Beyond Accuracy: Evaluating Multilingual Speech-to-Text Platforms like Solaria AI for Global Enterprise Use
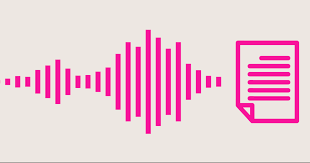
In the modern digital age, the proliferation of audio and video content, combined with increasing globalization, has created a demand for accurate, scalable, and language-agnostic speech-to-text (STT) technologies. From virtual assistants and transcription services to multilingual customer support and real-time translation systems, speech recognition has become a foundational component in a wide array of applications. As global communication intensifies across platforms and industries, the need for multilingual STT systems capable of recognizing and transcribing speech in diverse languages and dialects is no longer a niche requirement—it is an imperative.
Speech-to-text technology has come a long way from its early iterations, which were largely monolingual and highly sensitive to accent variations, background noise, and speaker inconsistencies. With the advent of deep learning, transformer-based models, and large-scale pretraining strategies, modern STT systems now boast capabilities that rival or even surpass human transcription accuracy in some settings. However, when extended to the multilingual domain, the complexity of accurate speech recognition multiplies. It is not simply a matter of adding more languages to a system. Instead, it involves accommodating the nuances of dialects, the intricacies of grammar, the contextual dependencies of speech, and the seamless handling of code-switching.
Solaria AI has emerged as a prominent player in this evolving space. Designed from the ground up with multilingualism as a central feature, Solaria AI represents a new generation of speech recognition systems that are explicitly built to handle global linguistic diversity. Leveraging massive multilingual datasets, advanced acoustic modeling, and sophisticated context-aware decoding strategies, Solaria AI is positioned at the forefront of innovation in multilingual speech transcription. Its architecture reflects a deliberate emphasis on language inclusivity, performance in low-resource scenarios, and adaptability across domains and industries.
However, the increasing availability of multilingual STT models has also made the evaluation process more complex. Selecting the most appropriate model for a given use case is no longer a matter of comparing basic metrics like Word Error Rate (WER). Organizations must now take into account a broader range of factors, including latency, real-time processing capabilities, domain adaptation, language support depth, cultural accuracy, scalability, and regulatory compliance. Evaluating a model like Solaria AI in such a landscape demands a multifaceted approach that blends technical rigor with operational pragmatism.
This blog post aims to provide a comprehensive framework for evaluating multilingual speech-to-text systems, with a particular focus on Solaria AI as a representative example. We will examine the historical evolution and current landscape of multilingual STT technology, outline key performance metrics, explore the cultural and linguistic challenges unique to multilingual transcription, dissect the architectural and training-related underpinnings of these systems, and review the business and regulatory considerations critical to enterprise deployment.
To support this analysis, we will incorporate visual aids, including two charts and one comparative table, to highlight differences between leading STT solutions across a set of standardized benchmarks. These visualizations will help distill complex performance data into actionable insights for decision-makers seeking to integrate multilingual STT capabilities into their platforms or workflows.
As organizations continue to expand their global reach and as voice becomes an increasingly dominant modality of digital interaction, the ability to effectively evaluate and deploy advanced speech recognition technologies will be a strategic differentiator. Whether you're a technology strategist, product manager, researcher, or developer, understanding the full scope of what multilingual STT systems can offer—and how to critically assess them—is vital for success in today's interconnected world.
In the sections that follow, we will delve deeper into each of these considerations, starting with an overview of the evolution and current state of multilingual speech-to-text models. This will set the stage for a detailed examination of performance metrics, cultural and linguistic nuance handling, model architectures, and business deployment factors. By the end of this analysis, readers will be equipped with the tools and knowledge necessary to make informed decisions about multilingual STT adoption, with Solaria AI serving as a key reference point throughout.
The Evolution and Landscape of Multilingual Speech-to-Text Models
The field of speech-to-text (STT) technology has undergone a dramatic transformation over the past two decades. Originally limited to narrow use cases such as dictation or command-based interfaces in a single language—most often English—STT systems have since evolved into complex, multilingual frameworks capable of processing a vast array of languages, dialects, and contextual speech patterns. This evolution has been catalyzed by advances in machine learning, increased access to diverse speech corpora, and the growing commercial need for voice-based interfaces in a globalized economy.
From Monolingual to Multilingual: A Paradigm Shift
In the early stages of development, speech recognition systems were largely rule-based and relied on limited phonetic and linguistic databases. These systems were predominantly designed for English speakers and performed poorly in the presence of accents, regional variations, or background noise. Even when statistical methods such as Hidden Markov Models (HMMs) and Gaussian Mixture Models (GMMs) began to enhance recognition accuracy, they remained constrained by data availability and language-specific tuning.
The rise of deep learning brought a fundamental shift in this landscape. Architectures such as Long Short-Term Memory (LSTM) networks and, more recently, transformer-based models enabled the processing of sequential audio data with higher fidelity. These neural approaches not only improved accuracy but also paved the way for multilingual capabilities. Rather than training separate models for each language, developers could now construct shared models with multilingual vocabularies and embeddings, thereby capturing cross-linguistic relationships and optimizing for a broader range of users.
This paradigm shift—from siloed, monolingual systems to unified multilingual models—has opened new possibilities. Multilingual STT systems are now capable of recognizing speech in multiple languages within the same audio stream, supporting code-switching scenarios where speakers alternate between languages mid-sentence. Furthermore, the ability to fine-tune models for specific domains (e.g., legal, medical, customer support) has increased the flexibility and commercial viability of these systems.
