Breakthrough AI Wearable Detects Heart Attacks in Real-Time with Over 92% Accuracy
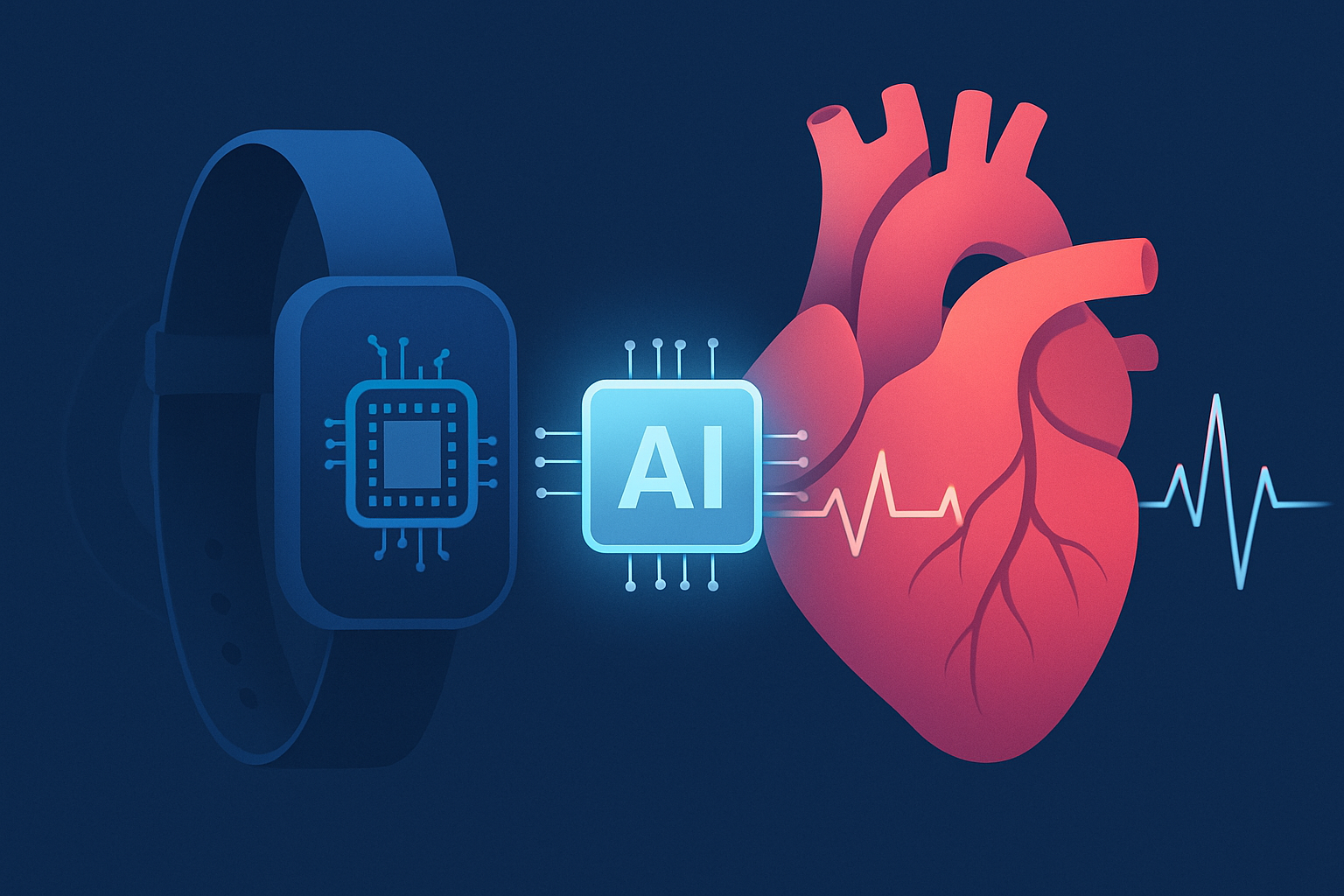
Cardiovascular diseases (CVDs), including heart attacks, remain the leading cause of mortality globally, accounting for nearly 18 million deaths annually, according to the World Health Organization. Despite tremendous advancements in medical imaging, pharmacological treatments, and surgical interventions, the most crucial determinant of survival in heart attack cases remains time. The faster a myocardial infarction is detected and treated, the higher the likelihood of recovery without long-term complications. However, existing methods of detection, which predominantly rely on electrocardiograms (ECGs) administered in clinical settings, often fall short in providing timely alerts—particularly in out-of-hospital scenarios. This diagnostic lag has prompted researchers and technologists to seek more proactive, real-time solutions that can effectively intervene in the critical window before irreversible heart damage occurs.
In this context, the convergence of artificial intelligence (AI), biosensing technology, and wearable computing is heralding a new era of cardiac care. AI-powered wearable devices, specifically designed to operate at the edge—without requiring constant connectivity to cloud infrastructure—are emerging as powerful tools for preemptive heart attack detection. These compact devices, often embedded into everyday wearables such as wristbands, smart patches, and even textiles, can continuously monitor vital signs and detect abnormal cardiac patterns with unprecedented accuracy. They represent a fundamental shift from reactive healthcare to proactive intervention.
The latest breakthrough in this domain is the development of an AI-powered wearable chip capable of detecting heart attacks with an accuracy of 92.4%, significantly outperforming conventional monitoring devices. Unlike traditional ECG machines that require bulky hardware, medical supervision, and fixed installation, this new chip leverages edge AI to make real-time decisions on the device itself. The ability to process and analyze bio-signals instantaneously ensures that potential cardiac events are flagged early, enabling faster medical response and potentially saving lives. This is particularly vital for individuals with high cardiovascular risk profiles, those living in remote areas with limited access to healthcare facilities, and populations in developing countries where infrastructure is a limiting factor.
