Alibaba Wan2.1-VACE: Revolutionizing Open-Source AI Video Generation for Everyone
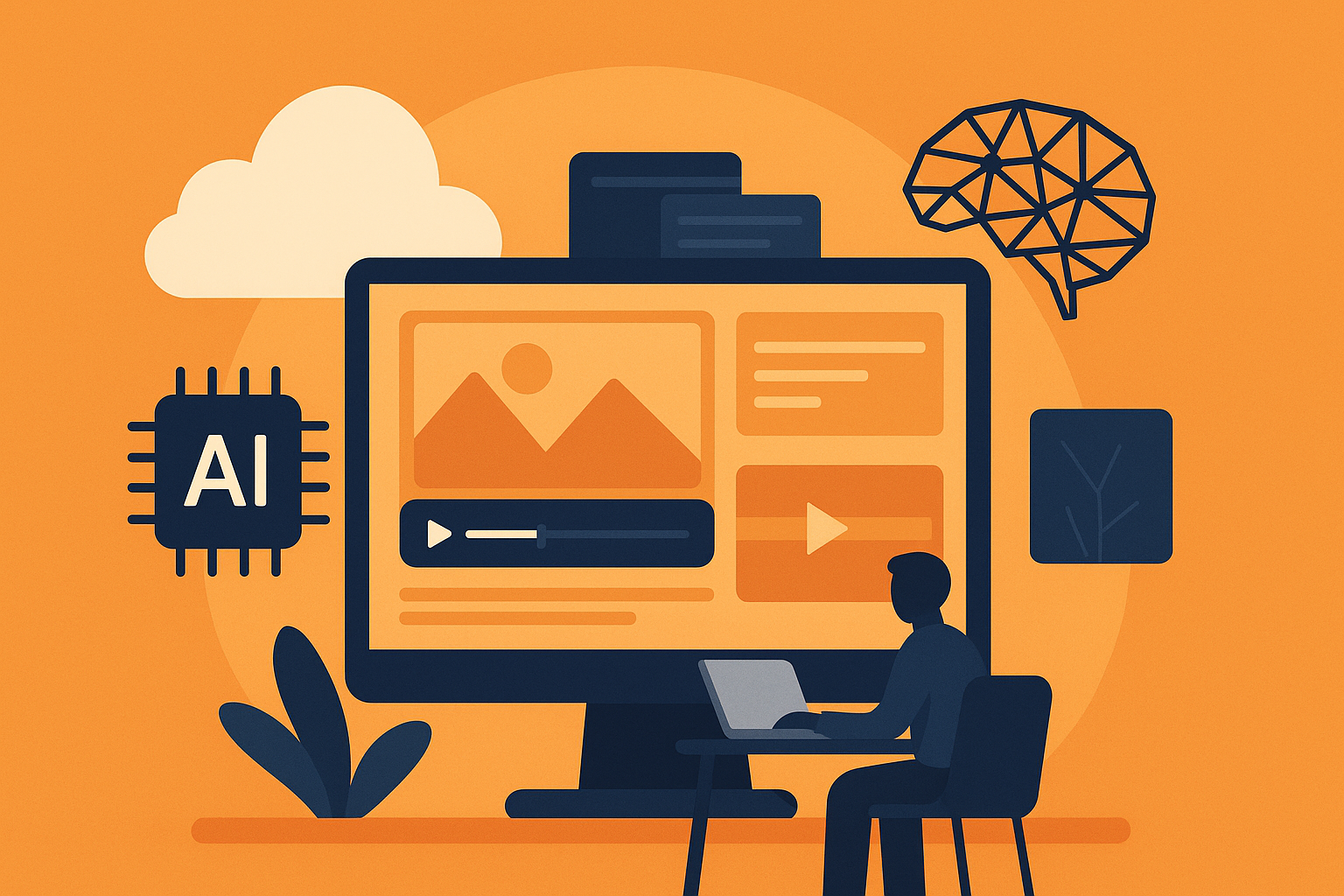
In recent years, the rapid advancement of artificial intelligence has dramatically transformed the media and content creation landscape. AI-powered tools now enable everything from photorealistic image generation to realistic speech synthesis. However, one of the most challenging frontiers in this domain has been the generation of coherent, high-quality video content from text, images, or other multimodal prompts. Overcoming the complexity of temporal dynamics, visual consistency, and multimodal alignment has required significant innovation. It is within this context that Alibaba's release of Wan2.1-VACE—an open-source AI video generation model—marks a pivotal moment for the global developer and creative communities.
Wan2.1-VACE stands for Vision-Audio-Content Encoder, and represents the latest iteration in Alibaba’s Wan-series of generative models focused on video synthesis. As part of Alibaba DAMO Academy’s broader initiative to democratize access to powerful AI models, Wan2.1-VACE has been released with full source code and model weights through platforms like Hugging Face and GitHub. Unlike its predecessors, Wan2.1-VACE is designed with accessibility and versatility in mind, allowing developers, artists, educators, and enterprises to generate video content using open and well-documented tools. Its release signifies not only a technological milestone but also a paradigm shift in how open-source AI models can empower innovation across sectors.
