How AI is Eliminating Financial Errors and Transforming Enterprise Risk Management
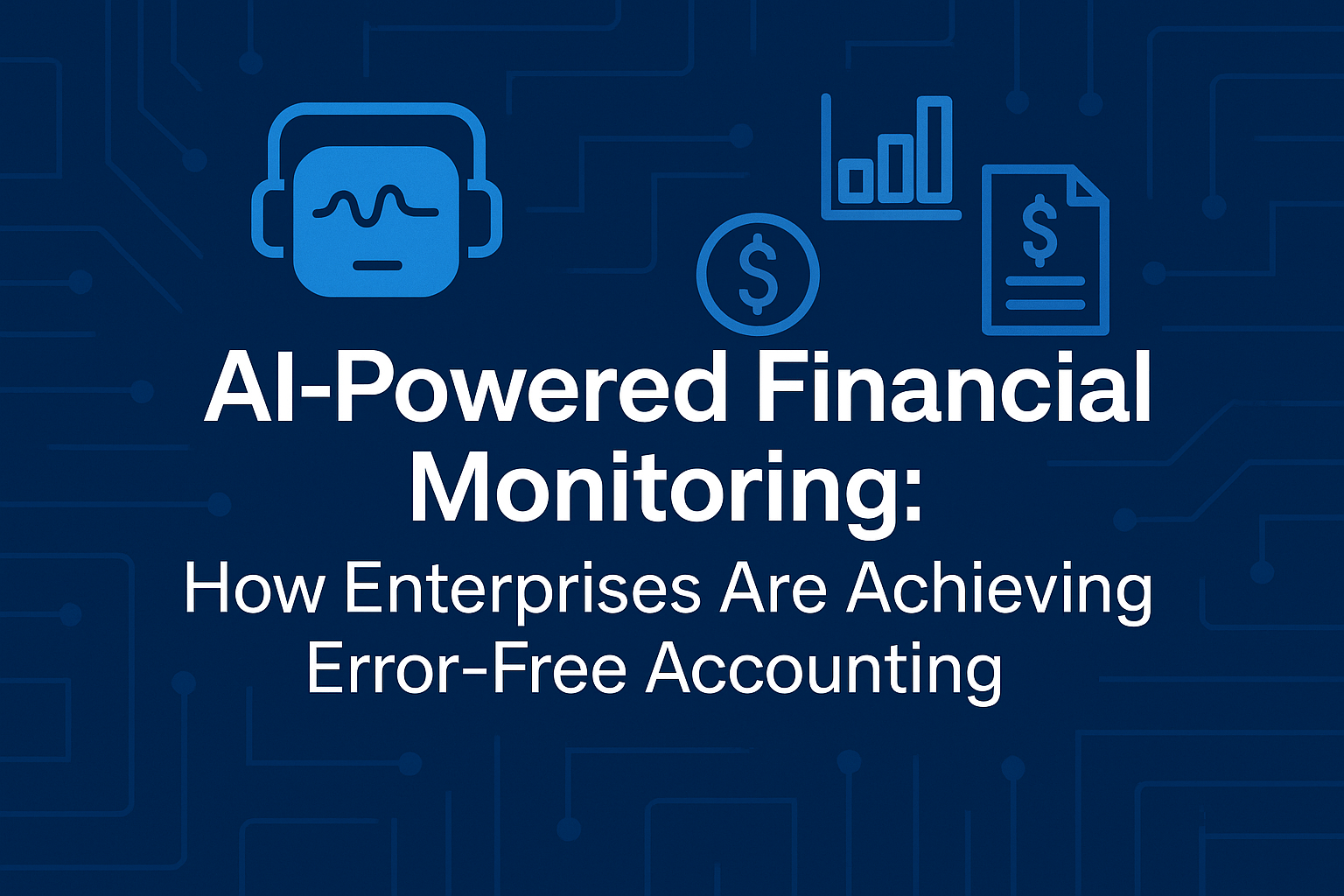
In today’s fast-paced financial environment, accuracy and accountability are not optional—they are critical pillars of institutional stability and investor trust. Yet, despite decades of automation and digital transformation, financial errors remain pervasive and costly. From erroneous journal entries and misclassified transactions to undetected fraud and overlooked regulatory breaches, these mistakes can ripple through entire financial ecosystems, resulting in substantial monetary losses, reputational damage, and compliance penalties. According to recent industry assessments, financial reporting errors cost global businesses hundreds of billions annually, and these figures are expected to rise as transactional volumes and data complexity continue to escalate.
Traditionally, organizations have relied on structured internal controls, periodic audits, and human-led reconciliation processes to identify and address financial anomalies. While these methods have served as the foundation of financial governance, they are increasingly ill-suited for the demands of modern financial operations. Manual checks are slow, resource-intensive, and often retrospective, detecting problems long after they have occurred. As financial systems grow more interconnected and data sources more diverse, the traditional models for error detection and risk assessment struggle to keep up with the scale, speed, and subtleties of real-time finance.
