How AI Is Reshaping Academic Integrity: Detection Tools, Strategies, and the Future of Education
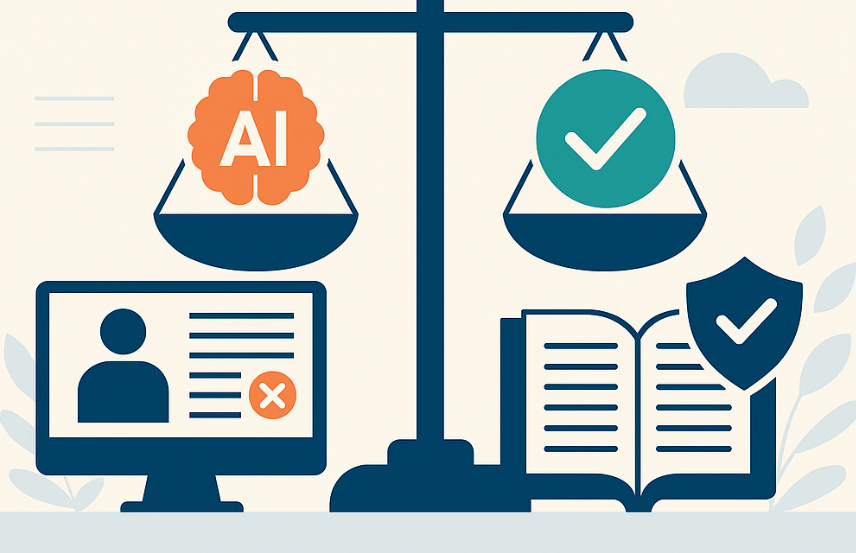
The proliferation of generative artificial intelligence (AI) tools has marked a profound shift in the landscape of education. From AI-powered writing assistants to advanced coding generators and language models capable of composing full essays, students and educators alike are navigating an increasingly complex technological environment. While these tools offer numerous benefits—ranging from enhanced productivity to personalized learning experiences—they simultaneously pose significant challenges to long-standing academic values. Chief among these is the principle of academic integrity, a cornerstone of educational excellence and scholarly trust.
Academic integrity traditionally encompasses the adherence to ethical standards such as honesty, fairness, responsibility, and respect for intellectual property. It is the foundation upon which scholarly inquiry is built and upon which degrees and qualifications are deemed credible. However, the integration of AI into the learning process has created a new dimension in which these ethical principles must be re-examined and reinforced. The ability of AI models to generate human-like text, solve complex problems, and simulate understanding raises questions about originality, authorship, and the evaluation of student performance.
The challenges are multifaceted. Students may be tempted to use AI tools to complete assignments with minimal effort, potentially bypassing the learning process entirely. Educators, in turn, face increasing difficulty in distinguishing between human and machine-generated work. Moreover, existing academic policies and honor codes, many of which were established before the advent of generative AI, are often ill-equipped to address the nuances of these emerging technologies. This disconnect creates a pressing need for institutions to update their frameworks and for all stakeholders to adopt more nuanced strategies.
Nonetheless, AI should not be viewed solely as a threat to academic integrity. When harnessed appropriately, these tools can support learning by providing scaffolding, enabling deeper engagement with course materials, and promoting critical thinking. The key lies in delineating ethical boundaries and creating an educational environment in which the responsible use of AI is encouraged, while misconduct is effectively deterred.
This blog post explores the evolving relationship between AI and academic integrity through four dimensions: the impact of AI on academic conduct, the tools available to detect AI-assisted dishonesty, the strategies institutions and educators can implement to uphold ethical standards, and the future of academic integrity in an AI-pervasive academic world. By examining both the risks and opportunities presented by AI, this analysis aims to provide a balanced and practical framework for maintaining academic honesty in an age of intelligent machines.
The Impact of Generative AI on Academic Integrity
The rapid adoption of generative artificial intelligence (AI) technologies across academic institutions has ushered in a paradigm shift in how students learn, engage with content, and complete assessments. Generative AI tools, most notably large language models such as OpenAI’s ChatGPT, Google’s Gemini, and Anthropic’s Claude, have democratized access to high-level content generation, making it possible for users to produce coherent essays, answer complex questions, and simulate human reasoning with minimal effort. While these tools promise considerable educational value when used ethically, they simultaneously challenge the core tenets of academic integrity by blurring the line between human cognition and machine assistance.
The Changing Landscape of Student Engagement
Traditionally, academic performance has been evaluated through mechanisms that require original thought, critical reasoning, and written articulation. These methods presuppose that the work submitted is the product of a student’s unaided intellectual effort. However, generative AI systems have introduced a new layer of complexity. By offering real-time responses to academic prompts and assisting in the generation of entire assignments, these tools may inadvertently encourage passive learning. Students may be tempted to rely on these systems not as supplementary aids but as substitutes for their own cognitive work.
A 2024 survey conducted by the International Center for Academic Standards (ICAS) revealed that approximately 42% of undergraduate students across the United States admitted to using AI tools to complete parts of their coursework, while 18% acknowledged submitting AI-generated content without any modifications. The motivations behind this behavior range from time constraints and academic pressure to a lack of understanding about what constitutes academic dishonesty in the context of AI. Such findings indicate that students are not necessarily acting with malicious intent, but rather navigating an unclear ethical terrain shaped by rapidly evolving technologies.