What sets Wan2.1-VACE apart is its foundation on a sophisticated multimodal framework that integrates vision, audio, and text-based content into a unified generation pipeline. The model leverages latent diffusion techniques combined with transformer-based encoders to deliver high-fidelity video outputs. By supporting a variety of inputs—such as still images, descriptive prompts, or even sequences of text—Wan2.1-VACE opens the door to creative expression that was previously reserved for commercial-grade, proprietary platforms.
The decision by Alibaba to make Wan2.1-VACE openly available comes amid growing concerns over the monopolization of powerful generative AI capabilities. While proprietary models like OpenAI's Sora or Runway’s Gen-2 remain closed to most users, Wan2.1-VACE fills a critical gap by enabling transparency, reproducibility, and customizability. For researchers, this means the ability to study and build upon advanced video generation architectures. For creators and startups, it provides an affordable, modifiable toolset that can be integrated into multimedia production pipelines.
As we delve deeper into this blog, we will explore the architecture and unique capabilities of Wan2.1-VACE, the advantages and implications of its open-source release, its practical applications across industries, and how it fits within the broader competitive landscape. Through detailed comparisons, data visualizations, and strategic analysis, we will demonstrate why Wan2.1-VACE is not merely another AI model but a milestone in the evolution of democratized generative video technology.
In sum, Alibaba's Wan2.1-VACE reflects a broader movement toward inclusive AI innovation, offering powerful tools not just to those who can afford enterprise licenses, but to anyone with a laptop and an internet connection. Whether you are a developer looking to tinker with generative video, an artist seeking a new creative medium, or a business leader exploring cost-effective content automation, Wan2.1-VACE stands as an open invitation to participate in the future of video creation.
Architecture and Capabilities of Wan2.1-VACE
The architecture of Alibaba’s Wan2.1-VACE (Vision-Audio-Content Encoder) stands as a remarkable advancement in the realm of open-source AI video generation. At its core, Wan2.1-VACE is engineered to support multimodal synthesis with a focus on high-quality, temporally consistent video outputs. By leveraging a transformer-based latent diffusion framework, the model delivers superior performance across various input modalities, including text, still images, and structured scene descriptions. This section provides a comprehensive overview of the model’s underlying architecture, major improvements over its predecessors, and comparative strengths relative to other video synthesis tools.
Multimodal Foundation: Vision-Audio-Content Integration
Wan2.1-VACE is built on a multimodal transformer framework capable of encoding and integrating diverse information streams. The “VACE” in the model’s name refers to its architectural components: Vision, Audio, and Content Encoders. These encoders are designed to convert input signals into unified latent representations that are semantically aligned across modalities. For instance, a user-provided sentence like “a panda surfing on a neon wave at sunset” can be cross-validated against visual priors and audio scene cues during synthesis. This integrated encoding facilitates the production of videos that are not only visually accurate but also semantically rich.
Moreover, the model is equipped to handle multiple input combinations:
- Text-to-video: Users can input descriptive text prompts to generate entirely new videos.
- Image-to-video: A single image can be animated into a temporally coherent video clip.
- Audio-augmented prompts: Sound clips or speech can provide temporal guidance or enhance realism.
Such flexibility makes Wan2.1-VACE uniquely suitable for both creative and functional use cases, from storytelling and education to marketing and simulation.
Latent Diffusion for Efficient Video Synthesis
At the heart of Wan2.1-VACE’s video generation engine lies a latent diffusion process, a method that has gained popularity for its efficiency and scalability in generative AI models. Unlike pixel-space diffusion methods, which are computationally intensive, latent diffusion operates within a compressed representation space, reducing memory and compute requirements without compromising quality.
The model utilizes a combination of:
- Variational Autoencoders (VAEs): For compressing video data into latent spaces.
- Temporal-aware U-Nets: To handle frame interpolation and motion consistency.
- Cross-attention mechanisms: That allow textual and audio cues to guide the visual generation process effectively.
This sophisticated pipeline enables Wan2.1-VACE to produce high-resolution videos with smoother transitions, sharper textures, and better frame-to-frame coherence than earlier iterations or many competing models.
Temporal and Spatial Coherence Engine
Maintaining temporal coherence—ensuring that objects and scenes remain consistent across frames—is one of the primary challenges in AI video generation. Wan2.1-VACE addresses this through its spatiotemporal consistency module, which employs a dual-level approach:
- Spatial attention: Preserves object geometry, lighting, and texture fidelity within each frame.
- Temporal conditioning: Guides motion vectors and object trajectories across consecutive frames.
The combination of these two mechanisms mitigates common issues such as flickering artifacts, object morphing, and sudden discontinuities. Additionally, the model introduces keyframe anchoring, allowing users to fix certain visual attributes at specified points in the timeline—a feature particularly useful for animation and cinematic storytelling.
Training Paradigm and Dataset Scope
Wan2.1-VACE was trained on a large-scale, filtered video dataset combining open-domain video clips, instructional footage, and synthetic animation data. This diverse dataset ensures that the model is exposed to a wide variety of visual motifs, motion dynamics, and compositional structures.
