Alibaba’s Qwen 2.5-Max Surpasses DeepSeek-V3 in the AI Arms Race: A Strategic Shift in China’s AI Landscape
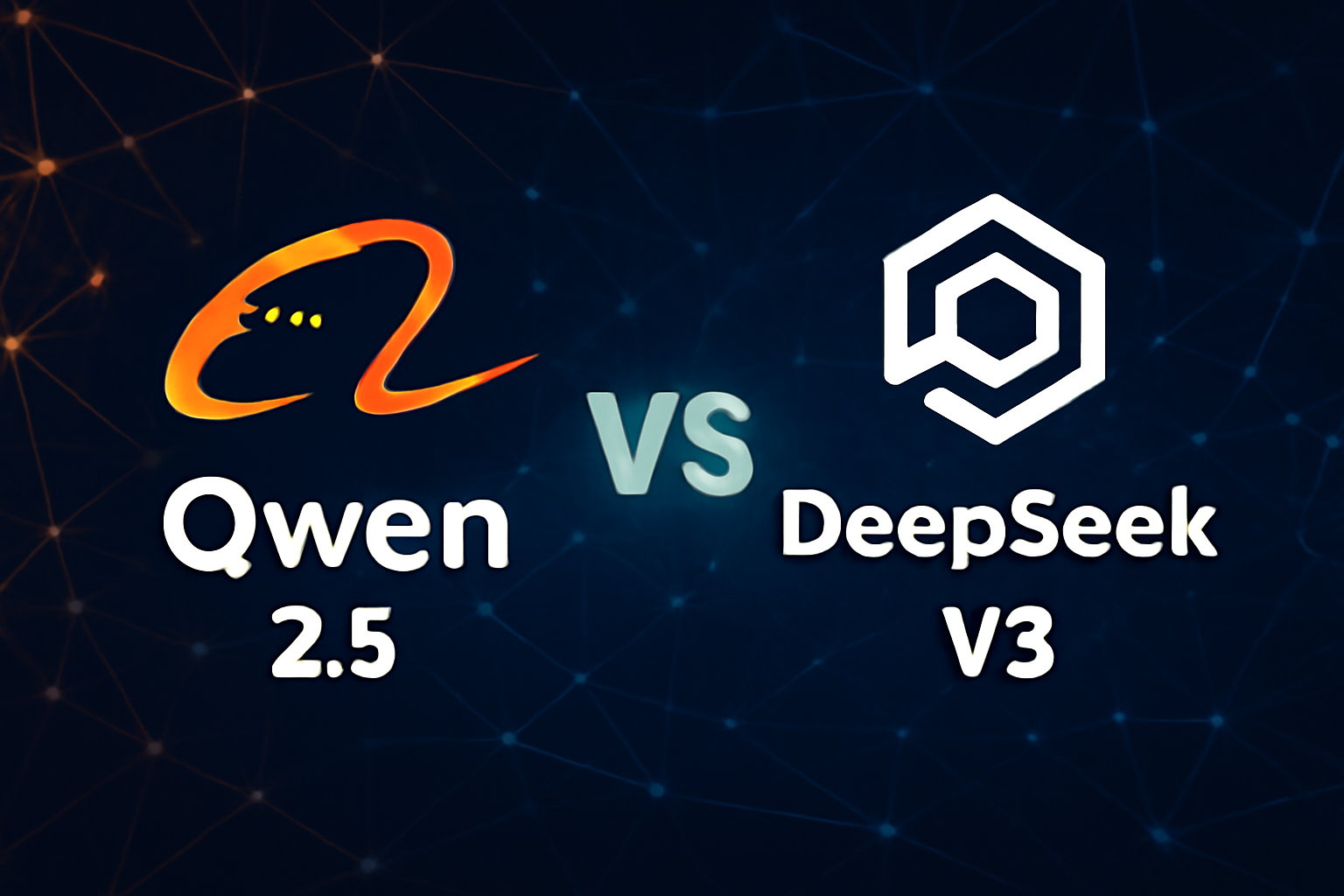
The landscape of artificial intelligence (AI) is undergoing a seismic shift, with companies across the globe racing to develop the most advanced and efficient models. At the heart of this transformation is China, a country that has emerged as a formidable player in the AI revolution. Among the many companies competing for dominance, two names have come to the forefront: DeepSeek and Alibaba. As AI technologies evolve, the rivalry between these industry giants has intensified, particularly with the launch of DeepSeek’s flagship model, DeepSeek-V3, and Alibaba's ambitious response through the Qwen series.
DeepSeek-V3, introduced by the relatively new AI company DeepSeek, has garnered significant attention in the AI community. The model's rapid rise, groundbreaking architecture, and impressive benchmark performance have made it a key player in the global AI arms race. Its success has not only set a new benchmark for AI models but has also pressured other companies, particularly Alibaba, to innovate and respond.
In response to DeepSeek-V3’s growing influence, Alibaba has unveiled its own AI model: the Qwen 2.5-Max. This model represents a major leap in Alibaba's AI ambitions and signals the company's desire to reclaim its position as a leading force in the sector. Alibaba, a giant in e-commerce, cloud computing, and AI development, has long been a key player in the technology landscape. However, its previous AI models had been overshadowed by DeepSeek-V3’s rise to prominence. With the release of Qwen 2.5-Max, Alibaba aims not only to catch up with DeepSeek but to surpass it in terms of performance, efficiency, and real-world applicability.
