How AI is Revolutionizing Healthcare: Overcoming Intellectual Bottlenecks and Shaping the Future
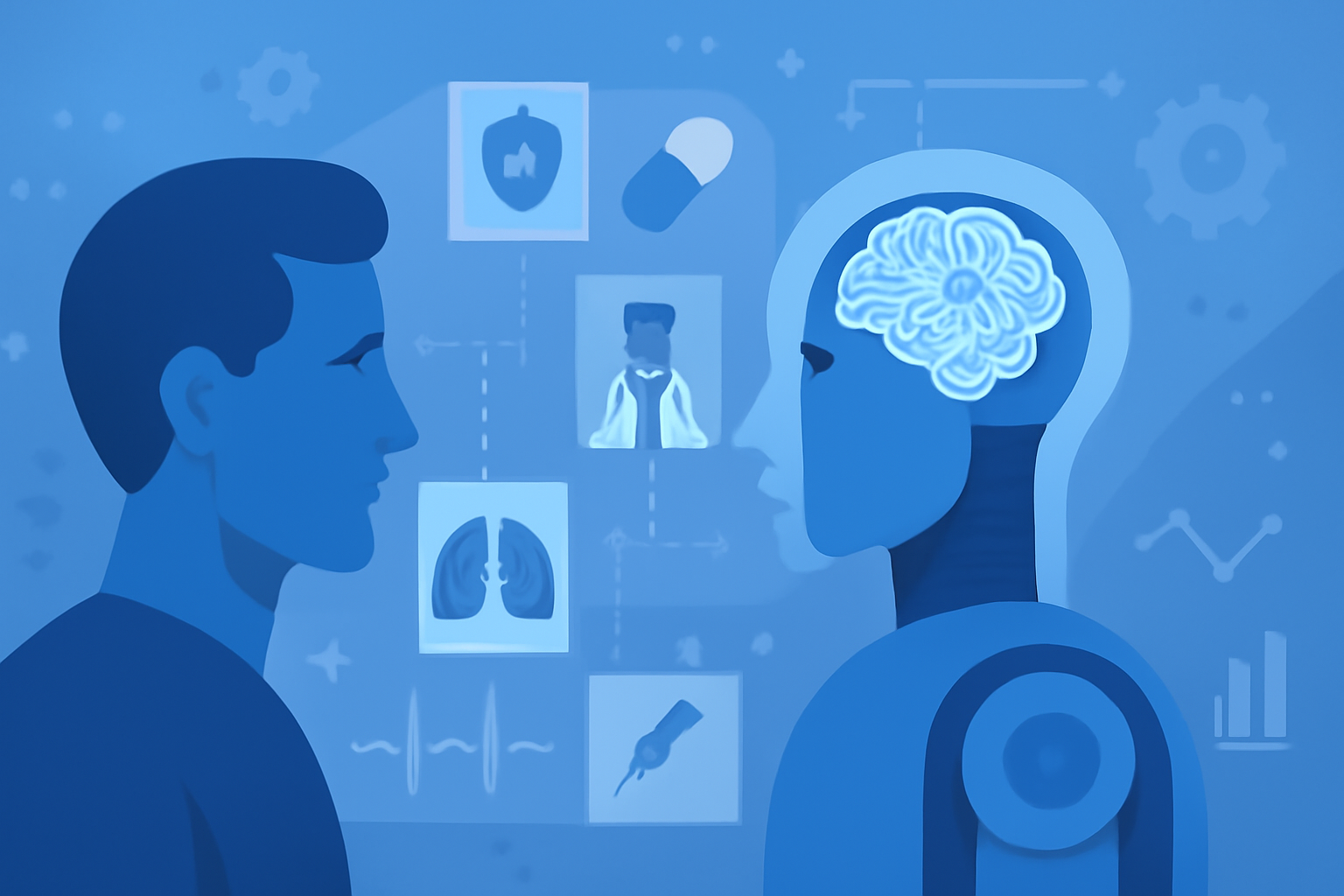
The healthcare industry stands at the intersection of advanced technological innovation and the complex challenges posed by human decision-making. Within this dynamic environment, one of the most pressing issues today is the intellectual bottleneck— a concept that refers to the cognitive and operational constraints limiting the ability of healthcare professionals to process and act on the vast amount of data available to them. These bottlenecks are not just a matter of limited human capacity; they are amplified by outdated systems, fragmented data, and the overwhelming volume of information that must be managed in real-time.
Healthcare professionals are tasked with making critical decisions based on intricate data—whether it's diagnosing a rare disease, choosing the most effective treatment plan, or interpreting the results of a new clinical trial. However, human minds have limits in terms of the volume of information they can process and how quickly they can assimilate new, often contradictory, data. The complexity of modern healthcare systems, which are becoming more interconnected but also more fragmented, exacerbates this problem. Doctors, nurses, and other healthcare providers often face cognitive overload as they try to sift through reams of electronic health records (EHRs), lab results, and medical literature while still providing timely, patient-centered care.
This intellectual bottleneck is a key barrier to improving healthcare delivery. It slows down decision-making, introduces opportunities for human error, and ultimately limits the quality of care that can be provided. In a world where precision, speed, and personalization are increasingly demanded in healthcare, these bottlenecks contribute to inefficiency, errors, and suboptimal outcomes. What is needed is a systemic shift in the way healthcare data is utilized, processed, and acted upon.