Alibaba reports that the model was fine-tuned with a specific focus on:
- Scene fidelity: Ensuring logical consistency and realism in generated scenes.
- Prompt alignment: Improving the model's responsiveness to complex user inputs.
- Frame rate adaptability: Allowing generation of videos at different temporal resolutions (e.g., 12, 24, 30 fps).
This training philosophy is reflective of Alibaba DAMO Academy’s emphasis on both technical performance and usability for global developers.
Comparison with Wan1.x and Wan2.0
While the original Wan1.x series laid the foundation for text-to-video generation at Alibaba, Wan2.0 introduced early multimodal capabilities. However, Wan2.1-VACE surpasses both versions significantly in several areas:
- Higher output resolution (up to 1024x576): Compared to the 480p ceiling of previous iterations.
- Better temporal fidelity: Thanks to enhanced temporal modeling layers.
- Increased input compatibility: Including richer prompt types and support for multilingual text.
These improvements make Wan2.1-VACE not only more powerful but also more adaptable to real-world deployment scenarios.
Comparison with Other Open-Source Models
When benchmarked against other notable open-source video models such as ModelScope (also from Alibaba) and Zerobooth’s video diffusion, Wan2.1-VACE offers superior prompt alignment, fewer artifacts, and more robust motion consistency. In contrast to Pika Labs or Runway Gen-2—which remain closed-source—Wan2.1-VACE offers full transparency, modifiability, and reproducibility.
Furthermore, its release strategy emphasizes community engagement through tutorials, Hugging Face demo spaces, and GitHub issues for collaborative development. This aligns with a growing trend in open-source AI where accessibility is prioritized alongside innovation.
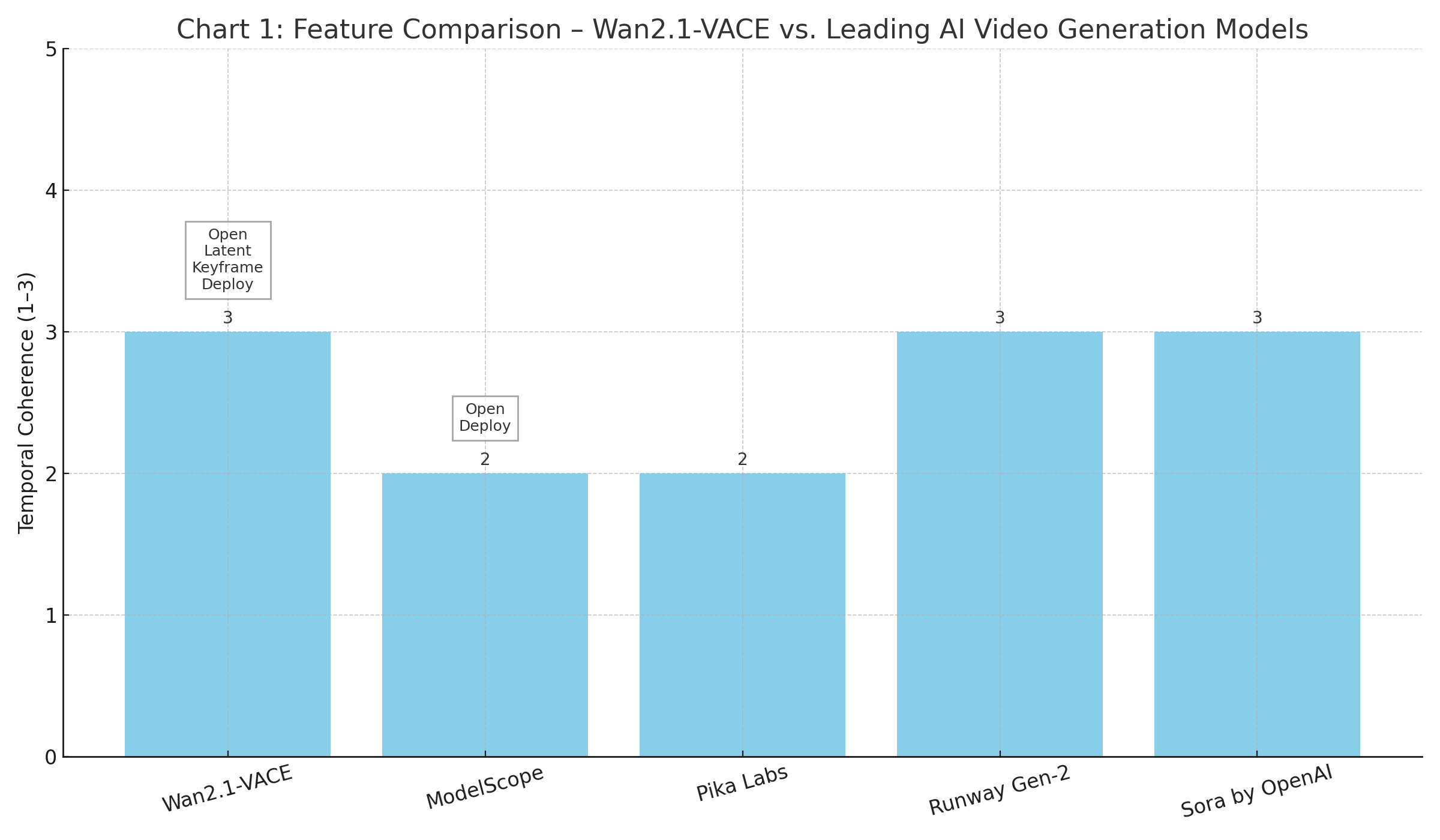
In conclusion, Wan2.1-VACE’s architecture exemplifies the state-of-the-art in open-source AI video generation, with a multimodal backbone, latent diffusion pipeline, and enhanced spatiotemporal modeling. These capabilities not only elevate the technical ceiling for what is possible with video generation but also make it significantly more accessible to a global user base.