Enter Artificial Intelligence. With its capacity to analyze vast quantities of structured and unstructured financial data, identify hidden patterns, and flag anomalies in real time, AI has emerged as a transformative force in financial error detection and management. Rather than merely replacing existing controls, AI enhances them by offering a new layer of predictive precision and automated response that was previously unattainable. From machine learning algorithms that pinpoint irregular transactions to natural language processing tools that interpret invoice narratives, AI technologies are fundamentally redefining how organizations perceive, identify, and rectify financial discrepancies.
The adoption of AI in financial workflows is not a distant vision—it is already taking shape across major enterprises and financial institutions. AI tools are now integrated into enterprise resource planning (ERP) systems, embedded in risk management platforms, and deployed across audit and compliance functions. Whether it’s detecting duplicate payments in accounts payable or forecasting the likelihood of accounting misstatements before quarterly close, AI brings not just speed and accuracy, but also continuous learning, allowing systems to evolve and adapt over time.
This blog explores the critical ways in which AI is revolutionizing financial error detection and management. Through a structured examination of how AI works, the benefits it delivers, the platforms driving innovation, and the challenges that still remain, we aim to provide a comprehensive overview of this rapidly advancing domain. In a world where financial integrity is paramount, and regulatory scrutiny is intensifying, AI offers a compelling path forward—toward a future where financial errors become not just detectable, but preventable.
How AI Detects Financial Errors in Real Time
The capacity to detect financial errors as they occur, rather than weeks or months after the fact, marks a critical advancement in financial operations. At the heart of this transformation lies Artificial Intelligence (AI), which enables the continuous monitoring of financial data flows, offering unprecedented precision, speed, and scalability. Unlike traditional rules-based systems, which rely on predefined parameters and are often brittle in the face of complexity or novelty, AI systems leverage machine learning (ML), natural language processing (NLP), and statistical anomaly detection to uncover hidden patterns and identify inconsistencies in real time.
Machine Learning and Anomaly Detection
At the core of AI-driven financial error detection is supervised and unsupervised machine learning. These models are trained on large datasets containing historical financial transactions, accounting entries, reconciliations, and corrections. Supervised learning algorithms are often used where labeled data—examples of both correct and incorrect entries—are available. These models learn the typical behavior of legitimate financial transactions and can flag deviations that may indicate errors, fraud, or non-compliance.
Unsupervised models, on the other hand, do not require labeled datasets and are especially effective in identifying outliers. These models analyze high-dimensional data to determine what constitutes “normal” behavior and trigger alerts when a transaction falls outside this learned distribution. For instance, an unusually large payment to a vendor, a journal entry with atypical account codes, or an entry processed outside of regular business hours can be flagged immediately for review. Such algorithms are adaptive and improve over time as they are exposed to more data, making them particularly well-suited for dynamic financial environments.
Natural Language Processing in Unstructured Data
Modern financial operations often involve a blend of structured and unstructured data. While structured data includes ledger entries, balances, and codes, unstructured data consists of invoice texts, email communications, contracts, and handwritten receipts. NLP, a branch of AI focused on language interpretation, is instrumental in parsing and extracting meaning from this unstructured content.
By applying NLP techniques such as named entity recognition, sentiment analysis, and semantic similarity, AI systems can, for example, read an invoice and verify whether the amounts, dates, and supplier names match the entries in the financial system. They can also identify language inconsistencies in contract clauses that may affect financial treatment. This enables organizations to reduce human errors caused by misinterpretation or manual data entry, and to automate compliance checks against financial documentation in real time.
Predictive Analytics and Behavior Modeling
AI does not merely operate reactively; it also offers predictive capabilities. Through time-series forecasting and behavioral modeling, AI can assess the likelihood of future financial irregularities. For example, based on historical data, an AI system can predict which accounts are most likely to exhibit reconciliation issues during period-end closing. Predictive analytics also assists in cash flow forecasting and fraud risk scoring by learning from trends across multiple datasets, including transaction logs, account history, and vendor behavior.
This forward-looking capacity allows financial teams to intervene proactively, correcting or preventing issues before they materialize in formal reports or external audits. Predictive modeling, when combined with workflow automation, can even trigger preemptive measures such as holding payments, issuing alerts, or requiring managerial approval for certain activities.
Integration with Enterprise Systems and APIs
For AI to function effectively in real time, it must be deeply integrated into the digital backbone of the organization. This is achieved through application programming interfaces (APIs) and connectors to enterprise resource planning (ERP) systems, general ledgers, procurement platforms, and bank feeds. These integrations ensure that AI tools have direct access to live financial data streams and can process transactions at the point of entry.
Many leading ERP platforms now offer native AI capabilities or third-party plugins that continuously evaluate financial activity. For instance, an AI layer embedded within an ERP can scan all outgoing vendor payments in real time, automatically comparing them to approved purchase orders and historical vendor behavior, instantly identifying duplications or unauthorized disbursements.