Artificial intelligence (AI), particularly in the form of machine learning (ML) and natural language processing (NLP), is emerging as a powerful tool to address these challenges. By leveraging AI, healthcare providers can gain faster insights, enhance decision-making accuracy, and reduce the intellectual burden placed on human practitioners. AI technologies are already making a tangible impact in various areas such as diagnostics, personalized treatment plans, and drug discovery. They are designed to process vast datasets at speeds and accuracy levels that far exceed human capability, thus reducing cognitive overload and enabling healthcare professionals to focus on the critical, high-level decisions that require human judgment and empathy.
This blog post delves into how AI is poised to alleviate the intellectual bottleneck in healthcare. We will explore the key applications of AI in healthcare, examine how these technologies help mitigate the limitations of human cognition, and highlight the challenges that still exist in the widespread adoption of AI. Finally, we will discuss the potential future of AI in healthcare, considering the ways in which it could reshape the intellectual landscape of the industry.
The Role of AI in Healthcare: Current Applications
Artificial Intelligence (AI) has rapidly transitioned from an emerging technology to a transformative force within various industries, with healthcare being one of the most significant sectors to benefit from its capabilities. At its core, AI in healthcare aims to leverage computational power to process and interpret vast quantities of data, offering insights that would be impractical or impossible for human professionals to achieve in the same timeframe. The integration of AI technologies—such as machine learning (ML), natural language processing (NLP), and deep learning—has proven to be an invaluable tool in tackling many of the intellectual bottlenecks that hinder medical professionals from making informed decisions efficiently.
AI in Diagnostics: Enhancing Precision and Speed
One of the most significant applications of AI in healthcare lies in its ability to assist in medical diagnostics. Traditional diagnostic procedures are often time-consuming, subject to human error, and limited by the availability of relevant data. AI systems, however, can analyze complex medical images (such as CT scans, MRIs, and X-rays) with a high degree of accuracy. These systems can identify patterns, anomalies, and subtle markers that might go unnoticed by even the most experienced radiologists.
For example, Google's DeepMind developed an AI system capable of diagnosing eye diseases by analyzing retinal scans. This system has shown comparable, if not superior, accuracy to human experts in identifying diabetic retinopathy and macular degeneration. Such advancements highlight AI's potential to assist healthcare professionals by providing them with a faster, more accurate assessment of a patient’s condition.
In pathology, AI is also making inroads by assisting pathologists in detecting cancerous cells in tissue samples. Systems like PathAI use deep learning to assess pathology slides, significantly improving diagnostic accuracy and efficiency. By automating the detection of abnormalities in tissue samples, AI can help reduce the time it takes to diagnose diseases, allowing for quicker intervention and better patient outcomes.
AI in Personalized Medicine: Tailoring Treatments to Individual Needs
Personalized medicine is another area where AI plays a crucial role in addressing intellectual bottlenecks. Traditional treatment methods often take a one-size-fits-all approach, relying on generalized protocols and limited patient data. However, AI enables the development of personalized treatment plans by analyzing a patient's genetic makeup, lifestyle data, and environmental factors.
For instance, AI-powered platforms like IBM Watson Health use vast amounts of medical literature, clinical trial data, and patient records to recommend personalized treatment plans. These AI systems can sift through a multitude of clinical studies, pinpointing the best therapies for individual patients based on their unique genetic and clinical profiles. This is particularly evident in the treatment of cancer, where AI has been used to predict how a patient’s tumors might respond to specific drugs, ultimately leading to more effective and individualized treatment regimens.
AI is also utilized in pharmacogenomics, where it helps identify how genetic variations influence a patient’s response to certain drugs. By incorporating AI into this process, doctors can avoid prescribing medications that may not be effective for specific genetic profiles, thereby reducing adverse drug reactions and enhancing therapeutic efficacy.
AI in Predictive Analytics: Anticipating Health Issues Before They Arise
Beyond immediate diagnostics, AI’s role in predictive analytics offers immense potential for proactive healthcare. Predictive models powered by AI can analyze historical medical data and detect patterns that suggest an increased risk of certain conditions, such as heart disease, stroke, or diabetes. These systems use machine learning algorithms to track risk factors, monitor patient data in real-time, and predict future health events, enabling healthcare professionals to intervene before serious issues arise.
For example, AI systems are being used in managing chronic diseases, such as diabetes, by continuously monitoring patient glucose levels and alerting patients and physicians when immediate intervention is needed. This predictive capability empowers patients to make adjustments to their lifestyle or medications, thus preventing complications and enhancing long-term health outcomes.
In hospitals, AI-driven predictive analytics tools are used to forecast patient deterioration, helping medical teams prioritize care for patients most at risk. By assessing a wide range of variables—vital signs, lab results, and even medical history—AI systems can predict which patients are likely to experience complications, ensuring timely and targeted interventions. This predictive capability reduces the intellectual burden on healthcare providers, allowing them to focus on the most critical cases and improving patient safety.
AI in Drug Discovery: Accelerating Research and Development
The process of drug discovery is notoriously lengthy and expensive. On average, developing a new drug can take over a decade and cost billions of dollars, with many potential drug candidates failing during clinical trials. AI has shown significant promise in accelerating this process, offering more efficient ways to identify viable drug candidates and predict their efficacy before moving into costly clinical trials.