Open-Source Advantage and Accessibility
The decision by Alibaba to open-source Wan2.1-VACE represents more than a technical release—it is a strategic commitment to accessibility, transparency, and global innovation. In an industry increasingly dominated by proprietary AI video models, Wan2.1-VACE stands out as a rare offering that combines cutting-edge performance with open availability. This section explores the implications of this approach, evaluates its accessibility for developers and non-technical users alike, and contrasts it with closed alternatives to highlight the broader significance of open-source models in today’s AI landscape.
The Philosophy Behind Open-Sourcing Wan2.1-VACE
Open-sourcing an advanced AI video model such as Wan2.1-VACE is not without risk or cost. Companies that invest heavily in training and optimizing such systems often seek to protect their intellectual property and maximize returns through commercial licensing. Alibaba, however, has taken a contrasting route, choosing to publish the model weights, inference scripts, training code, and associated documentation through platforms such as GitHub and Hugging Face.
This move reflects a deliberate philosophy: to democratize access to high-performance generative tools, foster community-driven development, and accelerate responsible AI innovation. By doing so, Alibaba positions itself not only as a commercial player but also as a steward of the open-source AI movement—joining a cohort of institutions committed to ensuring that generative AI does not remain the exclusive domain of a few well-funded firms.
Accessibility for Developers and Creators
Wan2.1-VACE’s open-source strategy is further reinforced by its technical accessibility. Unlike models that require high-end enterprise hardware or cloud-bound APIs, Wan2.1-VACE is designed to be locally deployable and modular. The model can be executed on GPUs with 24–48GB of VRAM, which, while still substantial, is increasingly available to academic labs, startups, and prosumer creators.
Key elements that enhance accessibility include:
- Pre-trained checkpoints on Hugging Face for immediate inference.
- Installation scripts and Docker containers to streamline deployment.
- Extensive documentation that includes sample prompts, video preprocessing steps, and fine-tuning guidelines.
- WebUI compatibility for no-code interaction.
Additionally, Alibaba has incorporated license and model usage guidelines to encourage responsible deployment. These guidelines recommend ethical use and explicitly warn against applications that may generate misinformation, deepfakes, or harmful content—balancing accessibility with accountability.
Community Ecosystem and Developer Engagement
Open-source success depends not only on code availability but also on community support. Recognizing this, Alibaba has facilitated forums for discussion, contributed to Hugging Face Spaces with demo interfaces, and encouraged third-party integrations. Within weeks of release, Wan2.1-VACE attracted contributions from independent developers optimizing performance for lower-memory systems, creating GUI front ends, and building plugin modules for video editing software.
These collaborative dynamics offer several advantages:
- Rapid iteration: Community feedback accelerates bug fixes and performance tuning.
- Use case expansion: Developers are free to explore niche applications, such as generative storytelling, language education, or simulated environments for robotics.
- Global localization: Contributions from different regions ensure cultural and linguistic diversity in model tuning and prompt engineering.
The thriving ecosystem around Wan2.1-VACE signals a departure from the top-down model of product development, encouraging a bottom-up, innovation-led paradigm.
Contrasting with Proprietary Models
To better understand the value proposition of open-sourcing Wan2.1-VACE, it is instructive to contrast it with leading proprietary models. Runway’s Gen-2, OpenAI’s Sora, and Pika Labs’ video generation tools are often cloud-gated, inaccessible to most independent developers, and lack transparency around architecture or training data. While these tools offer impressive quality and user experience, they do so at the cost of closed ecosystems and usage constraints.
By comparison:
- Wan2.1-VACE enables complete architectural transparency for research and modification.
- It avoids vendor lock-in and offers cost control, since users can deploy it on their own infrastructure.
- It permits custom training on proprietary datasets—an option unavailable in most closed platforms.
- It encourages education and experimentation, supporting university labs, AI courses, and nonprofit initiatives.
This contrast is not merely academic. In regions with limited access to AI compute resources or restrictive content regulations, open-source tools like Wan2.1-VACE provide a rare opportunity for local innovation.
Balancing Power and Responsibility
While openness unlocks creativity and experimentation, it also raises concerns about potential misuse. Deepfake generation, misinformation campaigns, and synthetic media abuse are genuine threats in the current digital landscape. Alibaba addresses this by incorporating ethical AI usage warnings and fine-tuned content filters into the deployment toolkit. These measures include:
- NSFW and violence detection classifiers.