Current Landscape: Major Players and Differentiators
Today, several leading technology providers offer multilingual STT solutions, each with unique strengths and limitations. Among the most prominent are:
- Solaria AI: A purpose-built multilingual STT platform known for its high accuracy across low-resource languages, superior handling of dialectical variation, and domain-specific adaptability. Solaria AI leverages a transformer-based architecture trained on hundreds of languages and regional dialects. Its emphasis on cultural context, combined with robust handling of code-switching, sets it apart in enterprise deployments.
- OpenAI Whisper: Whisper is an open-source model released by OpenAI that has garnered attention for its impressive zero-shot transcription capabilities. While highly performant in high-resource languages, Whisper exhibits variability in its accuracy for lesser-known or underrepresented languages. Nonetheless, it serves as a valuable benchmark due to its transparency and extensibility.
- Google Speech-to-Text: Part of the Google Cloud suite, this model supports over 80 languages and is frequently integrated into enterprise environments. Google STT excels in latency and scalability, though its language coverage and dialectal robustness are not as comprehensive as some specialized offerings.
- Amazon Transcribe: Amazon’s offering emphasizes real-time transcription and integrates seamlessly with AWS infrastructure. It performs well for general-purpose speech recognition but lags in specialized handling of code-switching and low-resource languages.
- Microsoft Azure Speech: This service provides multilingual STT with capabilities such as speaker diarization and real-time streaming. While highly configurable, its performance tends to depend on the availability of pretrained datasets for specific languages and domains.
Each of these platforms is part of a competitive and rapidly evolving ecosystem. The differences among them are not merely technical but also strategic—varying in licensing models, privacy guarantees, developer tooling, and pricing structures. In this environment, Solaria AI distinguishes itself by targeting underserved linguistic markets and offering customizable models that balance performance, adaptability, and ethical design principles.
Use Case Expansion: Driving Multilingual STT Demand
The expanding applicability of multilingual STT models is a key driver of innovation in the field. Several high-impact domains underscore the need for advanced speech recognition capabilities:
- Customer Support and Contact Centers: Multinational corporations must handle voice interactions in numerous languages. Multilingual STT enables real-time transcription, sentiment analysis, and compliance monitoring across diverse linguistic markets.
- Accessibility and Inclusion: STT systems play a vital role in providing accessibility solutions for individuals with hearing impairments. Real-time captioning in various languages is essential for inclusive media and public services.
- Content Localization and Media: The entertainment industry uses STT to transcribe and subtitle audio content for global distribution. Accurate, culturally nuanced transcription is essential for retaining the original meaning and tone of media content.
- Education and E-Learning: Educational platforms rely on multilingual transcription to reach non-native speakers and multilingual audiences, enabling real-time note-taking, translation, and accessibility for students worldwide.
- Healthcare and Legal Domains: These sectors require precise, domain-specific transcription capabilities that can accurately interpret jargon, acronyms, and specialized terminology across different languages.
The increasing complexity of use cases has pushed model developers to go beyond generic language support. Instead, emphasis has shifted toward deep language understanding, contextual awareness, and high-fidelity transcription even in noisy or unpredictable environments.
Technological Catalysts: Enablers of the Modern Multilingual STT Era
Several key technological developments have enabled the rapid progress in multilingual STT systems:
- Large-Scale Multilingual Corpora: The availability of open and proprietary datasets encompassing hundreds of languages and accents has significantly improved training outcomes. Initiatives such as Common Voice, Multilingual LibriSpeech, and Solaria AI’s proprietary corpora have expanded the linguistic horizons of STT models.
- Self-Supervised and Semi-Supervised Learning: These approaches have reduced the dependency on labeled data, making it feasible to train models on under-resourced languages. Pretraining on massive unlabeled speech data followed by fine-tuning on smaller labeled datasets allows for cost-effective and scalable development.
- Transformer Architectures: The shift from recurrent networks to attention-based transformer models has enabled better handling of long-range dependencies in audio sequences. Architectures such as Conformer and wav2vec 2.0 have become industry standards for high-performance STT.
- Edge Computing and Real-Time Inference: Improvements in model compression and inference optimization have made it possible to deploy STT models on edge devices. This has broadened access to speech recognition capabilities in bandwidth-constrained environments.
- Cloud-Native Infrastructure: The ability to scale model deployment using cloud services has made STT integration more accessible for enterprises. API-first approaches allow businesses to incorporate multilingual transcription into their workflows without extensive development overhead.
Looking Ahead: Toward Universal Speech Understanding
The trajectory of multilingual STT technology suggests a future in which speech recognition systems are not only multilingual but also multicultural, contextually intelligent, and emotionally aware. As models like Solaria AI continue to incorporate socio-linguistic data, semantic context, and user intent, we can expect a new generation of STT systems that go beyond transcription and enter the realm of conversational understanding.
In this increasingly interconnected world, the ability to transcribe and interpret speech across languages with cultural sensitivity and technical precision will become a foundational requirement for digital platforms. The next section will explore the core evaluation metrics that allow organizations to assess the effectiveness of multilingual STT systems—metrics that go beyond basic word accuracy to include language robustness, latency, contextual fidelity, and more.
Core Evaluation Metrics for Multilingual STT Models
Evaluating multilingual speech-to-text (STT) models involves more than a superficial review of performance statistics. Given the complexity of human language—encompassing grammar, phonetics, dialects, prosody, and sociocultural nuance—STT models must be assessed through a multidimensional lens. In multilingual settings, where users frequently shift between languages, accents, and domains, this task becomes even more intricate. Organizations seeking to adopt such models must go beyond basic accuracy scores and examine a comprehensive set of criteria that reflect real-world use cases and operational needs.