Generative AI as a Double-Edged Sword
Despite its risks, generative AI is not inherently antithetical to academic integrity. When integrated thoughtfully, it can serve as a powerful educational tool. For instance, AI writing assistants can help students draft outlines, identify grammatical errors, or explore new perspectives on a topic—functions that enhance rather than diminish the learning process. The distinction, however, lies in the manner of usage. Using AI to stimulate thinking and develop arguments aligns with educational goals, while deploying it to generate final answers or evade effort constitutes misconduct.
Educators are increasingly aware of this dichotomy. Yet institutional policies often lag behind, failing to account for the nuanced ways in which AI tools are employed. In some cases, blanket bans on AI use have proven counterproductive, prompting students to use such tools clandestinely. In others, overly permissive policies have led to widespread misuse. Academic integrity, therefore, must be reframed not in opposition to AI but in dialogue with it, acknowledging the value these tools offer while establishing clear ethical boundaries.
Case Studies: Institutional Responses and Student Misuse
Numerous academic institutions have already encountered incidents that underscore the urgency of addressing AI-related academic misconduct. In 2023, a prominent university in Canada reported that over 70 students were under investigation for submitting near-identical essays on a philosophy assignment, later found to be generated using ChatGPT. Similarly, a high school district in the United Kingdom faced scrutiny when several students produced AI-generated exam answers that evaded detection due to their nuanced construction.
These incidents illustrate that current detection mechanisms are not infallible and that students often operate in a gray area of compliance. They also expose a systemic vulnerability: many institutions have yet to update their codes of conduct to explicitly address AI usage. The absence of clear guidelines not only hinders enforcement but also leaves students uncertain about what constitutes permissible assistance.
In response, some universities have adopted forward-thinking strategies. The University of Sydney, for example, introduced a new academic integrity framework in late 2023 that distinguishes between different types of AI use—ranging from acceptable support (e.g., grammar correction) to prohibited actions (e.g., full assignment generation). This model emphasizes transparency and student education, requiring learners to disclose the use of AI tools in their submissions. Such proactive approaches represent a constructive path forward, balancing enforcement with ethical instruction.
Disrupting Traditional Assessment Models
Another significant impact of generative AI is its disruption of traditional assessment models. Essay-based and take-home assessments, once considered reliable indicators of student ability, are now susceptible to AI manipulation. As a result, educators are increasingly reconsidering the design of evaluations. Some have reverted to in-class exams and oral defenses, while others are exploring novel formats such as project-based learning, collaborative assessments, and iterative feedback loops that prioritize process over product.
This shift necessitates a broader pedagogical transformation. Assessments must evolve to measure not only outcomes but also the student’s engagement with the material over time. Assignments that require reflective writing, personal insight, or references to class-specific discussions are less likely to be effectively outsourced to AI tools. Furthermore, formative assessments—such as weekly drafts, peer reviews, and revision logs—can provide a more comprehensive picture of a student’s academic development.
Psychological and Ethical Implications
The presence of AI in academic settings also introduces psychological and ethical considerations. On one hand, students may experience anxiety or diminished self-confidence, feeling that they cannot match the efficiency or quality of AI-generated content. On the other hand, the normalization of AI use may erode the perceived value of genuine effort and intellectual honesty. If unchecked, this dynamic could foster a culture in which the ends justify the means—where success is measured solely by outcomes, regardless of the process.
Educators must therefore emphasize not only the rules governing AI usage but also the underlying values of academic integrity. Ethical instruction should extend beyond punitive deterrence to include discussions on the importance of originality, intellectual growth, and the long-term consequences of dishonest behavior. Framing academic integrity as a component of personal and professional development may foster a stronger commitment among students to uphold these standards.
Cross-Cultural and Global Considerations
As the use of generative AI becomes globalized, cultural differences in educational values and expectations further complicate the picture. In some regions, collaborative learning and shared resources are normalized to a greater extent, potentially conflicting with Western notions of individual academic authorship. Institutions hosting international students must be especially mindful of these disparities, providing clear guidance and culturally sensitive instruction to prevent unintentional violations.
Moreover, access to AI tools is not uniform across the globe. Students in well-resourced countries often benefit from early and frequent exposure to cutting-edge AI systems, while those in less developed regions may lag behind. This digital divide raises questions of equity and fairness, particularly when AI proficiency begins to influence academic performance. Addressing these disparities will be critical to ensuring that AI-integrated education remains inclusive and just.