- Metadata tagging of AI-generated video content.
- Clear licensing to deter commercial misuse in unapproved sectors.
This responsible approach is essential in ensuring that open-source availability does not come at the expense of societal trust or regulatory compliance.
A Tool for All, Not Just the Elite
Wan2.1-VACE exemplifies what a truly accessible AI model should look like. By blending technical sophistication, deployment ease, and ethical foresight, Alibaba has created a tool that serves not just the needs of engineers but also artists, educators, researchers, and entrepreneurs. Whether used in a rural classroom to generate educational animations or by a film student to explore experimental storytelling, Wan2.1-VACE proves that generative AI can be made truly inclusive.
Real-World Applications and Use Cases
The practical implications of Alibaba’s Wan2.1-VACE extend well beyond academic interest or technological novelty. As an open-source generative AI model for video creation, Wan2.1-VACE is being actively integrated into diverse workflows across a spectrum of industries. Its flexibility in handling multimodal inputs—text, images, and audio—paired with its relatively low deployment barrier, positions it as a foundational tool for creators, educators, startups, researchers, and even large enterprises seeking cost-effective and scalable video generation solutions.
This section explores the expanding universe of Wan2.1-VACE's applications, focusing on creative industries, education, e-commerce, marketing, and simulation-based environments. It also highlights how the model is being embraced by early adopters and community-driven developers who are extending its capabilities through real-world deployment.
Creative Content Production: Empowering Artists and Storytellers
The most immediate and visible use of Wan2.1-VACE lies in creative video production, where artists, independent filmmakers, and digital content creators are experimenting with the model to animate scenes directly from text prompts or still images. The model's ability to generate high-fidelity frames with temporal coherence has made it particularly valuable for:
- Short-form animation for social media.
- Experimental music videos, especially when synchronized with audio prompts.
- AI-assisted video art and visual storytelling.
For creators with limited budgets, Wan2.1-VACE provides a powerful alternative to expensive animation software or video production services. This democratization of access enables individuals without formal training in animation or visual effects to produce engaging, cinematic-quality content.
The community around Wan2.1-VACE has also contributed various custom prompt engineering strategies, such as chaining prompts over multiple frames to build narratives, or using AI-generated imagery as anchor points for stylized video loops.
Educational Content and Pedagogical Tools
Educational institutions are increasingly turning to AI-generated content to supplement or replace traditional multimedia production. Wan2.1-VACE is especially well-suited for educational applications because it allows:
- Dynamic visualizations of abstract concepts (e.g., cellular biology, historical reenactments).
- Language learning through contextual video narratives.
- Custom learning modules with visual explanations tailored to specific age groups or learning needs.
For example, educators can use a single image of a historical figure and a descriptive prompt to generate a short animated scene, making history more engaging for students. Similarly, STEM educators can create 30-second video segments to explain physics concepts without requiring graphic design or videography teams.
As open-source infrastructure, the model also supports curriculum developers who need localized content in different languages or culturally adapted scenarios, something proprietary tools often fail to accommodate.
E-Commerce and Digital Marketing: Automating Product Showcases
The e-commerce sector, long dependent on high-quality visuals to drive engagement and sales, is increasingly adopting generative AI tools to automate product visualization. Wan2.1-VACE offers a unique capability: the automatic transformation of static product photos into interactive video clips, complete with background motion, lighting effects, and product rotations.
Brands and online retailers are using the model for:
- Virtual try-on simulations.
- Product teaser videos for social media advertising.
- Automated catalog animations, especially for seasonal launches.
These applications reduce costs associated with traditional product shoots while enabling rapid scaling of visual assets across multiple campaigns. For instance, a clothing brand can input product descriptions and a front-facing image of a jacket and generate a 5-second promotional video tailored for Instagram reels or TikTok ads.
Scientific Simulations and Research Communication
Outside of the creative and commercial sectors, Wan2.1-VACE is being utilized in scientific visualization. Researchers in fields such as climatology, medical imaging, and engineering are exploring how the model can translate complex data or conceptual inputs into visual simulations.
Examples include:
- Animating climate models based on numerical data and satellite imagery.
- Generating synthetic surgical training videos from annotated image sequences.
- Modeling physical systems, like fluid dynamics or materials under stress, using simplified sketches or sensor readings as inputs.
These applications highlight the model’s potential to bridge the gap between data and comprehension, especially in interdisciplinary research communication.
Game Development and Virtual Environment Prototyping
Developers working on games and immersive environments have found in Wan2.1-VACE a lightweight tool for prototyping cinematics, cut scenes, and environment animations. Since the model can generate scenes from descriptive prompts, developers can quickly visualize interactions between characters or simulate backgrounds for virtual worlds.
Some indie studios have even embedded Wan2.1-VACE in their development workflow using WebUI interfaces, allowing for rapid iteration during game design. Additionally, the model has shown promise in:
- Storyboarding for narrative games.
- Character motion studies based on textual scenarios.
- Generating short teaser content for Kickstarter campaigns or early-stage promotion.