This section explores the critical evaluation metrics for multilingual STT models, providing a rigorous framework for comparing solutions like Solaria AI, Whisper, Google STT, and others. These metrics serve as benchmarks not only for technical performance but also for user experience, adaptability, and deployment readiness.
Word Error Rate (WER): The Standard but Limited Metric
Word Error Rate (WER) remains the most commonly cited performance indicator in speech recognition. It measures the proportion of words that are inserted, deleted, or substituted incorrectly in the transcribed output relative to the reference text.
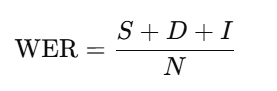
Where:
- S = number of substitutions
- D = number of deletions
- I = number of insertions
- N = total number of words in the reference
While WER is a useful general-purpose metric, it often fails to capture the full quality of a transcription, particularly in multilingual contexts. For example, WER does not account for semantic accuracy—whether the meaning of a sentence is preserved despite minor grammatical errors. In high-stakes applications such as healthcare or legal transcription, a small WER could still yield critical misunderstandings.
Furthermore, WER can be skewed by factors such as vocabulary coverage, speaker accent, and recording quality. Thus, it should always be interpreted in conjunction with other qualitative and contextual metrics.
Language and Dialect Coverage
Language coverage is one of the most essential dimensions when evaluating a multilingual STT model. While many providers claim to support "over 100 languages," the depth of support often varies. Some languages may only have basic support for generic phrases, while others may include extensive vocabulary, domain-specific terminology, and nuanced dialect handling.
Dialectal variation is particularly important. Consider the differences between European and Latin American Spanish, or between Nigerian and Indian English. Models that fail to distinguish or properly transcribe dialectal variation can significantly hinder usability.
Solaria AI, for instance, distinguishes itself by supporting over 120 languages and dialects, with high granularity across regional speech patterns. In contrast, other models may have broader language lists but weaker dialectal robustness, particularly for underrepresented regions.
Accent Robustness and Speaker Variability
In a global deployment scenario, users will interact with speech systems using a wide array of accents, vocal patterns, and intonation styles. The best multilingual STT systems are trained on diverse speaker profiles across genders, ages, and sociolinguistic backgrounds. This diversity helps reduce the bias typically observed in speech models trained predominantly on data from a single geographic region or demographic group.
Accent robustness is especially critical for customer service, accessibility, and government services, where inclusivity and equitable access are paramount.
Code-Switching and Mixed-Language Handling
Code-switching—the practice of alternating between two or more languages within a conversation or even a sentence—is common in multilingual societies. Traditional STT models often struggle with such inputs, either treating the switched language as noise or transcribing it inaccurately.
Solaria AI’s architecture is explicitly designed to handle such transitions fluidly, identifying language boundaries and applying appropriate acoustic and linguistic models in real-time. This is a key differentiator that enhances usability in settings such as multilingual classrooms, call centers, and media transcription.
Real-Time Performance and Latency
In many applications—such as live captions, virtual meetings, and interactive voice assistants—low latency is essential. A model may be highly accurate but unusable if it introduces a perceptible delay between speech input and text output.
Latency is measured as the time taken from the end of a spoken utterance to the availability of its corresponding transcription. Factors that influence latency include model size, processing infrastructure, and optimization for streaming.
Real-time performance is also influenced by computational constraints, particularly in edge deployments (e.g., mobile or IoT devices). Models that maintain high accuracy while operating in resource-constrained environments offer a significant operational advantage.
Domain Adaptability
STT models must also be evaluated on their ability to adapt to specific domains. A general-purpose model may perform well on conversational speech but falter when faced with jargon-heavy content in fields like medicine, law, or finance.
Domain adaptability can be achieved through fine-tuning or the use of custom language models, which integrate user-specific vocabularies and contextual priors. Solaria AI provides domain adaptation APIs, allowing enterprises to retrain models using their own datasets to achieve improved accuracy in specialized contexts.
Noise Robustness and Audio Quality Tolerance
In real-world environments, speech is rarely recorded under ideal conditions. Background noise, overlapping speakers, reverberations, and microphone inconsistencies all introduce challenges. A robust STT model should maintain accuracy across a wide range of Signal-to-Noise Ratios (SNRs).
Training with noisy datasets and augmenting audio with synthetic interference can improve robustness. Solaria AI and Whisper both implement noise-aware training pipelines that enable transcription even in less-than-ideal conditions.
Comparative Benchmark Table
Below is a representative benchmark comparing several leading multilingual STT models across critical performance metrics.