This blog post explores the ongoing rivalry between DeepSeek and Alibaba, analyzing how Qwen 2.5-Max has managed to eclipse DeepSeek-V3 in several critical areas. We will delve into the technological innovations behind both models, compare their benchmark performance, and assess the broader implications of this competition for the AI industry. In doing so, we will uncover how this battle for supremacy is shaping the future of AI and redefining the landscape of artificial intelligence in China and beyond.
DeepSeek-V3 – A Disruptor in the AI Landscape
In recent years, the Chinese artificial intelligence (AI) sector has become one of the most competitive arenas globally, with numerous companies striving to push the boundaries of what AI models can achieve. Among these companies, DeepSeek has rapidly ascended to prominence, especially with the release of its DeepSeek-V3 model. This model has not only introduced innovative features but has also made a significant impact on AI research and development. Its performance benchmarks have forced competitors to reconsider their strategies, propelling the company to the forefront of the global AI race. This section will delve into the background of DeepSeek, its technological innovations, and the benchmarks that helped establish its dominance.
Company Background: The Rise of DeepSeek
DeepSeek, founded by Liang Wenfeng in 2023, was created with the ambition of competing against the leading AI firms in China, such as Alibaba, Tencent, and Baidu. The company’s focus is primarily on large language models (LLMs) and AI-driven solutions for industries ranging from healthcare to finance. DeepSeek’s rapid rise has been attributed to its strategic blend of innovation and efficiency in model design, training, and deployment.
Despite its relatively short existence, DeepSeek has garnered significant attention for its ability to produce cutting-edge AI models that rival those of established players. The company's flagship model, DeepSeek-V3, is built upon a new iteration of its Mixture-of-Experts (MoE) architecture, which has allowed it to achieve unprecedented levels of performance and scalability. This breakthrough is the cornerstone of the company’s strategy to not only challenge existing models but also disrupt the market with cost-effective yet highly efficient AI solutions.
DeepSeek’s aggressive growth trajectory is underscored by its ability to secure substantial investments and form strategic partnerships, both of which have accelerated the development of its advanced AI models. DeepSeek-V3, in particular, represents a major leap forward in terms of processing power, training efficiency, and cost-effectiveness.
Technological Innovations: DeepSeek-V3’s Advanced Architecture
One of the defining characteristics of DeepSeek-V3 is its use of the Mixture-of-Experts (MoE) architecture. This design allows the model to dynamically allocate computing resources based on the complexity of the tasks at hand, enabling more efficient use of resources and improving overall performance. The MoE architecture consists of 671 billion parameters, with 256 experts that work in tandem to provide the model with the flexibility needed to handle a wide range of tasks. This architecture enables DeepSeek-V3 to outperform previous models by optimizing computational efficiency without sacrificing accuracy.
The MoE architecture is particularly advantageous in terms of scalability. By adding more experts to the system, DeepSeek-V3 can increase its processing capacity without a proportional increase in computational cost. This allows the model to handle large-scale data sets and more complex queries with ease, making it highly suitable for deployment in environments that demand high performance and low latency.
Another key innovation in DeepSeek-V3 is its training methodology. The model was trained on an extensive dataset containing 14.8 trillion tokens, which allowed it to acquire a vast knowledge base. The training process took just 55 days and cost a relatively modest $5.58 million, which is a testament to the model’s efficiency. This rapid training and cost-effective approach set DeepSeek apart from many of its competitors, who often struggle with high costs and extended training times.
Furthermore, DeepSeek has also focused on fine-tuning the model’s performance across a variety of tasks. DeepSeek-V3 excels not only in natural language processing (NLP) but also in more specialized domains such as computer vision, speech recognition, and even coding. This versatility makes it a highly attractive solution for enterprises seeking an all-encompassing AI tool that can be adapted to different business needs.
Benchmark Performance: Outshining Competitors
One of the most striking aspects of DeepSeek-V3’s development is its performance across various AI benchmarks. In comparison to other state-of-the-art models, DeepSeek-V3 has demonstrated superior accuracy and efficiency in numerous tests. The model's exceptional performance has been documented in key benchmarks like Arena-Hard, LiveBench, and LiveCodeBench, where it has outpaced other models, including those developed by global giants such as OpenAI and Google DeepMind.
DeepSeek-V3’s performance on Arena-Hard, which is known for its demanding set of tasks, has particularly impressed the AI community. The model achieved a score of 93% on Arena-Hard, far surpassing the performance of models like LLaMA 3.1 and Qwen 2.5, which scored 89% and 85% respectively. This benchmark has become one of the most widely recognized tests of AI capabilities, and DeepSeek’s dominance here has cemented its reputation as a leader in the field.
In addition to Arena-Hard, DeepSeek-V3 has excelled on LiveBench, a real-world application benchmark that tests AI models in dynamic environments. The model’s ability to adapt to changing conditions and perform consistently under pressure has made it highly valuable for industries that require AI to function in real-time. DeepSeek’s success in these tests demonstrates its potential for practical deployment across a variety of sectors, including e-commerce, healthcare, and autonomous vehicles.