AI algorithms can analyze massive datasets, including chemical compounds, genetic information, and clinical trial results, to identify patterns that may indicate promising drug candidates. For example, Insilico Medicine has developed AI models that can predict how compounds will interact with biological systems, enabling researchers to identify promising drug candidates more quickly. By reducing the time required for preclinical drug development, AI helps to lower costs and accelerate the time it takes to bring new therapies to market.
AI's role in drug repurposing is also noteworthy. AI systems can analyze existing drugs for potential new uses, helping to identify treatments for diseases that may not have been originally considered. During the COVID-19 pandemic, AI-powered platforms like BenevolentAI were used to identify potential treatments for the virus by analyzing existing drugs for their antiviral properties, ultimately speeding up the discovery of possible therapeutic options.
AI in Administrative Tasks: Reducing Cognitive Load and Improving Efficiency
In addition to clinical applications, AI is also being deployed in administrative areas of healthcare to reduce cognitive load and improve overall efficiency. Healthcare professionals often spend a significant amount of time on administrative tasks, such as managing patient records, billing, and scheduling. AI systems, particularly those powered by natural language processing (NLP), can help automate these time-consuming processes, freeing up valuable time for clinicians to focus on patient care.
For instance, AI-powered chatbots and virtual assistants are being used in hospitals and clinics to handle routine administrative inquiries, such as appointment scheduling and insurance verification. These systems can manage vast amounts of patient data, generate reports, and assist in billing, all while reducing human error and ensuring compliance with healthcare regulations. By automating these administrative tasks, AI reduces the intellectual burden on healthcare workers, allowing them to allocate more time and energy to clinical responsibilities.
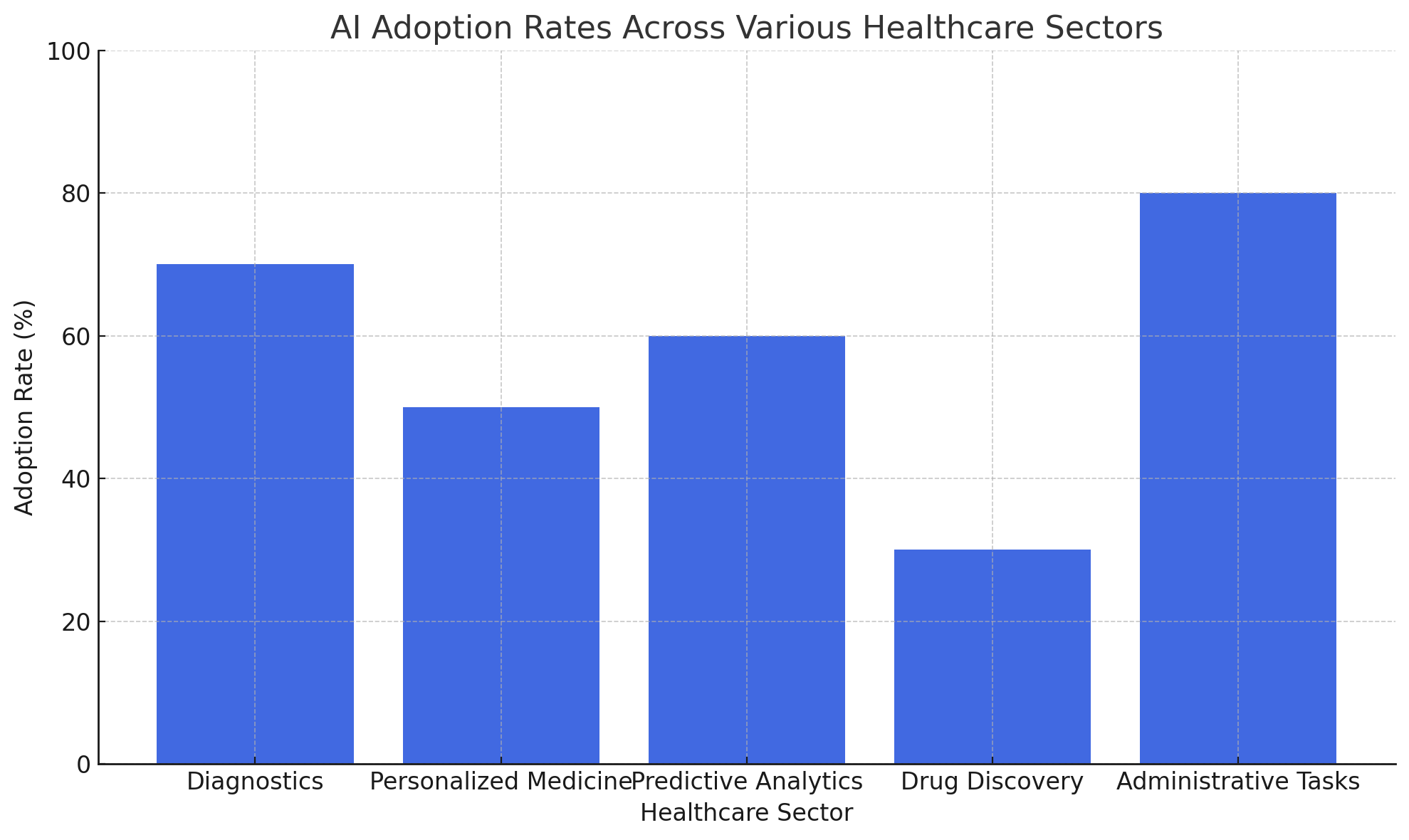
In summary, AI’s role in healthcare is multifaceted, with applications spanning diagnostics, personalized medicine, predictive analytics, drug discovery, and administrative tasks. These technologies not only help alleviate the intellectual bottleneck by processing and analyzing large datasets but also enable healthcare professionals to focus on higher-level decision-making and patient care. As AI continues to evolve, its integration into the healthcare system promises to enhance both the efficiency and quality of care, addressing some of the most pressing challenges in modern medicine.