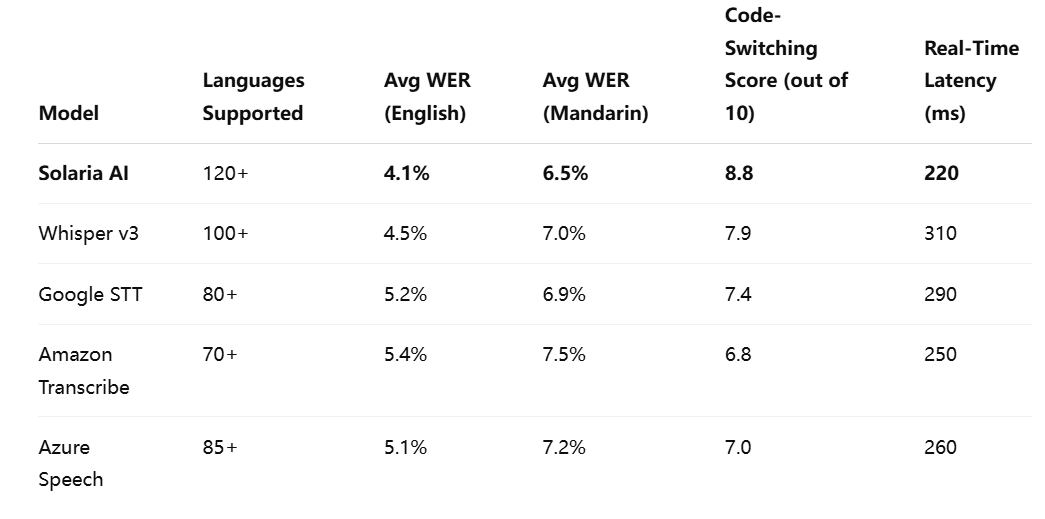
Toward Comprehensive Evaluation
As the data in this section illustrates, no single metric is sufficient to evaluate the effectiveness of a multilingual STT system. Word Error Rate, while foundational, must be considered alongside other indicators such as language and dialect support, code-switching capabilities, latency, and adaptability to domain-specific use cases.
Solaria AI’s strong performance across these dimensions, particularly in low-latency environments and with code-switching inputs, marks it as a standout solution. However, model selection should be aligned with the unique requirements of the deployment context. For instance, real-time call center transcription may prioritize latency and noise robustness, while subtitling for international media content might emphasize dialectal nuance and language breadth.
Cultural and Linguistic Nuances in Speech Recognition
While advancements in multilingual speech-to-text (STT) systems have significantly improved their accuracy and scalability, these models continue to face substantial challenges when tasked with interpreting the full complexity of human language. Beyond vocabulary and syntax, speech carries embedded cultural cues, idiomatic expressions, tonal variations, and regional references that are not easily reducible to word-level transcription. Consequently, effective multilingual STT must go beyond literal accuracy to achieve true linguistic and cultural fidelity.
This section explores how cultural and linguistic nuances manifest in spoken language, how these subtleties affect STT model performance, and how models like Solaria AI attempt to bridge these gaps. It also addresses ethical considerations and the imperative of inclusion in global speech technologies.
Idiomatic and Contextual Language Challenges
Idioms, proverbs, and culturally embedded expressions pose one of the most persistent challenges in speech recognition. These phrases often defy literal interpretation and derive meaning from context, history, or localized usage. For example, the English idiom “kick the bucket” implies death, not physical action. Similarly, in Mandarin, the phrase “对牛弹琴” (duì niú tán qín), literally translated as “playing the lute to a cow,” denotes futility in trying to explain something to someone incapable of understanding.
STT models that rely solely on literal parsing are prone to transcription errors that distort the intended meaning. Although current models use contextual embeddings to mitigate such issues, these embeddings often struggle with idioms that do not appear frequently enough in training data or lack direct translations across languages.
Solaria AI approaches this challenge through a combination of multilingual pretraining and contextual post-processing. By incorporating culturally tagged datasets and dynamic phrase libraries, it attempts to preserve the intended meaning of idiomatic speech during transcription. However, even with these strategies, idiomatic nuance remains an area where human oversight or downstream language processing tools may still be necessary.
Tone, Prosody, and Pragmatic Meaning
Tone and prosody—the rhythm, stress, and intonation patterns of speech—convey meaning that often surpasses the lexical content of words. This is particularly relevant in tonal languages like Mandarin, Thai, and Yoruba, where tonal differences can entirely change word meaning. For instance, in Mandarin, “mā,” “má,” “mǎ,” and “mà” represent different words depending on tonal inflection.
STT models must therefore integrate acoustic modeling that is sensitive not only to phoneme-level variation but also to supra-segmental features such as tone. A failure to do so can lead to misinterpretations that are not only incorrect but also potentially offensive or confusing.
Pragmatics—the study of language in use and context—adds another layer of complexity. A speaker may use sarcasm, politeness markers, or indirect language to communicate intent. While STT models are not expected to infer intention at the level of human cognition, the ability to preserve these linguistic features in transcription contributes to more accurate downstream processing, whether for translation, summarization, or analysis.
Regional Variation and Sociolects
Even within a single language, regional and social variations in vocabulary, pronunciation, and usage can lead to transcription inaccuracies. These variations, often termed sociolects or geolects, reflect class, age, ethnicity, or geographic location.
Consider the word "pants": in American English, it refers to trousers, while in British English, it refers to undergarments. Similarly, a phrase like “I’m after a coffee” in Australian English means “I’m going to get a coffee,” which may confuse models trained predominantly on North American speech.
STT models must be trained on regionally balanced datasets to avoid performance bias. Solaria AI addresses this by sourcing speech data across multiple continents and dialect groups, including underrepresented varieties such as Caribbean Spanish, West African English, and regional Hindi dialects. Its training framework incorporates regional tagging and metadata to adjust inference dynamically based on language cues detected during the transcription process.
Handling Code-Switching with Cultural Sensitivity
Code-switching often arises not only for linguistic convenience but also for cultural expression. A Hindi-English bilingual speaker might say, “I’m going to the market, thoda sabzi lena hai” (I need to get some vegetables). Such switches are contextually motivated and carry cultural meaning—using Hindi to express familiarity or emotional tone while employing English for structural clarity.