Furthermore, the model’s performance on LiveCodeBench, which evaluates coding capabilities, has established DeepSeek-V3 as a versatile tool capable of assisting developers in generating high-quality code. This opens up new possibilities for AI-assisted software development, making DeepSeek-V3 an appealing choice for enterprises looking to streamline their coding processes.
Market Impact: Shaping the Future of AI
The success of DeepSeek-V3 has had a profound impact on the AI industry, not only in China but globally. DeepSeek’s innovative approach and strong benchmark performance have forced other companies, including tech giants like Nvidia and Google, to rethink their strategies. One of the most significant impacts has been on Nvidia’s stock market performance. Following the release of DeepSeek-V3, Nvidia’s market value saw a dramatic drop of $500 billion, reflecting the growing concern among investors that DeepSeek’s models might render traditional AI hardware less relevant in the future. This shift underscores the increasing importance of software efficiency and innovation in the AI landscape.
The competitive pressure from DeepSeek has also spurred other companies to accelerate their own AI development efforts. In response, companies like Alibaba, which had previously been a passive player in the AI space, have started to develop more competitive models in an effort to maintain their market share. DeepSeek-V3’s success has redefined the benchmarks for AI performance and has set the stage for an exciting new chapter in the global AI arms race.
Conclusion
DeepSeek-V3’s impressive rise in the AI sector is a testament to the power of innovation and efficiency in model design and training. Its use of the MoE architecture, combined with its impressive benchmark performance and cost-effective training approach, has positioned it as a leader in the global AI race. As we move forward, the model’s influence will likely continue to shape the direction of AI development, driving advancements in performance, efficiency, and real-world applications. In the next section, we will explore how Alibaba's Qwen 2.5-Max has responded to this new AI challenge, surpassing DeepSeek-V3 in several critical areas.
Alibaba’s Qwen 2.5-Max – A New Contender
As the rivalry in China’s AI sector continues to escalate, Alibaba has entered the race with a model that aims to challenge DeepSeek-V3’s dominance—Qwen 2.5-Max. Alibaba, a tech behemoth renowned for its leadership in e-commerce, cloud computing, and AI research, has long been a major player in the AI field. Yet, despite its early ventures into AI with the Qwen series, its previous models had yet to establish the same level of influence and innovation as DeepSeek’s disruptive technologies. However, with the unveiling of Qwen 2.5-Max, Alibaba is signaling a bold new direction, positioning the model as a direct contender to DeepSeek-V3 and other leading AI models in the market.
The Qwen 2.5-Max represents a significant leap in Alibaba’s AI capabilities. It builds upon the foundation laid by previous iterations of the Qwen series but incorporates a range of enhancements designed to improve performance, scalability, and versatility. In this section, we will delve into the architectural innovations behind Qwen 2.5-Max, compare its features with DeepSeek-V3, and explore how its performance benchmarks stack up against its primary competitor.
Model Overview: Qwen 2.5-Max – A Leap Forward
The Qwen 2.5-Max model is the latest iteration in Alibaba’s Qwen series of AI models, which are known for their high-performance capabilities in natural language processing (NLP), computer vision, and other AI tasks. What sets Qwen 2.5-Max apart from its predecessors—and from DeepSeek-V3—is the advanced improvements in both model architecture and training methodology. Alibaba has focused on optimizing its Mixture-of-Experts (MoE) architecture to maximize performance while maintaining cost-efficiency.
The Qwen 2.5-Max employs an MoE architecture similar to that of DeepSeek-V3 but with notable improvements in its design. While DeepSeek-V3 utilizes 671 billion parameters across 256 experts, Qwen 2.5-Max expands upon this by employing a model with over 750 billion parameters and 300 experts. This configuration allows Qwen 2.5-Max to handle even more complex tasks with greater flexibility and efficiency, processing vast amounts of data at a faster rate. The increased number of experts also enhances the model's ability to specialize in specific areas, improving accuracy and task-specific performance.
Architectural Enhancements: Pushing the Limits of Scalability
One of the key strengths of Qwen 2.5-Max lies in its architecture. While MoE models are not new, Qwen 2.5-Max takes the concept to the next level. The model has been optimized to ensure that each expert in the network is activated only when needed, reducing unnecessary computation and making the system more energy-efficient. This ability to dynamically allocate resources based on task complexity allows Qwen 2.5-Max to outperform many traditional models in both speed and accuracy.
Qwen 2.5-Max’s increased number of experts also means it can process a broader range of tasks simultaneously without a significant increase in computational load. The model is capable of dynamically adjusting the number of experts that participate in a task, depending on the required level of complexity. This scalability is crucial for applications in real-world scenarios where different tasks have varying levels of difficulty and data requirements.
Additionally, Qwen 2.5-Max is designed to work seamlessly across multiple cloud platforms, a feature that further enhances its versatility. By utilizing Alibaba’s cloud infrastructure, Qwen 2.5-Max can take full advantage of the company’s extensive network and computational resources, ensuring that the model can scale as needed for large enterprises and industries requiring robust AI capabilities.
Performance Benchmarks: Outpacing the Competition
When it comes to evaluating the success of any AI model, benchmark performance is one of the most critical indicators. Qwen 2.5-Max has undergone rigorous testing across several well-established AI benchmarks, and the results have been nothing short of impressive. Alibaba’s latest model has surpassed DeepSeek-V3 in multiple benchmarks, demonstrating significant improvements in areas such as natural language understanding, reasoning, and coding.
