How AI Is Revolutionizing Weather Forecasting: Models, Impacts, and the Future of Prediction
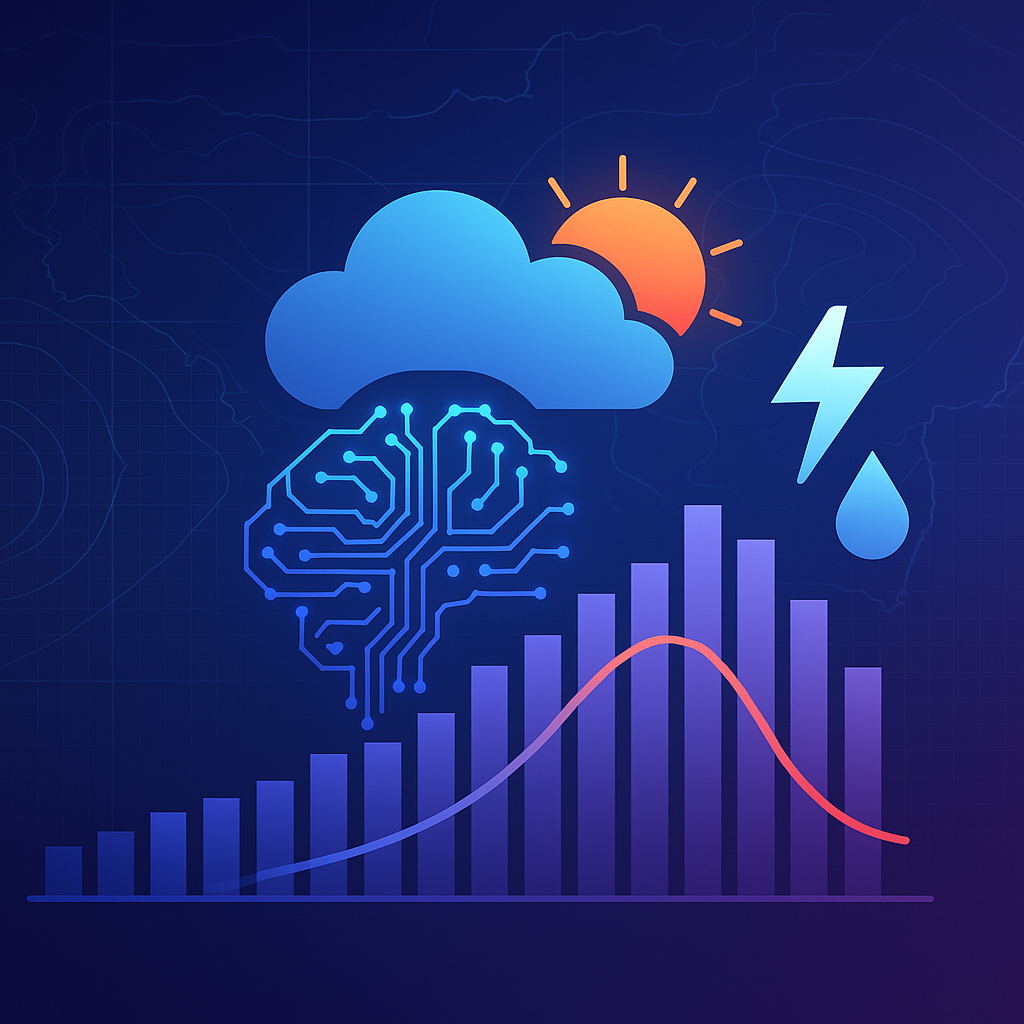
Weather prediction has long stood as one of humanity’s most essential yet formidable scientific pursuits. For centuries, people relied on barometers, cloud patterns, and empirical rules to anticipate shifts in atmospheric conditions. The advent of modern meteorology in the 20th century brought computational models based on fluid dynamics and thermodynamics, culminating in powerful Numerical Weather Prediction (NWP) systems like the European Centre for Medium-Range Weather Forecasts (ECMWF) and the Global Forecast System (GFS). These models, developed through decades of rigorous scientific effort, have been instrumental in transforming global disaster preparedness, agriculture, aviation, and daily life. Yet, despite these advancements, classical forecasting systems remain resource-intensive, time-consuming, and limited in resolution, especially when dealing with short-term or localized predictions.
In recent years, a paradigm shift has begun to unfold—one driven not by better physics-based simulations but by advances in artificial intelligence. AI, particularly deep learning and transformer architectures, is disrupting meteorology in ways that were once unimaginable. Unlike traditional models that solve complex equations for atmospheric flow, AI models learn directly from vast historical datasets, capturing patterns and anomalies through probabilistic reasoning and high-dimensional data correlations. This new approach allows for faster, more energy-efficient, and often more accurate weather predictions, challenging the supremacy of traditional NWP systems.
The breakthrough moment came when organizations like DeepMind introduced GraphCast, a transformer-based model capable of outperforming state-of-the-art forecasts with a fraction of the computational load. Not long after, NVIDIA’s FourCastNet, Huawei’s Pangu-Weather, and other AI models entered the scene, each pushing the boundaries of speed and precision. These AI systems are not just augmenting legacy tools; they are redefining the core of meteorological science. Their impact is especially profound in contexts requiring rapid updates—such as severe weather events—where timing can mean the difference between safety and catastrophe.
This revolution is not limited to research labs or elite meteorological centers. As cloud computing costs fall and models become open-source, AI weather forecasting is becoming more accessible, particularly in developing regions historically underserved by traditional infrastructure. The implications are vast—from enabling hyperlocal forecasts in remote communities to supporting renewable energy operations that depend on wind and solar predictability.
This blog post explores how AI is transforming the weather prediction landscape. It examines the constraints of legacy systems, the emergence of groundbreaking AI models, the tangible benefits across industries, and the ethical and technical challenges that lie ahead. Ultimately, it considers what the future may hold when artificial intelligence becomes a core architect of how humanity interacts with the atmosphere.
