AI Handwriting Analysis: Transforming Early Detection of Dyslexia and Dysgraphia
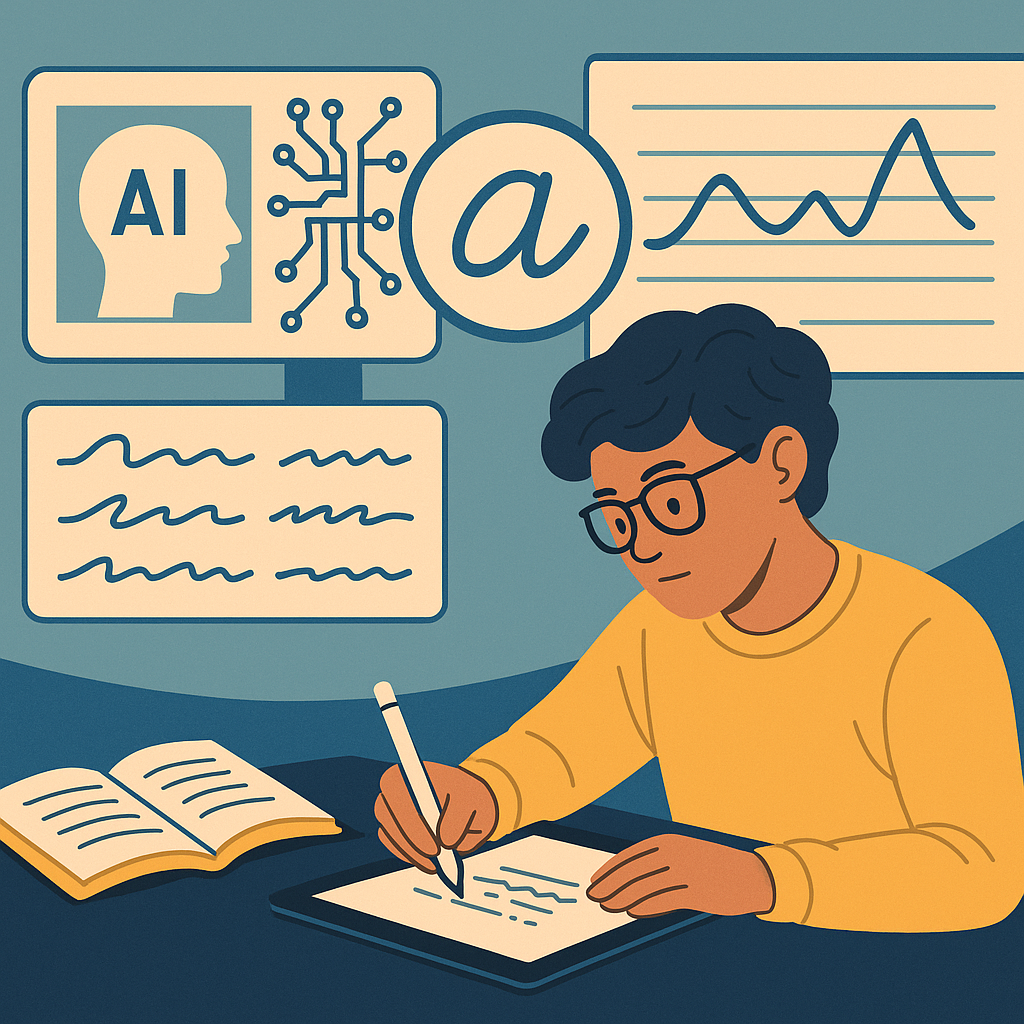
In an era marked by significant technological advancements in artificial intelligence (AI), healthcare and education stand out as domains that increasingly benefit from intelligent systems capable of early detection and intervention. Among the most promising innovations at the intersection of these two fields is AI-based handwriting analysis, which is rapidly emerging as a valuable tool for identifying neurodevelopmental learning disorders—specifically dyslexia and dysgraphia—at earlier stages than ever before.