The Intellectual Bottleneck: How AI Can Help Alleviate It
In healthcare, intellectual bottlenecks emerge when the human brain is overwhelmed by the sheer volume and complexity of data that needs to be processed. This cognitive overload is a direct consequence of the modern healthcare environment, which continuously generates massive amounts of medical data from multiple sources: electronic health records (EHRs), diagnostic imaging, laboratory results, patient histories, and real-time monitoring systems. Although these data points are essential for informed decision-making, their sheer volume can impede a healthcare provider’s ability to make quick, accurate, and well-informed judgments.
The intellectual bottleneck is evident at various levels of healthcare. For instance, in clinical decision-making, healthcare professionals must constantly evaluate large amounts of information from diverse sources. They need to assess lab results, compare symptoms, review imaging scans, and consider patient histories—often all at once. This task becomes even more challenging in the context of complex and rare diseases, where traditional methods of diagnosis and treatment may not suffice. Furthermore, the fragmented nature of healthcare data and systems only adds to the complexity, making it more difficult to obtain a holistic view of a patient’s health status.
The implications of this bottleneck are far-reaching. It slows down diagnostic processes, increases the likelihood of human error, and, in the worst cases, can result in delayed treatments or misdiagnoses, ultimately impacting patient outcomes. Healthcare professionals, already under immense pressure due to high workloads, are further burdened by these intellectual challenges, which can lead to burnout, inefficiency, and frustration.
AI has the potential to significantly reduce this cognitive burden and alleviate the intellectual bottleneck by offering technologies capable of processing and analyzing large amounts of data faster and more accurately than humans can. Through advanced algorithms and machine learning models, AI can help healthcare providers make better, data-driven decisions, ultimately enhancing the quality of care and improving patient outcomes.
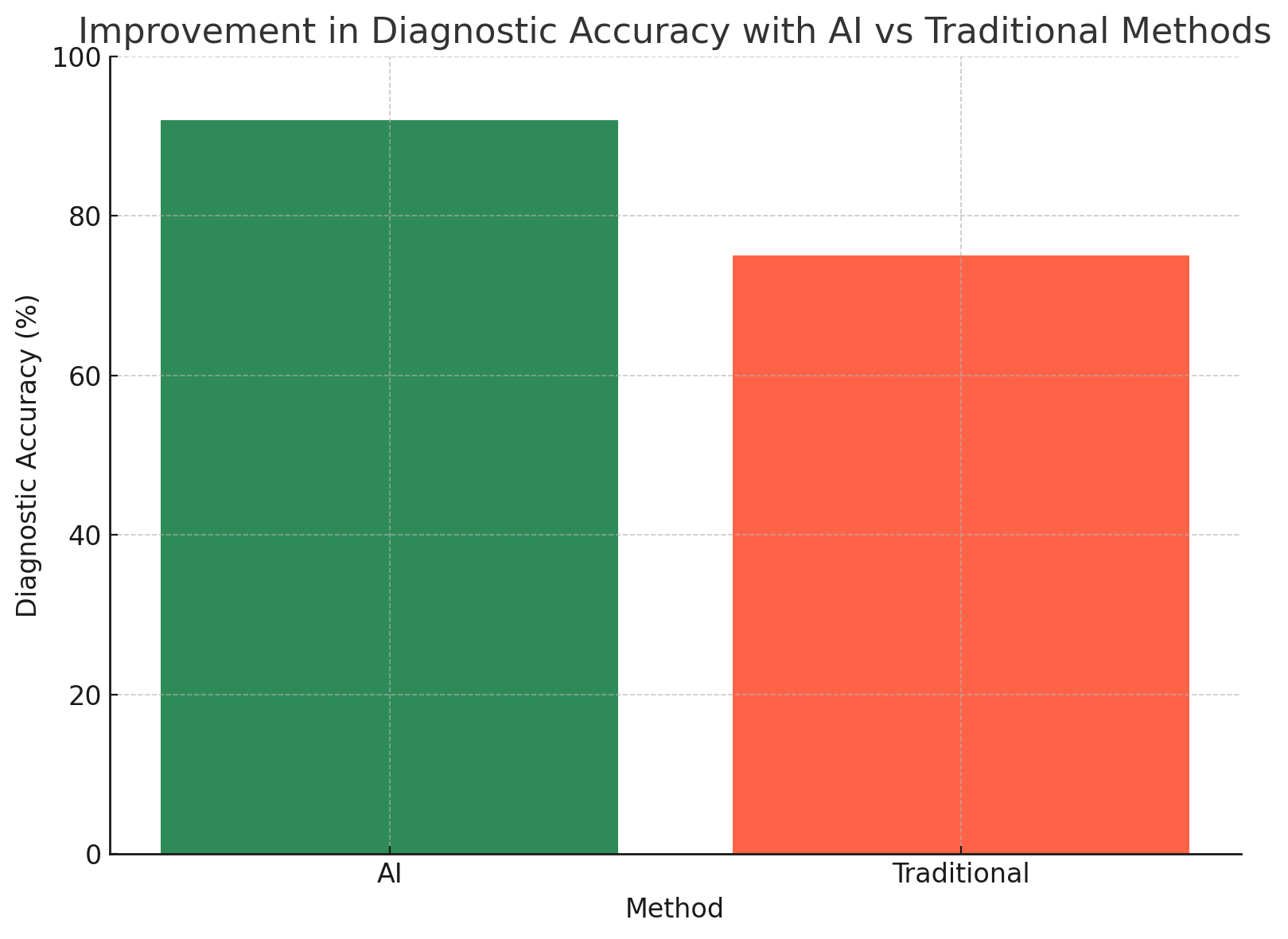
Reducing Cognitive Load with Data Processing and Interpretation
The first major way in which AI addresses the intellectual bottleneck in healthcare is by automating the processing and interpretation of complex medical data. Medical professionals are tasked with interpreting an overwhelming amount of information, which often includes unstructured data—such as free-text notes from doctors, test results, and medical images—that can be difficult to analyze manually. AI can help by using advanced natural language processing (NLP) models and image recognition technologies to automatically analyze this unstructured data.