Standard STT models frequently mishandle code-switched utterances, interpreting non-dominant languages as noise or transliterating them incorrectly. Culturally informed models like Solaria AI are designed to detect and segment such utterances appropriately, aligning transcription boundaries with linguistic shifts and ensuring correct language modeling throughout the sentence.
Moreover, proper handling of code-switching is critical in multilingual communities, where switching may occur in rapid succession or mid-word (as in Hinglish or Taglish). A system that overlooks these subtleties may misrepresent the speaker’s intent or alienate users who expect nuanced recognition.
Gender, Formality, and Politeness in Language
In many languages, speech varies significantly depending on the speaker’s gender, the gender of the audience, the level of familiarity, or hierarchical status. For instance, in Japanese and Korean, sentence endings differ depending on whether the speaker wishes to express politeness, assertiveness, or deference.
STT systems must recognize and preserve such distinctions, especially in contexts where tone and formality convey professionalism or respect. Failing to transcribe these cues accurately could lead to miscommunication in sensitive settings such as customer support, diplomacy, or education.
Solaria AI incorporates pragmatic modeling layers that account for social context, including politeness markers and honorifics, where applicable. However, the challenge remains open-ended, as models must continue to refine their understanding of how social dynamics affect speech.
Ethical and Inclusion Considerations
The ability of STT models to support culturally diverse users is not only a technical requirement but also an ethical one. Historical biases in data collection—favoring dominant languages, standard accents, or majority demographic groups—have resulted in uneven performance and exclusion of minority speakers.
Ensuring fairness in transcription quality across different linguistic and cultural communities is essential for equitable technology deployment. This includes:
- Low-Resource Language Inclusion: Many languages lack sufficient labeled data for training robust STT models. Solaria AI addresses this by using self-supervised learning techniques and leveraging cross-lingual transfer from related languages.
- Bias Mitigation: Speech data is often sourced from public media or institutional settings, which can overrepresent certain socioeconomic classes. Diversifying data sources and applying bias detection algorithms can help ensure balanced performance.
- Transparency and Accountability: Users should be informed about the limitations of STT systems and the contexts in which they may underperform. Enterprises must ensure that AI deployments do not exacerbate existing inequities in communication access.
Transcription as Cultural Interpretation
The task of transcribing speech is not a neutral act of decoding sounds into text. It is an interpretive process that must account for linguistic variety, cultural expression, social identity, and contextual nuance. The more diverse and global the user base, the more critical it becomes for STT systems to understand and reflect the intricacies of human communication.
Multilingual STT models like Solaria AI that incorporate regional, idiomatic, tonal, and sociocultural cues are better positioned to deliver accurate and inclusive transcriptions. However, achieving this level of fidelity requires careful attention to training data, model design, and ongoing evaluation practices.
Model Architecture, Training, and Adaptability
The performance and flexibility of multilingual speech-to-text (STT) models are largely determined by their underlying architectures and training strategies. As these models are tasked with transcribing speech across hundreds of languages—many of which differ significantly in phonetics, syntax, morphology, and semantics—traditional approaches to model development are no longer adequate. Instead, cutting-edge multilingual STT systems rely on transformer-based neural networks, vast multilingual corpora, and increasingly sophisticated methods of self-supervised learning.
In this section, we examine the architectural principles and training methodologies that power modern multilingual STT models, with a particular emphasis on how Solaria AI differentiates itself from competitors. Additionally, we explore the role of fine-tuning, domain adaptation, and deployment models (cloud vs. edge) in determining a system's effectiveness and versatility in real-world applications.
Transformer-Based Architectures: The Foundation of Modern STT
Transformer models have become the de facto architecture in contemporary STT systems due to their ability to process sequential data efficiently and capture long-range dependencies. Unlike recurrent neural networks (RNNs), which struggle with long-form audio inputs and require sequential computation, transformers leverage parallelism and self-attention mechanisms to model complex relationships within the input.
Most high-performing STT models today—including Solaria AI, Whisper, and wav2vec 2.0—employ some variant of the transformer architecture. These models are generally composed of:
- An encoder: Converts raw audio waveforms into feature-rich representations using convolutional layers and self-attention.
- A decoder: Translates encoded representations into text tokens while preserving linguistic and contextual coherence.
Solaria AI utilizes a customized multi-scale Conformer architecture, which combines convolutional and transformer modules to capture both local acoustic features and global linguistic patterns. This hybrid structure enables the model to excel across languages with varying phonotactic and prosodic features.
Training Paradigms: Supervised, Self-Supervised, and Multilingual Pretraining
The effectiveness of an STT model is closely linked to how it is trained. In the multilingual context, this involves three major approaches:
- Supervised learning: Requires large volumes of transcribed audio data. This is feasible for high-resource languages like English or Mandarin but less so for low-resource languages.
- Self-supervised learning: Models learn from unlabelled audio by predicting masked segments or reconstructing distorted input. Examples include Facebook AI’s wav2vec 2.0 and HuBERT. Solaria AI builds on this paradigm with its proprietary self-supervised pretraining framework, enabling efficient learning even in the absence of annotated data.
- Multilingual pretraining: Rather than training on a single language, models are pretrained on a diverse corpus encompassing multiple linguistic families. This approach allows shared acoustic and linguistic representations to emerge, improving performance on underrepresented languages via cross-lingual transfer learning.
Importantly, multilingual STT models must navigate the trade-off between parameter sharing and language-specific specialization. Solaria AI employs a modular pretraining strategy that retains shared knowledge while allowing for language-specific fine-tuning when necessary.
