From Prompts to Purpose: How Generative and Agentic AI Are Powering the Next Digital Revolution
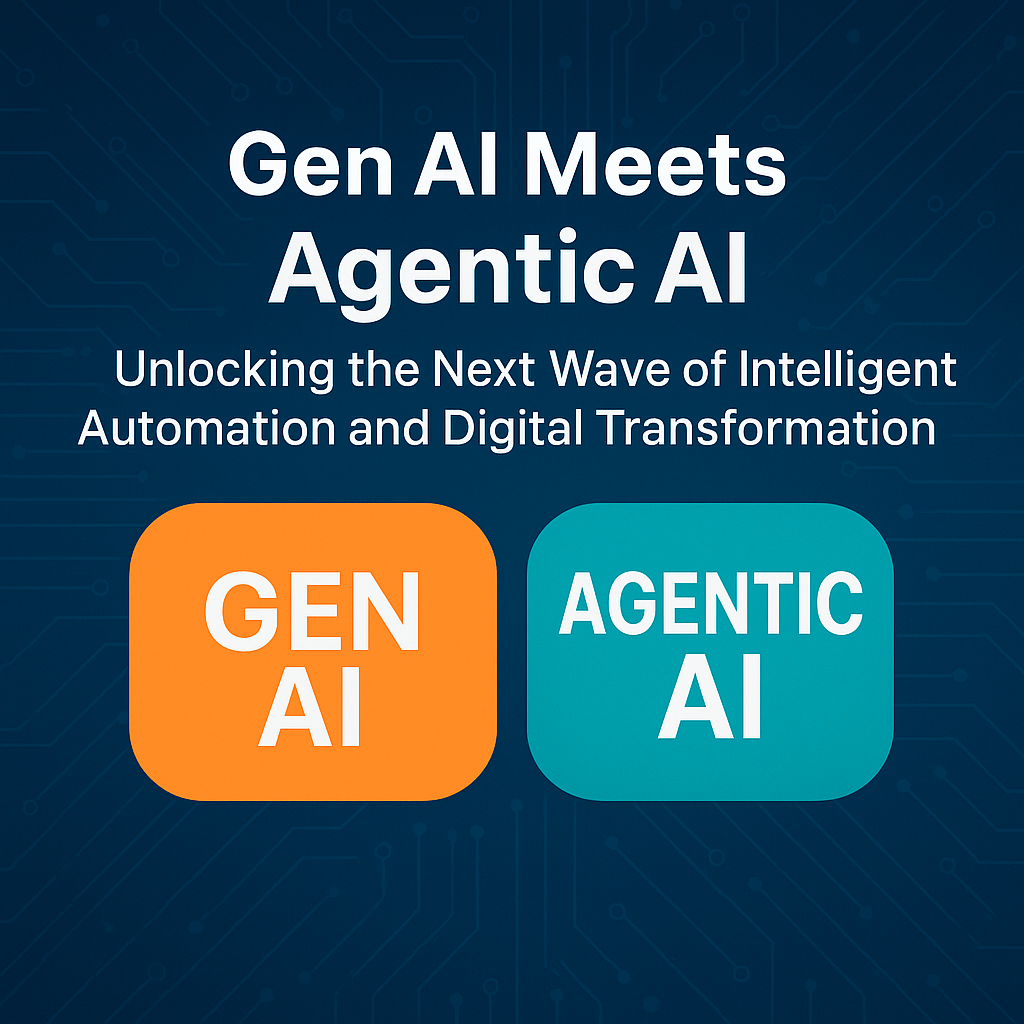
The rise of artificial intelligence over the past decade has been nothing short of revolutionary. However, the convergence of two powerful paradigms—Generative AI (Gen AI) and Agentic AI—is now ushering in an era that transcends earlier technological milestones. Gen AI, which enables machines to produce creative outputs such as text, images, music, and code, has already demonstrated its transformative potential across industries. On the other hand, Agentic AI, characterized by its autonomous, goal-driven behavior and decision-making capabilities, represents a paradigm shift toward machines that not only generate but also act, plan, and adapt in dynamic environments. Together, these two classes of AI technologies are poised to redefine the boundaries of innovation and unlock what can only be described as a billion applications and beyond.
As organizations across sectors—from healthcare to finance, from education to entertainment—rapidly adopt AI-driven tools, the distinction between passive software and active digital agents is becoming increasingly blurred. Generative AI models like GPT-4, DALL·E, and Stable Diffusion have already become household names, producing content that rivals human creativity. Meanwhile, autonomous agents such as Auto-GPT, Devin AI, and various orchestration frameworks are stepping into operational roles that traditionally required human intuition and sustained attention. These intelligent agents can browse the internet, write reports, analyze codebases, or even coordinate workflows across multiple software systems—without constant human intervention.
The most compelling aspect of this evolution is not merely the capability of these systems in isolation, but their composability. A Gen AI model, for instance, can be integrated with an Agentic AI system to form a composite that is both imaginative and functionally autonomous. Imagine a scenario in which a creative agent not only designs marketing campaigns using Gen AI but also deploys them, measures performance, and iterates on results—all autonomously. These are no longer hypothetical use cases. Enterprises and developers are actively experimenting with such solutions, often achieving astonishing levels of productivity and innovation.
The resulting explosion in AI capabilities has led to an accelerated diffusion of applications across every conceivable domain. Whether it is a startup automating customer onboarding through conversational agents, or a global pharmaceutical company using generative agents to streamline drug discovery, the breadth and depth of use cases are expanding at a pace that even the most optimistic forecasts struggle to accommodate. In fact, the idea of “a billion applications” is not hyperbole, but a reflection of the long-tail opportunities emerging from the democratization of AI capabilities.