Case Study: AI in Accounts Payable Monitoring
Consider the application of AI in the accounts payable (AP) function—a domain historically plagued by duplicate invoices, erroneous payments, and compliance failures. AI can analyze thousands of invoice line items, detect patterns that deviate from established norms, and cross-reference payment data with contractual terms. When an anomaly is detected—such as a vendor being paid twice for the same invoice—an automated workflow is triggered that notifies the AP team and places the payment on hold pending further review.
Moreover, AI systems can learn vendor-specific patterns and flag deviations that may be missed by static rule-based checks. For example, if a vendor historically charges a specific rate for a product but suddenly submits an invoice with a significant price increase, the AI model can recognize the discrepancy even if it technically passes the rule-based thresholds.
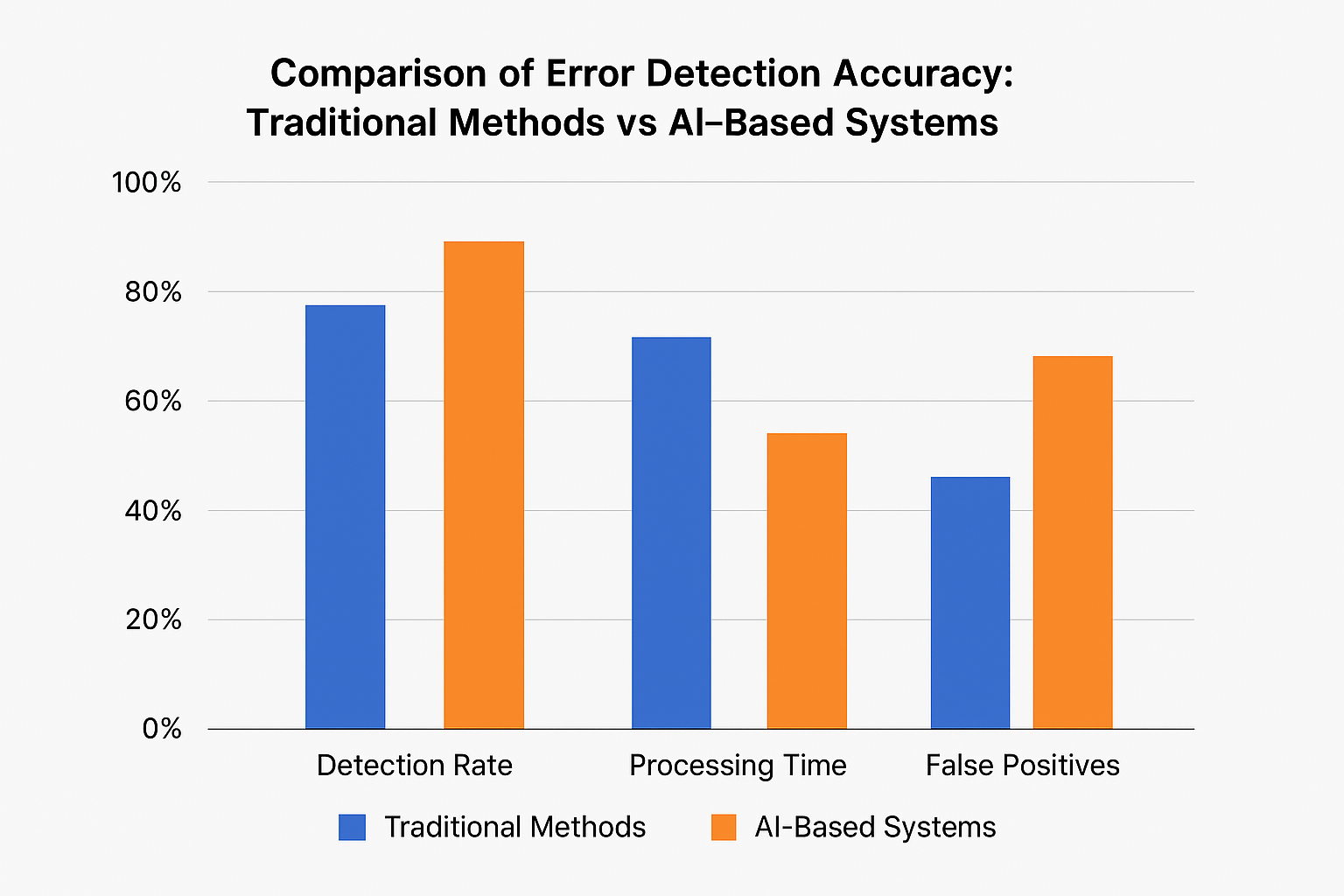
To illustrate the effectiveness of AI over traditional financial monitoring, we present a comparison of detection accuracy, processing time, and false positive rates. The chart will demonstrate how AI-based systems significantly outperform manual reviews and rule-based logic, offering real-time feedback with higher accuracy and efficiency.
By enabling continuous, intelligent scrutiny of financial operations, AI shifts the paradigm from retrospective correction to proactive error prevention. This foundational capability paves the way for more advanced applications, such as AI-assisted correction workflows and strategic decision-making based on real-time insights—topics we will explore in the next section.