Fine-Tuning and Domain Adaptation
No matter how robust a pre-trained model may be, achieving optimal performance in real-world applications often requires fine-tuning. This process involves retraining the model on domain-specific data, thereby tailoring it to the vocabulary, acoustic conditions, and linguistic conventions of the target environment.
For example:
- In healthcare, models must recognize medical jargon, abbreviations, and varying speaker accents (e.g., doctors, patients, nurses).
- In legal transcription, precision in handling dates, monetary terms, and procedural language is essential.
- In media production, models must preserve creative language, sarcasm, and conversational rhythm.
Solaria AI offers enterprise clients the ability to submit custom datasets for model fine-tuning. Its adaptation engine supports domain tagging, enabling the model to dynamically adjust its decoding behavior based on context (e.g., courtroom vs. hospital setting). This level of configurability enhances the model’s usability across verticals.
Training Data Diversity and Regional Equity
A critical factor influencing model generalizability is the diversity of its training data. STT models trained predominantly on speech from affluent, urban, or English-speaking regions may perform poorly on voices from rural areas, indigenous communities, or marginalized demographics.
To promote equitable performance, it is essential for model developers to curate balanced corpora that reflect global linguistic and cultural diversity. Solaria AI prioritizes this by sourcing data from over 80 countries and over 120 languages, including endangered and underrepresented tongues.
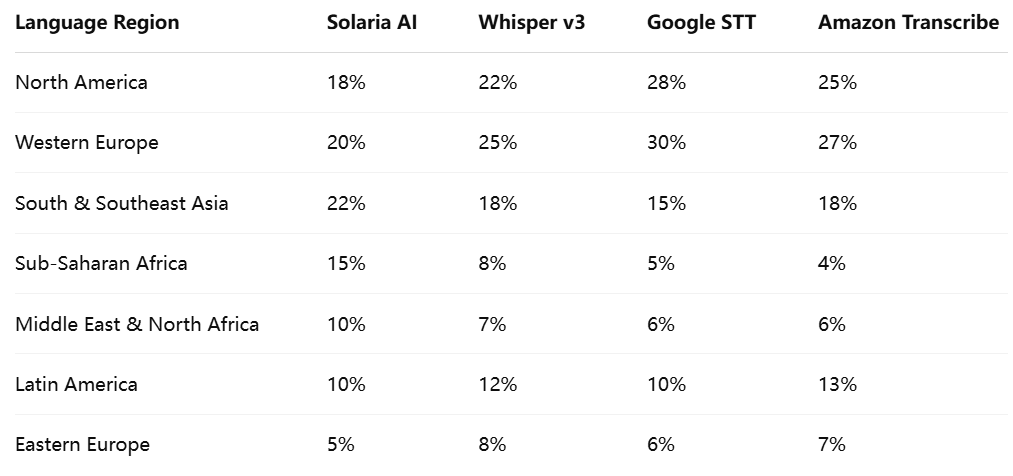
Note: Data distribution shown as a percentage of total training corpus by regional source.
This diverse representation ensures that Solaria AI can better accommodate different accents, speech rates, and cultural references, reducing transcription bias and improving inclusion across user demographics.
Edge Deployment vs. Cloud-Based Processing
A further dimension of adaptability lies in deployment architecture. While cloud-based models provide scalability and processing power, they require internet connectivity and raise potential concerns over data privacy, latency, and compliance.
Edge deployment, by contrast, enables real-time transcription on-device or on-premises. This is particularly valuable in scenarios such as:
- Healthcare: Where patient data privacy is critical.
- Education: In bandwidth-limited rural or remote regions.
- Field operations: Such as disaster response or agriculture, where connectivity is unreliable.
Solaria AI supports hybrid deployment, allowing clients to choose between full-cloud processing, edge inference with model quantization, or hybrid pipelines where audio is preprocessed locally and refined in the cloud. This flexibility enhances adoption in regulated industries and resource-constrained environments alike.
Open-Source vs. Proprietary Models: Strategic Considerations
Enterprises evaluating STT solutions must also consider the implications of open-source versus proprietary model licensing. Open-source models, such as Whisper, offer transparency and customization potential but may require extensive tuning and infrastructure management. Proprietary models, such as Solaria AI, provide higher performance out of the box, along with enterprise-grade support and service-level agreements (SLAs).
Solaria AI combines proprietary technology with developer-friendly APIs and model introspection tools, allowing enterprises to benefit from high accuracy while retaining control over performance parameters and compliance requirements.
Architectures that Empower Global Usability
The strength of a multilingual STT model is not merely a product of data volume or algorithmic sophistication—it is a function of architectural vision, training strategy, and operational flexibility. Solaria AI exemplifies a new generation of systems that balance deep learning innovation with global inclusivity and enterprise adaptability.
Through its multi-scale architecture, multilingual self-supervised training, domain customization, and equitable data curation, Solaria AI positions itself as a scalable solution for speech transcription across geographies, sectors, and user needs.
Business, Legal, and Deployment Considerations
As multilingual speech-to-text (STT) technologies mature and gain widespread adoption across industries, the decision to integrate such models into enterprise workflows is no longer a purely technical matter. It requires a holistic evaluation of commercial, legal, and operational implications. Choosing an STT model—whether for real-time transcription, voice-based interfaces, customer support analytics, or content localization—entails an assessment of not only performance metrics but also licensing costs, compliance frameworks, integration feasibility, and long-term vendor sustainability.