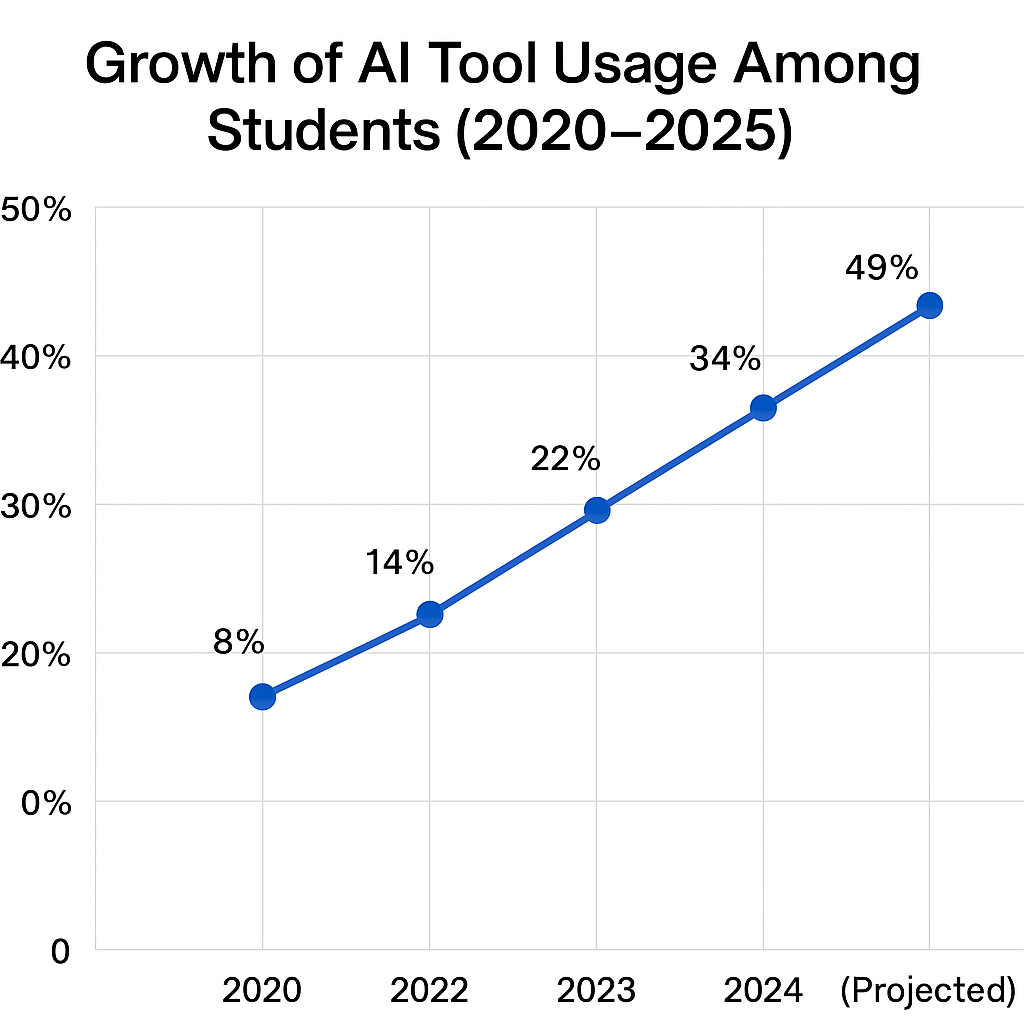
The data in Chart 1 illustrates a steady and significant increase in AI tool adoption among students over a five-year period. If current trends continue, nearly half of all students may be using AI for academic purposes by the end of 2025. This projection underscores the urgency for institutions to develop robust strategies to manage AI usage responsibly.
The advent of generative AI marks a transformative era in education—one filled with both opportunities and risks. As AI tools become increasingly embedded in the academic experience, their impact on integrity cannot be ignored. Students, often caught between efficiency and ethics, require clearer guidance and better-designed learning environments. Likewise, institutions must modernize their policies, assessments, and support systems to meet the demands of this new reality. Only through a balanced, informed, and forward-thinking approach can the core values of academic integrity be preserved in the age of artificial intelligence.
Current Tools for AI Detection and Academic Integrity Enforcement
As generative artificial intelligence (AI) tools become increasingly sophisticated and accessible, academic institutions face a growing imperative to develop and deploy countermeasures that uphold academic integrity. While ethical instruction and assessment redesign remain crucial, detection technologies play an equally pivotal role in identifying instances of AI-assisted misconduct. The emergence of AI-detection tools has created a technological arms race between generative content and content verification. In this section, we examine the most prominent AI-detection solutions, assess their effectiveness, and explore how institutions are integrating these tools into their academic frameworks.
Evolution of AI-Detection Technologies
Traditional plagiarism detection software, such as Turnitin and SafeAssign, has long been a staple in educational settings. These platforms compare submitted work against extensive databases of published materials, previously submitted student papers, and online content to identify copied material. However, generative AI represents a fundamentally different challenge. AI-generated text is often original in the sense that it does not reproduce existing content verbatim, but rather synthesizes plausible responses based on probabilistic models. As a result, conventional plagiarism tools may fail to identify AI-generated submissions because there is no direct textual overlap with known sources.
In response to this limitation, a new class of AI-detection tools has emerged, specifically designed to detect linguistic patterns and statistical markers associated with machine-generated text. These tools employ various methodologies, including perplexity and burstiness analysis, watermarking, stylometry, and deep learning classification models. While these approaches represent a significant advancement, their efficacy varies widely depending on the sophistication of the underlying AI and the nature of the prompt provided.
Overview of Leading AI-Detection Tools
A number of companies and research institutions have developed AI-detection tools tailored to academic environments. Below is an overview of some of the most widely used platforms:
- Turnitin AI Detection: As one of the most recognized names in academic integrity enforcement, Turnitin has expanded its services to include AI-detection capabilities. Its system analyzes writing style, sentence structure, and semantic coherence to identify likely AI-generated content. Integrated directly into many learning management systems (LMS), Turnitin offers seamless compatibility and real-time feedback. However, the company maintains a cautious approach, emphasizing that its detection scores are probabilistic and should be interpreted in conjunction with educator judgment.