AI-powered NLP tools are capable of scanning medical records, patient histories, and clinical notes, extracting relevant information, and presenting it in a structured format. For example, AI can parse a physician's handwritten notes or unstructured clinical text, pulling out key medical terms, symptoms, diagnoses, and treatment options. This organized data can then be integrated with other structured data (such as lab results and imaging scans), allowing healthcare providers to access comprehensive and actionable insights with ease. By taking over the routine and time-consuming task of data extraction, AI reduces the cognitive load on clinicians, enabling them to focus on more critical aspects of patient care.
In diagnostic imaging, AI systems can analyze X-rays, MRIs, and CT scans with incredible speed and precision. Rather than requiring a human radiologist to scrutinize hundreds of images to identify subtle signs of disease, AI algorithms can scan these images in seconds, highlighting areas of concern and offering diagnostic suggestions. This reduces the time required for image analysis and increases the likelihood of identifying conditions that may be overlooked by human practitioners. Furthermore, AI’s ability to cross-reference images with vast medical databases and clinical guidelines ensures that the information provided is both accurate and up-to-date.
Augmenting Clinical Decision-Making with AI-Driven Insights
Beyond data processing, AI plays a critical role in augmenting clinical decision-making. Healthcare professionals must often make complex decisions based on incomplete, conflicting, or ambiguous information. AI-driven decision support systems can assist by offering evidence-based recommendations, taking into account the latest research, clinical guidelines, and individual patient data.
For example, AI-powered clinical decision support systems (CDSS) can analyze a patient's medical history, lab results, and demographic information to recommend personalized treatment options. These systems can assess vast datasets in real-time, integrating insights from multiple sources and highlighting the most relevant information for a particular clinical scenario. This can include suggesting the best course of action for managing a patient’s chronic disease, recommending preventive measures, or proposing treatment alternatives based on a patient’s genetic makeup and medical history. By streamlining this decision-making process, AI helps clinicians make informed choices quickly, reducing the mental effort required to sift through large amounts of data.
Moreover, AI models can assist in identifying potential drug interactions, which is especially important when prescribing medications for patients with complex comorbidities. For instance, an AI system might flag a dangerous drug interaction that a clinician may have overlooked due to the complexity of the patient’s health profile. By assisting in this way, AI not only prevents cognitive overload but also reduces the chances of costly errors that could harm patients.
Predictive Analytics: Empowering Proactive Care
AI’s ability to predict future health events is another powerful way it helps alleviate intellectual bottlenecks in healthcare. Predictive analytics, powered by machine learning algorithms, can analyze a patient’s historical health data, genetic information, and lifestyle factors to forecast potential health risks. These predictive models can offer healthcare professionals the foresight they need to take proactive measures before health issues become critical.
For example, AI-driven models can be used to predict which patients are at risk of developing chronic conditions like heart disease or diabetes. By analyzing data such as blood pressure, cholesterol levels, and family medical histories, AI can predict the likelihood of a patient experiencing a cardiovascular event, allowing healthcare providers to intervene early with preventive measures. This proactive approach not only improves patient outcomes but also helps manage healthcare resources more effectively by preventing hospitalizations and expensive emergency interventions.
In addition to individual patient care, predictive models can also help healthcare systems forecast trends in population health. For example, AI can analyze data across large patient populations to predict the spread of infectious diseases, the demand for hospital beds, or the likelihood of an outbreak. These insights allow healthcare systems to prepare for potential public health crises in advance, ensuring they have the necessary resources and personnel in place to manage an influx of patients.
Supporting Research and Drug Development
AI's role in alleviating intellectual bottlenecks extends beyond direct patient care to drug discovery and medical research. The process of developing new treatments is a highly complex and resource-intensive endeavor. AI can accelerate the identification of promising drug candidates by analyzing vast amounts of biological data, such as genomic information, chemical compounds, and clinical trial outcomes.