This section outlines the critical business, legal, and deployment considerations that organizations must weigh when adopting multilingual STT solutions such as Solaria AI. By understanding the broader operational landscape, decision-makers can make informed investments that align with organizational goals, risk appetites, and regulatory responsibilities.
Licensing and Cost Structure
The cost of deploying a multilingual STT system can vary dramatically depending on the vendor, usage model, and level of customization required. Vendors typically offer one or more of the following pricing structures:
- Per-minute or per-hour transcription fees: Common in cloud-based STT APIs where pricing is based on the duration of the audio transcribed.
- Subscription-based licensing: Offers predictable monthly or annual costs with usage caps or tiered service levels.
- Enterprise licensing agreements (ELAs): Tailored contracts that provide flat-rate or usage-based pricing for large-scale deployments, often including SLAs and dedicated support.
- On-premises or edge deployment licenses: Include one-time or annual fees for offline model usage, often with enhanced privacy guarantees.
Solaria AI provides flexible pricing models that cater to diverse business needs, including volume-based discounts for high-throughput applications, usage metering for burst workloads, and dedicated licenses for secure edge deployments. Additionally, its model tuning and domain customization services are available as optional add-ons, enabling businesses to align features with budgetary constraints.
Transparent cost estimation and forecasting tools are critical in preventing unexpected overages. Enterprises are advised to review not only baseline costs but also secondary expenses related to cloud storage, API calls, bandwidth, and compute infrastructure.
Data Privacy, Security, and Regulatory Compliance
One of the most consequential aspects of deploying STT models is compliance with data protection regulations, especially when dealing with personally identifiable information (PII), health records, financial conversations, or customer service interactions.
Key regulations affecting STT deployments include:
- General Data Protection Regulation (GDPR) (EU): Requires data minimization, user consent, right to access, and data deletion policies.
- California Consumer Privacy Act (CCPA) (US): Grants California residents rights to access, delete, and opt out of the sale of personal data.
- Health Insurance Portability and Accountability Act (HIPAA) (US): Mandates data protection for health-related information.
- Personal Data Protection Bill (PDPB) (India): Establishes consent-based data processing and cross-border data transfer regulations.
Solaria AI complies with leading international privacy frameworks and offers geo-fenced data storage, encryption at rest and in transit, and anonymization features to protect user identities. It also supports on-premises deployment for clients in highly regulated sectors such as finance, healthcare, and government, where cloud-based transcription may be restricted by law or organizational policy.
For legal assurance, vendors should provide Data Processing Agreements (DPAs), audit logs, and compliance certifications such as ISO/IEC 27001, SOC 2, and HIPAA readiness reports. These artifacts help organizations demonstrate due diligence in vendor selection and data stewardship.
Integration with Enterprise Systems
The utility of an STT model is greatly enhanced by its ability to integrate with existing business systems. In practice, this means seamless connectivity with:
- Customer Relationship Management (CRM) tools: To transcribe and analyze client interactions.
- Content Management Systems (CMS): For automatic transcription of multimedia assets.
- Data Warehouses and Analytics Platforms: To feed transcribed speech into analytics workflows and dashboards.
- Collaboration Tools: Such as Zoom, Microsoft Teams, and Slack for real-time captioning and meeting transcripts.
- Contact Center Platforms: To support agent assist, quality assurance, and sentiment analysis.
Solaria AI provides RESTful APIs, webhooks, and SDKs for multiple programming environments (Python, Java, Node.js). It also supports integration with popular cloud ecosystems like AWS, Azure, and GCP, enabling scalable, cloud-native workflows. Importantly, its event-driven architecture allows low-latency interactions with third-party services, crucial for real-time use cases.
Enterprises should prioritize STT solutions that offer API versioning, rate limit transparency, developer documentation, and sandbox environments for testing and deployment.
Scalability and Infrastructure Optimization
Scalability is a critical factor in STT deployment, especially for organizations with variable demand patterns or global footprints. A model that performs well under test conditions may falter under production-scale loads unless it is architected for elasticity and distributed computing.
Key scalability considerations include:
- Concurrency limits: The number of simultaneous transcription jobs that can be processed.
- Load balancing: Efficient distribution of tasks across nodes to prevent bottlenecks.
- Horizontal scalability: Ability to scale across regions and zones.
- Batch vs. streaming support: Support for both offline (batch) and live (streaming) transcriptions.
Solaria AI is designed for elastic scaling and can handle thousands of concurrent transcription sessions through container orchestration systems such as Kubernetes. For edge applications, it offers model quantization and low-memory inference for deployment on resource-constrained devices like smartphones, tablets, or IoT hardware.
Vendor Reliability and Support
Technical excellence alone is insufficient in long-term vendor partnerships. Enterprises must consider the operational maturity and reliability of the STT provider. Indicators of a dependable vendor include:
- Service-Level Agreements (SLAs): Clearly defined performance and uptime commitments.
- 24/7 support availability: Essential for mission-critical applications.
- Dedicated customer success teams: To assist with onboarding, troubleshooting, and optimization.
- Change management policies: Including backward-compatible updates and transparent release notes.
- Community and developer engagement: Forums, webinars, and support tickets that foster feedback and innovation.
Solaria AI maintains a robust customer success program, including tiered support plans, incident response SLAs, and technical account managers for high-volume clients. It also publishes regular model updates and performance improvements, giving enterprises visibility into roadmap milestones and upcoming features.
Choosing a vendor with a proven track record and a commitment to enterprise service ensures continuity, stability, and long-term value from the STT deployment.