AI-Driven Error Management and Correction Workflows
While error detection is a vital first step, the true power of Artificial Intelligence (AI) in financial operations lies in its ability to drive intelligent error resolution and management. AI systems not only identify discrepancies in real time but also trigger automated workflows, generate actionable insights, and recommend corrective measures. This transformation in financial governance shifts the traditional reactive model of error correction to a more proactive, autonomous, and data-driven paradigm.
From Identification to Resolution: The Automation Pipeline
Once an anomaly is detected, the AI engine initiates a workflow tailored to the severity, nature, and context of the error. These workflows typically begin with triage—categorizing the error by type (e.g., misclassification, duplication, fraud, or reconciliation mismatch)—and assigning it a risk score. This risk score determines the urgency and the appropriate level of escalation.
Low-risk anomalies, such as minor ledger mispostings or duplicate entries, are often resolved through automated rule-based corrections. For instance, if an expense is mistakenly logged under the wrong department, AI can automatically reclassify it based on historical patterns or contextual cues in the transaction metadata. In contrast, high-risk errors, particularly those suggesting potential fraud or regulatory non-compliance, are routed to human reviewers, with AI providing an audit-ready trail of data points, evidence, and suggested actions.
This layered automation allows for both efficiency and oversight. Errors are no longer left to be discovered at the end of reporting cycles but are flagged and addressed in a continuous, real-time loop. As a result, finance teams can allocate their resources strategically—focusing on exception handling and compliance rather than mundane data corrections.
Intelligent Recommendations and Self-Healing Systems
A major advantage of AI over legacy financial tools is its ability to learn from past resolutions and apply those lessons to future errors. Through reinforcement learning and pattern recognition, AI systems build a repository of corrective actions and outcomes. When a new error emerges, the system can compare it to prior incidents, analyze the effectiveness of past responses, and recommend the most likely resolution.
This capability gives rise to what are known as “self-healing” financial systems. In these systems, AI not only detects and classifies the issue but also executes the corrective action autonomously—pending validation in sensitive environments. For example, when a foreign exchange transaction is entered using an outdated rate, the system can detect the inconsistency, fetch the current rate from integrated financial feeds, adjust the amount, and rebook the entry, all within seconds.
These intelligent workflows significantly reduce cycle times and improve accuracy, especially in high-volume functions like accounts receivable, treasury management, and payroll reconciliation. Furthermore, the learning component ensures that the system continually refines its models, becoming more accurate and efficient over time.
Enhanced Collaboration and Escalation Paths
AI does not operate in isolation; rather, it augments the decision-making of financial professionals. Advanced AI platforms include collaborative features such as intelligent notifications, automated reporting, and workflow dashboards. When an issue requires human intervention, the system provides the relevant data context—highlighting the root cause, the impacted accounts, prior similar cases, and risk assessments.
These tools reduce the cognitive load on analysts and allow for faster, more informed decisions. Escalation paths can be customized based on roles and responsibilities. For example, entries involving material adjustments may require controller approval, while low-value corrections can be auto-approved based on pre-set rules. Integration with communication platforms like Slack, Microsoft Teams, or internal ticketing systems further enhances workflow agility and responsiveness.
Audit-Readiness and Compliance by Design
Another critical outcome of AI-driven error management is the creation of an audit-ready environment. Every detected anomaly, the logic behind the detection, and the corrective steps taken are documented in an immutable digital log. This level of traceability simplifies internal audits, supports external regulatory examinations, and ensures compliance with standards such as Sarbanes-Oxley (SOX), IFRS, and GAAP.
Moreover, AI systems can be programmed to enforce compliance at the point of transaction, not merely during post-facto review. For example, if a journal entry violates a segregation-of-duties policy, the system can prevent it from being posted, notify compliance officers, and flag the user account for further review.
Such preventative controls are increasingly vital in a world where financial data is dispersed across cloud platforms, global teams, and third-party systems. AI ensures that governance protocols are embedded into the financial ecosystem, making compliance intrinsic rather than incidental.