Yet, this explosive growth also raises important questions about ethics, safety, regulation, and long-term societal impact. As Gen AI models generate increasingly realistic and persuasive content, and as Agentic AI systems take on greater operational autonomy, the stakes grow higher. Who is accountable when an agentic system makes an erroneous decision in a medical setting? How do we ensure that generative outputs do not propagate bias or misinformation? And perhaps most crucially, how do we design governance frameworks that balance innovation with oversight?
In this blog post, we will explore the fundamental differences and synergies between Gen AI and Agentic AI, examine the infrastructure and ecosystems enabling their rapid proliferation, and analyze their transformative impact across industries. We will also look toward the future to assess both the opportunities and the challenges that lie ahead. As we stand on the threshold of a new technological epoch, one thing is clear: Gen AI and Agentic AI are not just tools—they are catalysts for a radical rethinking of how humans and machines collaborate, create, and coexist.
Defining Gen AI and Agentic AI
As artificial intelligence becomes increasingly embedded in everyday systems, it is essential to delineate between its distinct but often overlapping paradigms. Among these, Generative AI (Gen AI) and Agentic AI have emerged as two foundational approaches shaping the future of intelligent systems. Though often used interchangeably in public discourse, these paradigms are conceptually and functionally different. Understanding their core mechanisms, capabilities, limitations, and points of convergence is critical to comprehending the AI-driven transformation unfolding across industries.
Generative AI: Creating from Data
Generative AI refers to a class of artificial intelligence models capable of producing novel content based on learned patterns from existing data. These models utilize advanced machine learning techniques, particularly deep learning architectures such as transformer networks and diffusion models, to synthesize outputs that range from natural language and code to images, video, and music. At the heart of Gen AI lies the concept of statistical pattern recognition—the ability to internalize massive datasets and recreate new, coherent artifacts in a similar style.
The most widely known examples of Gen AI include OpenAI’s GPT series, Anthropic’s Claude, Google DeepMind’s Gemini, Meta’s LLaMA, Mistral, and Cohere’s Command R, among others. These large language models (LLMs) are capable of performing complex natural language tasks such as summarization, translation, ideation, and even reasoning when supplemented with structured prompts. On the visual side, DALL·E, Midjourney, and Stable Diffusion can generate high-fidelity images from textual descriptions. In music and audio generation, tools like Suno, Udio, and Lyria represent the cutting edge.
One of the most remarkable achievements of Gen AI is its ability to democratize creativity. Tasks that once required professional expertise—such as composing a symphony, designing a logo, or writing functional software—can now be executed with human guidance and machine execution. This transformative accessibility has implications far beyond convenience; it enables individuals and small teams to compete at a scale previously reserved for well-resourced enterprises.
Yet, Gen AI is not without limitations. Despite their prowess in generation, these models operate within a stateless framework—they lack persistent memory, self-awareness, and intrinsic motivation. They generate outputs only in response to prompts and are unaware of any goals beyond the immediate context. Moreover, they are prone to issues such as hallucination (producing false but plausible-sounding content), bias (reflecting skewed data), and brittleness (failing outside of trained distributions).
Agentic AI: Acting with Purpose
In contrast to Gen AI, Agentic AI emphasizes autonomy, persistence, and goal orientation. Agentic systems are designed to operate as digital agents—entities that perceive their environment, make decisions, plan actions, and execute them with minimal or no human oversight. These systems are not simply reactive; they are proactive in nature, capable of adjusting strategies in response to dynamic conditions and pursuing long-term objectives.
Agentic AI systems often comprise a combination of modules, including reasoning engines, tool-use capabilities, memory modules, and planning algorithms. While they may integrate Gen AI components for communication or generation, their core value lies in their capacity for autonomous task completion. Tools like Auto-GPT, BabyAGI, OpenDevin, Devin AI by Cognition, and AutoGen represent early but potent examples of this paradigm. These systems can autonomously decompose tasks, execute multi-step workflows, invoke APIs, and interact with external systems such as databases, web browsers, and file systems.
One of the defining characteristics of Agentic AI is its stateful behavior. Unlike Gen AI, which responds statically to a given prompt, agentic systems maintain context over time. This allows them to build memory, adjust based on outcomes, and “learn” within the boundaries of a task session. This persistence enables them to simulate forms of cognitive continuity—an essential prerequisite for long-term automation in enterprise, scientific, and operational domains.
Moreover, Agentic AI introduces new opportunities for multi-agent collaboration, where different agents with specialized roles interact to solve complex problems. For instance, in software development, a project manager agent might assign tasks to developer agents, which in turn consult documentation agents and test agents. This kind of orchestration mimics real-world organizational behavior and suggests a future where software systems operate as dynamic teams of AI collaborators.
Despite their promise, Agentic AI systems face considerable challenges. The complexity of autonomous operation makes them vulnerable to cascading errors, unpredictable behaviors, and security risks. Designing robust reward functions, managing memory integrity, and ensuring reliable tool invocation are non-trivial problems that remain active areas of research. Furthermore, ethical concerns—particularly around agency, accountability, and intent—are magnified when systems begin to act with apparent autonomy.
Complementarity and Convergence
While Gen AI and Agentic AI are conceptually distinct, they are increasingly being integrated to form more capable systems. A Gen AI model might provide the linguistic or creative capabilities within an agentic architecture, effectively serving as a cognitive engine for the agent. Conversely, Agentic AI adds layers of persistence, task management, and execution control to otherwise stateless generative systems.
Consider the example of an autonomous legal assistant. A Gen AI model can draft contracts, summarize regulations, or answer questions. But with an agentic layer, this assistant can autonomously monitor changes in legislation, alert relevant stakeholders, recommend policy updates, and even initiate compliance checks—without needing to be manually prompted. In this hybrid model, Gen AI supplies the intelligence, and Agentic AI delivers the initiative.