Legacy Systems and Their Limits
Weather forecasting has historically relied on physics-based models that simulate the Earth’s atmosphere through complex mathematical equations grounded in fluid dynamics and thermodynamics. These models, known as Numerical Weather Prediction (NWP) systems, have formed the backbone of global forecasting efforts for more than half a century. Developed and operated by major meteorological agencies such as the European Centre for Medium-Range Weather Forecasts (ECMWF), the National Weather Service (NWS), and the UK Met Office, these systems use supercomputers to analyze vast amounts of observational data, providing forecasts from the scale of hours to several days or even weeks ahead. However, as the urgency and complexity of climate-related phenomena escalate, the limitations of these legacy systems have come under increased scrutiny.
The Inner Workings of Traditional Models
At the heart of traditional forecasting is a discretized mathematical representation of the atmosphere. The Earth is divided into a three-dimensional grid, and atmospheric variables such as temperature, pressure, humidity, and wind are computed at each grid point. These simulations are based on the Navier-Stokes equations and require input from a range of sources, including weather balloons, satellite sensors, Doppler radars, buoys, aircraft, and ground stations.
To generate forecasts, these inputs undergo a process called data assimilation, which integrates observational data into the model’s initial conditions. The model then iteratively projects atmospheric behavior forward in time, producing outputs that forecast various weather parameters at different time intervals and locations.
This approach, while rooted in sound scientific principles, is inherently computationally expensive. A single forecast run from ECMWF, for instance, can require millions of CPU hours and petabytes of data. Even with state-of-the-art supercomputers, global weather models often need hours to generate high-resolution forecasts. As a result, real-time responsiveness—especially during fast-developing extreme weather events—can be limited.
Resolution and Scale Trade-Offs
One of the key constraints of NWP systems is the trade-off between spatial resolution, forecast horizon, and runtime efficiency. Higher-resolution models, which provide more detailed forecasts, demand exponentially greater computational resources and longer runtimes. This often forces agencies to prioritize either global coverage with coarser resolution or regional models with finer detail but narrower scope.
For example, the GFS model typically operates at a grid resolution of 13 km for global forecasts, while regional models like the High-Resolution Rapid Refresh (HRRR) in the U.S. can achieve 3 km resolution but are limited to shorter forecast horizons and smaller geographic areas. Such trade-offs can compromise the granularity and accuracy needed for applications like urban flooding alerts or localized wind pattern predictions.
Challenges in Handling Extreme and Rapid-Onset Events
Another inherent limitation of traditional forecasting is the difficulty in predicting extreme or rapidly evolving weather events such as flash floods, tropical cyclones, and atmospheric rivers. These phenomena often unfold at spatial and temporal scales that exceed the resolving power of coarse-grid models. Moreover, the non-linear behavior of the atmosphere can cause small initial errors in model inputs to propagate into significant inaccuracies over time—a concept known as the butterfly effect.
Despite frequent updates and calibration, legacy models occasionally fail to capture sudden deviations in storm trajectories, precipitation levels, or temperature spikes. This issue is particularly problematic for emergency management, aviation, and supply chain operations, where timing and precision are critical.
Geographic Inequities in Forecast Accuracy
Access to accurate forecasts also varies widely around the globe. Advanced meteorological infrastructure—satellites, radars, and sensor networks—is unevenly distributed, often concentrated in North America, Europe, and parts of East Asia. Developing countries, especially in Sub-Saharan Africa, South Asia, and island nations, may lack the real-time data streams and computing capabilities needed to run or interpret NWP models.
This disparity can result in forecasting blind spots that leave vulnerable populations less equipped to prepare for natural disasters. Although efforts like the World Meteorological Organization's (WMO) Global Framework for Climate Services (GFCS) aim to bridge this gap, challenges persist in data standardization, model accessibility, and regional training.
High Cost and Environmental Impact
Running global weather models is not only computationally demanding but also energy-intensive. Supercomputers like the Cray XC40 and Fugaku consume megawatts of power to process global simulations. This has sparked growing concern about the environmental footprint of numerical forecasting in an era increasingly focused on sustainability.
In addition to direct energy use, the cost of maintaining and updating infrastructure—hardware, software, skilled personnel—represents a major financial burden for governments and research institutions. These expenses can limit the frequency of forecast updates or slow the adoption of innovations, especially in underfunded agencies.
Inflexibility in Model Evolution
Although traditional models are continually improved, their evolution is slow and methodologically constrained. Enhancements require updates to both the physical equations and the numerical solvers, followed by extensive validation. In contrast, AI-based models, as will be discussed in the next section, can leverage advances in computing and data availability much more rapidly, retraining on newer datasets and scaling their capabilities in ways that legacy systems cannot easily match.
Furthermore, NWP systems operate on rule-based, deterministic logic that can struggle to assimilate certain types of unstructured or indirect data—such as social media reports, satellite imagery in non-standard formats, or data from emerging Internet of Things (IoT) weather sensors. This limitation reduces the adaptability of classical models in real-time decision environments.
Summary of Legacy Limitations
In summary, traditional weather forecasting systems—while scientifically rigorous and historically transformative—are increasingly constrained by:
- Computational complexity and latency
- Resolution trade-offs and scalability challenges
- Difficulties in predicting high-impact weather events
- Inequitable access and infrastructure disparities
- High operational and environmental costs
- Limited flexibility and adaptability
As the demand for faster, more accurate, and more localized forecasts grows—driven by climate volatility, urbanization, and digital integration—the need for an alternative paradigm has become apparent. Artificial intelligence, with its capacity to model complex systems through data-driven learning, is emerging as a transformative force poised to complement or even surpass many of the capabilities of traditional systems.