For example, AI-driven platforms like Atomwise use machine learning algorithms to predict how different molecules will interact with specific biological targets, allowing researchers to identify potential drug candidates more efficiently. By automating this process, AI reduces the time required to test and validate compounds, significantly speeding up the drug discovery process and reducing costs. This is particularly important in the context of rare diseases or emerging threats like pandemics, where rapid drug development is critical.
Furthermore, AI can aid researchers in analyzing clinical trial data, identifying trends, and suggesting improvements to trial design. By quickly identifying which patient populations are most likely to benefit from certain treatments, AI can help streamline the clinical trial process, making it more efficient and cost-effective.
AI as a Key Enabler of Healthcare Innovation
The intellectual bottleneck in healthcare is a significant barrier to achieving optimal patient outcomes, efficient workflows, and innovative advancements in medical science. However, AI offers a promising solution by helping to alleviate the cognitive and operational burdens on healthcare professionals. From automating data processing and enhancing decision-making to providing predictive insights and accelerating drug discovery, AI enables healthcare providers to focus on the most important aspects of care while reducing errors and inefficiencies.
As AI technologies continue to evolve and become more integrated into healthcare systems, they will play an increasingly vital role in transforming how medical professionals approach diagnosis, treatment, and patient management. By alleviating the intellectual bottleneck, AI has the potential to revolutionize healthcare, improving both the quality and efficiency of care on a global scale.
Challenges to AI Adoption in Healthcare
While the potential benefits of artificial intelligence (AI) in healthcare are immense, the widespread adoption of AI technologies faces numerous challenges. These obstacles stem from a variety of sources, including regulatory hurdles, concerns over data privacy and security, the need for workforce adaptation, and the integration of AI systems into existing healthcare infrastructures. Despite the growing recognition of AI's transformative potential, these barriers must be addressed before AI can fully realize its promise in improving healthcare delivery.
Regulatory and Ethical Concerns
One of the most significant challenges in adopting AI in healthcare is navigating the complex regulatory landscape. Healthcare is one of the most highly regulated industries in the world, and the introduction of AI technologies introduces a new set of concerns regarding safety, efficacy, and accountability. Regulatory bodies such as the U.S. Food and Drug Administration (FDA), the European Medicines Agency (EMA), and other global agencies are tasked with ensuring that new healthcare technologies meet rigorous standards before they can be used in clinical practice.
Currently, many AI tools are still in the experimental or clinical trial phases, making it difficult for regulatory bodies to assess their long-term impacts. AI systems, particularly those driven by machine learning, can operate in ways that are not fully transparent, making it challenging to predict their behavior in all scenarios. This lack of transparency, often referred to as the "black box" problem, raises concerns about the ability to audit and validate AI systems effectively.
Additionally, the evolving nature of AI means that these systems can change and adapt over time, which further complicates regulatory oversight. Unlike traditional medical devices, which have a more static functionality, AI systems may evolve in response to new data, potentially altering their decision-making processes. This ongoing evolution creates difficulties for regulators, who must ensure that AI technologies remain safe and effective throughout their lifecycle.
Ethical concerns also play a significant role in AI adoption in healthcare. AI systems, if not properly designed and monitored, can perpetuate biases present in the data they are trained on. For instance, if an AI system is trained on biased healthcare data that underrepresents certain demographic groups, it may produce suboptimal or discriminatory recommendations for those populations. Ensuring that AI systems are trained on diverse and representative datasets, and that they are transparent and accountable in their decision-making, is essential to avoid ethical pitfalls.
Data Privacy and Security
Data privacy and security concerns are perhaps the most prominent barriers to the widespread adoption of AI in healthcare. Healthcare data is extremely sensitive, and patient privacy is of paramount importance. The widespread use of AI systems requires access to large amounts of patient data, including electronic health records (EHRs), diagnostic images, lab results, and personal health information. However, sharing such data raises significant concerns about privacy violations, cyberattacks, and data breaches.
Many healthcare organizations and patients remain hesitant to allow AI systems access to sensitive health data due to the fear of unauthorized access or misuse. The Health Insurance Portability and Accountability Act (HIPAA) in the United States, the General Data Protection Regulation (GDPR) in the European Union, and other regional regulations are designed to protect patient data, but these laws are often seen as cumbersome and insufficient to fully address the challenges posed by AI in healthcare. For example, while these regulations govern the handling of data, they may not be equipped to manage the complexities of AI systems that require access to vast amounts of real-time data.
Furthermore, as healthcare data becomes more interconnected—through the rise of health information exchanges, interoperable systems, and wearable health devices—new vulnerabilities emerge. AI systems must be able to protect data across multiple platforms and ensure that patient information remains secure as it is processed, shared, and analyzed. This requires robust cybersecurity protocols and compliance with stringent data protection standards. Without these protections in place, patients may be unwilling to trust AI systems with their data, thus hindering adoption.
Resistance from Healthcare Professionals
The adoption of AI in healthcare also faces significant resistance from healthcare professionals. Clinicians and healthcare workers are often wary of AI because of concerns that it may replace their roles, diminish their autonomy, or introduce new complexities into an already overburdened healthcare system. There is a fear that AI-driven systems may undermine the doctor-patient relationship by reducing the human element in care, or that they will make clinical decisions based solely on data, potentially overlooking the nuanced, individualized aspects of patient care.