This synthesis is driving a new generation of agentic copilots and workflow orchestrators, increasingly found in platforms like Microsoft Copilot, Notion AI, and enterprise SaaS integrations. By coupling generation with action, these systems are capable not just of augmenting human productivity, but also of replacing certain categories of human labor entirely.
To better understand the distinctions and synergies, the following table provides a high-level comparison:
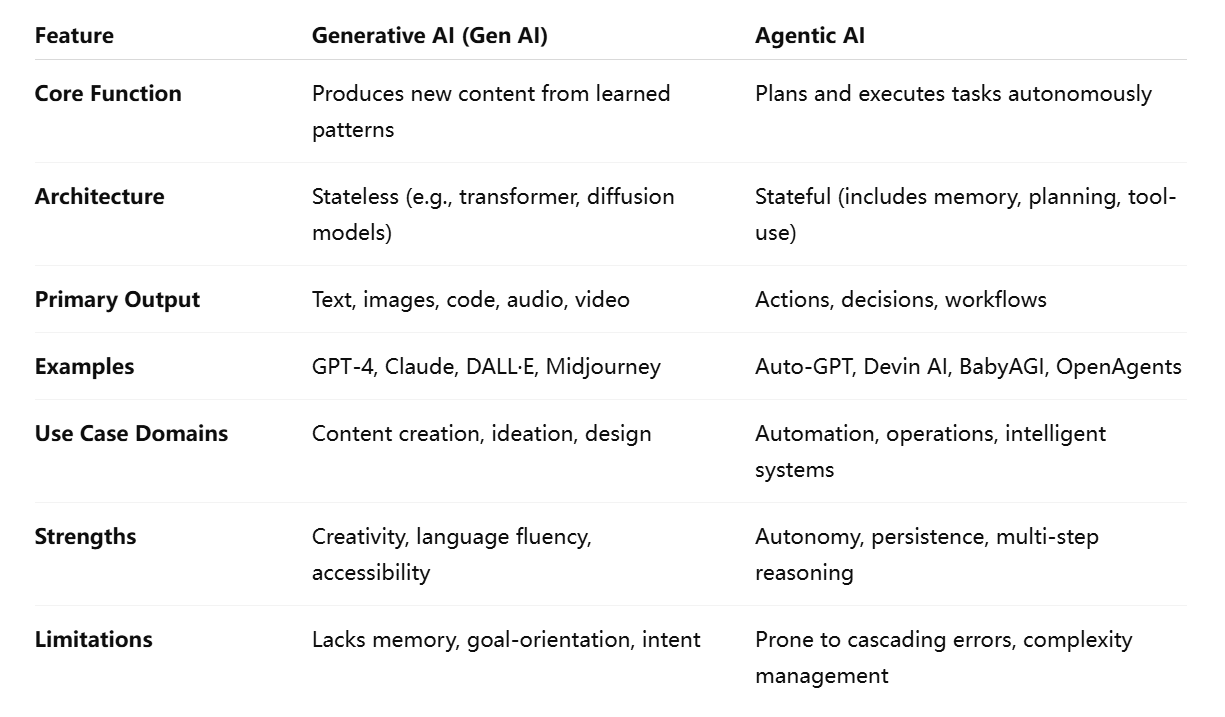
In conclusion, Generative AI and Agentic AI represent complementary pillars of next-generation artificial intelligence. While Gen AI dazzles with creativity and fluency, Agentic AI impresses with autonomy and adaptability. Their convergence is not merely an architectural convenience—it is the foundation of a future in which machines not only think and create but also act and collaborate. The implications of this synergy will become increasingly evident as we explore the vast landscape of their applications in the next section.
A Billion Applications: Sector-by-Sector Transformation
The fusion of Generative AI and Agentic AI has ushered in a technological transformation that is rapidly cascading across industries. With capabilities that span creative content generation, decision-making, autonomous task execution, and collaborative interaction, these AI systems are fundamentally altering the structure of work, the delivery of services, and the nature of innovation. What was once the domain of science fiction—machines reasoning, creating, and acting independently—is now being deployed in real-world settings with tangible outcomes. This section provides a comprehensive analysis of how Gen AI and Agentic AI are reshaping key sectors, enabling what can rightfully be called a billion applications.
Enterprise and Productivity
In the corporate landscape, the adoption of Gen AI and Agentic AI is revolutionizing workflows, knowledge management, and operational efficiency. Generative models are used to draft emails, generate reports, produce market analyses, and support strategic decision-making. Tools like Microsoft Copilot, Google Gemini for Workspace, and Salesforce Einstein GPT integrate Gen AI into commonly used enterprise platforms, empowering employees with enhanced cognitive support.
Agentic AI, meanwhile, is facilitating autonomous business operations. Digital agents can schedule meetings, respond to routine customer service requests, monitor data dashboards, and escalate anomalies without human supervision. More advanced implementations see agents coordinating entire processes: for instance, conducting due diligence in mergers and acquisitions, managing inventory procurement, or orchestrating project delivery timelines across distributed teams.
Furthermore, enterprises are increasingly deploying multi-agent systems to simulate entire departments, where specialized agents communicate and collaborate in a manner reminiscent of human organizational structures. This development signals a move toward AI-native enterprise architectures—organizations designed around and operated by intelligent systems.
Creative Industries
Generative AI has been a game-changer for the creative economy. Artists, designers, musicians, filmmakers, and writers now have access to tools that expand the boundaries of imagination and productivity. Platforms like Midjourney, DALL·E, and Runway allow for rapid concept art generation, visual effects editing, and design prototyping. Musicians use Suno, Lyria, and Udio to generate lyrics, compose scores, and experiment with new sonic textures. Authors can co-write novels or brainstorm plots with language models such as GPT-4 or Claude.