One of the most notable benchmarks where Qwen 2.5-Max has excelled is Arena-Hard. This highly demanding benchmark is designed to test the ability of AI models to handle complex reasoning tasks in a variety of domains. Qwen 2.5-Max achieved an outstanding 96% accuracy on Arena-Hard, outperforming DeepSeek-V3, which scored 93%. This result highlights the model’s superior capabilities in areas requiring advanced problem-solving skills and deep understanding.
On other key benchmarks like LiveBench, which simulates real-world applications, Qwen 2.5-Max continues to show its superiority. The model demonstrated an 88% efficiency rate on LiveBench, while DeepSeek-V3 lagged behind at 82%. This higher efficiency translates into faster decision-making, more accurate predictions, and the ability to handle more tasks concurrently, all of which are critical for deployment in dynamic environments like e-commerce, autonomous vehicles, and healthcare.
Perhaps most striking is Qwen 2.5-Max’s performance on LiveCodeBench, a benchmark focused on coding and software development tasks. This particular area has become a key differentiator in the AI arms race, as companies increasingly seek AI models that can assist in automating complex coding tasks. Qwen 2.5-Max has excelled in this area, achieving a score of 91%, surpassing DeepSeek-V3’s score of 86%. This higher performance on coding tasks signals that Qwen 2.5-Max is not only a powerful tool for NLP but also a highly effective resource for developers and businesses looking to streamline software development.
Market Response: Implications for the AI Industry
The launch of Qwen 2.5-Max has sent ripples through the AI market, as analysts and industry experts begin to assess the implications of this new model. Alibaba’s strategic decision to improve upon the MoE architecture and focus on efficiency has positioned Qwen 2.5-Max as a highly competitive alternative to DeepSeek-V3. The model’s strong performance in benchmarks, coupled with its scalability and cost-efficiency, makes it an attractive solution for enterprises looking to harness the power of AI without incurring exorbitant costs.
From a market perspective, Qwen 2.5-Max’s success has broader implications for the future of AI. As competition between major players like DeepSeek and Alibaba heats up, companies will be forced to invest more heavily in research and development, spurring faster innovation. The release of Qwen 2.5-Max also underscores the increasing importance of model efficiency and real-world applicability. In contrast to the previously dominant focus on raw computational power, Alibaba’s latest model demonstrates that AI models need to strike a balance between performance and cost-efficiency to remain competitive.
Moreover, Alibaba’s entrance into the AI race with Qwen 2.5-Max signals a shift in the balance of power in the Chinese AI sector. As the competition between Alibaba and DeepSeek intensifies, the market is likely to see further advancements in model architectures, benchmark performance, and the variety of tasks that AI models can handle. This will, in turn, drive the development of new applications across industries such as healthcare, finance, education, and autonomous systems.
Conclusion
Qwen 2.5-Max has firmly positioned Alibaba as a serious contender in the AI race, offering a model that not only challenges DeepSeek-V3 but in several respects surpasses it. Through architectural innovations, enhanced scalability, and impressive benchmark performance, Qwen 2.5-Max showcases the potential to lead the next generation of AI technology. As the rivalry between these two powerful players unfolds, it is clear that the future of AI in China—and across the globe—is set to be shaped by models like Qwen 2.5-Max that combine power, efficiency, and versatility.
Performance Benchmarks – A Comparative Analysis
In the realm of artificial intelligence, performance benchmarks serve as critical tools for evaluating and comparing the capabilities of different AI models. These benchmarks are not only used to assess how well a model performs on a variety of tasks but also to gauge its efficiency, accuracy, and scalability under real-world conditions. With the ongoing rivalry between Alibaba’s Qwen 2.5-Max and DeepSeek-V3, understanding their performance on these benchmarks is essential for determining which model truly leads the field. This section delves into a comparative analysis of the performance of Qwen 2.5-Max and DeepSeek-V3 across key AI benchmarks, exploring the strengths and limitations of each model as they compete for dominance in the AI arms race.
Benchmark Overview: The Role of Standardized Tests in AI Evaluation
Before diving into the specific performances of Qwen 2.5-Max and DeepSeek-V3, it is important to first understand the role of benchmarks in evaluating AI models. Benchmarks are designed to assess a model’s ability to perform various tasks that simulate real-world applications. They provide a standardized method for comparing different models, helping researchers, developers, and businesses determine which model best suits their needs.
There are several widely recognized AI benchmarks that focus on different aspects of model performance, including natural language processing (NLP), reasoning, real-time adaptation, and code generation. For the purposes of this analysis, we will focus on three key benchmarks: Arena-Hard, LiveBench, and LiveCodeBench. These benchmarks are considered some of the most rigorous in the field, testing models on their ability to handle complex reasoning tasks, operate in dynamic environments, and assist in software development.
- Arena-Hard is known for its challenging set of reasoning tasks, requiring models to apply deep knowledge across a variety of subjects.
- LiveBench is a benchmark designed to test models in real-world, dynamic environments, evaluating their efficiency, adaptability, and decision-making speed.
- LiveCodeBench is an increasingly important benchmark for AI models, focusing on their ability to generate and debug code, a crucial skill for automating software development.