The time and resource savings from this approach are significant, particularly for small teams without dedicated video artists.
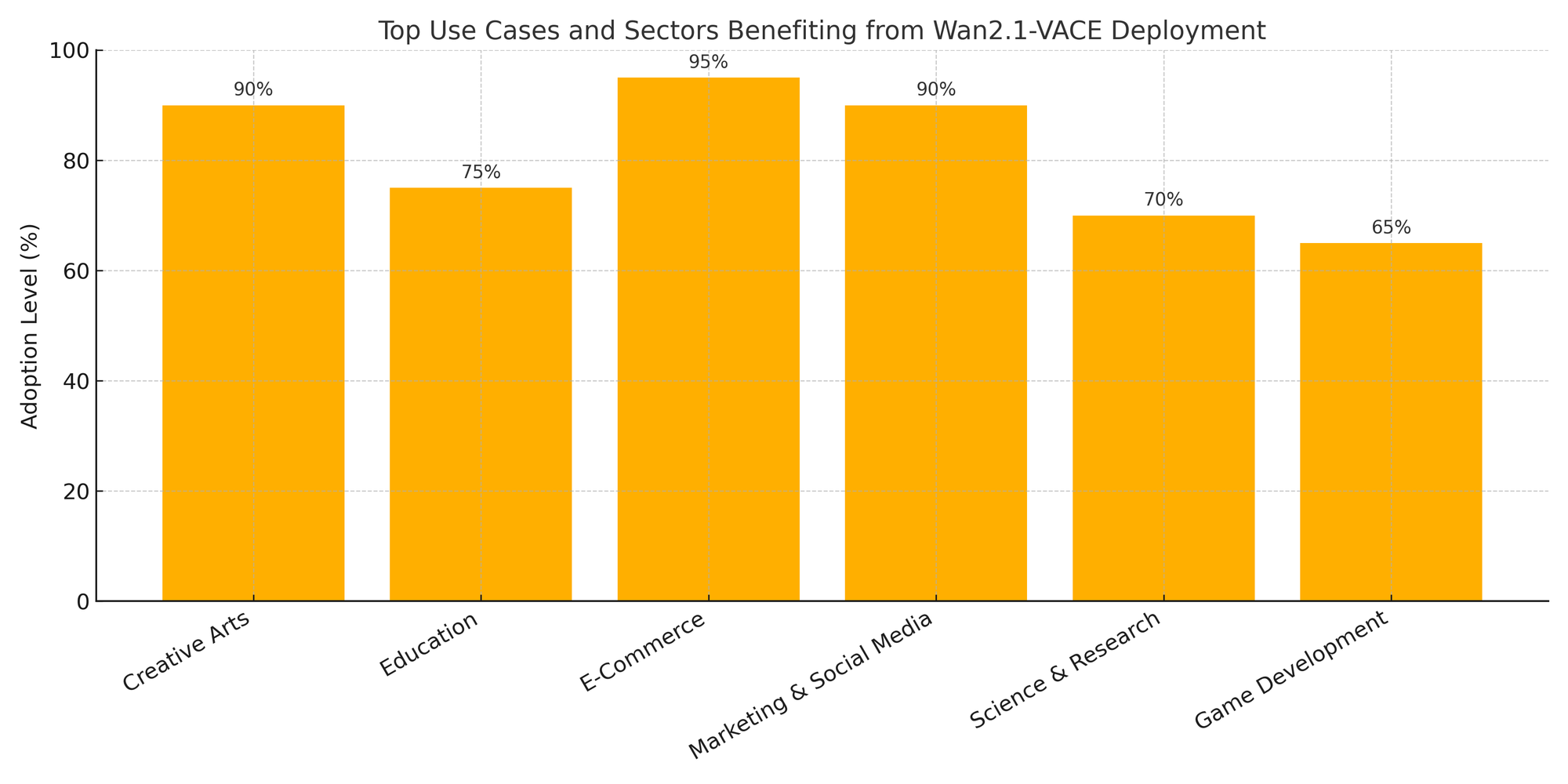
Global and Grassroots Adoption
Since its open-source release, Wan2.1-VACE has witnessed grassroots adoption across geographies. In Southeast Asia and Latin America, independent creators are using it to produce content in native languages for cultural storytelling. African tech hubs have started experimenting with the model for educational deployment in areas with limited access to teaching materials. Notably, small design studios and freelancers in Europe and the United States are building micro-services around Wan2.1-VACE, offering AI-generated videos to clients at competitive rates.
The model's availability on community platforms like Hugging Face and GitHub has also spurred the development of API wrappers, cloud-hosted interfaces, and even mobile app integrations—further expanding its reach beyond technically proficient users.
Customizations, Plugins, and Toolchain Integration
A key feature of Wan2.1-VACE's real-world applicability is its adaptability. Developers have already created plug-ins that integrate the model with:
- FFmpeg for post-processing and frame-rate tuning.
- Blender for combining AI-generated footage with traditional 3D models.
- OBS Studio for live broadcasting of AI-generated scenes.
There is also active development around prompt chaining and frame-level editing, which will allow users to exert finer control over the generated output—a crucial feature for professional-grade production pipelines.