When enhanced by agentic capabilities, these tools evolve into creative agents—digital collaborators that proactively contribute ideas, revise content, and align creative outputs with target audiences or brand identities. For example, in marketing, an agent can generate content across multiple channels (blogs, social media, emails), analyze engagement metrics, and iteratively optimize messaging strategies.
In the gaming industry, Gen AI and Agentic AI enable dynamic world-building and real-time story generation. Non-playable characters (NPCs) can be endowed with personalities, backstories, and decision-making autonomy, creating richer and more immersive player experiences. This not only reduces development time and cost but also elevates the narrative complexity of digital entertainment.
Software Development
Perhaps no domain has seen as radical a transformation from AI integration as software development. Gen AI tools such as GitHub Copilot, Replit Ghostwriter, and Amazon CodeWhisperer assist developers in writing, debugging, and optimizing code in real time. These models can understand context, generate boilerplate code, suggest algorithmic improvements, and even explain complex logic for human comprehension.
Agentic AI systems take this further by becoming autonomous developers. Devin AI, released by Cognition, is an early example of a coding agent capable of full-cycle software engineering. It can understand high-level product requirements, plan development tasks, write production-ready code, perform unit testing, and deploy the application—all with minimal human oversight.
Moreover, agent-based developer environments are enabling continuous integration and delivery pipelines powered by intelligent agents that monitor dependencies, update codebases, and resolve conflicts. This leads to significant improvements in agility and productivity while reducing the cognitive burden on human developers.
Healthcare and Life Sciences
In healthcare, the impact of Gen AI and Agentic AI is both profound and multifaceted. On the generative side, AI models are used to create synthetic medical data for research, generate clinical notes, draft radiology reports, and assist in medical education. Language models trained on biomedical corpora (e.g., PubMedGPT, Med-PaLM) provide accurate, context-sensitive insights to clinicians and researchers.
Agentic AI systems serve as diagnostic assistants, treatment coordinators, and care management agents. These agents can monitor patient data in real time, detect anomalies, suggest interventions, and coordinate care across interdisciplinary teams. In drug discovery, agentic workflows automate high-throughput screening, candidate selection, and toxicology assessment, significantly accelerating R&D cycles.
AI agents are also playing a crucial role in public health management. They can forecast disease outbreaks, analyze epidemiological trends, and support vaccination logistics. During global crises such as the COVID-19 pandemic, the fusion of generative and agentic models demonstrated their utility in modeling viral mutations, optimizing supply chains, and disseminating critical information to the public.
Education and Training
Education is being fundamentally reshaped by intelligent tutoring systems powered by Gen AI and Agentic AI. Gen AI models personalize learning content, generate practice exercises, and provide real-time feedback. Teachers use these models to create customized lesson plans, adapt materials for diverse learners, and translate curriculum into multiple languages.
Agentic systems elevate this by functioning as virtual tutors, capable of managing individualized learning pathways. These agents monitor learner progress, identify knowledge gaps, suggest remedial content, and even adjust pedagogical strategies based on student responses. In higher education and vocational training, AI agents support skills development in programming, data science, and language acquisition.
Moreover, multi-modal capabilities are expanding access to education for students with disabilities. Voice-activated agents, AI-powered sign language interpreters, and tactile-responsive content generators are closing longstanding accessibility gaps.
Finance and Legal Services
The highly structured and information-dense nature of finance and law makes these sectors ideal for Gen AI and Agentic AI applications. In finance, Gen AI generates market reports, financial summaries, and investment memos. Agentic AI systems operate as robo-advisors, fraud detection monitors, and compliance officers. These agents analyze large volumes of data in real time, execute trades based on strategic objectives, and ensure adherence to regulatory frameworks.
In legal services, Gen AI assists with drafting contracts, summarizing case law, and interpreting statutes. Legal agents can autonomously review documents for compliance, flag potential risks, and even suggest negotiation strategies. They streamline operations in law firms, reduce costs for clients, and enhance access to justice in under-resourced communities.
Startups and established firms alike are building agent-powered legaltech platforms capable of handling end-to-end case management—from intake and document discovery to resolution and billing—transforming legal workflows into intelligent services.
Manufacturing, Logistics, and Robotics
In industrial sectors, Agentic AI is taking automation to new heights. Intelligent agents manage supply chains, forecast demand, and optimize logistics routes. In factories, Gen AI is used for designing parts, simulating production outcomes, and generating equipment maintenance reports.
Agentic systems take on operational roles such as inventory monitoring, order fulfillment, and predictive maintenance. These agents can initiate actions autonomously based on sensor data, interact with enterprise resource planning (ERP) systems, and coordinate with human workers and physical robots on the shop floor.
In robotics, AI agents are embedded in mobile units to execute complex tasks in warehousing, mining, agriculture, and construction. These agents learn from experience, adapt to changing conditions, and collaborate with other robots to achieve collective objectives, laying the foundation for agentic swarm intelligence in real-world settings.