Dyslexia and dysgraphia are commonly diagnosed in childhood but often go unnoticed until learning difficulties become pronounced. Dyslexia primarily affects a person's ability to read, while dysgraphia impacts writing abilities, including fine motor skills, letter formation, and coherence of written expression. Both conditions can significantly hinder academic progress and self-esteem if not addressed promptly and appropriately. Traditional methods for diagnosing these disorders involve extensive testing by educational psychologists and occupational therapists, typically after a student shows signs of struggle. These approaches, while valuable, are often time-consuming, subjective, and limited in scalability.
Recent breakthroughs in machine learning, computer vision, and natural language processing have created opportunities for more nuanced and data-driven approaches to screening for learning disorders. AI handwriting analysis utilizes sophisticated algorithms to evaluate a child's handwriting samples, capturing minute details in letter formation, pressure dynamics, spacing, slant, and movement trajectories. These parameters, often imperceptible to the human eye, can signal atypical neurological processing patterns associated with dyslexia and dysgraphia. More importantly, AI systems can perform these evaluations consistently, rapidly, and at scale, making them ideal for early screening programs in schools and clinics.
The technology behind AI handwriting analysis is multifaceted. At its core, it combines image recognition techniques with behavioral modeling to infer cognitive and motor anomalies. For example, a student struggling with dysgraphia may display inconsistent letter sizes, irregular spacing, or slow writing speed—features that an AI model can detect and quantify over time. Similarly, children with dyslexia might exhibit reversed letters, non-linear writing paths, or spelling errors stemming from phonological processing deficits, which can also be flagged through handwriting analytics.
What sets AI apart in this context is not merely its capacity to replicate human observation but its ability to surpass it. AI models trained on large and diverse datasets can identify subtle, composite indicators of learning disorders long before a child’s academic performance declines enough to raise concern. This early detection opens the door to timely interventions such as occupational therapy, specialized tutoring, or classroom accommodations—each of which can significantly improve long-term educational outcomes and emotional well-being.
Moreover, AI-based handwriting tools can be deployed via tablets, digital pens, and even scanned worksheets, making the technology accessible and integrable into existing classroom workflows. As such, educators and parents no longer need to wait for visible academic difficulties before seeking expert advice. Instead, AI can serve as a proactive measure, offering insights based on objective data and empowering stakeholders to take early and informed action.
This blog delves into the full scope of how AI handwriting analysis is revolutionizing early detection of dyslexia and dysgraphia. In the sections that follow, we will explore the scientific understanding of these learning disorders, the technical underpinnings of AI-based handwriting assessment, real-world case studies demonstrating the efficacy of such systems, and the broader implications for education, healthcare, and public policy. Through this lens, we aim to underscore the potential of AI not only as a diagnostic enhancer but also as a tool for educational equity and inclusion.
Understanding Dyslexia, Dysgraphia, and Their Early Indicators
Dyslexia and dysgraphia are two distinct, yet often co-occurring, neurodevelopmental learning disorders that can significantly impede a child's educational trajectory if not identified and addressed in a timely manner. While they both manifest through academic difficulties—particularly in reading and writing—their underlying cognitive and neurological mechanisms differ. Understanding these disorders in depth is crucial for appreciating the significance of recent technological interventions, including artificial intelligence (AI)-driven handwriting analysis, which promises to transform how these conditions are detected and managed.
Dyslexia: A Disorder of Language-Based Processing
Dyslexia is a specific learning disorder characterized by difficulties in accurate and/or fluent word recognition, poor spelling, and decoding abilities. These challenges are typically rooted in deficits in the phonological component of language, making it difficult for individuals to connect sounds with written letters or words. Contrary to popular misconceptions, dyslexia is not indicative of low intelligence or lack of motivation. Many individuals with dyslexia possess average or above-average IQs and exhibit strengths in reasoning, creativity, and problem-solving.
Globally, it is estimated that approximately 5% to 10% of the population is affected by dyslexia, although prevalence rates may vary due to differences in diagnostic criteria, language systems, and educational awareness. In alphabetic languages such as English, dyslexia may present more prominently due to the irregularity of spelling patterns. Children with dyslexia often struggle with phoneme-grapheme correspondence, exhibit letter reversals (such as confusing "b" and "d"), and may read at a slower pace than their peers. These symptoms typically become evident in the early years of formal schooling when reading instruction begins in earnest.