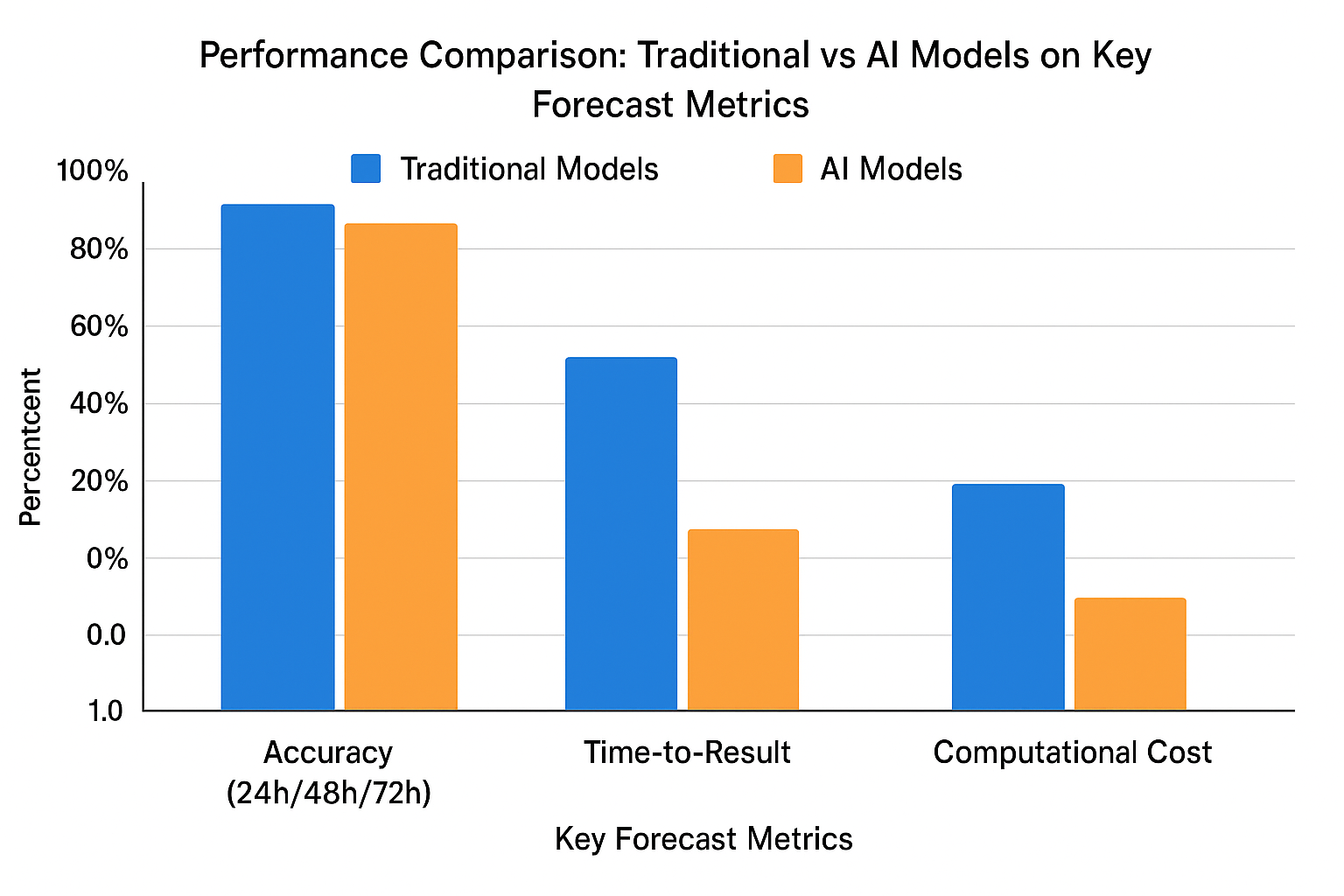
AI Enters the Forecast – Models, Methods, and Breakthroughs
The limitations of traditional Numerical Weather Prediction (NWP) systems have catalyzed interest in a fundamentally different approach to weather forecasting—one that leverages the data-rich, pattern-driven strengths of artificial intelligence. Rather than explicitly solving the physical equations that govern atmospheric motion, AI-based forecasting systems learn to emulate the behavior of the atmosphere through exposure to massive volumes of historical data. This paradigm shift is redefining not only how forecasts are produced but also the pace, scale, and accessibility with which they are delivered.
The Emergence of AI in Meteorology
The growing adoption of artificial intelligence in meteorology is a product of both technological advancement and practical necessity. High-performance computing (HPC) infrastructure, access to exabyte-scale historical weather archives, and breakthroughs in deep learning have all converged to make AI-powered weather forecasting feasible. AI systems ingest large datasets containing reanalysis products, satellite imagery, and ground observations to train models that can forecast future states of the atmosphere with high fidelity.
Unlike deterministic NWP models, AI models are statistical or machine learning-based systems. These models can identify complex spatial and temporal patterns without the need to explicitly encode atmospheric physics. By analyzing historical input-output pairs—such as past satellite data and the corresponding weather conditions—these systems generalize rules that are then applied to new data inputs to predict future weather states. This model-free approach is particularly effective for short- to medium-term forecasting and excels in speed and scalability.
Breakthrough AI Models in Weather Forecasting
Several pioneering AI models have emerged as leaders in this evolving domain, each contributing unique architectural innovations and practical achievements.
GraphCast (DeepMind)
Developed by DeepMind, GraphCast is a transformer-based deep learning model that forecasts future atmospheric conditions using historical weather data as its sole input. Unlike traditional models, GraphCast does not solve fluid dynamic equations. Instead, it relies on a graph neural network architecture that encodes spatial dependencies between grid cells, allowing it to learn patterns across space and time.
GraphCast has demonstrated state-of-the-art performance, outperforming the ECMWF's operational HRES model on over 90% of tested forecast variables and lead times. It can produce 10-day global forecasts in under a minute on a single Google TPU, compared to hours on traditional supercomputers. The model’s efficiency, scalability, and accuracy represent a watershed moment in AI-driven meteorology.
FourCastNet (NVIDIA and Lawrence Berkeley National Laboratory)
FourCastNet is another transformative model that combines a Fourier Neural Operator (FNO) with advanced parallel computing techniques. Developed by NVIDIA in partnership with Lawrence Berkeley National Laboratory, this model has shown remarkable success in emulating high-resolution global climate simulations.