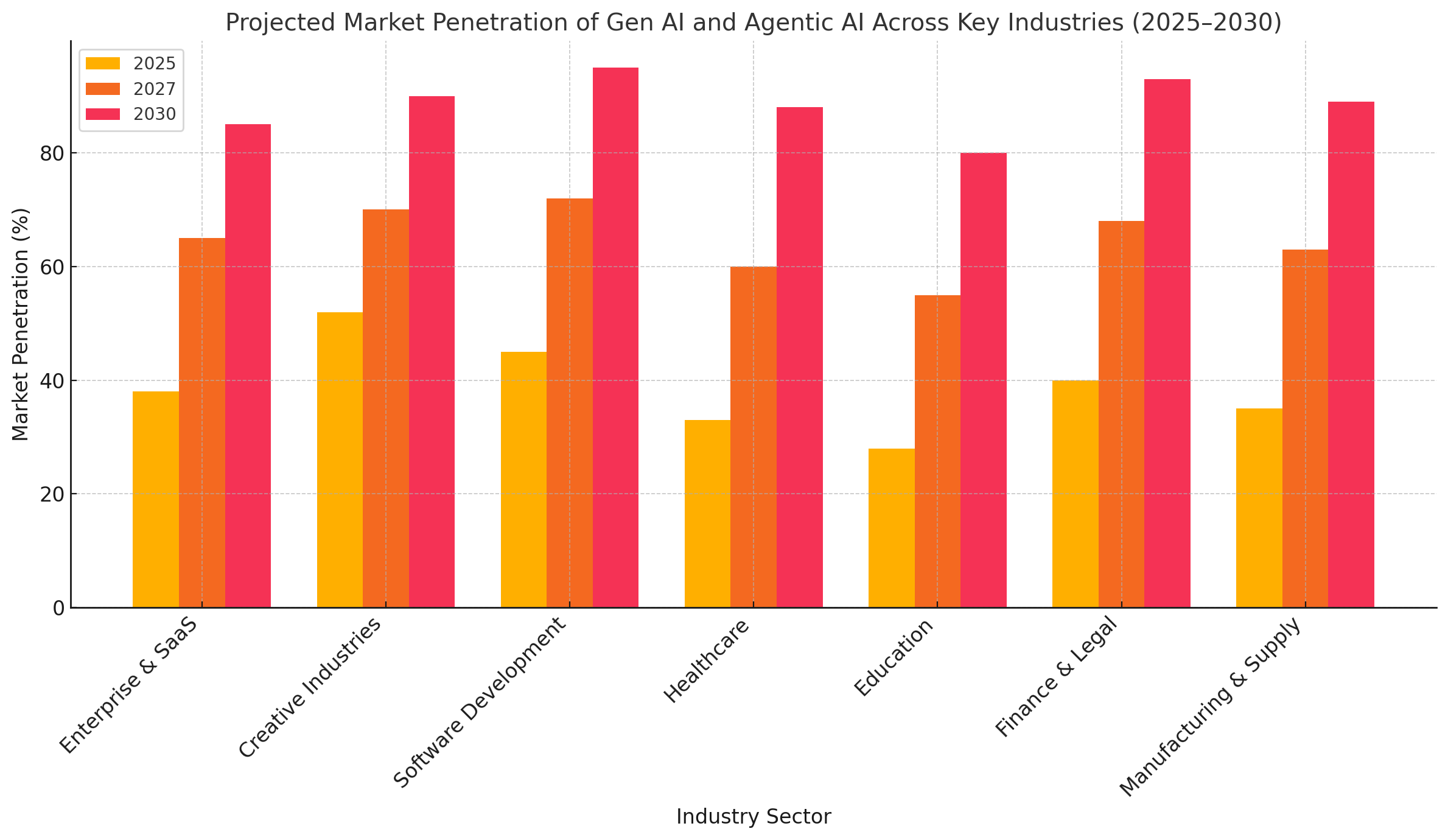
The sectoral impact of Gen AI and Agentic AI is not merely additive—it is exponential. These technologies act as force multipliers, enabling organizations to scale capabilities without proportionally increasing resources. As adoption accelerates and infrastructure matures, we are witnessing the emergence of an intelligent ecosystem where machines not only support but also lead innovation. The billion applications are not aspirational—they are inevitable.
Infrastructure and Ecosystems Powering Agentic Intelligence
The growing integration of Generative AI and Agentic AI into mainstream applications hinges not only on breakthroughs in model architecture and reasoning capabilities but also on the evolution of a robust technological foundation. As these AI systems scale from isolated tools to full-scale autonomous agents capable of interfacing with digital environments, the underlying infrastructure—ranging from compute and memory to data orchestration and system interoperability—becomes a decisive factor in their utility and proliferation. This section provides an in-depth exploration of the critical components enabling the development, deployment, and scaling of agentic intelligence, along with the burgeoning ecosystems supporting it.
Compute, Memory, and Latency: Foundations of Intelligence
Agentic systems, unlike traditional stateless Gen AI models, must retain and process long-context information, remember intermediate steps, invoke external tools, and make decisions based on cumulative knowledge. These requirements impose significantly greater demands on compute resources, contextual memory, and low-latency inference.
Modern agentic systems often depend on high-performance GPUs and AI accelerators for real-time operation, particularly when engaging in multi-turn dialogue, code analysis, or interactive tool use. While foundation models like GPT-4 and Claude operate in the cloud, emerging trends in edge AI suggest a future where local agentic intelligence becomes feasible in mobile devices, autonomous vehicles, and IoT ecosystems.
Memory is another critical aspect. Whereas traditional Gen AI models operate within a sliding window of tokens, agentic systems benefit from external memory architectures, such as vector databases, document retrievers, and episodic memory stores. These components allow agents to retrieve relevant historical data, maintain task state across sessions, and dynamically update knowledge as they operate.
To ensure seamless interactivity, latency must be minimized. Techniques such as speculative decoding, parameter-efficient fine-tuning, and asynchronous agent execution are being actively explored to accelerate response times while preserving accuracy. The convergence of fast inference with persistent memory and adaptive compute allocation is foundational to the effective deployment of autonomous agents.
Tool Use and API Integration: Expanding Agent Capabilities
One of the distinguishing features of Agentic AI is its ability to interact with external systems through tools, APIs, and plugins. These capabilities transform otherwise passive models into powerful actors capable of executing commands, retrieving information, and performing operations on real-world systems.