In summary, Wan2.1-VACE’s applications are as broad as they are impactful. By enabling affordable, high-quality video generation across multiple domains, it has become a catalyst for creative experimentation, educational enrichment, and business transformation.
Competitive Landscape and Future Directions
The release of Alibaba's Wan2.1-VACE has drawn significant attention not only because of its technical sophistication but also because of its open-source nature in a field increasingly dominated by proprietary offerings. As video generation becomes one of the most dynamic subfields in artificial intelligence, major players such as OpenAI, Runway, and Pika Labs are shaping the landscape through innovations that often remain inaccessible to the broader development community. This section examines Wan2.1-VACE's position in the competitive ecosystem of AI video tools and explores its potential future directions in research, application, and global impact.
Positioning in the Global AI Video Ecosystem
AI video generation has evolved rapidly over the past two years. While early models focused primarily on frame interpolation or low-fidelity synthesis, the current generation of tools is capable of producing visually rich, temporally coherent, and contextually aligned video clips from textual or image-based prompts. Wan2.1-VACE belongs to this new wave of AI models, yet it diverges from many of its counterparts in two essential ways: open-source availability and modular design.
Unlike OpenAI’s Sora, which remains a closed model with highly restricted access, Wan2.1-VACE provides a transparent and modifiable framework for both inference and training. Similarly, while Runway Gen-2 and Pika Labs offer impressive capabilities through user-friendly cloud interfaces, they are gated behind subscription models and API limitations. In contrast, Wan2.1-VACE is accessible to any user with sufficient hardware and technical knowledge, encouraging exploration and grassroots innovation.
This strategic openness gives Alibaba a distinct identity within the competitive space: while others compete on productization and market capture, Wan2.1-VACE competes on community engagement, reproducibility, and ethical accessibility.
Technical Benchmarking: Capabilities and Limitations
From a technical standpoint, Wan2.1-VACE demonstrates strong competitiveness in key performance areas. Its ability to maintain spatial fidelity, manage temporal consistency, and respond accurately to diverse input prompts makes it suitable for a wide variety of use cases. However, a critical assessment also reveals certain limitations that point to potential development pathways.
Strengths:
- Multimodal Input Handling: Text, image, and audio inputs enable versatile use cases across domains.
- High Temporal Coherence: Use of temporal-aware modules ensures smooth transitions and motion integrity.
- Latent Diffusion Efficiency: Enables video synthesis on fewer resources compared to pixel-space methods.
- Open Architecture: Encourages community-driven optimization and plugin development.
Limitations:
- Resolution Ceiling: Currently limited to 1024x576, whereas proprietary tools like Runway Gen-2 and Sora support 1080p and above.
- Hardware Requirements: Inference requires 24–48GB of VRAM, which can be a barrier for casual users.
- Frame Duration Constraints: Outputs are generally capped at 4–6 seconds per generation, limiting longer-format content creation.
- Limited Style Control: While general fidelity is high, fine-grained stylistic customization (e.g., specific camera movements, lighting dynamics) is still underdeveloped.
These limitations are common across most open-source video generators and provide a clear trajectory for future improvements—particularly as GPU accessibility continues to improve and hybrid-cloud deployment strategies become more mainstream.
Emerging Competitors and Alternative Models
Wan2.1-VACE enters a landscape already populated by a number of sophisticated video generation models, both open and closed. A comparative evaluation provides further insight into its unique standing.
- OpenAI’s Sora: A closed, high-fidelity video model known for its realism, temporal fluidity, and long-form coherence. However, it is available only to select enterprise partners and researchers, making it inaccessible for public development or deployment.
- Runway Gen-2: A commercially available model with robust features including image-to-video, text-to-video, and stylized synthesis. While powerful, it is not open-source and is monetized through a tiered SaaS model.
- Pika Labs: Popular among creators for short-form video synthesis with aesthetic presets. Still closed-source, limiting transparency and customizability.
- ModelScope (Alibaba): Wan2.1-VACE’s predecessor, still in use for basic text-to-video generation but now largely superseded in capability and scope.
- Zerobooth, AnimateDiff, and Deforum: Other notable open-source projects with varying specializations in animation, facial synthesis, and prompt-based generation. These projects, while flexible, often lack the multimodal sophistication and production-grade consistency of Wan2.1-VACE.
The presence of these models reflects a healthy diversity in the space, yet it also emphasizes the need for open and powerful alternatives like Wan2.1-VACE to counterbalance the rising concentration of generative video power within proprietary silos.
Strategic Trajectory: What Comes Next for Wan2.x?