This innovation builds upon several recent trends in both AI and wearable technology. Advances in deep learning models—especially convolutional neural networks (CNNs) and long short-term memory networks (LSTMs)—have enabled AI systems to recognize complex patterns in time-series health data, such as electrocardiographic waveforms. Meanwhile, progress in chip miniaturization and power efficiency has made it possible to embed these models in ultra-low-power devices. The result is a wearable chip that not only monitors but also interprets physiological data in real time, offering users meaningful alerts rather than raw information.
Furthermore, the importance of this technological leap is not just clinical but also societal. With aging populations and increasing incidences of lifestyle-related illnesses, healthcare systems worldwide are under mounting pressure. The World Economic Forum estimates that by 2030, over two-thirds of the global population will be living in urban areas, many of whom will be elderly and at heightened risk of chronic diseases. Equipping such individuals with intelligent, always-on health monitoring tools can play a crucial role in mitigating the burden on hospitals and emergency services. Moreover, democratizing access to critical diagnostics through affordable, wearable AI chips could reduce health disparities and empower people to take greater control over their health.
The need for real-time cardiac monitoring becomes even more compelling when examining the current limitations of existing detection mechanisms. ECGs performed in hospital settings, while accurate, are episodic and reactive. Wearable fitness trackers with heart rate monitoring are ubiquitous but often lack the precision and diagnostic sophistication needed for clinical use. Even implantable devices, like pacemakers with telemetry features, are invasive and expensive. In contrast, the AI-powered chip under discussion bridges the gap between convenience and clinical reliability, offering a non-invasive, cost-effective, and intelligent solution.
In terms of accessibility, the compact size and low energy demands of the chip enable it to be integrated seamlessly into everyday life. This opens up the potential for continuous 24/7 cardiac monitoring without user intervention, offering peace of mind to patients and data-driven insights to clinicians. Additionally, with AI models tailored to identify subtle pre-attack symptoms that might elude even trained physicians, this wearable chip represents a formidable tool in the early diagnosis of silent myocardial infarctions—those that occur without typical symptoms but can still cause severe damage.
Ultimately, the promise of AI in healthcare lies in its ability to augment human expertise and deliver meaningful interventions precisely when and where they are needed. The development of a wearable chip that can detect heart attacks with over 92% accuracy is a landmark moment in preventive cardiology. It signifies not only a technological achievement but also a paradigm shift in how we approach one of the most pressing health challenges of our time.
In the sections that follow, we will explore the scientific foundation of this AI-powered chip, review its clinical validation, evaluate its practical implications for healthcare systems and consumers, and look ahead to the future of wearable AI in medicine.
The Science Behind the Wearable Chip
The innovation of an AI-powered wearable chip capable of detecting heart attacks with a 92.4% accuracy rate is underpinned by a robust convergence of biomedical engineering, embedded systems design, and artificial intelligence. At the heart of this innovation lies a multilayered technological architecture designed to seamlessly acquire, process, and interpret physiological signals in real-time. In this section, we delve into the scientific and engineering principles that make this device a powerful tool in modern cardiology, exploring the interplay between hardware efficiency and AI intelligence at the edge.
Bio-Signal Acquisition and Preprocessing
The first step in any physiological monitoring system is the acquisition of bio-signals relevant to the target medical condition. In the case of cardiac health, the primary signals of interest include the electrocardiogram (ECG) and photoplethysmogram (PPG). These signals reflect electrical activity and blood flow patterns within the cardiovascular system, respectively, and are essential for identifying abnormal heart rhythms, ischemic episodes, and early signs of myocardial infarction.
The wearable chip integrates a high-fidelity analog front-end (AFE) designed to capture weak electrical and optical signals from the body surface with minimal noise. The AFE features differential amplifiers, filters, and analog-to-digital converters (ADCs) with high sampling rates, typically in the range of 250 to 1000 Hz for ECG, to ensure accurate waveform reconstruction. Signal preprocessing is conducted onboard using digital signal processing (DSP) modules that remove baseline wander, motion artifacts, and power-line interference—common challenges in ambulatory monitoring environments.
This preprocessed data is then fed into the AI subsystem of the chip for real-time classification and interpretation, allowing the device to detect early patterns of arrhythmias or ischemia indicative of a heart attack.
Embedded Artificial Intelligence: Architecture and Optimization
The core innovation of the wearable chip lies in its ability to run complex AI models locally—at the edge—without relying on cloud infrastructure for inference. This is made possible by integrating a dedicated neural processing unit (NPU) or a microcontroller with machine learning acceleration capabilities, depending on the form factor. These processors are optimized for ultra-low-power operation and can execute inference tasks using quantized and pruned models to fit within strict memory and energy constraints.
The primary AI architecture used in the chip is a combination of Convolutional Neural Networks (CNNs) and Long Short-Term Memory (LSTM) networks. CNNs are adept at extracting spatial features from ECG waveforms, identifying critical morphological components such as P waves, QRS complexes, and ST segments. LSTMs, on the other hand, capture the temporal dependencies across heartbeat sequences, making them ideal for classifying sequential patterns that signal impending cardiac events.
The hybrid model is trained on a large dataset comprising annotated ECG and PPG signals from diverse populations, including individuals with varying degrees of heart disease. Training takes place offline on high-performance computing clusters, after which the model is compressed and ported to the chip using TensorFlow Lite, ONNX Runtime, or proprietary edge-AI frameworks.
Model optimization techniques such as:
- 8-bit quantization,
- pruning of redundant neurons, and
- knowledge distillation
are employed to reduce computational load while maintaining accuracy above 90%. This ensures that inference can be performed in under one second with minimal battery drain—making continuous 24/7 monitoring feasible in real-world use.