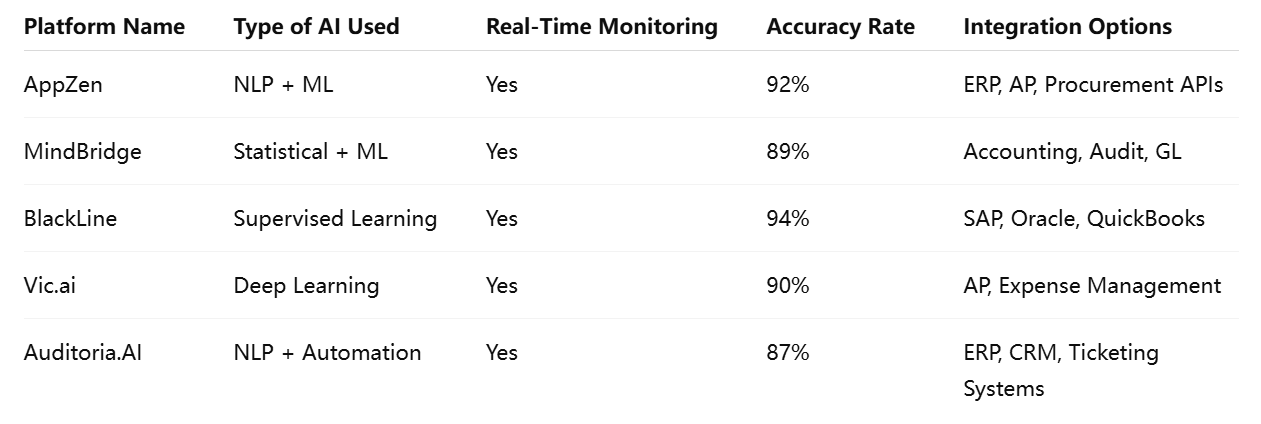
Through the orchestration of intelligent workflows, AI allows financial teams to move from a state of reactive correction to one of proactive control and continuous assurance. These capabilities not only streamline operational efficiency but also build organizational resilience against internal errors and external scrutiny. As we will explore in the next section, these benefits extend beyond technical efficiency and offer tangible strategic advantages for enterprises seeking to modernize their financial operations.
Strategic Benefits for Enterprises and Financial Institutions
As enterprises increasingly adopt Artificial Intelligence (AI) in their financial workflows, the shift is not merely technological—it is profoundly strategic. AI-driven error detection and correction systems yield a suite of tangible benefits that extend far beyond operational efficiency. These advantages resonate across the enterprise value chain, impacting cost structures, risk management, compliance posture, stakeholder trust, and even long-term competitiveness. For financial institutions and global enterprises alike, leveraging AI in financial management is becoming a critical differentiator in a rapidly evolving digital economy.
Enhanced Accuracy and Reduction of Financial Risk
At its core, the use of AI in financial systems delivers significant improvements in the accuracy and integrity of financial data. By analyzing large volumes of structured and unstructured data in real time, AI minimizes the likelihood of errors that may otherwise go unnoticed in traditional workflows. This proactive approach reduces the frequency of financial restatements, minimizes regulatory violations, and improves overall audit outcomes.
Moreover, reducing manual processing errors directly translates into lower financial risk. For instance, in areas such as treasury operations or foreign exchange transactions, even minor discrepancies can lead to substantial financial losses. AI algorithms can validate transaction values, match them to real-time market data, and flag inconsistencies instantaneously, preventing potential leakage or misreporting.
Operational Cost Savings and Efficiency Gains
Another key advantage of AI in financial error management is its ability to drive down operational costs. Traditional financial controls require extensive human labor for monitoring, reconciliation, and auditing—activities that are both time-consuming and prone to oversight. AI automates many of these functions, enabling leaner finance departments and freeing up staff to focus on higher-value tasks such as strategy, forecasting, and risk analysis.
For example, a multinational enterprise using AI-powered tools to manage accounts payable can reduce invoice processing time by more than 50%, cut down on late payment penalties, and avoid duplicate payments. These savings are not marginal; they represent millions of dollars in potential annual efficiency gains for large organizations.
In financial institutions, AI can also replace or augment legacy software systems, reducing licensing costs, IT support burdens, and integration overhead. With the emergence of cloud-native AI platforms, the barriers to entry are lower, and scalability is far greater than before.
Accelerated Financial Close and Real-Time Reporting
One of the most impactful outcomes of AI integration is the acceleration of financial closing processes. Monthly, quarterly, and annual closes are often marred by data delays, reconciliation bottlenecks, and error correction cycles. AI addresses these pain points by continuously auditing transactions, reconciling mismatches, and verifying documentation in real time.
As a result, organizations can achieve what is increasingly referred to as a “continuous close”—an environment where financial statements can be generated with near-instant accuracy and minimal manual intervention. This agility is crucial in today’s fast-paced business environment, where executive teams require real-time visibility into financial performance to make timely strategic decisions.
Moreover, real-time reporting powered by AI enables more granular, drill-down insights into financial anomalies, segment-level performance, and operational inefficiencies. This empowers finance leaders with actionable intelligence rather than static summaries, elevating the finance function from scorekeeper to strategic advisor.
Improved Compliance and Regulatory Assurance
In an era of increasing regulatory scrutiny and complex global compliance standards, AI offers a vital layer of assurance. Financial institutions are particularly burdened by regulations such as Basel III, MiFID II, SOX, and the Dodd-Frank Act, all of which demand real-time visibility, audit trails, and risk transparency.
AI systems can continuously monitor for violations of internal policies and external regulatory requirements, automatically flagging and documenting irregularities for compliance teams. This not only helps avoid penalties but also streamlines the audit process by providing automated evidence logs and explanation layers that are critical for regulatory submissions.
Furthermore, the transparency and traceability embedded in AI workflows help strengthen governance. By maintaining a secure digital trail of every transaction and its validation process, organizations can demonstrate accountability and control to both internal and external stakeholders.