Dysgraphia: The Underrecognized Challenge in Written Expression
In contrast to dyslexia, dysgraphia is a learning disability that primarily affects written expression. It involves impairments in handwriting, spelling, and the organization of written language. Dysgraphia can stem from difficulties with fine motor skills, language processing, or a combination of both. Children with dysgraphia may produce illegible handwriting, inconsistently sized letters, unusual spacing between words or letters, and poor spelling even when they can verbally articulate ideas clearly.
Unlike dyslexia, which has been the focus of substantial public and academic attention, dysgraphia remains underdiagnosed and under-researched. It is frequently mistaken for laziness, lack of effort, or behavioral issues, thereby delaying the implementation of supportive measures. Estimates suggest that between 5% to 20% of school-age children may exhibit symptoms of dysgraphia to varying degrees. Importantly, dysgraphia often coexists with other learning disorders, including dyslexia and attention-deficit/hyperactivity disorder (ADHD), further complicating diagnosis.
Conventional Diagnostic Practices: Limitations and Challenges
Traditional methods of diagnosing dyslexia and dysgraphia involve comprehensive psychoeducational assessments conducted by specialists such as educational psychologists, neuropsychologists, or speech-language pathologists. These assessments typically include standardized tests of reading fluency, spelling, phonological processing, handwriting tasks, and working memory evaluations. Observational reports from teachers and parents also play a critical role in the diagnostic process.
However, these methods face several limitations. First, they are often initiated only after a child has experienced significant academic struggles, which may delay diagnosis by several years. Second, assessments are resource-intensive, requiring specialized personnel, time, and financial investment. This can result in disparities in access to early diagnosis, particularly in under-resourced educational settings or among marginalized communities. Third, the subjective nature of some evaluations may introduce inconsistencies in interpretation, leading to misdiagnosis or overlooked cases.
Furthermore, children often develop coping mechanisms or masking strategies that can obscure early symptoms, making it harder for educators and parents to identify red flags. For instance, a child with dyslexia might memorize sight words to avoid decoding, while one with dysgraphia might produce minimal written output to avoid exposing handwriting difficulties.
The Significance of Early Indicators
Detecting dyslexia and dysgraphia at the earliest possible stage is critical for effective intervention. Research has consistently shown that early intervention significantly improves long-term outcomes in reading fluency, writing competence, and overall academic performance. Early support can also mitigate the psychological impact of learning disorders, such as anxiety, low self-esteem, and school avoidance.
For dyslexia, early indicators may appear in preschool or kindergarten and include delayed speech development, difficulty rhyming, trouble recognizing letters, and limited phonemic awareness. As children begin learning to read, persistent struggles with decoding, blending sounds, or learning sight words become more evident.
In the case of dysgraphia, warning signs may manifest as reluctance to write, complaints of hand fatigue, unusually slow writing speed, and poor alignment or organization on the page. Educators may observe a disconnect between a child’s verbal abilities and their written output. These symptoms may initially be dismissed as developmental variability, causing delays in formal evaluation.
Critically, these early signs often manifest through a child’s handwriting. While it might seem rudimentary or anecdotal to analyze a child’s penmanship, research has shown that handwriting provides a rich source of information about neurological development, motor coordination, visual processing, and cognitive planning. For example, inconsistent letter size or shape may indicate motor control issues, while erratic spacing or alignment could point to spatial processing challenges. Similarly, excessive erasing or corrections may suggest self-monitoring difficulties linked to executive function.