- GPTZero: Developed by Princeton student Edward Tian, GPTZero is a lightweight, web-based application that uses perplexity and burstiness to determine whether text is more likely written by a human or an AI. The tool gained popularity due to its simplicity and speed, although it is less accurate on highly edited or short pieces of text. GPTZero remains a useful option for educators seeking a quick, accessible solution.
- Copyleaks AI Content Detector: Copyleaks provides a multi-language AI-detection tool capable of analyzing large volumes of text. Its dashboard includes detailed reports with confidence scores and heatmaps highlighting suspect content. It supports integration with various LMS platforms and offers an API for institutions seeking customized implementation.
- Originality.AI: Positioned as a commercial-grade solution, Originality.AI boasts high accuracy rates and enterprise-level integration options. It uses natural language processing (NLP) and AI classification models to evaluate text for both plagiarism and generative AI usage. The platform is particularly favored by content publishers and online education platforms due to its robust analytics features.
- Hive Moderation AI Detector: Although primarily developed for content moderation in digital media, Hive AI’s detection tool has been adapted for academic contexts. It uses a neural network model trained on a large corpus of human and AI-generated text. While it offers promising accuracy, its primary use cases remain outside the traditional classroom environment.
Comparative Evaluation of Detection Tools
To better understand the capabilities and limitations of these tools, a comparative evaluation is essential. The table below outlines key performance indicators including detection accuracy, false positive rates, integration support, and cost structure.
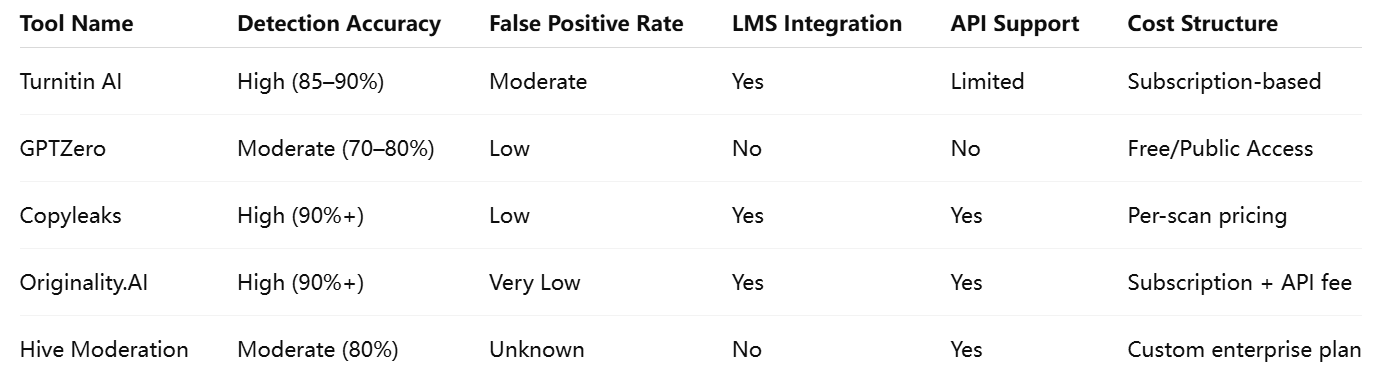
Limitations and Concerns
Despite the growing availability of AI-detection tools, several limitations undermine their reliability and adoption. First, most tools operate on probabilistic models, meaning there is no definitive way to prove authorship without supporting contextual information. This probabilistic nature can lead to false positives, where human-written text is mistakenly flagged as AI-generated. Such errors can have severe academic consequences, particularly when institutional policies rely solely on automated results for disciplinary action.
Second, the effectiveness of detection tools diminishes significantly when students edit or paraphrase AI-generated content. Some students may even use multiple AI tools in succession to "mask" the origin of the text. In such cases, the writing retains human-like qualities, reducing the efficacy of detection algorithms. Additionally, short-form content, such as discussion posts or brief essays, offers insufficient linguistic data for accurate classification.
Third, privacy concerns have emerged regarding how these tools handle and store student submissions. Institutions must ensure that any platform they adopt complies with data protection regulations, such as the General Data Protection Regulation (GDPR) or the Family Educational Rights and Privacy Act (FERPA), especially if the tool operates on cloud-based infrastructure.
Institutional Implementation and Policy Integration
Effective implementation of AI-detection tools requires more than technical integration—it necessitates a comprehensive institutional strategy. Universities that have successfully adopted these tools typically follow a multi-pronged approach:
- Policy Update: Institutions revise their academic integrity policies to explicitly address AI-generated content, defining acceptable and unacceptable uses. These revisions also outline the consequences of misuse and the evidentiary role of detection tools.
- Faculty Training: Educators receive training on how to interpret AI-detection results and contextualize them within broader patterns of student behavior. This human oversight ensures that automated tools serve as guides rather than final arbiters.
- Student Awareness Campaigns: Institutions launch informational campaigns to educate students about the implications of AI misuse and the tools used to detect it. Transparency in detection practices often acts as a deterrent.