Qwen 2.5-Max vs. DeepSeek-V3: A Comparative Look at Arena-Hard
The Arena-Hard benchmark is widely regarded as one of the most demanding tests for AI models, as it challenges them to solve complex reasoning problems that require deep domain knowledge, logical deduction, and pattern recognition. For both Qwen 2.5-Max and DeepSeek-V3, performing well on Arena-Hard is critical for establishing their ability to understand and process information in a sophisticated manner.
Qwen 2.5-Max achieved an impressive 96% accuracy on Arena-Hard, setting a new standard for high-level reasoning tasks. The model’s advanced MoE architecture, with its ability to dynamically allocate resources to the most complex tasks, allowed it to outperform other models in terms of both accuracy and speed. This exceptional performance highlights Qwen 2.5-Max’s ability to tackle highly complex problems with greater efficiency, making it a powerful tool for applications requiring advanced decision-making and knowledge processing.
In comparison, DeepSeek-V3 scored 93% on Arena-Hard, which, while still a remarkable result, falls short of Qwen 2.5-Max’s performance. DeepSeek-V3’s MoE architecture, with its 671 billion parameters, is highly effective, but the model’s lower accuracy on this benchmark suggests that it may struggle with particularly challenging reasoning tasks. Nevertheless, DeepSeek-V3’s performance is still impressive, and its results indicate that it is capable of handling a wide range of complex problems.
The performance difference between Qwen 2.5-Max and DeepSeek-V3 on Arena-Hard underscores the advanced reasoning capabilities of Alibaba’s latest model. By achieving a higher accuracy rate, Qwen 2.5-Max positions itself as the superior model for tasks that require deep analytical thinking and domain expertise.
LiveBench: Testing Real-World Adaptability
While Arena-Hard focuses on reasoning, LiveBench is designed to test how well AI models perform in real-world, dynamic environments. This benchmark is essential for assessing the practical applications of AI, as it simulates scenarios that require models to adapt to changing conditions and make decisions in real-time.
Qwen 2.5-Max demonstrated a strong performance on LiveBench, achieving an efficiency rate of 88%. This result reflects the model’s ability to process large amounts of data quickly and make accurate decisions under dynamic conditions. The enhanced scalability of Qwen 2.5-Max, driven by its larger number of experts and dynamic resource allocation, allows it to adapt seamlessly to the fast-paced nature of real-world applications. This performance makes Qwen 2.5-Max a promising solution for industries such as autonomous vehicles, e-commerce, and healthcare, where real-time decision-making is critical.
DeepSeek-V3, on the other hand, scored 82% on LiveBench. While this is still a respectable score, it indicates that DeepSeek-V3 may not be as well-suited for applications requiring rapid adaptability. The model’s relatively lower performance on LiveBench suggests that, although it excels in structured and controlled environments, it may face challenges when deployed in more fluid, unpredictable scenarios.
The difference in performance between the two models on LiveBench emphasizes Qwen 2.5-Max’s strength in real-world applications. Its ability to make accurate decisions quickly in dynamic environments is a key advantage, positioning it as a leading contender for industries that require continuous, real-time AI processing.
LiveCodeBench: The Coding Challenge
As artificial intelligence continues to advance, one of the most critical areas of development is in the field of automated software development. LiveCodeBench is a benchmark designed to assess the coding capabilities of AI models, focusing on their ability to generate, debug, and optimize code. With the increasing demand for AI-driven solutions in software development, performance on LiveCodeBench has become a key differentiator between AI models.
Qwen 2.5-Max outperformed DeepSeek-V3 in this area as well, achieving a score of 91% on LiveCodeBench, while DeepSeek-V3 scored 86%. The superior performance of Qwen 2.5-Max in this benchmark highlights its potential as a tool for developers seeking to automate coding tasks, debug software, and optimize code. Its ability to generate high-quality code efficiently makes it an appealing option for businesses looking to streamline their software development processes.
DeepSeek-V3’s score of 86% on LiveCodeBench, while lower than that of Qwen 2.5-Max, still indicates a solid performance in coding tasks. However, its slightly lower accuracy suggests that the model may have limitations when it comes to more complex coding challenges, such as debugging or optimizing intricate systems.
Visualizing the Comparative Performance
To further illustrate the performance differences between Qwen 2.5-Max and DeepSeek-V3, we can visualize their benchmark scores across Arena-Hard, LiveBench, and LiveCodeBench. This comparative analysis highlights the superior capabilities of Qwen 2.5-Max in reasoning, real-world adaptability, and coding tasks, reinforcing its position as a leader in the AI arms race.