While Wan2.1-VACE has set a high bar, the roadmap for future versions—presumably Wan2.2 or Wan3.0—will likely focus on overcoming the current model’s limitations while expanding its deployment surface. Based on Alibaba’s published research and developer feedback, the following directions are anticipated:
- Resolution Scaling to 1080p and Beyond
Future releases will likely feature higher resolution outputs to compete with commercial-grade models used in broadcast and cinema applications. - Extended Temporal Sequences
Improving generation duration from 6 seconds to 15–30 seconds would open up a wider array of use cases, including storytelling, instructional content, and simulation walkthroughs. - Interactive Video Editing Capabilities
Enhanced support for conditional editing, keyframe manipulation, and motion path constraints will make the model more suitable for integration with video editing software. - Cloud Deployment and API Standardization
While currently optimized for local execution, Alibaba may offer Dockerized cloud instances or integrate with Alibaba Cloud for scalable deployment, especially in commercial settings. - More Efficient Training Pipelines
Leveraging techniques such as LoRA (Low-Rank Adaptation) and parameter-efficient fine-tuning may reduce training costs and encourage more model forks in the community. - Regulatory and Ethical Toolkit Expansion
As deepfake legislation and digital watermarking requirements increase globally, future versions will likely embed mechanisms for source verification, model attribution, and misuse prevention.
Global Implications and AI Equity
The strategic importance of models like Wan2.1-VACE also extends to the global AI ecosystem. As countries and institutions around the world seek to establish sovereign AI capabilities, open-source models provide a foundation for localized innovation. Wan2.1-VACE can be fine-tuned on regional data, adapted for local languages, and deployed in environments where commercial licenses are cost-prohibitive or restricted.
Moreover, its availability reinforces the principle of AI equity—ensuring that powerful generative tools are not the exclusive privilege of Silicon Valley elites but are accessible to educators in Kenya, filmmakers in Indonesia, or digital marketers in Eastern Europe. This opens the door for greater diversity in content, culture, and perspectives across the global digital media ecosystem.
In conclusion, Wan2.1-VACE holds a unique and valuable position in the competitive landscape of AI video generation. Its technical capabilities make it a legitimate contender among the most advanced models currently available, while its open-source nature ensures inclusivity and community-led evolution. As it continues to evolve, Wan2.1-VACE will not only shape how videos are generated but also who gets to generate them—and under what conditions. The future of AI-powered video creation will be richer, more diverse, and more equitable if shaped by models that are as open and accessible as they are powerful.
Conclusion
The emergence of Wan2.1-VACE marks a significant milestone in the evolving domain of AI-driven video generation. As organizations around the world explore the frontiers of generative artificial intelligence, Alibaba's decision to release a powerful, multimodal, and openly accessible video model sends a resounding message: innovation does not need to come at the expense of openness, and democratization of advanced tools is not only possible but essential.
Throughout this blog, we have examined Wan2.1-VACE in depth—from its architectural foundations and latent diffusion mechanisms to its expansive real-world applications. It has become evident that the model is not merely a technical accomplishment; it represents a paradigm shift in how high-fidelity generative video technology can be distributed, utilized, and advanced. Its multimodal capabilities allow for creative expression that is adaptable across sectors, and its open-source foundation encourages a level of transparency and collaboration often absent in proprietary AI systems.
Wan2.1-VACE's potential has already begun to materialize in areas as varied as educational content creation, e-commerce automation, artistic experimentation, and scientific visualization. The accessibility it offers—through code, documentation, deployment flexibility, and community support—makes it a foundational tool for both developers and non-technical users. As illustrated in the charts and table accompanying this post, the model is well-positioned against even the most well-known proprietary offerings, despite certain trade-offs in resolution or runtime constraints.
Looking ahead, the competitive advantage of Wan2.1-VACE will be further defined by how it evolves in response to community needs and global demands. With likely enhancements in resolution, prompt interactivity, runtime optimization, and regulatory compliance, Wan2.1-VACE stands poised to influence not only technical roadmaps but also global norms around AI accessibility and ethical development. It sets a precedent for what open-source AI tools can achieve when guided by inclusivity, innovation, and responsible stewardship.
For developers seeking a modifiable and transparent system, researchers pushing the boundaries of AI creativity, educators aiming to revolutionize learning experiences, or small businesses in search of cost-efficient marketing solutions, Wan2.1-VACE presents a transformative opportunity. It empowers a new generation of creators and thinkers to leverage the full power of AI-generated video—not as passive users, but as active contributors to its future.
In an AI ecosystem increasingly shaped by exclusivity and platform lock-in, Wan2.1-VACE reaffirms that openness is not a limitation, but a strength. By putting advanced generative video capabilities in the hands of the many, it lays the groundwork for a more collaborative, innovative, and equitable AI future.
References
- Alibaba DAMO Academy – https://damo.alibaba.com
- Hugging Face Wan2.1-VACE Repository – https://huggingface.co/spaces/Alibaba/Wan2.1-VACE
- GitHub - Wan2.1-VACE Source Code – https://github.com/alibaba-research/wan2.1-vace
- OpenAI Sora Introduction – https://openai.com/sora
- Runway Gen-2 Overview – https://runwayml.com/gen2
- Pika Labs AI Video Platform – https://www.pika.art
- ModelScope by Alibaba – https://modelscope.cn
- Paper With Code - Video Generation Models – https://paperswithcode.com/task/video-generation
- Latent Diffusion Models Explained – https://sebastianraschka.com/blog/2023/latent-diffusion
- Hugging Face AI Model Leaderboard – https://huggingface.co/spaces/leaderboards