FourCastNet is trained on the ERA5 reanalysis dataset and can forecast multiple atmospheric variables, including wind speed, pressure, and temperature, up to 10 days in advance. Its use of spectral methods enables fast convergence and effective representation of geophysical phenomena. Crucially, FourCastNet can achieve 100x speedups over traditional models while maintaining competitive accuracy, which has made it particularly attractive for operational use and disaster preparedness.
Pangu-Weather (Huawei)
Huawei's Pangu-Weather is a deep learning model specifically designed to produce high-resolution weather predictions. It employs a hybrid transformer-based architecture and a three-dimensional hierarchical forecasting mechanism that operates across multiple atmospheric layers.
Notably, Pangu-Weather has surpassed many traditional forecasting systems in metrics such as root-mean-square error (RMSE) and anomaly correlation coefficient (ACC). One of its key innovations lies in its ability to forecast typhoons and extreme precipitation events with improved spatial precision. In benchmark tests, Pangu-Weather achieved forecast accuracy comparable to ECMWF HRES while using significantly fewer computational resources.
Fengwu (China Meteorological Administration)
Fengwu is a powerful AI-driven weather model developed by the China Meteorological Administration (CMA). Designed to address regional forecasting needs in East Asia, Fengwu integrates both satellite data and historical forecasts to deliver rapid updates across multiple vertical layers of the atmosphere.
Its architecture combines convolutional and recurrent neural networks, allowing it to handle spatiotemporal dependencies effectively. Fengwu has been credited with improving early warning systems for extreme rainfall and heatwaves in China’s central and southern provinces. Its real-time deployment in national emergency protocols highlights the growing operational trust placed in AI weather forecasting.
AI Methods and Core Architectural Features
The success of these AI weather models is grounded in several key methodological innovations:
- Transformers and Attention Mechanisms: Borrowed from natural language processing, transformers excel at identifying long-range dependencies, which are critical in understanding large-scale weather phenomena like jet streams and atmospheric rivers.
- Fourier Neural Operators (FNOs): These allow AI models to learn solutions to partial differential equations in frequency space, enabling high-resolution forecasting without resorting to grid-based solvers.
- Diffusion Models: Emerging techniques such as generative diffusion models are being explored for probabilistic weather forecasting, offering more realistic uncertainty quantification.
- Data Assimilation and Pretraining: Modern AI systems increasingly incorporate techniques akin to data assimilation by fine-tuning on real-time data streams or using contrastive learning on satellite imagery and reanalysis archives.
- Multi-Scale Learning: Hierarchical models operate at both coarse and fine resolutions, enabling accurate forecasts across global and regional scales.
Advantages over Traditional Models
AI weather models bring numerous advantages over their traditional counterparts:
- Speed and Efficiency: While conventional NWP runs can take hours on a supercomputer, AI models can produce results in seconds using consumer-grade GPUs or TPUs.
- Lower Computational Costs: AI systems require significantly less energy, making them more sustainable and cost-effective.
- Higher Update Frequency: Fast inference enables more frequent updates, critical for nowcasting and short-term alerts.
- Improved Accuracy in Key Areas: Benchmarking has shown AI models performing better in predicting wind fields, precipitation patterns, and hurricane trajectories.
- Scalability and Accessibility: Many AI models are open-source and cloud-deployable, allowing broader access in developing regions.
- Adaptability: AI models can be retrained or fine-tuned with new data, allowing them to evolve continuously without manual reprogramming.
Independent Validation and Benchmarking
Credibility in weather forecasting is built on rigorous validation. AI models have been subject to peer-reviewed studies, cross-evaluation against benchmark datasets, and side-by-side comparisons with ECMWF and GFS outputs. Studies published in journals such as Nature and Geophysical Research Letters have confirmed that AI models meet or exceed the performance of traditional methods across multiple forecast horizons.
Moreover, the World Meteorological Organization (WMO) and national weather services are beginning to formally evaluate these models for operational use. Initiatives like the AI Weather Challenge and Climate Hackathons are further driving innovation and transparency.
Barriers to Entry and Remaining Challenges
Despite their promise, AI weather models are not without limitations. They often require:
- Massive volumes of high-quality, well-labeled historical data.
- Advanced compute resources for initial training.
- Careful tuning to avoid overfitting and generalization errors.
Additionally, while AI models excel at prediction, they may lack interpretability, making it harder for meteorologists to diagnose the cause of a specific forecast. Hybrid approaches, combining AI forecasts with traditional NWP outputs and domain expertise, are currently being explored to mitigate this issue.
Real-World Applications and Impact
The practical implications of artificial intelligence in weather forecasting extend far beyond academic benchmarks and experimental models. AI-powered weather systems are increasingly being adopted across diverse sectors, offering substantial improvements in accuracy, lead time, and granularity. These advancements are particularly consequential in domains where timely and precise meteorological information directly influences safety, productivity, and resilience. From early warning systems and agricultural planning to logistics optimization and disaster response, the integration of AI in weather prediction is catalyzing systemic change across industries and geographies.
Enhancing Early Warning Systems and Disaster Response
Perhaps the most life-critical application of AI-driven forecasting lies in extreme weather event detection and response coordination. Traditional systems often struggle to deliver high-confidence predictions of localized, fast-evolving events such as flash floods, cyclones, and heatwaves. AI models, by contrast, can rapidly ingest updated satellite imagery, radar inputs, and historical weather anomalies to generate more dynamic and adaptive forecasts.
During Hurricane Ian in 2022, for instance, AI-enabled forecasting tools provided emergency responders and meteorological agencies with earlier insights into the storm's landfall trajectory and wind field evolution. This additional lead time proved instrumental in issuing evacuation orders and staging relief efforts, especially in vulnerable coastal areas. Similarly, in Southeast Asia, Huawei’s Pangu-Weather model has been used by local governments to detect impending typhoons and cloudbursts with improved resolution, allowing for more timely alerts to rural populations.