- Due Process Frameworks: When AI-generated work is suspected, institutions implement clear procedures for investigation and appeal. Students are typically allowed to explain their process and present evidence of their authorship.
One notable example is the University of Melbourne, which introduced a tiered response system in 2024. Low-confidence flags from detection tools are reviewed by a committee, while high-confidence flags trigger direct instructor review. Students are notified and given an opportunity to respond before any disciplinary measures are taken. This approach balances technological rigor with fairness and transparency.
Future Trends in Detection Technologies
The field of AI-detection is evolving rapidly. Researchers are exploring new techniques such as cryptographic watermarking, in which generative AI systems embed hidden patterns into generated text that can later be verified. OpenAI and other model developers have discussed incorporating such features in future versions to help trace authorship.
Another emerging area is stylometric fingerprinting, which builds a linguistic profile of a student’s past writing to compare against new submissions. While promising, this method raises additional ethical questions around surveillance and consent.
Finally, hybrid models that combine traditional plagiarism detection with AI-based classifiers are gaining traction. These integrated systems provide a more comprehensive assessment of originality and are expected to become standard in institutional settings.
As generative AI continues to redefine the educational landscape, the role of AI-detection tools in safeguarding academic integrity becomes increasingly important. While no tool offers absolute certainty, the best outcomes emerge when detection technologies are embedded within a broader institutional framework that emphasizes education, transparency, and procedural fairness. By combining technical solutions with ethical guidance and adaptive policy-making, academic institutions can effectively mitigate the risks associated with AI-assisted misconduct and uphold the values of scholarship in the digital age.
Proactive Strategies for Educators and Institutions
While detection tools serve a critical role in identifying academic misconduct facilitated by generative AI, a truly effective response to the evolving integrity challenge lies in proactive strategies. These strategies are designed not only to deter unethical behavior but also to promote a culture of trust, transparency, and responsible technology use. By reshaping assessments, rethinking pedagogy, updating institutional policies, and fostering ethical awareness, educators and institutions can reinforce academic integrity without resorting to purely punitive measures. This section explores a comprehensive set of proactive strategies that higher education stakeholders can adopt to address the realities of AI in contemporary academic life.
Redesigning Assessments for Authentic Learning
One of the most effective ways to preserve academic integrity is to reduce the incentives and opportunities for misconduct. This can be accomplished through thoughtful redesign of assessment formats. Traditional take-home essays and online quizzes, which are highly susceptible to AI-assisted generation, must evolve to prioritize originality, context-based evaluation, and iterative engagement.
Project-Based Learning (PBL) and problem-based assessments are particularly effective alternatives. These approaches require students to apply concepts to real-world scenarios, collaborate with peers, and generate unique outputs that reflect their individual thinking. For example, a business course might replace a standard marketing plan assignment with a region-specific campaign proposal based on field interviews and current market analysis—tasks that require input beyond the capabilities of generative AI.
Another promising model is the oral examination or viva voce, where students articulate their thought processes and defend their work in real-time. This method not only discourages reliance on AI but also reinforces communication skills and conceptual understanding. Similarly, portfolio assessments, where students compile and reflect on their work across a semester, provide a longitudinal view of intellectual development that cannot be easily fabricated.
Moreover, scaffolded assignments, which include multiple drafts, peer reviews, and instructor feedback loops, increase transparency and emphasize the learning process. These assessments make it difficult to outsource tasks wholesale, as instructors can track changes and identify inconsistencies in writing style or conceptual growth over time.
Embedding AI Literacy into the Curriculum
A proactive integrity strategy must also include AI literacy education. Rather than treating generative AI tools as forbidden technologies, institutions should integrate instruction on their responsible use into the curriculum. This dual purpose—awareness and empowerment—helps students understand the ethical boundaries of AI in academic and professional contexts.
Courses or modules on digital ethics, algorithmic bias, and intellectual property in the age of AI should be introduced across disciplines. Instructors can create assignments where students critically evaluate the outputs of AI tools, compare them to scholarly sources, and discuss their limitations. For instance, a history class might ask students to fact-check AI-generated content against primary source documents, thereby enhancing both digital literacy and academic rigor.
Such instruction can be reinforced with honor code orientations, where students sign AI-use agreements that clarify permissible behaviors and underscore their personal responsibility. When students comprehend the "why" behind integrity standards—not just the "what"—they are more likely to internalize ethical behavior.
Faculty Development and Pedagogical Innovation
Educators themselves must be equipped with the skills, knowledge, and resources to navigate this new landscape. Faculty development initiatives should focus on pedagogical innovation, assessment design, and technological competence. Workshops, seminars, and interdepartmental collaborations can help instructors rethink their course delivery in light of AI’s capabilities and limitations.
One practical approach is reverse engineering assignments—evaluating how easily an AI could respond to a prompt and adjusting the design accordingly. Prompts that rely on course-specific discussions, personal reflections, or real-time problem solving are less vulnerable to AI replication.
Faculty should also be trained in the interpretation and contextualization of AI-detection tool results. Since such tools generate probabilistic scores, instructors need to combine machine data with qualitative judgment—examining writing voice, development over time, and student participation patterns.