The global disparity between estimated prevalence and confirmed diagnoses of dyslexia and dysgraphia highlights the diagnostic gap. This gap is particularly pronounced in under-resourced educational systems, where access to specialists remains limited. The chart below offers a comparative view of prevalence and diagnosis rates across key countries:
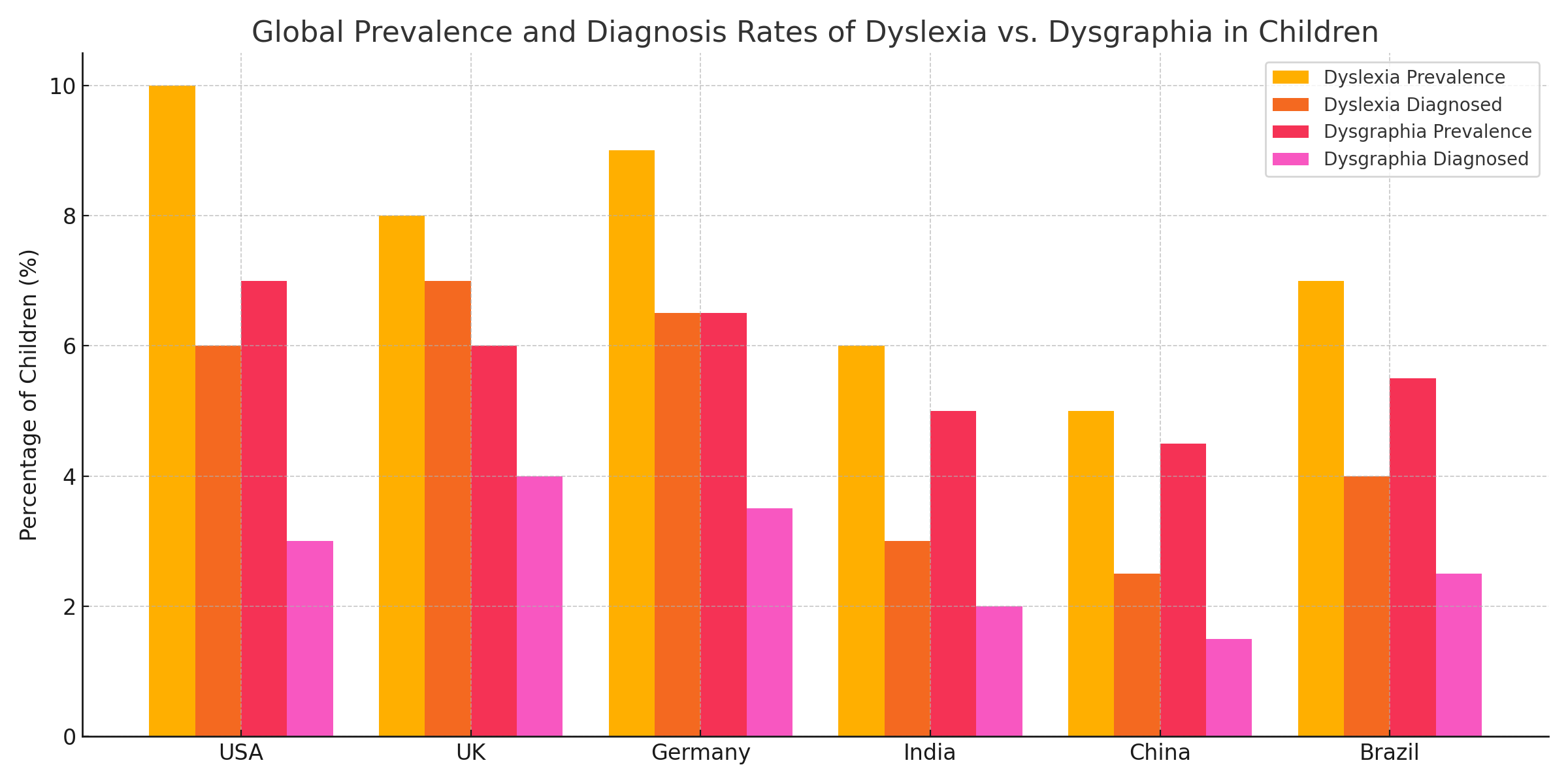
As this data shows, there is a clear need for scalable, objective tools capable of closing the diagnosis gap. This is where artificial intelligence offers transformative promise.
A Converging Point: Handwriting as a Diagnostic Lens
Given that handwriting reflects a complex interplay between multiple brain functions—including motor execution, visual-spatial integration, and language processing—it serves as a valuable window into a child's cognitive development. Recognizing this, researchers and clinicians have increasingly explored the potential of handwriting as a diagnostic lens for detecting learning disorders. Yet, until recently, the manual analysis of handwriting has been limited by human subjectivity, time constraints, and lack of standardized metrics.
This is where AI-driven handwriting analysis holds particular promise. By leveraging machine learning algorithms trained on large, annotated datasets of handwritten samples, AI systems can identify subtle patterns and anomalies that human evaluators might overlook. These systems can quantify aspects such as stroke speed, pressure, curvature, and sequence, providing a granular, objective profile of handwriting behavior. Moreover, AI can detect these markers in real time, offering the potential for dynamic, ongoing assessment rather than a one-time evaluation.