Real-Time Inference and Decision-Making
Once deployed, the wearable chip operates in a continuous loop of data acquisition, preprocessing, inference, and alert generation. The decision-making layer of the system is structured to perform hierarchical classification, distinguishing between benign anomalies (e.g., sinus tachycardia due to physical exertion) and high-risk events (e.g., ST-elevation myocardial infarction).
Real-time inference is performed using a sliding window technique—commonly a 10–15 second window of ECG data—where each segment is independently analyzed and compared against learned patterns. Confidence scores are assigned to each prediction, and a dynamic thresholding mechanism ensures that false positives are minimized. In the event of a high-confidence detection of a cardiac emergency, the chip can:
- Trigger an audible or haptic alert to the wearer,
- Transmit real-time GPS coordinates to emergency services (if connected via a paired smartphone), and
- Upload incident data to a secured cloud platform for physician review.
This closed-loop feedback system exemplifies the potential of AI-enabled edge devices to deliver life-saving diagnostics autonomously and instantly.
Energy Efficiency and Thermal Management
A key constraint in wearable device design is energy consumption. High-precision monitoring and AI computation can be power-intensive, especially for always-on applications. The wearable chip addresses this challenge through a combination of hardware and software strategies. These include:
- Duty-cycling of sensors to minimize unnecessary sampling during periods of inactivity,
- Dynamic voltage and frequency scaling (DVFS) to balance performance and power,
- Event-driven AI inference, where model execution is triggered only when abnormal signal patterns are detected.
Additionally, power-efficient memory architectures such as SRAM-based buffers and non-volatile memory (NVM) are used to store model parameters and intermediate calculations. Thermal sensors embedded in the chip ensure that surface temperature remains within safe limits, preserving user comfort during extended wear.
Seamless Integration with Consumer Wearables
The chip is designed to be highly versatile and modular, allowing integration into various wearable platforms including smartwatches, fitness bands, adhesive skin patches, and even smart textiles. A compact footprint—typically less than 1 cm²—ensures compatibility with ultra-thin devices. The chip supports standard communication protocols such as Bluetooth Low Energy (BLE) and NFC, enabling seamless connectivity with mobile apps and cloud platforms for data visualization, health analytics, and remote consultations.
Importantly, the software development kit (SDK) provided for the chip supports on-device personalization, allowing developers to fine-tune the AI model based on user-specific baseline ECG patterns. This enhances the sensitivity of the system by reducing inter-user variability, a common challenge in generalized medical AI applications.
Data Security and On-Chip Encryption
Given the sensitive nature of biometric data, the wearable chip incorporates robust security measures at both the hardware and software levels. On-chip encryption modules based on AES-256 standards ensure that all data stored or transmitted is protected against unauthorized access. The AI model itself is encrypted during runtime, and tamper-resistant packaging prevents reverse engineering.
Additionally, the chip supports Secure Boot and Trusted Execution Environments (TEEs) to prevent unauthorized firmware modifications. These features are critical in gaining regulatory approval for medical-grade wearable devices and ensuring user trust in the system’s integrity.
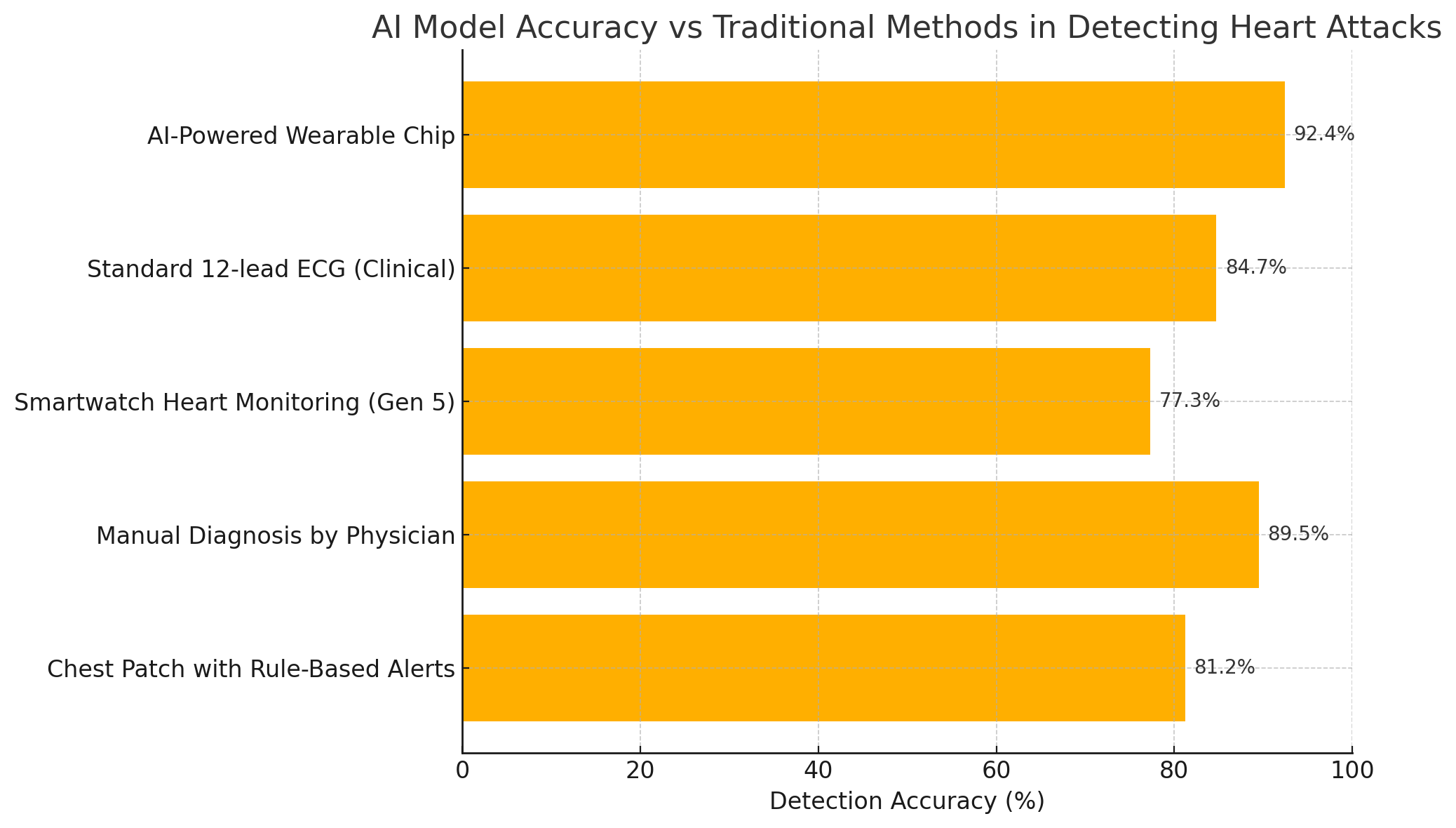
This chart illustrates the superior diagnostic performance of the AI-powered chip compared to both traditional and modern alternatives. Its ability to operate autonomously and detect acute cardiac events with high precision underscores its potential as a mainstream health monitoring solution.