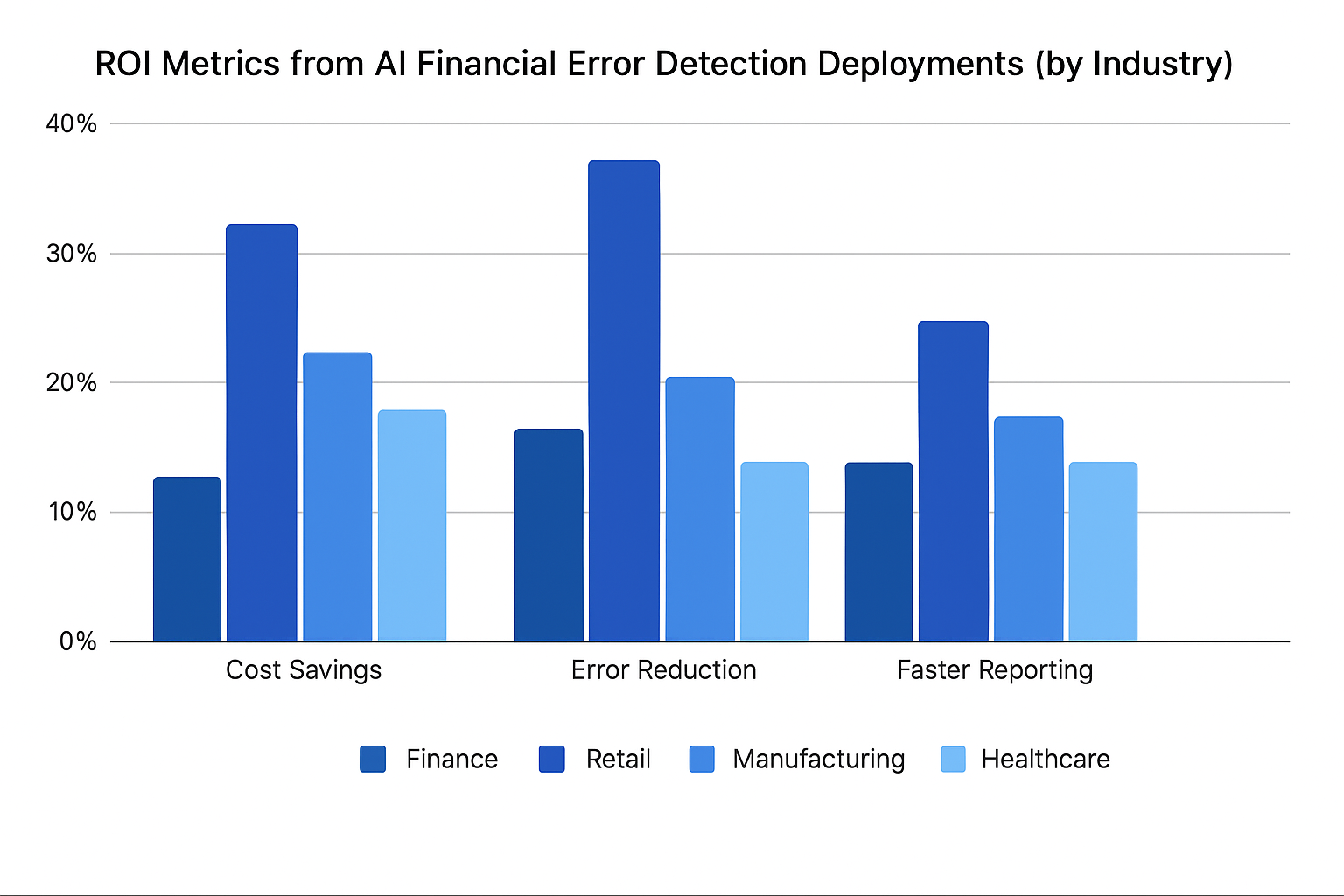
This chart presents a comparative analysis of return on investment (ROI) across various industries—including banking, retail, manufacturing, and technology—that have adopted AI for financial error detection. Metrics include cost savings, error reduction rates, and improvements in reporting speed. The data highlights how sectors with high transaction volumes see the most significant gains, reinforcing AI's scalability and effectiveness across diverse financial environments.
Strengthening Stakeholder Confidence
In the broader context of corporate trust and investor relations, AI-enhanced financial accuracy can significantly strengthen stakeholder confidence. When financial statements are free from material errors and audits uncover fewer discrepancies, the credibility of leadership increases. This credibility becomes a strategic asset—especially for publicly traded firms, investment banks, and insurance companies that operate under intense investor scrutiny.
AI also aids in proactive investor communication. With real-time access to validated financial data, CFOs and investor relations teams can offer more accurate forward guidance, defend valuation metrics, and provide transparent responses to queries about performance anomalies.
In turn, this increased transparency reduces market speculation and bolsters share price stability. For financial institutions managing client assets or underwriting risk, it also translates to improved customer trust and retention.