Peer communities of practice, where instructors share experiences, challenges, and best practices in AI-integrated learning environments, can accelerate institutional adaptation. When faculty engage with these issues collectively, the institution develops a more consistent and informed response.
Strengthening Academic Integrity Policies and Governance
Institutional policies play a foundational role in reinforcing proactive strategies. Outdated honor codes and academic integrity policies—many of which predate the emergence of generative AI—must be revised to address new realities. These revisions should go beyond prohibition to establish a graded framework that reflects varying levels of AI use and intent.
A three-tier policy model may include:
- Permitted Use: AI is allowed for basic tasks such as grammar checking or outline generation, provided it is disclosed.
- Conditional Use: AI is allowed under specific conditions and with instructor approval (e.g., drafting code for technical assignments).
- Prohibited Use: AI use is banned in final submission of original essays, exam responses, or research interpretations.
This model supports clarity and flexibility while allowing departments to customize guidelines based on disciplinary norms. Policies must also be transparent and enforceable, offering clear definitions, examples, and a robust appeals process to ensure due process for accused students.
Institutional governance bodies—such as academic senates, integrity boards, and curriculum committees—should work collaboratively to implement and periodically review these policies. Establishing AI Integrity Officers or Ethics Coordinators can further centralize expertise and provide support for both faculty and students.
Cultivating a Culture of Integrity and Trust
Beyond formal structures, fostering a culture of academic integrity requires sustained efforts in community building and values alignment. Institutions must communicate that integrity is not merely a rule to be enforced but a shared commitment to truth, effort, and mutual respect.
Public campaigns, student-led initiatives, and faculty-student dialogues can all reinforce these values. Student academic integrity ambassadors, for example, can lead peer workshops and serve as liaisons between administration and the student body. By giving students a voice in shaping the culture, institutions make ethics feel like a participatory endeavor rather than a top-down imposition.
Additionally, celebrating positive examples—such as students who overcame challenges without compromising their values—can normalize honesty as a form of strength. Honor ceremonies, academic recognition programs, and ethical leadership awards can help reinforce the importance of integrity in academic and future professional life.
Addressing Equity and Accessibility
It is also vital that proactive strategies account for equity and inclusion. Not all students have equal access to generative AI tools, nor do they have equal familiarity with the technology. For instance, international students or those from under-resourced backgrounds may be at a disadvantage in navigating AI’s ethical boundaries or using AI tools for learning enhancement.
Institutions should ensure that policies and instructional materials are inclusive and accessible. This includes:
- Providing equal access to AI tools in controlled settings (e.g., institutional licenses for educational AI software).
- Offering multilingual resources on academic integrity and AI literacy.
- Delivering targeted support for students unfamiliar with digital tools or academic expectations in their new learning environment.
Only when proactive measures are paired with equitable implementation can institutions maintain both integrity and fairness in AI-integrated education.
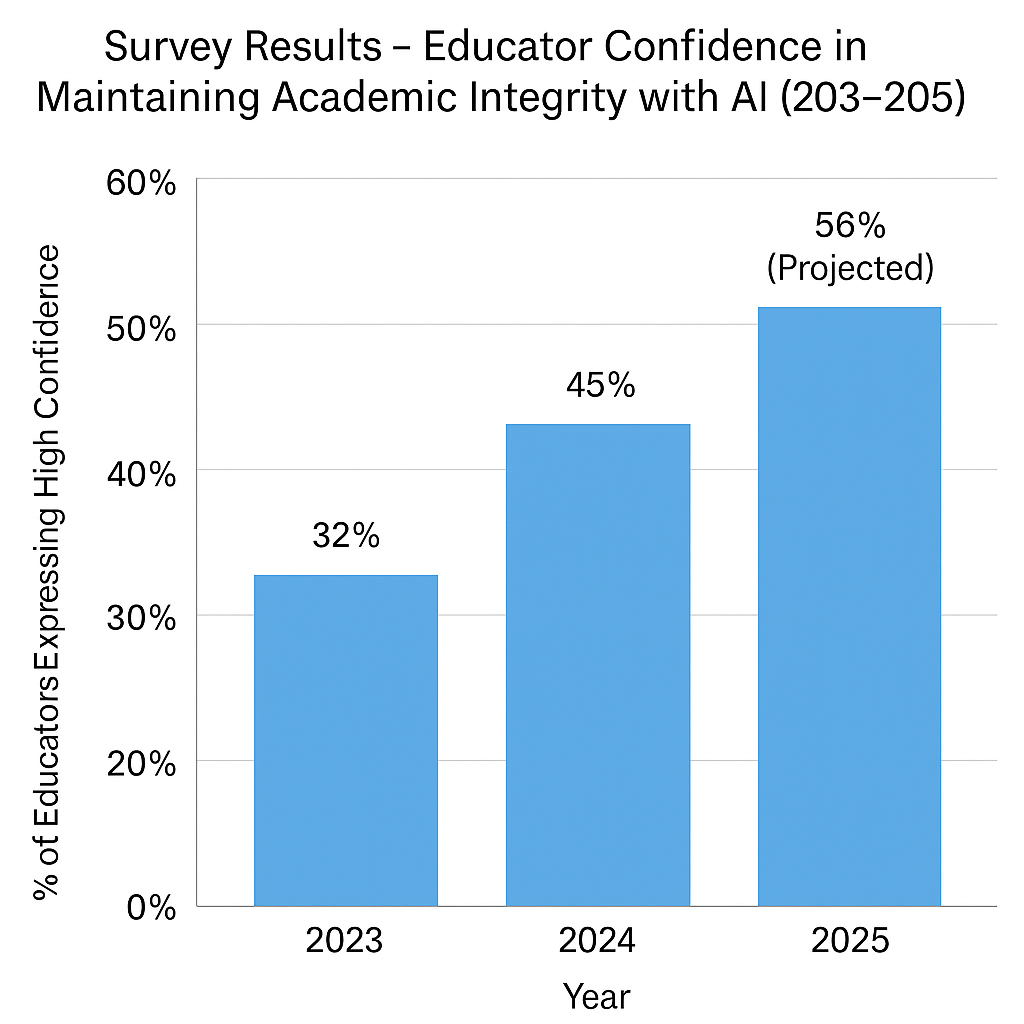
The growing confidence among educators reflects the increasing adoption of training programs, policy adaptations, and collaborative strategies designed to confront AI-related challenges. It suggests a positive trajectory toward institutional readiness.