Agents may invoke web browsers to gather current information, interact with file systems to store or read data, query databases to retrieve structured content, or operate enterprise software via API calls. This modular architecture enables agents to scale their functionality beyond model limitations by outsourcing tasks to specialized tools.
Frameworks such as LangChain, AutoGen, CrewAI, MetaGPT, and Semantic Kernel have emerged to streamline this capability. These tools provide abstractions that allow developers to define tasks, allocate tools, manage memory, and compose complex workflows. For instance, an agent tasked with financial analysis might use a language model for interpreting news, a vector database for historical data retrieval, and a spreadsheet tool for generating charts—seamlessly combining diverse resources into coherent action plans.
Moreover, with the rise of toolformer-style models—models trained to autonomously decide when and how to use tools—we are moving toward a more integrated paradigm where agentic behavior is learned rather than manually scripted. This will be instrumental in enabling agents to generalize across domains with minimal human input.
Orchestration and Multi-Agent Collaboration
While single-agent systems offer utility in narrow tasks, real-world challenges often require coordination across multiple agents with specialized roles. Multi-agent systems (MAS) are designed to emulate collaborative behavior, where individual agents communicate, delegate, and resolve conflicts toward a shared objective. This mirrors human organizational structures and unlocks scalability in complex environments.
For instance, in a software development workflow, distinct agents may represent roles such as product manager, developer, tester, and release engineer. These agents interact over shared context, negotiate task boundaries, and synchronize their actions to deliver a working product. This form of orchestration requires sophisticated messaging protocols, shared memory spaces, and role-based planning logic.
Ecosystems such as OpenAgents, SuperAgent, and CrewAI facilitate the creation of multi-agent environments with clearly defined schemas for communication, resource access, and task division. These systems also offer inter-agent reasoning layers, allowing agents to evaluate each other's outputs, challenge assumptions, or escalate problems to higher-level agents or humans.
In enterprise settings, this collaborative model supports dynamic workflows where agents can be spun up, reconfigured, or retired based on changing requirements. As such, orchestration is not merely a technical feature—it is a foundational strategy for deploying intelligent automation at scale.
Vector Databases and Long-Term Memory
The ephemeral memory of traditional Gen AI models poses a major limitation for agentic applications. In contrast, persistent, retrievable memory is crucial for agents that must maintain context over long durations, recall previous interactions, or integrate external knowledge dynamically.
Vector databases—such as Pinecone, Weaviate, Chroma, and FAISS—solve this challenge by storing semantic embeddings of documents, conversations, or artifacts. When queried, these databases return the most relevant results based on vector similarity rather than keyword matching, enabling agents to reason more effectively over unstructured data.
In practice, this allows agents to:
- Retrieve past decisions to avoid repeating mistakes.
- Store procedural knowledge for recurring tasks.
- Integrate enterprise documentation and FAQs.
- Build user profiles and preferences for personalized interaction.
Integration with retrieval-augmented generation (RAG) pipelines further enhances the intelligence of Gen AI components, enabling fact-based generation informed by long-term memory. These capabilities are particularly critical in customer service, legal analysis, technical support, and any domain requiring continuity across sessions.
Open Source vs Proprietary Ecosystems
The rapid evolution of Agentic AI is being shaped by both open-source innovation and proprietary investment. On the open-source side, projects like Auto-GPT, LangChain, and GPT-Engineer have democratized access to sophisticated agent architectures, allowing developers to experiment, iterate, and contribute to a growing knowledge base. These projects foster transparency, community involvement, and modular extensibility.
Conversely, proprietary ecosystems—led by companies such as OpenAI, Microsoft, Google, and Amazon—offer tightly integrated platforms with optimized performance, enterprise-grade security, and broader service integration. Products such as Microsoft Copilot, Google Gemini for Workspace, and AWS Bedrock Agents reflect this commercial orientation, combining large models, plugin frameworks, and orchestration engines under unified interfaces.
Each ecosystem offers trade-offs. Open-source platforms encourage rapid prototyping and customization but may face challenges in reliability, maintenance, and compliance. Proprietary platforms offer greater robustness and support but can limit extensibility and innovation due to closed-source constraints. As the ecosystem matures, hybrid models may emerge where open components interface with commercial infrastructure to provide the best of both worlds.