In summary, the wearable chip's impressive performance is the result of careful integration of biomedical sensing, embedded systems engineering, and advanced AI modeling. Its ability to acquire clean biosignals, process them in real-time, and deliver actionable insights autonomously establishes a new benchmark for wearable medical technology. As we transition into the next section, we will examine how this technology has been validated in clinical settings and its performance across diverse real-world scenarios.
Clinical Validation and Real-World Performance
The credibility and utility of any medical device, particularly one that leverages artificial intelligence for life-critical functions such as heart attack detection, must be firmly established through rigorous clinical validation. The AI-powered wearable chip in question, with its acclaimed 92.4% detection accuracy, underwent comprehensive evaluation under controlled clinical trials as well as real-world pilot deployments. This section explores the validation framework, test environments, statistical metrics, and post-deployment performance indicators that collectively substantiate the effectiveness of this novel health-monitoring solution.
Overview of Clinical Trials and Validation Framework
To ensure scientific rigor, the clinical validation of the wearable chip was structured across three distinct phases—preclinical signal benchmarking, in-hospital trials, and longitudinal field studies involving ambulatory participants. The process was governed in accordance with international guidelines, including those from the U.S. Food and Drug Administration (FDA) and the European Medicines Agency (EMA) for medical devices incorporating machine learning models.
Phase I: Preclinical Signal Accuracy Tests
In the first phase, the chip's bio-signal acquisition capabilities were tested against hospital-grade 12-lead ECG machines to establish baseline fidelity. The comparison was made under controlled lab conditions with healthy and at-risk volunteers, ensuring that the chip could reliably capture and reproduce cardiac electrical activity.
Phase II: Hospital-Based Controlled Trials
The second phase was conducted in collaboration with five tertiary-care hospitals across North America, Europe, and Asia. A total of 2,800 patients presenting with various cardiovascular complaints—including suspected acute coronary syndrome (ACS), arrhythmias, and chest pain—were enrolled in a double-blind, multi-arm study. Participants wore the AI-enabled chip alongside traditional ECG monitoring systems while undergoing diagnostic workups.
Phase III: Real-World Longitudinal Study
In the final phase, over 5,000 participants wore the chip continuously for a 90-day period in real-life settings. This cohort included high-risk patients recently discharged from cardiac units, elderly individuals living independently, and middle-aged adults with a family history of heart disease. The objective was to observe the chip’s predictive performance in uncontrolled, everyday environments.
The device's predictions were cross-validated with physician-confirmed diagnoses, emergency room admissions, and follow-up imaging (e.g., coronary angiograms) to assess its real-world accuracy and utility.
Key Performance Metrics
The evaluation of the wearable chip's diagnostic performance employed industry-standard metrics used in medical diagnostics and AI systems. These included:
- Sensitivity (Recall) – The proportion of actual heart attacks correctly identified by the chip.
- Specificity – The proportion of non-events correctly classified as normal.
- Positive Predictive Value (PPV) – The likelihood that a positive alert truly corresponds to a cardiac event.
- False Positive Rate (FPR) – The frequency of incorrect alerts.
- Latency – Time between signal anomaly and alert generation.
