent and data privacy to equitable access and distributive justice. One pressing concern is the potential for algorithmic bias to perpetuate or even exacerbate existing health disparities. If AI models are trained predominantly on data from wealthier nations or majority populations, they may produce suboptimal outcomes for marginalized or underrepresented groups.
In clinical trials, AI-driven participant selection mechanisms must ensure fairness and inclusivity. Over-optimization for speed or efficacy should not come at the cost of demographic representation or ethical trial design. Moreover, patients must be adequately informed when AI tools are used to guide decisions that may affect their inclusion, treatment assignment, or outcomes.
Data privacy is another ethical frontier. As AI systems increasingly rely on sensitive health data, ensuring compliance with global privacy standards such as the General Data Protection Regulation (GDPR) and the Health Insurance Portability and Accountability Act (HIPAA) is paramount. Differential privacy, data anonymization, and encryption protocols are vital technical safeguards, but ethical compliance also requires transparent communication and oversight.
Finally, questions around intellectual property (IP) and authorship are becoming more prominent. Who owns the rights to a molecule discovered by an AI? How should credit and compensation be allocated among human researchers and algorithmic contributors? These questions demand not only legal clarity but also philosophical reflection on the evolving definition of innovation.
Regulatory Evolution and Policy Challenges
The regulatory landscape for AI in pharmaceutical R&D is still in flux. While agencies such as the U.S. Food and Drug Administration (FDA), the European Medicines Agency (EMA), and the Medicines and Healthcare products Regulatory Agency (MHRA) in the UK have acknowledged the role of AI, comprehensive frameworks remain under development.
Key regulatory priorities include model validation, real-time monitoring, data traceability, and algorithmic update mechanisms. Regulatory sandboxes—controlled environments where AI systems can be tested under supervision—are being proposed as a way to encourage innovation while safeguarding public health. However, regulatory agility must be balanced with due diligence, especially in scenarios involving patient risk.
International harmonization is also a critical need. Divergent national policies could fragment the development and approval landscape, discouraging cross-border collaboration and impeding the global scaling of AI solutions. Multilateral initiatives, such as those spearheaded by the International Council for Harmonisation of Technical Requirements for Pharmaceuticals for Human Use (ICH), are essential to aligning global standards.
Long-Term Outlook: Toward Fully AI-Integrated Drug Development
The long-term trajectory of AI in pharmaceutical R&D suggests a future in which drug development becomes increasingly autonomous, continuous, and personalized. Advances in self-learning algorithms, high-fidelity simulations, and quantum computing may soon enable AI systems to design, test, and optimize drug candidates with minimal human intervention.
In parallel, AI is expected to play a pivotal role in enabling “lab-on-a-chip” systems, automated cloud-based experimentation platforms, and real-time adaptive clinical trials. These innovations will further compress development timelines, lower costs, and open the door to bespoke therapeutics tailored to individual patients or subpopulations.
However, realizing this future requires more than technological prowess. It demands a multidisciplinary approach that integrates ethical foresight, regulatory innovation, public trust, and global cooperation. The pharmaceutical industry must move beyond viewing AI as a tool and begin treating it as a partner—one that coexists with human expertise to redefine the art and science of medicine.
The transformative potential of AI in drug discovery and development is evident, but its realization hinges on navigating a complex array of challenges. From data quality and transparency to ethics and policy, the road ahead is marked by both opportunity and responsibility. Addressing these issues with rigor and foresight will ensure that AI serves not only as a catalyst for innovation but also as a cornerstone of an equitable and sustainable healthcare future.
In conclusion, the strategic deployment of AI in the pharmaceutical domain stands to redefine the economics, speed, and efficacy of therapeutic innovation—provided its integration is guided by principles of transparency, accountability, and inclusivity.
References
- Insilico Medicine – AI-powered drug discovery platform
https://insilico.com - BenevolentAI – Machine learning for target identification
https://benevolent.com - Atomwise – AI for molecular screening
https://atomwise.com - Deep 6 AI – AI-enhanced clinical trial recruitment
https://deep6.ai - Unlearn.AI – Synthetic control arms for clinical trials
https://unlearn.ai - Saama Technologies – AI analytics for clinical operations
https://saama.com - Exscientia – End-to-end AI drug design
https://exscientia.ai - Owkin – Federated learning in biomedical research
https://owkin.com - Recursion Pharmaceuticals – AI for cellular phenomics
https://recursion.com - Pfizer x Iktos Collaboration – Generative AI in drug design
https://iktos.ai