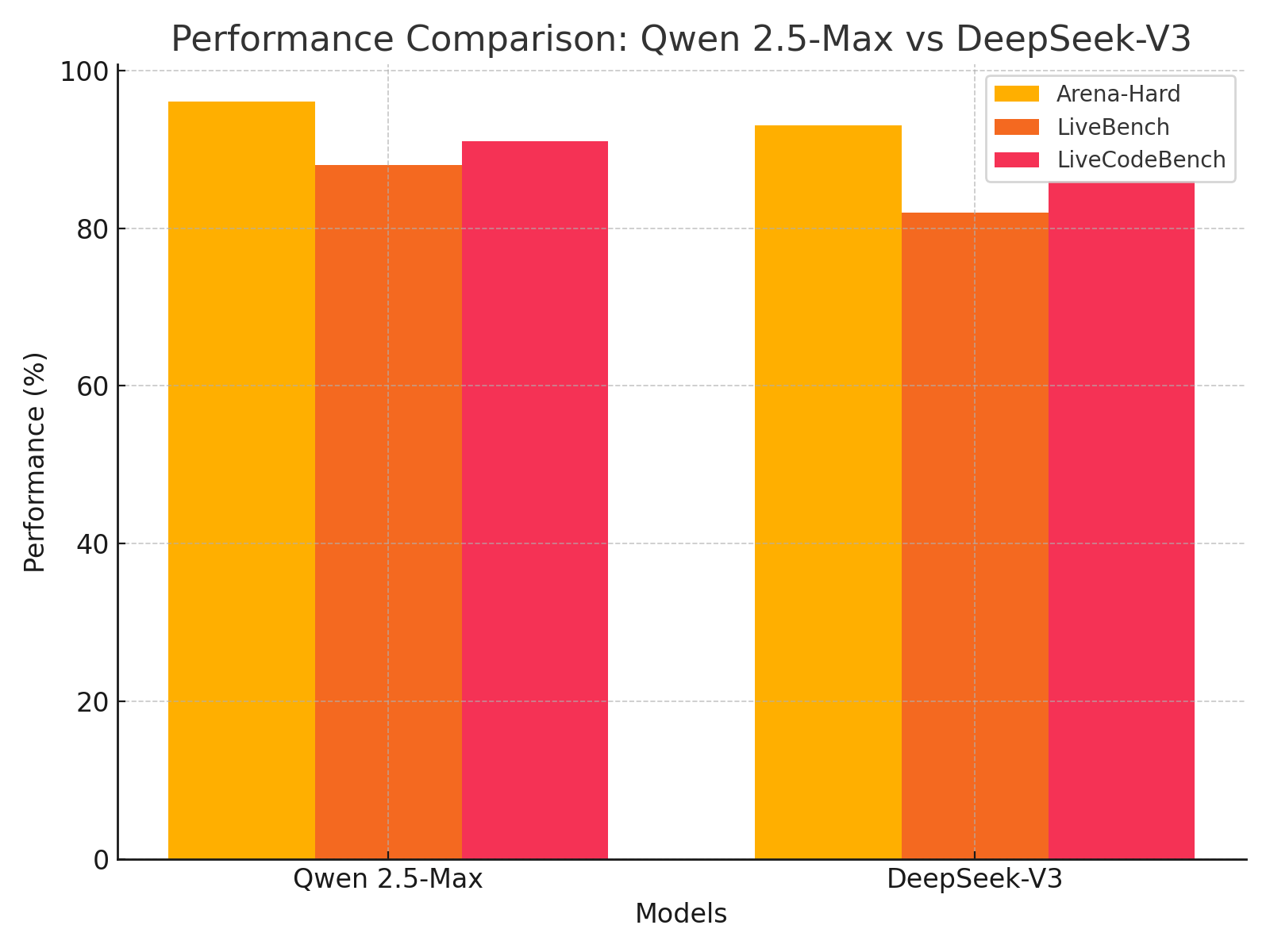
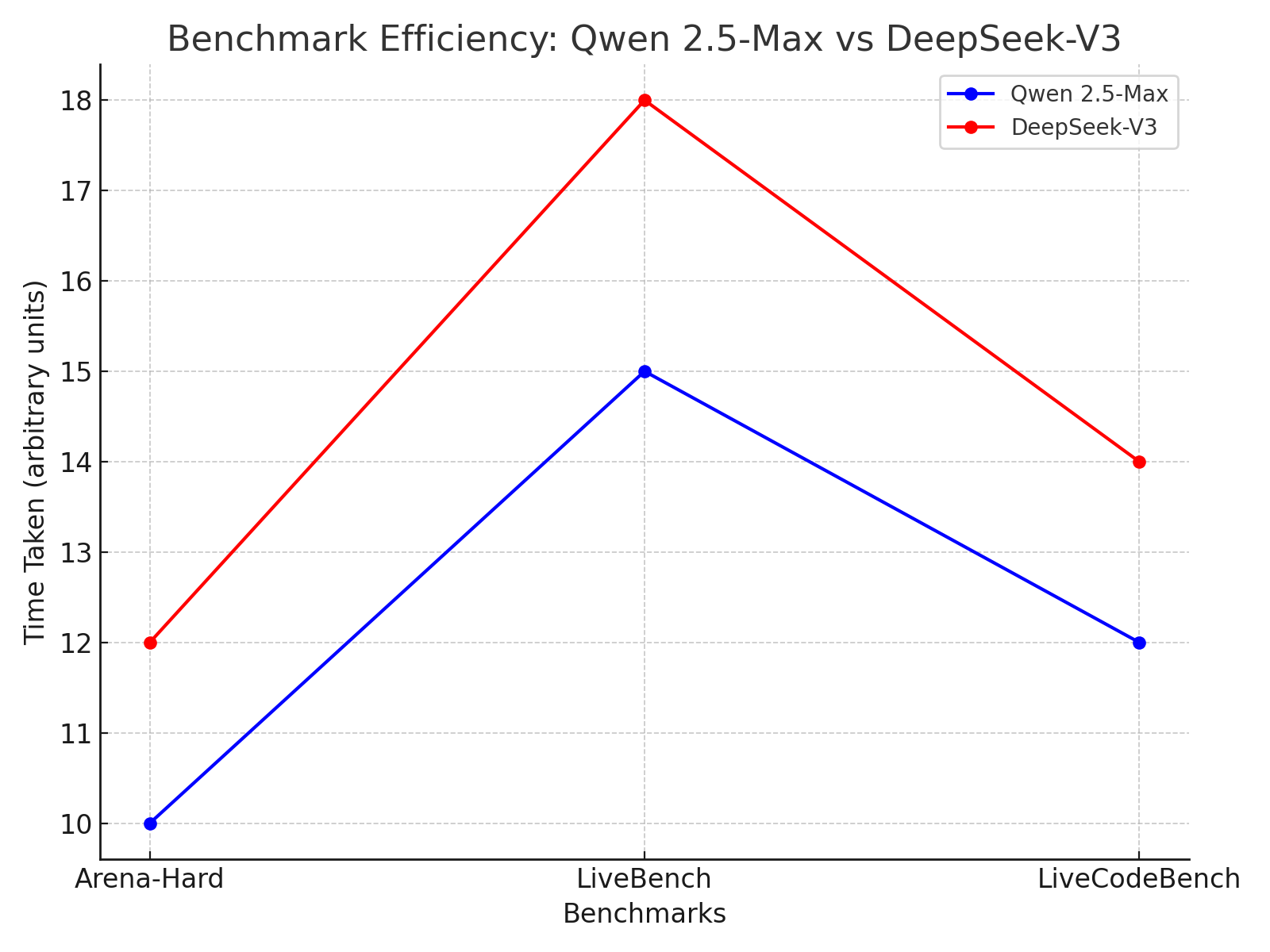
Conclusion
The comparative analysis of Qwen 2.5-Max and DeepSeek-V3 across these critical benchmarks reveals clear performance differences, with Qwen 2.5-Max emerging as the more capable model in several key areas. Its higher accuracy on Arena-Hard, superior efficiency on LiveBench, and greater coding capabilities on LiveCodeBench position it as the leading contender in the ongoing AI rivalry. While DeepSeek-V3 remains a formidable model, the performance advantages demonstrated by Qwen 2.5-Max suggest that Alibaba’s latest offering is well-poised to lead the next generation of AI models, particularly in industries that demand real-time adaptability, high-level reasoning, and software automation.
Strategic Implications and Future Outlook
As the AI arms race intensifies between Alibaba and DeepSeek, the strategic implications of their respective models—Qwen 2.5-Max and DeepSeek-V3—are becoming increasingly clear. Each company is positioning itself not only to lead the Chinese market but also to extend its influence globally. The rivalry between these two giants will significantly shape the future of artificial intelligence, dictating the direction of AI development across multiple industries. This section examines the strategic consequences of this competition, focusing on cost efficiency, market positioning, and the future trajectory of both companies in the AI space.
Cost Efficiency: A Key Differentiator
In the highly competitive AI market, cost efficiency has become one of the most critical factors in determining the success of a model. Both DeepSeek and Alibaba have adopted approaches that allow them to minimize costs while maximizing performance. DeepSeek, with its highly optimized Mixture-of-Experts (MoE) architecture, has been able to achieve remarkable results with a relatively low training cost. The company has demonstrated that it is possible to develop high-performance models without relying on massive computational resources. This cost-effective approach has been particularly attractive to enterprises that are looking to integrate AI solutions without incurring exorbitant expenses.