Ensuring academic integrity in the era of generative AI requires more than surveillance and detection—it demands systemic innovation, ethical education, and cultural transformation. By proactively redesigning assessments, embedding AI literacy, investing in faculty development, modernizing policies, and cultivating an inclusive academic culture, institutions can uphold scholarly values while embracing the realities of technological progress. The path forward is not to reject AI, but to integrate it responsibly, ensuring that academic achievement remains a reflection of authentic effort and intellectual growth.
The Future of Academic Integrity in an AI-Integrated World
As artificial intelligence becomes increasingly embedded in the educational experience, the concept of academic integrity must undergo a significant transformation. No longer can institutions rely solely on traditional honor codes, static assessment formats, or reactive policies. Instead, the future of academic integrity will depend on a dynamic interplay of technological innovation, ethical evolution, and global collaboration. This section explores the emerging paradigms, tools, and frameworks that will shape academic integrity in a world where human and machine capabilities coexist.
Reframing Integrity in the Age of Collaboration
The widespread availability of generative AI challenges the long-held assumption that academic work must be completed independently and without advanced digital assistance. As AI tools evolve from optional aids to integrated elements of daily academic life, the distinction between cheating and legitimate collaboration becomes increasingly blurred. Future frameworks of academic integrity must shift from an exclusive focus on prohibition toward a more inclusive understanding of authorship and contribution.
In this context, transparency and attribution will become central pillars of integrity. Rather than forbidding the use of AI tools outright, institutions will increasingly require students to disclose how and when such tools were used. Just as citations acknowledge the intellectual labor of others, future academic work may include AI disclosure statements, clarifying the boundaries of human and machine-generated input. For example, a student might note that an AI tool assisted in grammar correction or brainstorming but that all substantive arguments and final composition were their own.
This reframing of integrity does not lower standards; rather, it reasserts the importance of intellectual honesty, even in technologically mediated environments. Students must be taught not simply to avoid cheating, but to take ownership of their learning process—even when supported by intelligent systems.
Technological Advancements: Watermarking, Stylometry, and Blockchain
To ensure that academic integrity can be verified in a scalable and defensible manner, the next generation of detection and verification technologies is expected to advance in three primary areas: cryptographic watermarking, stylometric analysis, and blockchain-based authorship tracking.
Cryptographic watermarking refers to the embedding of imperceptible markers within AI-generated content that can be later detected by authorized tools. OpenAI and other large language model providers are researching watermarking mechanisms that could signal whether a piece of text was generated by their systems. These markers would not be visible to the human eye but could be extracted algorithmically, thereby enabling educators to distinguish AI-authored content with higher confidence.
Stylometric analysis focuses on identifying authorship based on individual writing style. Each person has a unique linguistic fingerprint—patterns in syntax, word choice, sentence length, and punctuation usage. Stylometry tools can compare a student's previous submissions to new work and flag inconsistencies that suggest outside assistance. While powerful, this method also raises ethical concerns related to privacy and consent, and must therefore be deployed within clear legal and procedural boundaries.
Blockchain-based systems represent a novel approach to validating the authenticity of academic work. By recording each stage of the writing process—drafts, edits, and peer reviews—on a decentralized ledger, blockchain can establish a tamper-proof chain of authorship. Students and educators would have access to a transparent timeline of content creation, making it far more difficult to fabricate or outsource academic submissions. Though still in early stages, blockchain has the potential to revolutionize how academic work is documented and verified.
Global Harmonization of Academic Standards
The ethical challenges posed by generative AI are not confined to a single country or education system. As online learning becomes more globalized, there is a growing need for international consensus on academic integrity standards. Students from different cultural and regulatory backgrounds interact in digital classrooms, and without a common framework, inconsistencies in expectations and enforcement can lead to confusion or perceived injustice.
Organizations such as the International Center for Academic Integrity (ICAI) and UNESCO are well-positioned to spearhead global initiatives. These may include the development of international AI ethics guidelines, model academic integrity policies, and cross-border credentialing protocols that incorporate AI-awareness training.