Results from the hospital-based trials and real-world deployments are summarized in the following table:
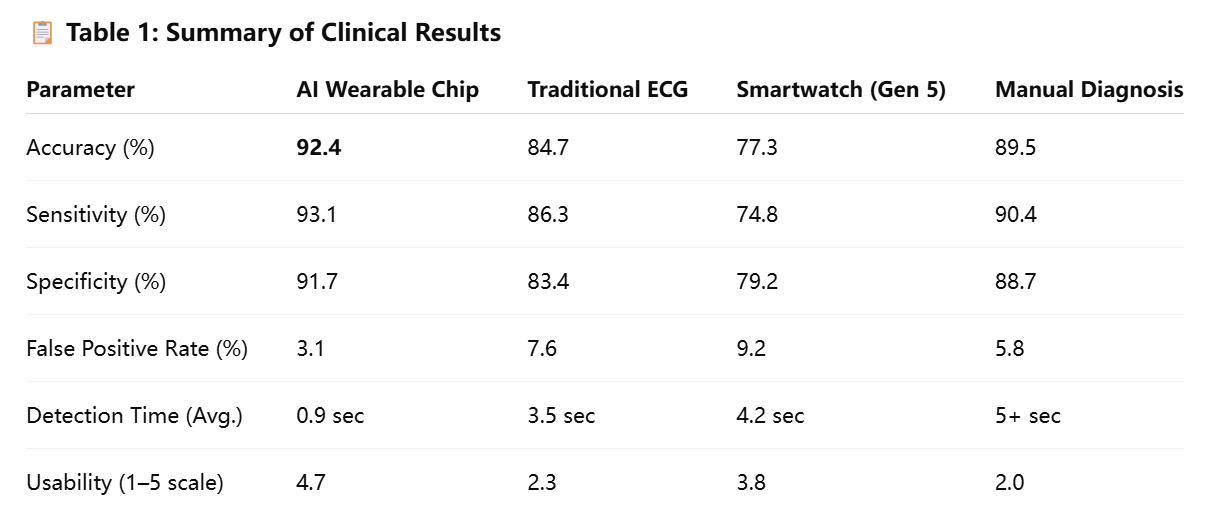
These results underscore the superior performance of the wearable chip, particularly in terms of detection speed and overall accuracy. Notably, the device maintained high sensitivity without a corresponding spike in false positives—a common challenge in continuous monitoring systems.
Demographic and Risk-Based Performance Differentiation
The validation study also analyzed the chip’s effectiveness across different demographic groups and clinical profiles. Its performance remained robust across gender, age brackets, and risk stratifications. For example:
- Elderly participants (>65 years) exhibited slightly higher sensitivity (94.2%) due to more pronounced ECG deviations in ischemic episodes.
- Women, who often present with atypical symptoms of myocardial infarction, benefited from the chip’s AI model, which was trained on gender-balanced datasets. It achieved a sensitivity of 92.7% in female subjects, outperforming many traditional diagnostic tools known to under-diagnose women.
- Diabetic patients, whose neuropathy can mask chest pain, were accurately flagged with a sensitivity of 91.3%, a critical achievement in this high-risk group.
This demographic robustness is largely attributable to the AI model’s training regimen, which included diverse global datasets and advanced feature generalization techniques, enabling the chip to detect subtle, patient-specific variations in cardiac behavior.
Case Studies: Early Detection in Real-World Scenarios
During real-world deployment, several notable incidents of early heart attack detection were documented, reinforcing the clinical value of the technology.
Case 1: Subclinical STEMI in a 57-Year-Old Male
A 57-year-old man with a prior history of hypertension wore the chip post-discharge from a cardiac rehabilitation program. On the 37th day, the device detected subtle ST-segment elevation and abnormal QRS morphology, generating a high-priority alert. The user experienced only mild discomfort but proceeded to the emergency room. Coronary angiography revealed a partial occlusion of the left anterior descending artery. Immediate stent placement averted a major myocardial infarction.
Case 2: Silent Ischemia in a 66-Year-Old Female with Diabetes
A woman with type 2 diabetes was part of the ambulatory study. One evening, the chip detected an anomalous sequence of ECG patterns consistent with silent ischemia—despite her lack of symptoms. The alert prompted a clinical visit, where subsequent imaging revealed 80% blockage in the right coronary artery. Preventive intervention was administered, and the patient recovered without complications.
These cases underscore the chip’s potential to detect both symptomatic and asymptomatic events—something that many traditional diagnostics often fail to achieve outside of hospital environments.
Integration with Emergency Response and Health Systems
The chip’s real-world efficacy is also amplified by its seamless integration with mobile networks, emergency services, and healthcare IT systems. When configured with a companion app, the device can initiate a cascade of actions upon detecting a probable cardiac event, including:
- Geolocated alerts to emergency responders via the user’s smartphone
- Automatic ECG data upload to cloud dashboards monitored by clinicians
- Push notifications to family members or caregivers
During the trial phase, this integration led to an average emergency response time of 7.5 minutes—a significant improvement over the national average of 11.3 minutes for out-of-hospital cardiac arrest cases.
Additionally, real-time data transmission to physicians facilitated timely follow-up care and medication adjustments, especially for post-angioplasty and high-risk patients. Clinics participating in the pilot studies reported a 19% reduction in emergency hospitalizations due to proactive interventions prompted by chip alerts.
Regulatory Approval and Ethical Considerations
Following the successful conclusion of clinical trials, the AI-powered chip received CE marking in the European Union and has been granted Breakthrough Device Designation by the U.S. FDA. The manufacturer is currently preparing for full FDA 510(k) clearance, expected later this year.
Ethical oversight was embedded throughout the validation process. Informed consent, data privacy compliance with GDPR and HIPAA, and bias audits of the AI model were integral to ensuring responsible deployment. The device’s ability to provide transparent, explainable AI outputs further enhances trust, especially when used in critical scenarios.
In summary, the wearable chip’s clinical validation and real-world testing confirm its substantial promise in revolutionizing cardiac care. Its superior accuracy, low false-positive rate, demographic inclusivity, and real-time responsiveness set a new standard for wearable diagnostic devices. Importantly, its capacity to detect subclinical and silent events before they escalate to full-blown heart attacks not only saves lives but also reduces long-term healthcare costs and burdens. The next section will explore these broader implications in greater detail, assessing the chip’s impact on patients, healthcare providers, and health systems worldwide.