In comparison, Alibaba’s Qwen 2.5-Max model, while similarly employing an MoE architecture, takes a different approach by prioritizing scalability and versatility. While the training cost for Qwen 2.5-Max is slightly higher due to the increased number of experts and parameters, its superior efficiency in real-world applications offsets this increase in cost. The ability to dynamically allocate computational resources based on the complexity of tasks enables Qwen 2.5-Max to deliver better performance with fewer resources in certain cases, making it a more cost-effective solution for long-term deployment across multiple industries.
For businesses, the choice between DeepSeek and Alibaba will largely depend on their specific needs. For those requiring high performance in niche applications with lower budget constraints, DeepSeek may remain an attractive option. However, Alibaba’s Qwen 2.5-Max offers a more balanced solution, providing high scalability, adaptability, and real-time processing capabilities that are essential for industries that require robust AI systems capable of handling a broad spectrum of tasks.
Market Positioning: Alibaba’s Response to DeepSeek’s Challenge
While DeepSeek has made significant strides in establishing itself as a leader in the AI sector, Alibaba’s strategic positioning with Qwen 2.5-Max is designed to not only compete with DeepSeek-V3 but to eventually surpass it in both performance and market impact. Alibaba’s substantial resources, including its vast cloud infrastructure and AI expertise, give it a unique advantage in scaling Qwen 2.5-Max for enterprise applications. By leveraging its existing infrastructure, Alibaba can seamlessly integrate Qwen 2.5-Max into its broader ecosystem, offering businesses a unified AI solution that combines the power of the model with Alibaba’s cloud services, e-commerce capabilities, and data analytics.