In parallel, collaborative platforms will emerge to enable institutions to share data, case studies, and detection algorithms—much like global banking systems share fraud intelligence. These platforms will help standardize best practices and reduce duplication of effort across institutions.
Shifting Toward Constructive Integrity Models
Future approaches to academic integrity will increasingly emphasize constructive rather than punitive frameworks. Rather than focusing primarily on catching violations and issuing penalties, educators will work to build environments where students are motivated to act ethically from the outset.
This model of integrity recognizes that most academic misconduct is not driven by malice but by pressure, confusion, or lack of preparation. Accordingly, support systems such as academic coaching, time management workshops, and mental health services will be recognized as integrity-enhancing resources. Preventing misconduct will be viewed as a function of creating resilient learners, not just vigilant supervisors.
Moreover, formative assessments that encourage reflection on the learning journey, rather than only rewarding outcomes, will gain prominence. Students may be asked to submit learning journals alongside assignments, documenting their research paths, moments of confusion, and decisions to seek help—offering insight into their academic integrity beyond the final product.
Professional Implications and Lifelong Ethics
Finally, academic integrity in the AI-integrated world must be viewed not as an isolated phase of student life, but as a foundation for professional and lifelong ethical conduct. As AI tools become commonplace in medicine, law, journalism, engineering, and other professions, the habits formed during university years will influence how future workers interact with automation, data, and digital tools.
Institutions of higher education thus bear a responsibility to instill transferable ethical principles that extend beyond campus. Curricula should address the responsible use of AI in professional contexts, equipping students to make morally sound decisions in environments where automation and accountability intersect.
Professional accreditation bodies and employers are also likely to demand AI-ethics certifications or digital literacy credentials as part of hiring and promotion criteria. Forward-thinking universities will align their integrity education with these emerging workforce expectations, making ethical readiness a hallmark of academic success.
The future of academic integrity in an AI-integrated world demands a reimagining of how knowledge is created, assessed, and attributed. Technological advancements such as watermarking and stylometry will enhance verification, while global standards and collaborative ecosystems will support consistency. Yet the most enduring transformation will be philosophical: a shift from punishing violations to cultivating a culture of ethical learning and responsible innovation. By proactively engaging with these trends, educators and institutions can ensure that academic integrity not only survives but evolves to meet the challenges—and opportunities—of the AI era.
Conclusion
The advent of generative artificial intelligence has profoundly reshaped the educational landscape, introducing both unprecedented opportunities for learning and complex challenges to academic integrity. As AI technologies become ubiquitous, traditional paradigms of scholarship—centered on individual authorship, originality, and effort—are being tested in ways that few institutions were prepared to address. The question, therefore, is not whether AI will continue to influence education, but how stakeholders can uphold the foundational values of academia within this new context.
Throughout this analysis, we have examined the multifaceted nature of AI's impact on academic integrity. From the evolving ways students engage with AI tools to the challenges educators face in discerning machine-generated work, it is clear that passive approaches are no longer adequate. Detection tools, while valuable, are not foolproof; they must be complemented by proactive strategies that emphasize ethical awareness, assessment innovation, and institutional adaptability.
The integration of AI-detection technologies has emerged as a critical line of defense. Platforms like Turnitin AI, GPTZero, and Copyleaks offer educators new methods of verification, but their effectiveness depends on proper implementation, human oversight, and the clarity of institutional policies. As the capabilities of AI grow, so too must the sophistication of these tools—through watermarking, stylometric analysis, and even blockchain verification. Yet, even the most advanced detection systems cannot substitute for a culture of integrity.
Proactive strategies are indispensable. By redesigning assessments to prioritize critical thinking and originality, embedding AI literacy into the curriculum, and investing in faculty development, institutions can foster environments that naturally deter misconduct. Students, when empowered with clear guidelines and ethical reasoning skills, are more likely to use AI as a constructive aid rather than a means of evasion.
Looking ahead, the very definition of academic integrity must evolve. In an AI-integrated world, transparency, accountability, and collaborative ethics will become the cornerstones of scholarly conduct. Integrity will be less about resisting new tools and more about using them responsibly, with a full understanding of their implications. This evolution demands updated policies, global cooperation, and a shift in mindset—from punitive enforcement to constructive engagement.
In conclusion, the future of academic integrity lies not in attempting to eliminate AI from the educational process, but in learning to coexist with it ethically and intelligently. Institutions that rise to this challenge will not only safeguard their academic standards but also prepare students for a future in which integrity, innovation, and human agency must go hand in hand.
References
- Turnitin AI Writing Detection Capabilities
https://www.turnitin.com/products/ai-writing-detection - GPTZero — AI Detection for Educators
https://gptzero.me - Copyleaks — AI Content Detection Tool
https://copyleaks.com/ai-content-detector - Originality.AI — AI Detection & Plagiarism Checker
https://originality.ai - UNESCO — Guidance for Generative AI in Education
https://www.unesco.org/en/articles/guidance-generative-ai-education - International Center for Academic Integrity (ICAI)
https://academicintegrity.org - EdSurge — AI in Classrooms and Policy Implications
https://www.edsurge.com/news - ISTE — Artificial Intelligence in Education Resources
https://www.iste.org/areas-of-focus/AI-in-education - The Conversation — How AI Is Changing Student Learning
https://theconversation.com