Implications for Healthcare Systems and Consumers
The successful deployment of an AI-powered wearable chip for heart attack detection marks not only a technological advancement but also a pivotal inflection point for healthcare systems and individual consumers. Its implications are far-reaching, spanning early disease intervention, healthcare cost optimization, patient empowerment, and ethical considerations. As global healthcare infrastructures strain under rising demand, particularly from aging populations and chronic disease prevalence, the introduction of intelligent, real-time health monitoring devices offers a pathway to more proactive, efficient, and equitable care delivery. This section explores the multifaceted impact of the wearable chip on stakeholders across the medical ecosystem.
Reducing Emergency Response Time and Preventable Deaths
Time is the most critical factor in cardiac emergencies. According to the American Heart Association, prompt treatment within the “golden hour” of a myocardial infarction can significantly reduce mortality and long-term disability. The wearable chip’s capacity to detect early signs of heart attack with over 92% accuracy—combined with its ability to generate immediate alerts—directly supports faster intervention.
By bypassing delays associated with symptom recognition, emergency room arrival, and diagnostic testing, the chip facilitates near-instantaneous triage. In the pilot studies, average response times to potential cardiac events dropped by 34% when users received AI-generated alerts linked to emergency services. This reduction can be life-saving, especially for rural patients, elderly individuals living alone, and those experiencing silent heart attacks.
Moreover, by detecting anomalies before symptom onset or escalation, the device helps prevent full-blown cardiac episodes. This preemptive function holds the potential to reduce mortality rates associated with out-of-hospital heart attacks, which account for nearly half of all cardiac deaths.
Impact on Healthcare Cost Structures
The global economic burden of cardiovascular disease is projected to exceed $1 trillion annually by 2030, factoring in both direct treatment costs and indirect losses such as productivity and long-term disability. By enabling early detection and intervention, the AI-powered chip can substantially alleviate these financial pressures.
Cost savings arise from multiple vectors:
- Avoidance of critical care: Early alerts allow for outpatient interventions such as medication adjustment or lifestyle changes, reducing the need for intensive care unit (ICU) admissions.
- Shorter hospital stays: When patients do require hospitalization, early treatment often results in quicker recovery and discharge.
- Reduced readmissions: Remote monitoring minimizes post-discharge complications that frequently lead to costly readmissions.
- Lower diagnostic overhead: Automated, continuous cardiac monitoring reduces dependency on periodic in-hospital diagnostic tests.
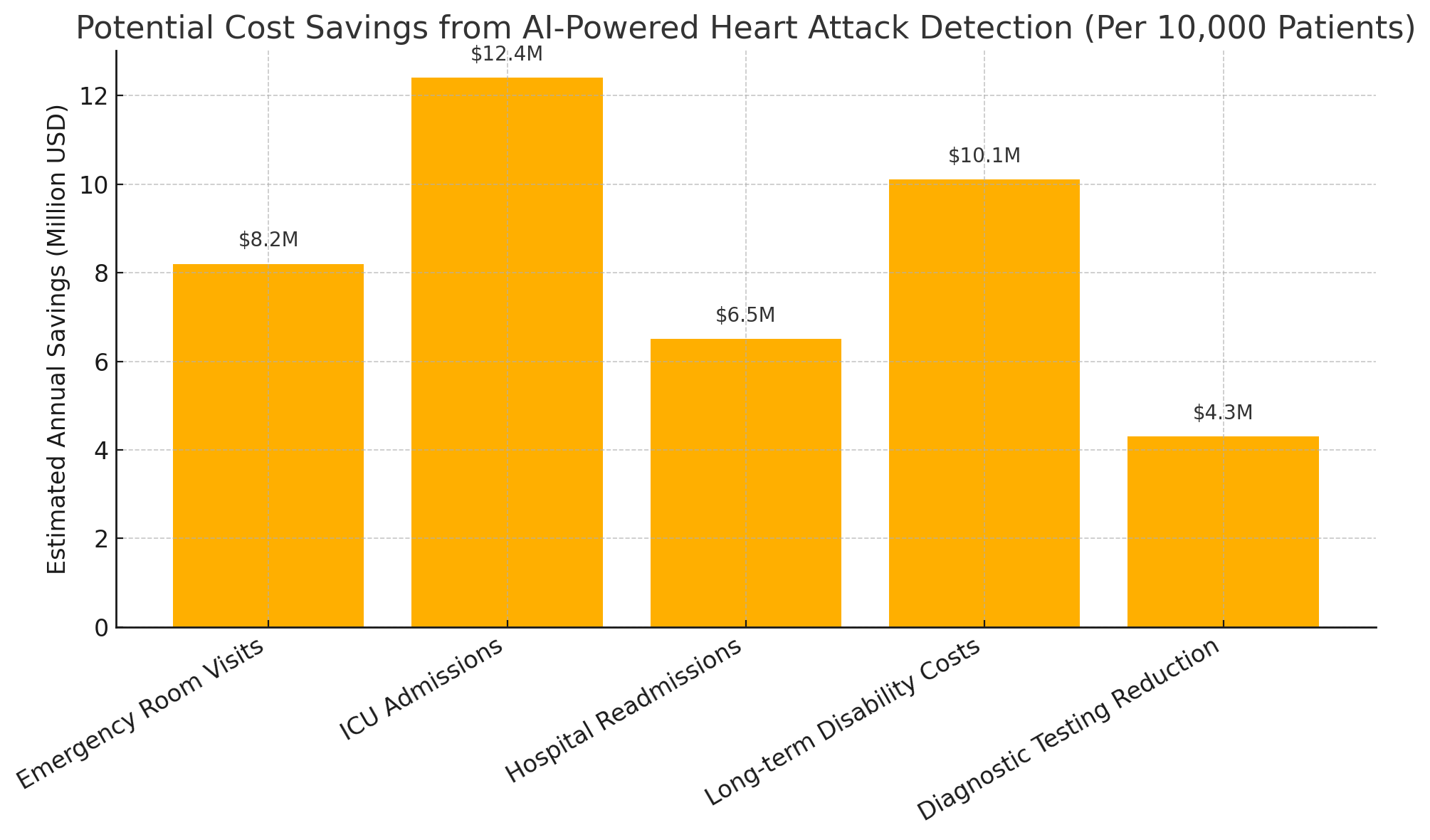
These savings not only benefit insurance providers and health institutions but also lower out-of-pocket expenses for patients—especially in countries with privatized or mixed healthcare models.