AI also enables more targeted communication strategies. Using natural language processing (NLP) and sentiment analysis, agencies can assess the public's response to alerts disseminated via social media and adjust messaging in real time. This feedback loop ensures that life-saving information is not only accurate but also effectively received and acted upon.
Agricultural Optimization and Food Security
Agriculture is inherently weather-dependent, and small variations in temperature, precipitation, or wind can significantly impact crop yield and food prices. AI-driven weather models empower farmers, agronomists, and agritech firms with predictive tools that offer more localized and actionable insights.
In Africa and South Asia, where traditional meteorological infrastructure is often sparse, AI-based mobile platforms like Tomorrow.io and ClimaCell have been deployed to deliver hyperlocal forecasts to smallholder farmers. These tools inform decisions about planting schedules, irrigation timing, and pest control, thereby reducing crop failure risk and enhancing resilience against climate variability.
Furthermore, AI can integrate with precision agriculture systems, combining meteorological forecasts with soil sensors, satellite imagery, and drone surveillance to enable real-time agricultural management. This integration facilitates more efficient fertilizer use, reduces water waste, and improves harvest scheduling, ultimately contributing to both economic and environmental sustainability.
Logistics, Aviation, and Maritime Operations
In the transportation sector, weather forecasting plays a pivotal role in route planning, delay mitigation, and risk management. Airlines, shipping companies, and logistics providers are increasingly turning to AI models for rapid, accurate forecasts that allow for dynamic scheduling and operational adjustments.
Airlines, for example, use AI-enhanced forecasts to anticipate turbulence, icing conditions, and wind shear, enhancing passenger safety and fuel efficiency. Real-time wind predictions derived from AI models enable fuel-optimized flight paths, cutting costs and emissions. Similarly, ports and maritime fleets rely on AI to forecast sea conditions, improving docking schedules and minimizing weather-related disruptions.
In the trucking and rail industries, AI-enabled weather platforms support intelligent fleet routing, helping logistics firms avoid hazardous conditions and improve delivery timelines. During winter storms or floods, AI can prioritize routes less susceptible to closure, reroute cargo proactively, and even forecast impacts on inventory levels across warehouses.
Renewable Energy and Grid Stability
The proliferation of renewable energy infrastructure—solar, wind, and hydropower—has created a heightened need for short-term and ultra-short-term forecasting. Since these energy sources are inherently variable and weather-dependent, AI models are now essential to managing their integration into national power grids.
Solar and wind farm operators use AI weather forecasts to anticipate generation capacity, allowing for better alignment of supply with demand. Predictive analytics inform when turbines should be shut down to avoid mechanical stress or when to activate battery storage systems to buffer supply fluctuations. For example, in Germany, AI-driven weather forecasting has been linked with predictive maintenance schedules and automated curtailment decisions, enhancing grid reliability while reducing financial losses.
Moreover, utility companies use AI forecasts to anticipate weather-driven spikes in electricity usage—such as heatwaves triggering air conditioning demand—and adjust energy pricing, procurement, and distribution accordingly. This real-time adaptability not only ensures grid stability but also reduces the risk of blackouts and overloading during peak conditions.
Climate Resilience in Underserved Regions
One of the most transformative impacts of AI weather forecasting is the democratization of meteorological intelligence. In many low-income or geographically isolated regions, traditional forecasting services remain inaccessible due to a lack of ground stations, high-performance computing infrastructure, or technical expertise.
AI systems, especially when deployed via cloud services or edge devices, are lowering the barrier to entry. Projects like Microsoft’s AI for Earth and the WMO’s Global Framework for Climate Services are facilitating open access to AI weather APIs and training datasets, enabling local governments, NGOs, and research institutions to develop context-specific forecast tools.
For example, in the Pacific Islands, where early detection of tropical storms is critical, open-source AI models have been implemented on local servers to run near-real-time simulations. These solutions not only fill geographic blind spots but also empower local agencies to make informed decisions without reliance on distant or under-prioritized global systems.
Urban Planning and Smart City Applications
Urban areas present complex microclimates due to heat islands, dense construction, and transportation systems. AI-enhanced nowcasting models, which predict weather within a 0–6 hour window, are becoming increasingly useful in urban planning and smart infrastructure deployment.
Municipal governments use AI-generated forecasts to manage drainage systems, activate snow removal fleets, and regulate public transit during adverse weather. Integration with Internet of Things (IoT) devices such as smart traffic lights, air quality sensors, and digital billboards allows for real-time adjustments that enhance public safety and mobility.
Additionally, emergency shelters, hospitals, and public utilities are leveraging AI forecasts to simulate impact scenarios and preposition resources in anticipation of storms, floods, or heatwaves. As cities become more data-driven, AI weather models will play a central role in shaping resilient urban ecosystems.
A Paradigm Shift in Forecasting Culture
Perhaps most importantly, the proliferation of AI forecasting tools is leading to a cultural shift in how weather data is consumed and applied. Traditional forecasts were often centralized, produced by national agencies and disseminated in fixed formats. Today, weather prediction is increasingly personalized, context-aware, and integrated into digital ecosystems.
Mobile apps now deliver real-time alerts based on a user’s GPS location and risk profile. API-based platforms enable developers to build custom forecasting solutions for sectors as diverse as tourism, event planning, insurance, and healthcare. Weather is no longer a static bulletin—it is a living data stream, shaped by machine learning and tailored to decision-making contexts.
This democratization of weather forecasting, enabled by artificial intelligence, is not merely about faster computation. It represents a shift toward proactive, anticipatory governance and operational agility across every domain affected by meteorological risk.
Limitations, Ethical Challenges, and Human-AI Synergy
While the rise of artificial intelligence in meteorology promises transformative improvements in speed, accuracy, and accessibility, it is not without its drawbacks. As with any powerful technology, AI-based weather forecasting introduces new limitations, ethical concerns, and systemic dependencies that must be carefully navigated. These challenges are especially significant in the context of public safety, scientific accountability, and global equity. Understanding and addressing these issues is critical to building trustworthy and sustainable AI-powered forecasting systems.