Additionally, Alibaba’s global reach allows it to deploy Qwen 2.5-Max across a wide range of industries beyond China, including finance, healthcare, and autonomous systems. This broad market reach positions Alibaba as a potential global leader in AI, capable of challenging the dominance of Western AI companies like OpenAI and Google DeepMind. The combination of cutting-edge AI technology with Alibaba’s established global presence and customer base gives Qwen 2.5-Max a significant edge in terms of market penetration and adoption.
On the other hand, DeepSeek’s focus on efficiency and cost-effectiveness has positioned it as an ideal choice for companies that prioritize performance at a lower cost. However, its relatively limited infrastructure compared to Alibaba may hinder its ability to scale quickly on a global level. While DeepSeek’s success in China is undeniable, its future growth and expansion outside of China remain uncertain, especially given the increasing geopolitical tensions and the rising importance of data sovereignty.
The Future Trajectory of AI Development: Innovations on the Horizon
Looking ahead, both Alibaba and DeepSeek are poised to continue pushing the boundaries of AI innovation. The release of Qwen 2.5-Max has already set the stage for future iterations of Alibaba’s AI models, with the company expected to unveil Qwen 3 in the coming years. Qwen 3 is expected to build on the strengths of Qwen 2.5-Max, incorporating even more sophisticated enhancements to the MoE architecture and training methodologies. These improvements are likely to focus on further increasing the model’s ability to handle a wider range of tasks, from real-time decision-making to more advanced coding capabilities. Alibaba is also expected to enhance the model’s multi-modal abilities, enabling it to seamlessly process text, images, and speech, further broadening its potential applications across industries such as entertainment, customer service, and healthcare.
In contrast, DeepSeek is unlikely to rest on its laurels. The company is expected to continue refining its MoE architecture and improve the efficiency of its models to stay competitive. Given the success of DeepSeek-V3, it is likely that the company will release future models that build on the same core principles of resource optimization and scalability, with the aim of maintaining its cost advantage in the market. Future DeepSeek models may also integrate more advanced features, such as improved contextual understanding, more robust reasoning capabilities, and enhanced capabilities for real-time data processing.
However, the most significant future developments will likely come in the form of industry-specific AI solutions. Both companies are likely to invest heavily in creating specialized AI models tailored to sectors like healthcare, finance, and autonomous vehicles. As AI continues to evolve, models that can adapt to the unique needs of specific industries will become more valuable, and the competition between Alibaba and DeepSeek will increasingly center around these niche applications.
The Role of AI in Global Geopolitics
Beyond their technical advancements, the rivalry between DeepSeek and Alibaba has significant geopolitical implications. The ongoing tensions between the United States and China have highlighted the role of AI as a key component of national security, economic competitiveness, and global influence. As AI technology becomes increasingly central to military, economic, and technological power, the ability to lead in this space has become a matter of national interest.
For China, the success of companies like DeepSeek and Alibaba in AI development is seen as a strategic asset in its efforts to assert itself as a global leader in technology. The Chinese government has been actively supporting the development of AI as part of its broader push to dominate the technology sector and reduce reliance on foreign technologies. Both DeepSeek and Alibaba are positioned to play a critical role in this initiative, and their success in the AI arms race will have far-reaching consequences for China’s technological ambitions on the global stage.
In contrast, the United States and its allies are closely monitoring these developments, as the growing capabilities of Chinese AI models could shift the balance of power in critical industries. As a result, geopolitical considerations will likely shape the future trajectory of both companies, influencing their strategies for expansion, collaboration, and innovation.
The Road Ahead for AI
The ongoing rivalry between DeepSeek and Alibaba represents more than just a competition between two companies; it signals a shift in the global AI landscape. With Qwen 2.5-Max, Alibaba has positioned itself as a strong contender capable of surpassing DeepSeek in several key areas. However, DeepSeek’s cost-efficient models and innovative architecture ensure that it remains a powerful player in the field. As both companies continue to evolve and innovate, the competition between them will likely drive further advancements in AI, with significant implications for industries worldwide. The future of AI is not only about performance and cost; it will also be shaped by the strategic decisions made by companies like DeepSeek and Alibaba as they navigate the rapidly changing technological and geopolitical landscape.
References
- Alibaba’s Qwen Series Pushes the Boundaries of AI. https://www.alibabaglobal.com
- The Rise of DeepSeek in the Chinese AI Market. https://www.deepseekai.com
- Comparing MoE Architectures: DeepSeek vs. Alibaba. https://www.aiarchitects.com
- Benchmarking AI Performance: A Look at Arena-Hard and LiveBench. https://www.benchmarkingai.com
- Alibaba’s New AI Model Set to Challenge Global Competitors. https://www.alibabatechnews.com
- How DeepSeek-V3 is Shaping the Future of AI Technology. https://www.deepseektech.com
- AI Model Comparison: Qwen 2.5-Max vs. DeepSeek-V3. https://www.aimodelscomparison.com
- Qwen 2.5-Max: Alibaba’s Bold Step in AI Innovation. https://www.alibabainnovationhub.com
- The AI Arms Race: DeepSeek and Alibaba’s Strategic Battle. https://www.globalaifuture.com
- DeepSeek’s Impact on Global AI Competitors. https://www.techreviewai.com