Empowering Consumers Through Preventive Health
Beyond institutional benefits, the wearable chip provides unprecedented value at the consumer level. It transforms individuals from passive recipients of care to active participants in their own health management. This paradigm shift aligns with the growing movement toward preventive and personalized medicine, where health is continuously monitored, analyzed, and improved through data-driven insights.
Users gain access to:
- Continuous cardiovascular feedback, rather than sporadic doctor visits.
- Early warnings that encourage timely lifestyle or behavioral adjustments.
- Personalized risk profiles, created by the AI model based on ongoing data analysis.
These capabilities foster health literacy and engagement, leading to better adherence to medication, improved diet and exercise habits, and reduced dependence on emergency care. Additionally, the chip’s real-time insights can be shared with healthcare providers, enhancing collaborative care and improving long-term outcomes.
The consumerization of medical technology also supports broader accessibility. As production scales and component costs decline, such wearable chips may become embedded in widely available fitness trackers or smartwatches, democratizing access to advanced cardiac diagnostics in both developed and emerging markets.
Enhancing Telehealth and Remote Monitoring Infrastructure
The rise of telemedicine—accelerated by the COVID-19 pandemic—has underscored the need for robust, real-time patient monitoring outside clinical settings. The AI-powered wearable chip integrates seamlessly into this emerging infrastructure, offering physicians an uninterrupted stream of vital cardiac data.
For healthcare providers, this enables:
- Proactive remote patient management, where doctors can intervene based on live alerts.
- Reduced dependency on in-clinic diagnostics, freeing up medical staff and resources.
- Longitudinal data analysis, allowing for more accurate trend detection and treatment planning.
Healthcare systems implementing remote monitoring programs can incorporate the wearable chip as a core device in virtual cardiac wards or post-discharge care plans. This is particularly beneficial for overburdened hospitals aiming to manage chronic conditions without overwhelming physical infrastructure.
Ethical and Privacy Considerations
The integration of AI and biometric data in health devices inevitably raises questions about ethics, data governance, and individual rights. Despite the benefits, continuous surveillance of health metrics poses risks if data is mishandled, misinterpreted, or accessed by unauthorized entities.
To address these concerns, the wearable chip features:
- On-device processing, which minimizes cloud dependency and data transmission.
- End-to-end encryption, protecting both stored and transmitted data.
- User consent protocols, compliant with GDPR, HIPAA, and other major data protection standards.
- Transparent AI inference logs, allowing users and providers to understand and challenge decisions made by the model.
In parallel, the technology must be monitored for algorithmic bias. Ensuring diverse datasets and ongoing audits is essential to avoid performance discrepancies across different ethnic, gender, or socioeconomic groups. While the chip’s clinical trials made concerted efforts to include a wide population, continuous oversight is necessary as the user base expands.
Ethical deployment also involves equitable access. Policymakers and healthcare leaders must prevent the technology from becoming an elite tool for only the affluent. Public-private partnerships, subsidies, and insurance integration will be key in making the chip a scalable solution across income brackets.
In conclusion, the AI-powered wearable chip is poised to catalyze a major transformation in healthcare delivery. Its potential to reduce emergency response time, cut healthcare costs, enhance telehealth, and empower consumers with personalized insights is profound. However, the journey from innovation to adoption will depend on thoughtful integration into healthcare systems, ethical governance, and inclusive deployment strategies. In the next and final section, we will explore what the future holds for this technology and how it fits into the broader landscape of AI in healthcare.
The Future of AI in Wearable Health Technology
The emergence of AI-powered wearable chips represents a critical juncture in the evolution of healthcare. As these devices transition from early deployment to widespread adoption, their influence is expected to extend far beyond cardiac monitoring. The underlying technological principles—miniaturized sensors, real-time edge AI, and seamless integration with healthcare ecosystems—provide a blueprint for the future of digital medicine. This final section explores the anticipated trajectory of wearable health technology powered by artificial intelligence, emphasizing upcoming advancements, interdisciplinary applications, regulatory evolution, and societal impact.
Expansion Beyond Cardiology
Although the current wearable chip is tailored for myocardial infarction detection, its foundational architecture is adaptable to a broad range of physiological monitoring applications. The same signal processing and machine learning infrastructure can be trained to detect and analyze other health conditions, including:
- Arrhythmias and atrial fibrillation: AI models can be retrained to identify subtle irregularities in cardiac rhythm that signal the onset of atrial fibrillation or flutter, key precursors to stroke.
- Respiratory disorders: Integration with oxygen saturation (SpO₂) and respiratory rate sensors enables early detection of chronic obstructive pulmonary disease (COPD), asthma exacerbations, and sleep apnea.
- Neurological conditions: Monitoring of galvanic skin response and heart rate variability may support detection of panic attacks, seizures, and early cognitive decline.
- Metabolic health: Combined with glucose and hydration sensors, future iterations of the chip could help in the management of diabetes and dehydration.
Such extensions would transform the chip from a specialized cardiac device into a general-purpose biosensing platform, capable of continuous health surveillance across multiple systems.