Technical Limitations and Model Transparency
One of the primary technical limitations of AI weather models lies in their lack of interpretability. Unlike traditional Numerical Weather Prediction (NWP) systems, which are grounded in physical laws and equations, most AI models operate as “black boxes.” Their internal decision-making processes—how they weigh inputs, process variables, and produce outputs—are often opaque even to their developers. This poses a serious challenge in scientific contexts where model transparency is vital for validation, error diagnosis, and public trust.
For example, when an AI system produces an unexpected or incorrect forecast, it is often difficult to trace the specific cause of the error. Was it due to a poor-quality input, a statistical anomaly in the training data, or a flaw in the model’s structure? This lack of traceability undermines confidence in critical scenarios such as storm tracking or flood warnings, where accountability can have legal and life-or-death implications.
Efforts are underway to improve model explainability through techniques such as saliency mapping, attention visualization, and post hoc interpretability tools, but these approaches remain in early stages. Bridging the gap between statistical performance and scientific transparency is essential if AI is to be embraced as a foundational forecasting tool.
Data Quality and Representation Bias
AI models are inherently dependent on the quality and distribution of their training data. Many leading models have been trained on datasets like ERA5 or historical satellite records, which are richer in data from North America, Europe, and parts of East Asia. As a result, regions with limited observational infrastructure—such as Sub-Saharan Africa, parts of South America, and small island nations—are underrepresented in these training corpora.
This data imbalance can lead to regional forecast inaccuracies and exacerbate existing disparities in weather intelligence. AI models may perform exceptionally well in data-rich environments while failing to generalize in regions where accurate predictions are most needed. Moreover, low-resolution or outdated input data can degrade model performance, even if the model architecture itself is state-of-the-art.
To mitigate these issues, efforts must be made to increase data diversity, global sensor coverage, and open access to training repositories. Public-private collaborations, international data-sharing agreements, and low-cost sensor networks can all contribute to closing these representation gaps.
Risk of Overreliance and Skill Erosion
As AI systems become more accurate and efficient, there is a growing concern about the overreliance on automated forecasts at the expense of human meteorological expertise. This dynamic mirrors developments in other AI-integrated domains—such as aviation, medicine, and finance—where automation can lead to skill erosion and diminished situational awareness among professionals.
Human meteorologists bring contextual knowledge, interpretative nuance, and an understanding of local microclimates that purely statistical models may overlook. There is a legitimate fear that, in the rush to adopt AI tools, organizations may marginalize human judgment or reduce investments in traditional expertise development.
To address this, a hybrid model of human-AI collaboration must be embraced. Rather than replacing meteorologists, AI should be positioned as a decision-support system—augmenting human analysis, automating repetitive tasks, and freeing experts to focus on complex, ambiguous, or high-impact forecasting challenges.
Ethical Use and Misuse of Forecasting Technology
The ethical implications of AI in weather forecasting extend beyond questions of accuracy and bias. As AI-generated forecasts become more granular and probabilistic, they also become more valuable in commercial, political, and security contexts. This opens the door to potential misuse.
For example, highly localized forecasts could be exploited for financial speculation in agriculture or energy markets, giving unfair advantage to entities with exclusive access to AI platforms. Similarly, governments or corporations may withhold high-resolution forecasts from certain populations for strategic or economic reasons. These scenarios raise critical questions about data ownership, access equity, and the role of private companies in managing essential meteorological infrastructure.
Ethical frameworks, transparency mandates, and international guidelines are necessary to ensure that AI-powered weather intelligence serves the public good. Organizations such as the World Meteorological Organization (WMO) and UNESCO must take the lead in establishing norms around responsible AI deployment in meteorology.
Adversarial Risks and Security Concerns
AI systems are also vulnerable to adversarial attacks and manipulation. Malicious actors could theoretically introduce corrupted input data—through spoofed satellite imagery, manipulated sensor feeds, or compromised API endpoints—to influence AI forecasts in targeted regions. This could have profound implications in wartime, disaster response, or political disruption scenarios.
Moreover, as weather forecasting becomes increasingly digitized and cloud-based, cybersecurity threats to forecasting infrastructure are becoming more relevant. AI models and the data pipelines that support them must be secured with the same rigor applied to other critical infrastructure systems.
Legal and Liability Complexities
AI weather forecasts are increasingly influencing decisions in transportation, agriculture, insurance, and emergency management. This raises a thorny legal question: Who is liable when an AI forecast causes harm? If a model incorrectly predicts a storm’s path, leading to property damage or loss of life, can the developers, users, or deploying agencies be held accountable?
Current legal frameworks are ill-equipped to handle such cases. While traditional meteorological errors are often protected by sovereign immunity or scientific uncertainty clauses, AI-generated outputs complicate the attribution of fault. As adoption scales, new legal standards must be developed to address responsibility, transparency, and redress mechanisms.
Building Trust Through Human-AI Synergy
Despite these challenges, the future of AI in meteorology is not one of replacement, but partnership. Meteorologists and AI systems each bring unique strengths to the table. While AI excels at pattern recognition, fast inference, and large-scale optimization, human forecasters offer contextual awareness, scientific skepticism, and empathy—particularly crucial when communicating risk to the public.
To maximize the value of this partnership, institutions must invest in:
- Training programs to educate meteorologists on AI tools and limitations.
- Collaborative platforms that combine NWP outputs, AI models, and human interpretations.
- Feedback systems that allow humans to fine-tune or override AI-generated forecasts based on new observations or emerging patterns.
Furthermore, the integration of AI must be framed within ethical design principles, including fairness, accountability, transparency, and inclusivity. Only by foregrounding these values can society fully harness the benefits of AI-driven forecasting while avoiding its potential pitfalls.