In summary, the adoption of AI in financial error detection and management yields a profound set of strategic advantages. From reducing operational costs and mitigating financial risk to accelerating reporting cycles and strengthening compliance, the business case for AI is robust and multifaceted. These benefits are not merely incremental—they are transformative. In the next section, we will address the challenges that organizations must navigate to fully realize these advantages, including the ethical, technical, and regulatory complexities of deploying AI in financial domains.
Challenges and Ethical Considerations in AI-Based Financial Monitoring
Despite the impressive capabilities and strategic advantages of Artificial Intelligence (AI) in financial error detection and management, the adoption of these systems is not without challenges. As organizations implement AI tools to automate and optimize financial oversight, they must confront a series of technical, operational, ethical, and regulatory issues. These challenges underscore the importance of designing AI systems with transparency, accountability, and governance at the forefront. Failure to do so may not only compromise the effectiveness of these technologies but also expose organizations to new forms of risk—both financial and reputational.
Data Quality and Systemic Bias
AI systems are only as reliable as the data on which they are trained. Inaccurate, incomplete, or unstructured financial data can lead to flawed learning outcomes and poor decision-making. For example, if an AI model is trained on historical financial records that include misclassifications or uncorrected anomalies, it may normalize these inaccuracies and even reproduce them in future predictions or recommendations.
Moreover, systemic bias embedded in historical data can skew AI outputs, particularly when models are used to predict financial risk or flag suspicious activity. If, for instance, a dataset overrepresents certain types of transactions as “high risk” due to human-driven mislabeling in the past, the AI system may unfairly target those categories, leading to operational inefficiencies and potential discrimination claims.
To mitigate these risks, organizations must establish rigorous data governance frameworks. This includes cleansing and validating datasets, using diverse data inputs, and regularly auditing AI model performance. Continuous monitoring is critical to ensure that AI systems adapt to evolving financial behaviors while maintaining accuracy and fairness.
Lack of Explainability and Transparency
One of the most persistent concerns in AI adoption—especially in financial contexts—is the “black box” problem. Many AI models, particularly those based on deep learning, generate outputs that are difficult for users to interpret or explain. This opacity can be especially problematic in regulated industries like banking and insurance, where financial professionals are expected to justify decisions based on traceable logic.
Auditors, regulators, and internal stakeholders may find it challenging to trust decisions made by a system that cannot clearly articulate how it reached a particular conclusion. For example, if an AI system blocks a multi-million-dollar transaction due to an “anomalous pattern,” financial executives must be able to understand and verify the rationale behind the decision.
To address this, financial AI solutions must include explainability features, such as interpretable models, decision trees, or visual interfaces that map data pathways and scoring logic. Emerging fields like eXplainable AI (XAI) are making strides in this area, but significant development is still needed to ensure that AI systems meet the transparency standards required for financial governance.
Privacy and Confidentiality Risks
Financial data is among the most sensitive information held by any organization. The use of AI systems to ingest, process, and analyze this data introduces privacy concerns—especially if data is being shared across systems, stored in the cloud, or processed by third-party vendors.
Unauthorized access, data breaches, or improper handling of sensitive financial records could result in severe legal and reputational consequences. Moreover, regulations such as the General Data Protection Regulation (GDPR), California Consumer Privacy Act (CCPA), and other data protection laws impose strict requirements on data use, consent, storage, and deletion.
To ensure compliance, organizations must implement robust data encryption, role-based access controls, and end-to-end data lifecycle management within their AI ecosystems. Additionally, any AI system processing personal or financial data should undergo regular security audits and third-party assessments to identify vulnerabilities.
Regulatory Ambiguity and Evolving Standards
While AI has outpaced many existing financial technologies in sophistication and scale, regulatory frameworks have struggled to keep up. Most financial regulations were written for traditional systems and human decision-makers, not for autonomous algorithms capable of learning and evolving independently.
This regulatory lag presents a double-edged sword. On one hand, it allows early adopters of AI to innovate with fewer constraints; on the other, it creates uncertainty around compliance, liability, and auditability. Organizations may find themselves exposed to regulatory penalties if their AI-driven decisions cannot be justified or aligned with established accounting and reporting standards.
Global regulators are beginning to issue guidelines on AI use in finance—such as the European Union’s proposed AI Act and the Financial Conduct Authority’s (FCA) principles for ethical AI—but many questions remain unanswered. These include: Who is accountable for an AI system’s decision? What constitutes due diligence in AI monitoring? How can fairness be measured?
As such, enterprises must take a proactive approach. Legal, compliance, and finance departments should collaborate to establish internal governance policies that anticipate regulatory developments and set internal benchmarks for ethical AI use.
The Need for Human Oversight and Ethical Design
Despite their autonomy, AI systems cannot and should not operate in a vacuum. Human oversight remains essential to ensure ethical decision-making, mitigate risks, and maintain organizational accountability. Financial professionals must be involved in designing workflows, reviewing flagged anomalies, and approving high-impact corrective actions.
Furthermore, ethical considerations must be embedded into the design of AI systems from the outset. This includes ensuring inclusivity in training data, avoiding algorithmic favoritism, and setting thresholds that balance automation with human review. Organizations should also implement AI ethics boards or review committees to assess the social and organizational implications of AI deployment in financial domains.