As handwriting continues to decline in emphasis within modern curricula—often displaced by keyboarding and touchscreen input—there is an urgent need to preserve its diagnostic utility through advanced technological means. AI tools are well-positioned to fill this gap, ensuring that handwriting remains a vital source of developmental insight in both educational and clinical contexts.
How AI Analyzes Handwriting – From Pixels to Patterns
The analysis of handwriting has long been a domain reserved for educators, clinicians, and graphologists who rely on visual observation, experiential judgment, and structured tests to assess written output. However, the advent of artificial intelligence (AI), especially in the fields of computer vision and machine learning, has introduced a paradigm shift—enabling an unprecedented level of granularity and scale in the evaluation of handwriting. In the context of diagnosing neurodevelopmental disorders such as dyslexia and dysgraphia, AI offers not only a novel methodology but also a more objective, data-driven approach to early detection.
The Foundations of AI Handwriting Analysis
At its core, AI handwriting analysis utilizes a suite of technologies to examine static and dynamic features of handwriting. This involves the application of image processing algorithms, deep learning models, and temporal behavior tracking to interpret handwriting in ways that mimic—and in many respects surpass—human capabilities. The process typically begins with the acquisition of handwritten input, which can be sourced from physical paper (scanned documents), stylus-based digital interfaces, or smart pens that capture motion data in real time.
Once the input is obtained, the AI system processes it using a combination of convolutional neural networks (CNNs), recurrent neural networks (RNNs), and transformers. CNNs are particularly effective in recognizing visual patterns such as the shape and formation of letters, while RNNs and transformer-based models can analyze the temporal sequence of strokes, allowing the system to understand the order, direction, and fluidity of writing.
This dual-layered analysis—of both visual structure and behavioral dynamics—is crucial in identifying anomalies linked to learning disorders. For instance, a CNN might detect malformed or reversed characters, while an RNN can uncover irregular pressure patterns or abnormal writing speeds. Together, these insights form a comprehensive profile that reveals not just what is written, but how it is written.
Static and Dynamic Feature Extraction
AI handwriting analysis is divided into two primary domains of feature extraction: static and dynamic.
- Static Features: These are characteristics that can be extracted from an image of the written text, such as:For children with dysgraphia, static analysis often reveals a lack of uniformity in letter formation, disjointed writing, and inconsistent baseline adherence. In dyslexia, frequent letter reversals or substitutions (e.g., "p" for "q") can be detected through these visual markers.
- Letter shape and formation
- Alignment on the page
- Consistency in letter size and spacing
- Line orientation and slant
- Connectivity between letters (cursive vs. print)
- Dynamic Features: These are captured via devices that track the motion and force of writing in real time. Key dynamic metrics include:Dynamic data is particularly informative when assessing motor control deficiencies, which are common in dysgraphia. Abnormal pause durations and inconsistent stroke sequences can indicate underlying cognitive or neuromotor issues.
- Stroke order and direction
- Pen pressure variation
- Writing speed and pauses
- Stroke duration and hesitation
- Pen lift frequency
These features, when analyzed collectively by AI systems, enable the generation of a multi-dimensional behavioral map that is statistically compared against benchmarks derived from both neurotypical individuals and those diagnosed with learning disorders.
Technological Ecosystem: Tools and Platforms
Several commercial and academic platforms are already employing AI to analyze handwriting for diagnostic or educational purposes. For example:
- Google's Teachable Machine and AutoML Vision have been leveraged in research settings to train handwriting classifiers that detect early signs of writing-based learning disorders.
- Microsoft's Ink Recognizer API allows applications to digitize and semantically analyze handwriting in real time, providing the scaffolding for more specialized diagnostic modules.
- NeuWrite is a tablet-based solution developed for schools, which uses machine learning to flag atypical handwriting traits among students as early as kindergarten.
- MyScript and Wacom have collaborated on stylus-integrated tablets that capture pressure, tilt, and velocity data, which can be fed into diagnostic models.