The Future of Forecasting – Beyond Earth’s Weather
As artificial intelligence redefines the capabilities of terrestrial weather prediction, its potential reaches even further—toward an entirely new frontier of forecasting. The convergence of AI, space exploration, climate science, and sensor networks signals a profound evolution in how humanity understands and anticipates atmospheric behavior, not only on Earth but across multiple environments and temporal scales. This section explores the future of forecasting in an age of intelligent systems—one where prediction becomes global, autonomous, and extended far beyond the immediate concerns of tomorrow’s weather.
Nowcasting and Ultra-Short-Term Precision
One of the most promising frontiers in AI-driven forecasting lies in nowcasting, or ultra-short-term prediction ranging from minutes to six hours ahead. This domain is especially critical for managing sudden, high-impact weather phenomena such as thunderstorms, urban flash floods, tornadoes, and aviation turbulence. Traditional NWP systems often lack the speed and resolution to deliver reliable nowcasts at scale. In contrast, AI models trained on high-frequency radar and satellite data are ideally suited for this task.
Emerging systems like DeepMind’s MetNet and Google’s GraphCast+ are being optimized to produce granular, localized nowcasts that update in near real time. These tools will not only support emergency response but also power autonomous systems such as self-driving vehicles, drone fleets, and smart infrastructure that require instantaneous environmental awareness. In essence, AI is transforming weather from a static broadcast into a dynamic data feed, fueling decision-making in milliseconds.
Fusion of Climate Modeling and AI Forecasting
In addition to short-term weather prediction, AI is poised to enhance climate modeling and long-term scenario analysis. While weather and climate operate on different timescales, they are intimately connected through shared data structures, physical dynamics, and regional feedback loops. Historically, climate models have required months of supercomputer runtime to simulate century-scale scenarios. AI models, trained on both synthetic climate data and reanalysis archives, offer a path toward accelerating these simulations.
Research efforts such as NVIDIA’s Earth-2, a digital twin of the planet built for climate forecasting, illustrate how AI can compress computational timelines and deliver multi-decade projections with regional granularity. This capability is essential for urban planners, environmental policy-makers, and insurers who must anticipate long-term climate risk with both precision and agility. Moreover, the integration of AI enables ensemble forecasting, where multiple climate futures are explored in parallel, accounting for diverse emissions pathways and socio-political assumptions.
Space Weather and Interplanetary Meteorology
AI’s utility in forecasting is not confined to Earth’s atmosphere. The field of space weather—which includes solar flares, coronal mass ejections, and geomagnetic storms—is increasingly reliant on machine learning for real-time monitoring and prediction. These events can disrupt satellites, power grids, aviation routes, and communication systems, with global implications for infrastructure and security.
AI models trained on solar imagery and magnetospheric data from spacecraft such as NASA’s Solar Dynamics Observatory (SDO) are already being used to predict space weather anomalies. By analyzing time series data and spectral signatures, these models can offer early warnings of geomagnetic disturbances that were previously undetectable until after impact. Future AI systems may even integrate multi-planet telemetry to track interplanetary atmospheric flows, paving the way for space-based climate synchronization models.
Meanwhile, space agencies are applying AI to planetary weather forecasting. NASA’s Mars rovers, for example, use on-board machine learning to anticipate dust storms, temperature drops, and wind events—critical for both robotic autonomy and future human missions. As interplanetary exploration expands, AI-driven meteorology will become a foundational component of extraterrestrial logistics, colonization strategies, and planetary science.
Internet of Things (IoT) and Edge AI Integration
As forecasting becomes more localized and dynamic, the demand for edge-based AI systems is rising. Edge AI refers to the deployment of machine learning models on decentralized devices such as smartphones, drones, weather balloons, and IoT sensors. These systems can perform on-device inference, enabling rapid decision-making without relying on cloud infrastructure or internet connectivity.
Edge AI forecasting systems are especially beneficial in rural or disaster-prone areas, where connectivity may be limited. For example, an AI model embedded in an agricultural sensor can detect early signs of frost or drought and trigger automated alerts or irrigation adjustments. In coastal zones, edge-based tsunami detectors can analyze wave behavior in real time and issue rapid warnings without centralized processing.
Moreover, as urban infrastructure becomes “smart,” AI weather forecasts will interface with city-wide sensor networks to regulate traffic flows, adjust building HVAC systems, and manage electric vehicle charging schedules—all in response to real-time environmental conditions.
Toward a Global Autonomous Forecasting Network
Looking ahead, the trajectory of AI weather forecasting points toward a global autonomous forecasting network—a continuously learning, decentralized ecosystem that assimilates data from satellites, terrestrial sensors, and digital platforms. This system would not only forecast the weather but also simulate its potential impacts across domains: health, mobility, finance, food, and infrastructure.
Such a network would be capable of:
- Self-updating models based on new observations and outcomes.
- Localized feedback loops, where forecasts adapt based on user behavior and regional dynamics.
- Cross-disciplinary integration, blending meteorological insights with epidemiology, economics, and environmental science.
The foundation for this vision is already being laid by cloud platforms, AI research labs, and international meteorological alliances. However, to achieve it fully, significant challenges around data governance, model interoperability, energy efficiency, and equitable access must be addressed.
A New Era of Atmospheric Intelligence
In conclusion, the future of forecasting is not simply about knowing tomorrow’s temperature—it is about understanding the probabilistic evolution of planetary systems and preparing humanity to thrive amid uncertainty. Artificial intelligence enables this shift by translating massive, chaotic data flows into structured, actionable knowledge.