Below is a comparative visualization of the AI infrastructure stack that supports Gen AI and Agentic AI deployments:
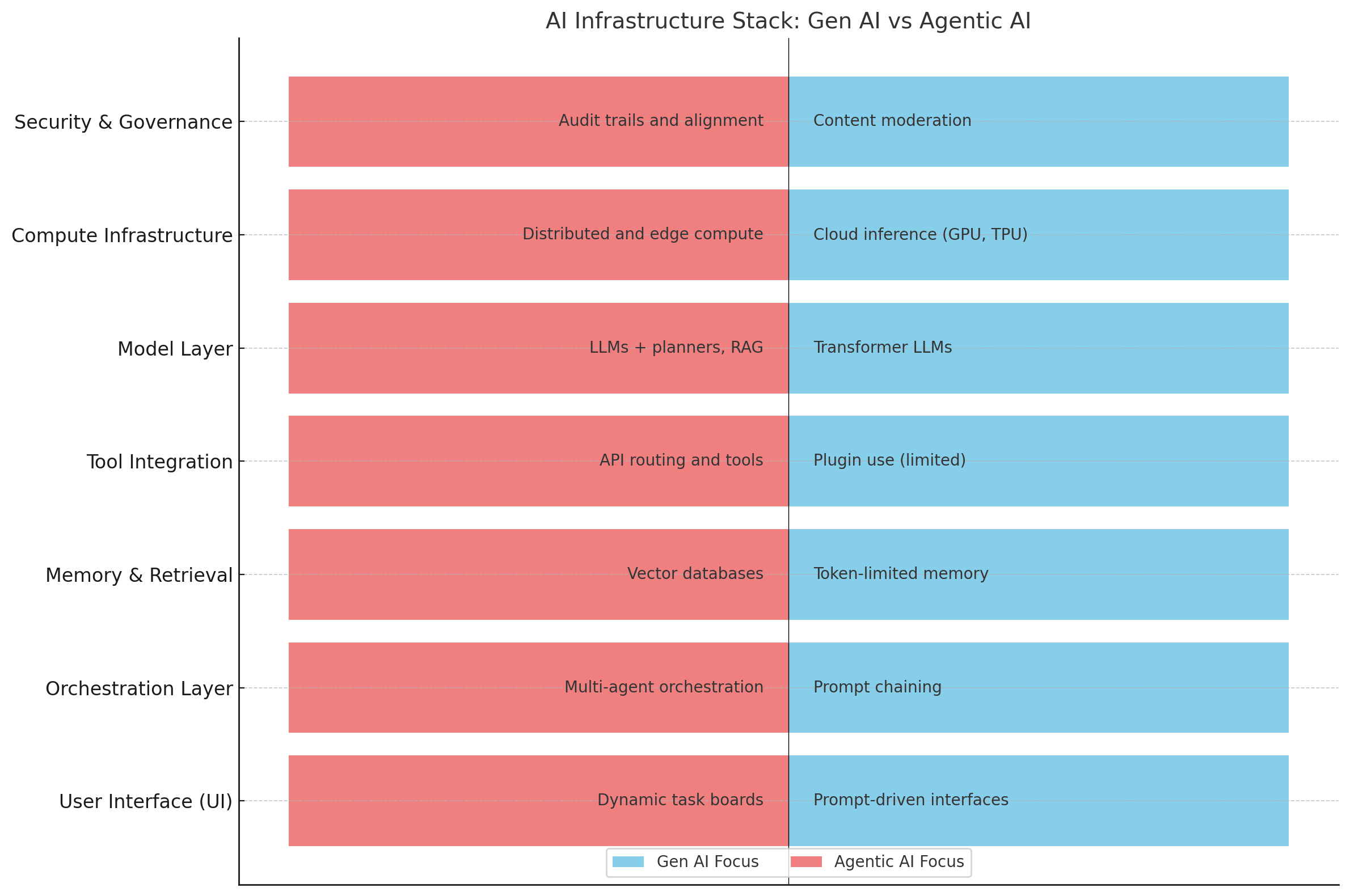
This layered structure illustrates how Agentic AI requires a more complex and modular stack to enable autonomy, adaptability, and collaboration. The orchestration and memory layers in particular are critical differentiators, representing the cognitive continuity and goal-directed behavior that distinguish agentic systems from stateless generators.
In sum, the infrastructure powering Agentic AI is both an enabler and a bottleneck. While current advances have opened the door to highly capable agents, further progress depends on scaling compute efficiency, refining orchestration logic, standardizing memory models, and ensuring security and governance. As developers, enterprises, and policymakers align their efforts, a more intelligent, interactive, and autonomous digital landscape is beginning to take shape—one where Agentic AI is not merely reactive, but proactive, persistent, and collaborative.
Looking Ahead: Risks, Ethics, and the Future of Autonomous Creation
As the integration of Generative AI and Agentic AI accelerates across industries and consumer applications, it is imperative to assess not only the opportunities but also the complex challenges these technologies present. With unprecedented capabilities comes the responsibility to navigate ethical dilemmas, societal impacts, and regulatory imperatives. This final section explores the future trajectory of autonomous systems, highlighting the risks they pose, the frameworks required for their governance, and the broader implications for human-AI collaboration.
The Risks of Autonomy at Scale
Autonomous AI agents, while offering the promise of efficiency and scalability, also introduce layers of risk that extend beyond those associated with static generative models. These risks are particularly acute when agents are given the capacity to act on external systems, make decisions with real-world consequences, or interact with other agents.
One of the most pressing concerns is misalignment—when an agent’s actions deviate from human intentions due to faulty instructions, misunderstood context, or misoptimized objectives. In highly sensitive sectors such as finance, medicine, or infrastructure, such deviations can cause operational failures, financial losses, or even loss of life. As agents begin to self-assign tasks and adapt strategies, ensuring that their behavior remains safe and predictable becomes increasingly difficult.
Additionally, compounding errors in multi-step or multi-agent workflows represent a significant vulnerability. A minor error in one agent’s output can cascade through a chain of decisions, leading to unintended or even harmful outcomes. Unlike traditional software bugs, these failures may emerge from dynamic interactions and may be difficult to replicate or trace.
Moreover, the opacity of agentic systems—especially when they rely on large black-box models—hampers auditing and accountability. Determining why an agent chose a particular course of action may be infeasible without full visibility into its memory, planning logic, and decision-making parameters. This raises fundamental questions about responsibility and liability in automated environments.
Ethical Challenges and Societal Impact
Beyond technical risks, Gen AI and Agentic AI introduce profound ethical considerations. One major concern is the devaluation of human labor and creativity. As agents become capable of performing knowledge work, content creation, and decision-making autonomously, millions of jobs may face transformation or displacement. This shift could exacerbate economic inequality unless offset by proactive workforce adaptation, retraining programs, and policies promoting inclusive innovation.
The blurring of authorship is another ethical dilemma. When content—be it a novel, a symphony, or a legal brief—is generated by an AI agent, questions arise around intellectual property, originality, and ownership. If an autonomous system is responsible for a novel insight or artistic expression, does it merit credit? Or does credit belong solely to its developer or operator?
There are also concerns regarding privacy and surveillance. Agents embedded in enterprise systems or personal environments collect vast amounts of behavioral and contextual data to function effectively. Without strict data governance, this could lead to invasive profiling, unauthorized data retention, or discriminatory outcomes.