These platforms underscore the growing interest in building ecosystems where handwriting data becomes a biomarker—a measurable indicator of cognitive development.
Data Collection and Annotation: Training the Models
A foundational requirement for the effectiveness of AI handwriting analysis is the availability of large, high-quality datasets. Training a machine learning model necessitates thousands of annotated handwriting samples across various age groups, languages, and educational backgrounds. These datasets must be labeled meticulously by experts who classify samples based on the presence or absence of dyslexia or dysgraphia, as well as other relevant conditions.
Some of the leading handwriting datasets include:
- IAM Handwriting Database: Contains both scanned and digital handwriting samples.
- UNIPEN Dataset: Focuses on online pen motion tracking for character recognition.
- UJI Pen Characters Dataset: Offers a broad range of character-level handwriting data from stylus input.
Such datasets are not only used for training but also for validating and fine-tuning AI models. Transfer learning—a method where models trained on large general datasets are adapted to specific tasks—is often employed to customize analysis tools for regional or linguistic variations.
Accuracy, Interpretability, and Feedback Loops
One of the most compelling advantages of AI handwriting analysis is its ability to produce repeatable and quantifiable assessments. Unlike traditional evaluations that may vary based on the examiner’s subjectivity or contextual bias, AI systems apply consistent criteria across all samples. This leads to higher diagnostic accuracy, especially when detecting subtle symptoms that human evaluators might overlook.
However, interpretability remains a key concern. AI-generated outputs must be intelligible to educators and clinicians who rely on these insights to make intervention decisions. To address this, many systems now include explainable AI (XAI) modules that highlight which specific features (e.g., high pen pressure or frequent reversals) influenced the model’s judgment. Heatmaps, trace visualizations, and probability scores are often provided alongside written reports to enhance transparency.
Moreover, some systems incorporate adaptive feedback loops, where results from real-world usage continuously inform model updates. For instance, if a flagged sample is later confirmed by a human specialist to represent a false positive, the model can adjust its parameters accordingly—thereby improving its precision over time.
Deployment Modalities: From Classrooms to Clinics
AI handwriting analysis is versatile in its deployment. In classroom settings, it can be embedded into standard digital learning tools, providing teachers with automated alerts when a student’s writing exhibits signs of developmental delay. In clinical environments, it serves as a preliminary screening tool, expediting referrals to specialists. Additionally, mobile applications and web platforms make it possible for parents to conduct at-home assessments, further democratizing access to early intervention.
Importantly, the integration of handwriting analysis with other diagnostic modalities—such as speech recognition, eye-tracking, or cognitive games—promises to usher in a new era of multi-modal, AI-powered developmental health assessments. Such systems can provide a holistic view of a child’s learning profile, reducing the likelihood of misdiagnosis and enabling tailored educational strategies.
Real-World Case Studies and Pilot Programs
Theoretical advances in artificial intelligence (AI) handwriting analysis have progressively transitioned into real-world applications, especially in the education and clinical domains. Across various countries and institutional contexts, pilot programs and case studies have demonstrated the transformative potential of AI-powered handwriting diagnostics in identifying learning disorders such as dyslexia and dysgraphia. These implementations not only validate the technological feasibility of AI handwriting analysis but also highlight its profound implications for educational equity, early intervention, and systemic reform.
United States: AI Tools in Public School Systems
One of the most notable implementations of AI handwriting analysis occurred in the public school system of a large metropolitan district in California. In collaboration with a private ed-tech company specializing in machine learning for education, the school district launched a pilot program across 25 elementary schools. The initiative involved the use of stylus-enabled tablets equipped with custom handwriting assessment software. Over the course of the academic year, more than 7,000 students in grades K–3 submitted handwriting samples through classroom exercises.
The AI software was trained to detect markers associated with dysgraphia, such as inconsistent pressure, stroke hesitations, spatial misalignments, and abnormal slant angles. Simultaneously, it screened for dyslexia-related features by analyzing frequent letter reversals and spelling inconsistencies in context. The system flagged approximately 14% of the students for potential learning disorders.