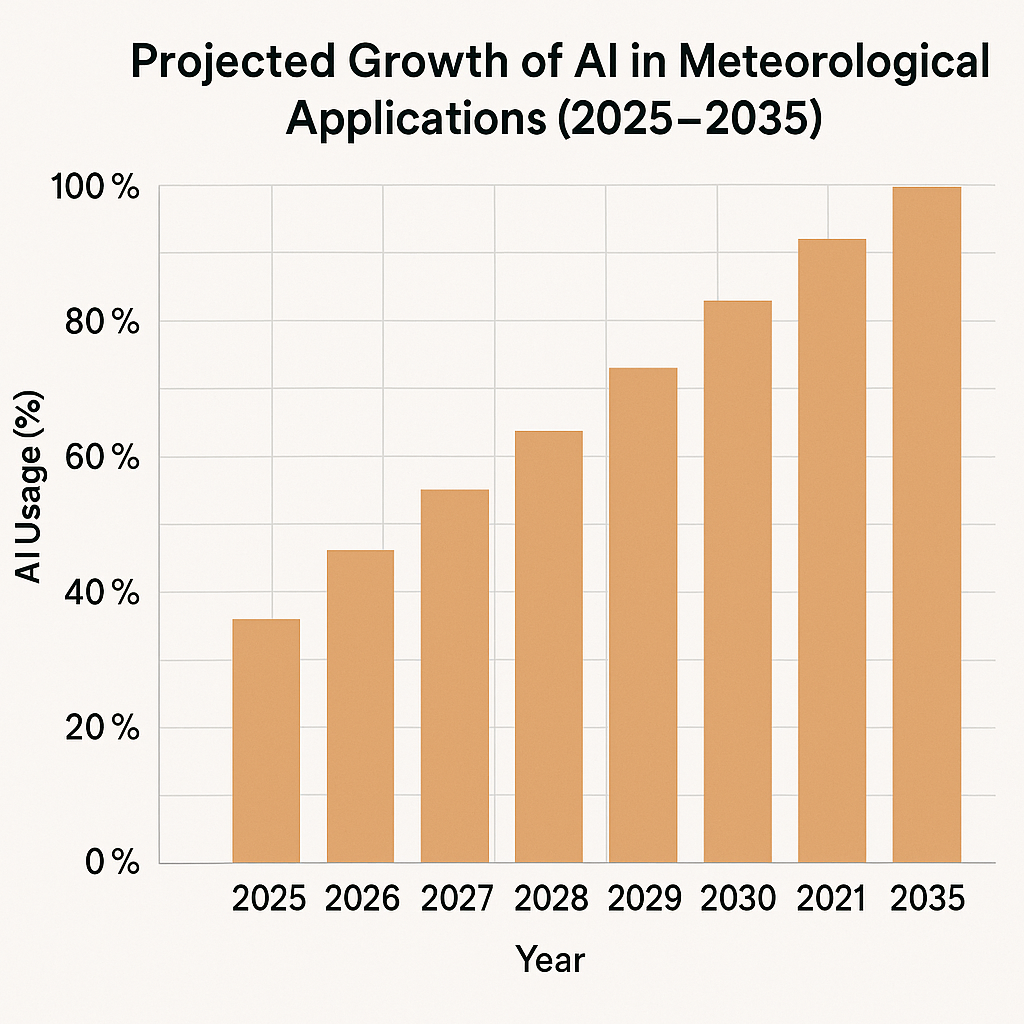
From ultra-local nowcasts to planetary simulations, from Earth’s tropics to Mars’s plains, AI is broadening the scope and depth of what is forecastable. As we look to the future, it is not hyperbole to suggest that the next generation of forecasting systems will be as intelligent and adaptive as the atmospheric systems they seek to model.
Conclusion
The integration of artificial intelligence into the domain of weather forecasting represents one of the most significant scientific and technological transformations in the history of meteorology. What was once an endeavor dominated by deterministic equations, supercomputing infrastructure, and centralized national agencies is now evolving into a dynamic, data-driven ecosystem powered by machine learning, real-time inference, and global accessibility. AI models have not merely accelerated forecasting—they have redefined its architecture, applications, and potential.
From outperforming legacy Numerical Weather Prediction systems in both speed and accuracy to enabling hyperlocal nowcasts and planetary-scale climate simulations, AI is reshaping how forecasts are produced, consumed, and acted upon. Its real-world impacts are already evident: more effective early warning systems, optimized agriculture, enhanced renewable energy integration, and smarter cities. Yet, these advancements come with necessary caveats. As this blog has shown, concerns around model transparency, data equity, ethical governance, and human expertise must remain central to the development and deployment of AI-based forecasting systems.
Importantly, the future of weather prediction should not be envisioned as a binary replacement of human meteorologists by machines. Instead, it should be seen as a collaborative evolution—one where AI augments human insight and expands our collective capacity to understand and respond to atmospheric phenomena. Meteorologists, with their contextual judgment and scientific rigor, are indispensable partners in interpreting, refining, and communicating AI-generated forecasts.
As the climate crisis intensifies, extreme weather becomes more frequent, and digital systems permeate every aspect of daily life, the value of precise, fast, and adaptive forecasting cannot be overstated. Artificial intelligence offers the tools to meet these challenges—but only if developed with care, distributed equitably, and governed responsibly.
The AI revolution in weather forecasting is not a distant prospect; it is unfolding now. By embracing its promise while acknowledging its risks, society can usher in an era of atmospheric intelligence that is not only more accurate, but also more inclusive, ethical, and resilient.
References
- DeepMind’s GraphCast Overview
https://deepmind.google/discover/blog/graphcast-ml-weather-forecasting - NVIDIA FourCastNet Technical Blog
https://developer.nvidia.com/blog/ai-weather-forecasting-fourcastnet - Huawei Pangu-Weather Whitepaper
https://www.huaweicloud.com/intl/en-us/blogs/panguweather - ECMWF Operational Forecasts
https://www.ecmwf.int/en/forecasts/datasets - Google AI Blog: Nowcasting with Machine Learning
https://ai.googleblog.com/nowcasting-precipitation-with-machine.html - World Meteorological Organization (WMO) AI Position
https://public.wmo.int/en/media/news/ai-and-weather-forecasting - Microsoft AI for Earth Initiative
https://www.microsoft.com/en-us/ai/ai-for-earth - NASA’s Solar Dynamics Observatory
https://sdo.gsfc.nasa.gov - NOAA Weather and Climate Tools
https://www.noaa.gov/tools-and-resources - Tomorrow.io AI Forecasting Platform
https://www.tomorrow.io/weather-api