Lastly, value alignment becomes especially important in agentic systems tasked with open-ended goals. Unlike Gen AI models that respond to specific prompts, agents must weigh trade-offs and choose between competing objectives. Embedding human ethics into these decision processes—especially across cultural and societal boundaries—remains an open research challenge.
Regulatory and Governance Imperatives
Given the societal scale and transformative power of Gen AI and Agentic AI, there is a growing consensus on the need for robust regulatory frameworks. However, current governance structures are often ill-equipped to handle the speed and complexity of AI development.
In the United States, regulatory discussions have centered around transparency, safety testing, and alignment standards, while the European Union’s AI Act proposes tiered risk-based classifications with specific obligations for high-risk applications. Other countries, including China, Canada, and the UK, are exploring various models of AI oversight through national agencies and multilateral forums.
For agentic systems in particular, governance must go beyond content moderation and model transparency. It must address:
- Agent capability disclosures (what can the agent do, under what constraints)
- Auditability of decisions and memory logs
- Control mechanisms (kill switches, permission settings, escalation paths)
- Interoperability standards for multi-agent collaboration
- Data usage policies including consent, access, and retention protocols
Industry initiatives—such as the Frontier Model Forum and Open Source AI Consortia—are emerging to provide voluntary guardrails, but these efforts must eventually harmonize with enforceable global standards to ensure safety, fairness, and accountability at scale.
The Human-AI Interface: Collaboration, Not Competition
Despite fears of job displacement or creative obsolescence, the most sustainable path forward lies in human-AI collaboration rather than competition. Gen AI and Agentic AI, when properly integrated into human workflows, can serve as powerful augmenters of human capability.
Consider the case of a medical researcher who uses a generative model to analyze vast clinical trial data while an agentic assistant identifies relevant literature, tracks ongoing trials, and prepares submission-ready reports. In this model, the human retains strategic oversight while the AI accelerates execution and enhances insight.
Similarly, in education, a teacher can oversee a classroom where AI agents deliver personalized instruction, track student progress, and provide real-time recommendations. The teacher's role shifts from content delivery to mentorship and emotional support—areas where human empathy remains irreplaceable.
The long-term vision should emphasize complementarity: designing AI systems that amplify human judgment, creativity, and values rather than supplant them. By aligning AI development with human-centered design principles, we can foster a future where agents serve as collaborators, not competitors.
Toward Artificial General Intelligence (AGI)
The convergence of Gen AI and Agentic AI may also accelerate progress toward Artificial General Intelligence (AGI)—systems capable of performing any cognitive task that a human can do. While still speculative, the current trajectory suggests that composite systems combining memory, planning, tool use, and generative reasoning may approximate increasingly general forms of intelligence.
AGI systems would not be limited to task-specific prompts or workflows but could autonomously set goals, reflect on outcomes, and improve over time. This raises existential questions about control, alignment, and coexistence.
Leading research labs have already begun implementing alignment research units, constitutional AI principles, and red teaming exercises to mitigate long-term risks. OpenAI, for example, has proposed a multi-tiered oversight model involving human feedback, automated evaluation, and third-party audits. However, these efforts remain in early stages and must be expanded as agentic capabilities grow more sophisticated.
Whether AGI arrives in years or decades, the preparatory work must begin now. As the boundary between tool and collaborator blurs, societies will need to engage in collective reflection on what roles we assign to machines—and how we preserve human agency in a world of autonomous intelligence.
In sum, the future of Gen AI and Agentic AI is not solely a technological journey but a societal one. It demands interdisciplinary cooperation, ethical foresight, and responsible stewardship. By confronting the risks head-on while embracing the promise of intelligent collaboration, we can chart a path that enhances human potential without compromising core values. The billion applications on the horizon must be guided not just by innovation, but by intentionality.
Conclusion
The convergence of Generative AI and Agentic AI marks a defining moment in the trajectory of artificial intelligence. As these two paradigms continue to evolve and integrate, they are reshaping not only the capabilities of machines but also the fabric of industries, societies, and human experience. From automating enterprise operations to democratizing creativity and transforming scientific research, the applications of Gen AI and Agentic AI are as vast as they are profound.
Yet, this era of exponential intelligence also brings complex challenges—technical, ethical, and societal—that demand thoughtful engagement. Ensuring safety, preserving human agency, and embedding transparency into AI systems are not optional safeguards; they are essential conditions for sustainable progress. The development of regulatory frameworks, ethical norms, and collaborative ecosystems will determine whether this revolution uplifts human potential or undermines it.
What lies ahead is not merely a technological frontier, but a human one. In embracing AI agents as partners rather than replacements, and in aligning innovation with shared values, we can unlock a future where intelligence is not centralized in machines alone but is distributed across human-AI networks that amplify creativity, compassion, and capability. A billion applications are just the beginning—the real transformation lies in how we choose to wield them.
References
- OpenAI – GPT-4 and AI Agents
https://openai.com/gpt-4 - Anthropic – Claude AI Overview
https://www.anthropic.com/index/claude - Cognition Labs – Devin AI Introduction
https://www.cognition-labs.com/devin - LangChain – Agentic Framework Documentation
https://docs.langchain.com/docs/components/agents - Microsoft Copilot – Enterprise AI Assistant
https://www.microsoft.com/en-us/microsoft-copilot - Pinecone – Vector Database for AI
https://www.pinecone.io - AutoGen by Microsoft – Multi-Agent Framework
https://github.com/microsoft/autogen - Google Gemini – AI for Workspace and More
https://deepmind.google/technologies/gemini - CrewAI – Multi-Agent Collaboration Toolkit
https://www.crewai.io - Hugging Face – Open Source AI Agent Repositories
https://huggingface.co