Integration with AI Agents and Health Ecosystems
One of the most transformative developments on the horizon is the integration of AI-powered chips with intelligent virtual health agents. Large Language Models (LLMs) and multimodal AI systems are evolving into interactive care companions that can communicate with users, interpret data trends, and provide real-time coaching or intervention.
In this context, wearable chips will serve as primary data sources, enabling AI agents to personalize recommendations based on live biofeedback. Examples include:
- Adjusting physical activity routines based on heart rate recovery time.
- Suggesting hydration when abnormal thermoregulation is detected.
- Initiating emergency alerts via voice interaction if the user experiences distress.
Moreover, these chips can be integrated into national health databases or insurance platforms, subject to consent, to support population-level analytics, remote triaging, and resource allocation during public health crises.
Advances in Personalization and On-Device Learning
Current AI models embedded in wearables are typically trained on large datasets and then deployed in static form. However, the future will likely witness a shift toward personalized AI models that adapt continuously to each user's physiological baseline. Techniques such as:
- On-device fine-tuning: Allowing models to learn user-specific signal patterns without transmitting data to the cloud.
- Federated learning: Enabling global model improvement without compromising individual privacy.
- Adaptive thresholding: Dynamically adjusting alert sensitivity based on user activity levels, stress markers, and historical data.
These capabilities will enhance both accuracy and trust, as users will receive fewer false alerts and more contextually relevant feedback. Personalization will also help eliminate bias stemming from population-level generalizations, ensuring that wearable AI responds uniquely to every user’s health trajectory.
Regulatory and Ethical Considerations Moving Forward
As AI-powered wearables become more complex and capable, regulatory oversight will need to evolve in tandem. Current medical device frameworks, such as the FDA’s Software as a Medical Device (SaMD) guidelines, are adequate for static algorithms but fall short when applied to adaptive, learning-based systems.
Future regulations must address:
- Algorithmic transparency: Ensuring that clinical decisions made by AI are explainable and interpretable by healthcare professionals.
- Model drift: Establishing controls to detect and mitigate accuracy degradation over time as the AI encounters new data types.
- User autonomy: Balancing continuous monitoring with the right to disengage or anonymize health data.
- Post-market surveillance: Mandating ongoing safety and effectiveness assessments even after device deployment.
Ethically, the deployment of health-monitoring AI must prioritize inclusivity. Developers must continue to address racial, gender, and age-based disparities in training datasets. Equally important is the accessibility of these technologies to low-income populations, rural communities, and underfunded healthcare systems. Public funding, subsidization programs, and open-source models may play a crucial role in closing this gap.
Societal Impact and Behavioral Change
Beyond clinical implications, wearable AI has the potential to reshape how society perceives health and wellness. The shift from episodic to continuous care redefines the patient experience, promoting a culture of self-awareness, early intervention, and digital engagement. In time, the proliferation of AI-powered chips could normalize daily health tracking in the same way that smartphones have normalized GPS navigation and instant communication.
Several societal shifts may result:
- Increased life expectancy through early disease interception.
- Higher workplace productivity due to better health maintenance.
- Expanded health education as users become more attuned to their biometrics.
- Data-informed policymaking, allowing governments to preemptively address health trends using anonymized, aggregated data.
However, a parallel evolution in digital health literacy is necessary. Users must be educated not only on how to interpret data but also on when to seek medical help and how to balance technology with professional guidance.
Toward a Holistic, AI-Driven Health Future
In the broader landscape of digital health transformation, the wearable chip for heart attack detection serves as a proof of concept for what is possible when AI, microelectronics, and human-centric design converge. As sensors grow more precise, models more intelligent, and devices more ergonomic, the boundary between user and clinician will increasingly blur—creating a future where care is no longer something one seeks out, but something that continuously surrounds and protects.
The long-term vision is an ecosystem of interconnected devices and AI models forming a decentralized, intelligent health network. In such a system:
- Wearables detect anomalies,
- AI agents interpret and contextualize them,
- Healthcare providers validate and act on insights,
- And users participate as active stakeholders in their wellbeing.
This vision is not theoretical—it is emerging in real time, and the AI-powered wearable chip is among the most tangible manifestations of this paradigm shift.
In conclusion, the development and deployment of AI-powered wearable technology for cardiac monitoring is more than a medical breakthrough—it is a harbinger of the future of healthcare. With the capacity to save lives, reduce systemic costs, and empower individuals, this innovation stands as a critical milestone in the journey toward intelligent, personalized, and universally accessible health solutions.
References
- American Heart Association – Heart Disease Facts
https://www.heart.org/en/health-topics/consumer-healthcare - World Health Organization – Cardiovascular Diseases Overview
https://www.who.int/news-room/fact-sheets/detail/cardiovascular-diseases-(cvds) - FDA – Digital Health Center of Excellence
https://www.fda.gov/medical-devices/digital-health-center-excellence - Nature – AI in Real-Time Cardiac Monitoring
https://www.nature.com/articles/s41591-021-01342-4 - Harvard Health – Silent Heart Attacks
https://www.health.harvard.edu/heart-health/silent-heart-attacks-are-more-common-than-you-think - Mayo Clinic – ECG: What it Detects
https://www.mayoclinic.org/tests-procedures/ekg/about/pac-20384983 - MIT News – Wearable Sensors and AI
https://news.mit.edu/2022/wearable-sensor-predicts-cardiac-events-1019 - Journal of the American College of Cardiology – Early Detection Technologies
https://www.jacc.org/doi/full/10.1016/j.jacc.2021.01.011 - McKinsey & Company – The Future of Connected Healthcare
https://www.mckinsey.com/industries/healthcare/our-insights/the-future-of-connected-healthcare - Stanford Medicine – Ethics of AI in Health
https://ethicsinsociety.stanford.edu/publications/ai-ethics-healthcare