Moreover, many healthcare professionals, particularly those who have worked with traditional systems for years, may find it difficult to adapt to new technologies. Training and upskilling are critical components of AI adoption, but the integration of AI into clinical workflows requires significant changes to how healthcare professionals operate. For instance, healthcare workers may need to learn to interpret AI-generated recommendations and understand the underlying models behind those suggestions. This shift requires time, resources, and a commitment to continuous education, which many healthcare institutions may be ill-prepared to provide.
Additionally, healthcare professionals may feel a lack of trust in AI systems, especially if they do not fully understand how these systems work. Trust in AI is essential for its successful implementation, and if healthcare workers do not feel confident in the reliability and accuracy of AI tools, they may be reluctant to incorporate them into their practices. Overcoming this resistance requires not only training and education but also fostering a culture of collaboration between AI systems and healthcare providers, ensuring that AI is seen as an augmentation to human decision-making rather than a replacement.
Integration with Existing Healthcare Infrastructure
Another significant barrier to AI adoption in healthcare is the challenge of integrating AI systems with existing healthcare infrastructure. Many healthcare organizations rely on legacy systems that are outdated and incompatible with modern AI technologies. These systems may not be equipped to handle the data processing requirements of AI or may lack the necessary interoperability to communicate with newer AI tools.
For instance, electronic health record (EHR) systems, which are the backbone of many healthcare organizations, were not initially designed with AI in mind. As a result, integrating AI technologies with EHRs can be a complex and resource-intensive process. AI systems may need to be customized to work with specific EHR platforms, which can create additional costs and delays in implementation. Moreover, ensuring that these systems communicate seamlessly across different departments, organizations, and even countries adds another layer of complexity.
Healthcare systems must also invest in upgrading their technological infrastructure to support AI. This may involve enhancing data storage capacity, improving computational power, and ensuring that networks are robust enough to handle the increased demands of AI applications. However, the financial and logistical challenges associated with these upgrades can be a significant barrier, particularly for smaller healthcare providers or those operating in resource-constrained settings.
Cost Considerations and Resource Allocation
Finally, the cost of implementing AI technologies in healthcare can be a significant hurdle. Developing, testing, and deploying AI systems requires substantial financial investments in both technology and expertise. For instance, healthcare organizations must budget for the acquisition of hardware, software, and AI models, as well as the personnel required to develop, maintain, and monitor these systems. Smaller healthcare institutions, particularly in developing regions, may find these costs prohibitive.
Moreover, healthcare systems must allocate resources to training staff, integrating AI into clinical workflows, and ensuring that the necessary infrastructure is in place. These investments can take years to yield a return, which may deter healthcare organizations from adopting AI, especially if they face financial constraints or are uncertain about the long-term benefits of the technology.
In conclusion, while AI offers tremendous potential to improve healthcare delivery and alleviate intellectual bottlenecks, its widespread adoption faces numerous challenges. Regulatory concerns, data privacy issues, resistance from healthcare professionals, integration difficulties, and the costs associated with implementation must all be addressed before AI can fully transform the healthcare sector. Overcoming these barriers will require collaboration between regulators, healthcare providers, technology developers, and policymakers to create an environment where AI can thrive, benefiting both patients and healthcare professionals alike.
The Future: Transforming Healthcare with AI
The integration of artificial intelligence (AI) into healthcare is no longer a distant vision; it is an ongoing revolution that promises to reshape how healthcare is delivered, managed, and experienced. While the challenges of AI adoption in healthcare remain, the future looks promising, with continuous advancements in AI technologies offering new opportunities to enhance care, streamline processes, and improve patient outcomes. The potential for AI to transform healthcare lies not only in its ability to address existing intellectual bottlenecks but also in its capacity to push the boundaries of medical science and practice to unprecedented levels of efficiency and precision.
AI as a Catalyst for Precision Medicine
One of the most exciting possibilities for AI in the future of healthcare is its role in advancing precision medicine. Traditionally, medical treatments have followed a one-size-fits-all approach, with treatment protocols based on generalizations about disease progression and patient demographics. However, AI is making it possible to tailor treatments to individual patients based on their genetic makeup, lifestyle, and environmental factors. This approach—often referred to as personalized or precision medicine—offers the potential for significantly more effective treatments with fewer side effects.
AI models, especially those incorporating machine learning and deep learning techniques, can analyze vast datasets from a variety of sources, including genomic data, clinical records, and even wearable health devices. By identifying patterns and correlations within these datasets, AI systems can predict how individual patients will respond to different treatments, allowing healthcare providers to choose the most effective options tailored to each patient’s unique profile. This could be particularly transformative in areas like cancer treatment, where AI could help identify the most promising therapies based on a patient’s specific genetic mutations.
Furthermore, AI has the potential to revolutionize early detection and diagnosis by identifying biomarkers for diseases that were previously undetectable or difficult to diagnose at an early stage. This shift toward early detection, coupled with personalized treatment options, could dramatically improve survival rates, reduce treatment costs, and improve the overall quality of life for patients.
The Role of AI in Preventive Healthcare
In addition to its impact on personalized treatment, AI is poised to play a critical role in preventive healthcare. As healthcare systems worldwide continue to face rising costs and overwhelmed infrastructure, preventive care offers a pathway to reduce the burden on healthcare providers and improve long-term population health. AI can help shift the focus of healthcare from treating illness to preventing it by identifying risks and providing actionable insights before diseases manifest.