Such measures are not only good practice—they are critical to building trust. As financial stakeholders become more attuned to the use of advanced technologies, they will demand transparency, ethical safeguards, and clear lines of responsibility for AI-driven decisions.
In conclusion, while the promise of AI in financial monitoring is immense, it must be pursued with caution, diligence, and a firm commitment to ethical integrity. The challenges of data quality, explainability, privacy, and regulatory compliance are not insurmountable—but they require deliberate, organization-wide strategies. By approaching AI adoption through a lens of responsible innovation, enterprises can harness its benefits while safeguarding their stakeholders and upholding the standards of financial governance. In the final section, we will explore the future trajectory of AI in finance, including emerging innovations and the potential for fully autonomous financial ecosystems.
Toward a Zero-Error Financial Future
The financial landscape is undergoing a profound transformation, driven by the integration of Artificial Intelligence (AI) into the core of error detection, management, and compliance systems. This shift is not simply a matter of adopting new tools—it represents a fundamental reimagining of how financial accuracy, governance, and responsiveness are achieved at scale. As demonstrated across this blog, AI is no longer a speculative technology in the realm of finance; it is a powerful operational engine delivering measurable improvements in error detection precision, cost reduction, reporting speed, and regulatory compliance.
Enterprises and financial institutions that have implemented AI-powered financial workflows are witnessing a departure from the traditional, labor-intensive model of retrospective error correction. Instead, they are moving toward real-time financial ecosystems that monitor, flag, and correct discrepancies as they occur. This evolution not only improves operational efficiency but also reduces financial exposure and bolsters internal controls. The integration of machine learning, natural language processing, and predictive analytics empowers organizations to go beyond detection and embrace proactive, and in some cases autonomous, error remediation.
The strategic benefits of this transition are far-reaching. From accelerating the financial close process and enhancing audit readiness to improving stakeholder transparency and investor confidence, AI augments both performance and trust. At a time when financial integrity is under increasing scrutiny—from regulators, shareholders, and the public—these capabilities offer a critical edge in safeguarding reputation and preserving enterprise value.
However, the path to AI-enabled financial excellence is not without its complexities. As discussed, organizations must navigate a range of technical and ethical challenges, including data integrity, algorithmic transparency, regulatory ambiguity, and privacy concerns. Success demands more than technological investment; it requires a holistic governance model that combines robust data stewardship, cross-functional collaboration, and continuous human oversight. Ethical considerations must be embedded into AI design, implementation, and use—ensuring that the pursuit of automation does not come at the expense of accountability or fairness.
Looking ahead, the trajectory of AI in finance points toward even more advanced capabilities. Future innovations will likely include autonomous audit agents, AI-powered scenario planning tools, generative AI for dynamic financial modeling, and deeply integrated cognitive finance assistants within enterprise resource planning platforms. These systems will not only detect and correct errors—they will anticipate them, explain their origins, and simulate corrective outcomes across complex global operations.
The concept of a “zero-error” financial environment may once have seemed aspirational, but AI brings this vision within reach. As AI continues to mature, the standard for financial operations will inevitably shift toward real-time precision, embedded compliance, and intelligent oversight. Enterprises that act now—investing in infrastructure, talent, and ethical frameworks—will be best positioned to lead in this new era of financial integrity.
In conclusion, the AI revolution in financial error detection and management is not a temporary trend but a structural transformation. For CFOs, controllers, auditors, and compliance professionals, the imperative is clear: embrace AI as a strategic partner, not just a technological solution. Doing so will not only mitigate risk and drive efficiency but will also prepare organizations for a future where financial operations are not only faster and smarter—but fundamentally more reliable.
Reference
- PwC – How AI is transforming the finance function
https://www.pwc.com/gx/en/services/audit-assurance/corporate-reporting/ai-in-finance.html - Deloitte – Cognitive technologies in finance
https://www2.deloitte.com/insights/us/en/focus/cognitive-technologies/cognitive-technologies-in-finance.html - KPMG – AI in Financial Reporting
https://home.kpmg/xx/en/home/insights/ai-in-financial-reporting.html - McKinsey – How CFOs can harness AI to manage risk
https://www.mckinsey.com/business-functions/strategy-and-corporate-finance/our-insights/harnessing-ai-for-risk-management - EY – Financial crime risk management with AI
https://www.ey.com/en_gl/financial-services-emeia/using-ai-in-financial-crime-risk-management - IBM – Applying AI to finance: automation and insight
https://www.ibm.com/blogs/industries/ai-in-finance/ - Gartner – AI use cases in finance operations
https://www.gartner.com/en/articles/top-ai-use-cases-in-finance - Oracle – AI-Driven Finance Transformation
https://www.oracle.com/erp/financials/ai-in-finance/ - Forbes – AI and ML redefining accounting accuracy
https://www.forbes.com/sites/forbestechcouncil/ai-in-accounting/ - Harvard Business Review – AI’s growing role in finance
https://hbr.org/ai-in-financial-management