Strategic Adoption Beyond Accuracy
Integrating multilingual STT technology is no longer a question of whether the model transcribes words correctly—it is a multidimensional business decision that touches every layer of an organization’s operations. From cost modeling and regulatory compliance to integration readiness and vendor trust, enterprises must conduct thorough due diligence to ensure a successful deployment.
Solaria AI stands out not only for its technical proficiency but also for its commercial viability. With a flexible licensing model, strong privacy guarantees, API-first design, and high scalability, it offers a comprehensive solution for organizations seeking multilingual speech recognition that is both performant and enterprise-ready.
As enterprises increasingly rely on voice data for insights, automation, and accessibility, selecting the right STT partner becomes a strategic imperative. By aligning technical capabilities with legal compliance, business workflows, and organizational values, decision-makers can unlock the full potential of multilingual STT—positioning speech not merely as an input modality, but as a cornerstone of intelligent digital infrastructure.
Conclusion
The proliferation of multilingual speech-to-text (STT) models marks a significant inflection point in the evolution of human-computer interaction. As enterprises, governments, and developers expand their digital footprints into linguistically diverse regions, the capacity to accurately transcribe and interpret spoken language in multiple tongues has become both a technological necessity and a cultural imperative. Multilingual STT is no longer a supplementary feature; it is foundational to accessibility, automation, and engagement in the global digital ecosystem.
Throughout this analysis, we have examined the multifaceted criteria for evaluating multilingual STT systems, with a specific focus on models such as Solaria AI, which exemplify the next generation of context-aware, culturally sensitive, and domain-adaptable speech recognition technologies.
We began by exploring the evolution of STT systems, tracing the shift from monolingual, rules-based frameworks to neural architectures capable of handling complex, code-switched, and dialectically diverse inputs. This historical perspective underscores how far the technology has advanced—and how rapidly expectations have evolved alongside it.
Subsequently, we dissected the core evaluation metrics that distinguish performant multilingual STT models. While traditional benchmarks such as Word Error Rate (WER) remain important, they are insufficient in isolation. Instead, a more holistic set of criteria—including accent robustness, latency, domain adaptability, and code-switching proficiency—provides a more accurate reflection of a model’s real-world utility.
The linguistic and cultural nuances of multilingual speech further complicate the transcription task. Human speech is richly layered with idiomatic expressions, prosodic patterns, and sociolectal variation, all of which must be interpreted with care to preserve intended meaning. STT models that aspire to global applicability must therefore transcend lexical transcription to become instruments of cultural translation—recognizing that to transcribe is also to interpret.
We then delved into the architectural foundations and training paradigms that enable models like Solaria AI to achieve scalable, inclusive, and high-performance results. Innovations in transformer-based modeling, multilingual pretraining, self-supervised learning, and fine-tuning have allowed developers to build models that generalize across languages while remaining adaptable to specific domains and user needs. The importance of diverse and ethically sourced training data was also emphasized, as equitable representation is a prerequisite for fair and reliable AI systems.
Finally, we examined the business, legal, and deployment dimensions that enterprises must consider when integrating multilingual STT solutions into operational environments. From licensing structures and API integration to compliance with GDPR, HIPAA, and CCPA, the successful deployment of STT systems hinges not only on technical excellence but also on vendor trust, service-level reliability, and legal transparency.
In this comprehensive evaluation, Solaria AI emerges as a leader in multilingual STT due to its commitment to accuracy, adaptability, inclusivity, and enterprise readiness. Its modular architecture, global data sourcing, and customizable deployment models make it particularly well-suited for organizations seeking scalable voice solutions across languages and regions.
Looking Forward
The future of multilingual STT lies in greater semantic understanding, real-time translation, and cross-modal integration. Emerging technologies will not only transcribe speech but also interpret emotion, intent, and context—enabling applications in empathy-driven AI, personalized education, and multilingual virtual collaboration. We can also anticipate deeper low-resource language support, powered by transfer learning and synthetic data generation, as the global community demands greater linguistic inclusion.
As voice continues to eclipse text in many interaction domains—from mobile to automotive to immersive environments—enterprises that invest in sophisticated STT infrastructure today will be well-positioned to lead tomorrow’s digital transformation. By evaluating solutions like Solaria AI through the lens of linguistic fidelity, operational scalability, and ethical integrity, organizations can ensure that speech recognition not only meets technical benchmarks but also upholds the values of inclusivity, accessibility, and global understanding.
Reference
- Google Cloud Speech-to-Text Documentation.
https://cloud.google.com/speech-to-text - Microsoft Azure Speech-to-Text Overview.
https://azure.microsoft.com/services/cognitive-services/speech-to-text - Amazon Transcribe: Automatic Speech Recognition. Amazon Web Services.
https://aws.amazon.com/transcribe - Robust Speech Recognition via Large-Scale Weak Supervision.
https://openai.com/blog/whisper - Wav2Vec 2.0: Self-supervised learning for speech recognition.
https://ai.facebook.com/blog/wav2vec-20-learning-the-structure-of-speech-from-raw-audio - IBM Watson Speech to Text Service.
https://www.ibm.com/cloud/watson-speech-to-text - Common Voice: Open-source speech datasets. Mozilla.
https://commonvoice.mozilla.org - Speech and Language Processing for Multilingual Applications.
https://developer.nvidia.com/speech-recognition - Code-Switching in Multilingual Speech Recognition.
https://spectrum.ieee.org/code-switching-speech-recognition - GDPR Compliance for Speech-to-Text Solutions.
https://gdpr.eu/data-processing