Predictive analytics powered by AI can analyze a patient’s health data, lifestyle choices, and genetic information to identify early warning signs of chronic conditions such as cardiovascular disease, diabetes, and cancer. For example, AI systems can track patterns in a patient’s behavior, such as physical activity, diet, and sleep, and use this data to predict the likelihood of future health problems. By doing so, healthcare providers can intervene early, offering lifestyle modifications, preventive medications, and regular monitoring to prevent the onset of disease.
The future of AI in preventive healthcare extends beyond individual patients to population-level health management. AI-driven epidemiological models can predict the spread of infectious diseases, track vaccination rates, and anticipate future outbreaks. These predictive tools are critical in managing public health crises, such as pandemics, by enabling timely interventions and better resource allocation. As healthcare data becomes more interconnected across regions and institutions, AI will play an increasingly vital role in shaping global health initiatives and preventing widespread disease.
AI-Powered Medical Research and Drug Discovery
The future of AI in healthcare is also deeply intertwined with advancements in medical research and drug discovery. The traditional drug discovery process is notoriously slow, expensive, and fraught with inefficiencies. On average, it takes more than a decade and billions of dollars to bring a new drug to market. However, AI’s ability to analyze vast datasets and identify patterns that might elude human researchers offers the potential to significantly accelerate this process.
In drug discovery, AI models can analyze millions of compounds to identify candidates that have the highest likelihood of being effective against specific diseases. For example, AI systems can screen chemical databases to predict how molecules will interact with biological targets, significantly reducing the time required for preclinical testing. AI is already being used to identify potential drug candidates for complex conditions like Alzheimer’s disease, where traditional approaches have struggled to yield breakthroughs. AI models can also optimize the design of clinical trials, ensuring that the right patient populations are selected and that the trials are structured to maximize their chances of success.
Moreover, AI can assist in the repurposing of existing drugs for new therapeutic applications. This was particularly evident during the COVID-19 pandemic when AI platforms were used to rapidly identify existing medications that might have potential efficacy against the virus. Such capabilities not only shorten the drug discovery timeline but also lower the costs associated with developing new treatments, ultimately bringing more affordable medications to market faster.
As AI continues to evolve, its role in medical research and drug discovery will only expand, leading to faster development of therapies and cures for previously untreatable or poorly understood diseases.
AI and the Digital Transformation of Healthcare Delivery
The digital transformation of healthcare is already underway, and AI will be at the forefront of this evolution. As the healthcare sector increasingly shifts toward digital systems—such as electronic health records (EHRs), telemedicine, and remote patient monitoring—AI will serve as the driving force behind the integration and optimization of these technologies.
Telemedicine, in particular, stands to benefit immensely from AI, particularly in improving diagnostic capabilities, remote patient monitoring, and treatment recommendations. AI-powered virtual assistants and chatbots can provide patients with immediate access to medical advice, reducing the strain on healthcare providers and ensuring that patients receive timely guidance for non-emergency situations. AI systems can also analyze data from wearable devices to monitor patients remotely, flagging potential health issues before they require emergency intervention. This shift toward digital health platforms will allow healthcare providers to reach more patients, enhance care delivery, and improve patient engagement.
In the long term, AI’s ability to analyze and integrate data from various healthcare sources—including hospitals, private practices, wearable devices, and even public health records—will enable the creation of comprehensive, real-time health profiles for individuals. This will allow healthcare providers to offer more personalized, data-driven care that is not confined to a single location or provider but is continuously informed by a broader ecosystem of health information.
Overcoming Barriers and Embracing AI’s Full Potential
While the future of AI in healthcare holds immense promise, realizing this potential requires addressing the existing barriers to adoption. Regulatory, ethical, and data privacy concerns must be proactively managed, ensuring that AI systems are safe, transparent, and equitable. Additionally, healthcare providers must invest in training and education to ensure that clinicians can effectively integrate AI tools into their workflows without undermining the human element of care.
The next phase of AI in healthcare will require collaboration among healthcare providers, technology developers, regulators, and policymakers to create an environment that supports AI innovation while safeguarding patient well-being. It will also require the ongoing development of AI models that are both more accurate and more explainable, so that healthcare professionals can trust and rely on AI-driven insights in their decision-making.
As AI continues to evolve, it will become an indispensable tool for transforming healthcare into a more personalized, efficient, and accessible system. By addressing intellectual bottlenecks, improving patient care, and accelerating medical advancements, AI has the potential to reshape healthcare as we know it—ushering in a new era of precision, prevention, and proactive health management.
References:
- "AI in Healthcare: A Comprehensive Guide," HealthIT.gov, https://www.healthit.gov/
- "The Role of Artificial Intelligence in Healthcare," Mayo Clinic, https://www.mayoclinic.org/
- "Machine Learning for Healthcare Applications," IBM Watson Health, https://www.ibm.com/watson-health
- "How AI is Transforming Healthcare," World Health Organization, https://www.who.int/
- "AI-Powered Diagnostics: The Future of Healthcare," Google Health, https://health.google/
- "AI and the Future of Medicine," Harvard Medical School, https://hms.harvard.edu/
- "The Benefits of AI in Healthcare," Forbes, https://www.forbes.com/
- "Artificial Intelligence in Drug Discovery," Nature, https://www.nature.com/
- "The Impact of AI on Healthcare Systems," McKinsey & Company, https://www.mckinsey.com/
- "AI in Healthcare: Challenges and Opportunities," National Institutes of Health, https://www.nih.gov/