Subsequent evaluations by school psychologists and occupational therapists confirmed that nearly 70% of the flagged cases met the diagnostic criteria for either dyslexia, dysgraphia, or both. The remaining false positives were used to refine the algorithm’s sensitivity. Importantly, the flagged students were provided with tailored educational interventions months earlier than would have occurred under traditional screening protocols. Teachers reported improved student engagement and a reduction in behavioral disruptions associated with learning frustration.
This case exemplifies how AI handwriting tools can serve as an initial layer of screening, prompting timely professional evaluation and supporting early-stage intervention within mainstream educational systems.
United Kingdom: NHS-Backed Digital Health Trials
In the United Kingdom, the National Health Service (NHS) funded a pilot project under its Digital Health Strategy to explore non-invasive methods for early detection of developmental disorders in school-aged children. Conducted in partnership with a university-affiliated child development lab, the trial recruited 500 students from five state-funded primary schools.
Children were asked to complete a series of digitized writing tasks using tablets with pressure-sensitive styluses. Tasks included copying passages, writing dictated sentences, and completing unfinished words. The AI model used in this pilot had been pre-trained on thousands of labeled handwriting samples and could identify more than 20 anomaly types.
The results were conclusive. The AI system correctly identified 78% of the children who had existing clinical diagnoses and flagged an additional 10% for further review. Of the newly flagged group, 6% were later diagnosed with dysgraphia or dyslexia by NHS professionals. The NHS reported that the use of AI tools reduced the average time to diagnosis by nearly six months—a significant gain in developmental terms.
Moreover, feedback from educators and parents was overwhelmingly positive. Teachers appreciated the unobtrusive nature of the assessments, which blended seamlessly into regular instruction, while parents welcomed the prospect of earlier, data-backed insights into their child’s learning profile.
India: Low-Cost Implementation in Resource-Constrained Settings
In a country where educational resources are unevenly distributed, the potential of scalable AI tools is especially relevant. A case study in Karnataka, India, involved a collaboration between a local educational NGO and an AI research lab from a prominent Indian Institute of Technology (IIT). The goal was to determine whether affordable handwriting analysis tools could improve the detection of learning disorders in rural schools with limited access to specialized personnel.
Over a six-month period, 1,200 students across 15 government schools participated in a handwriting screening program using basic Android tablets and styluses. The software—developed in-house—emphasized low-computation algorithms capable of running on inexpensive devices without internet connectivity.
Despite these constraints, the system demonstrated a 65% accuracy rate in identifying children later diagnosed with dyslexia or dysgraphia through follow-up assessments conducted by trained volunteers. While less precise than higher-end solutions in developed countries, the pilot illustrated that even basic AI handwriting tools can significantly narrow the diagnostic gap in underserved regions.
The success of the program has led to ongoing discussions with state education authorities about scaling the initiative across other rural districts. It also underscores the importance of inclusive technology design that accommodates infrastructural limitations without sacrificing diagnostic potential.
Japan: Integration with Special Education Programs
Japan’s education system has also begun integrating AI handwriting tools into its well-established special education framework. In a Tokyo-based experimental school for children with diverse learning needs, AI handwriting analysis has been deployed alongside traditional psychological evaluations for students aged 6–12.
The school’s hybrid model involves both teachers and therapists interacting with the AI system, which provides color-coded overlays on student writing samples to highlight areas of concern. For instance, green areas indicate normal stroke patterns, yellow denotes inconsistencies, and red flags represent potential indicators of dysgraphia or dyslexia. This visual feedback has proven useful not only for diagnostics but also for planning customized intervention strategies.
A review of the school’s data over two academic terms found that students identified through AI tools responded better to therapy when interventions were initiated within three months of the initial handwriting anomaly detection. This reinforces the notion that AI can support not just early identification, but also the timing and precision of treatment protocols.
Evaluating Success Metrics and Stakeholder Impact
Across these case studies, certain themes emerge that speak to the broader effectiveness and impact of AI handwriting analysis:
- Time Efficiency: On average, AI tools reduced the time from suspicion to formal diagnosis by 4–6 months.
- Scalability: Even in resource-constrained settings, basic AI systems performed with acceptable levels of diagnostic accuracy.
- Professional Support: AI was not used as a standalone diagnostic tool but as an early-stage filter that complemented expert evaluations.
- Educational Outcomes: Early identification led to timely interventions, often resulting in improved classroom performance and student well-being.
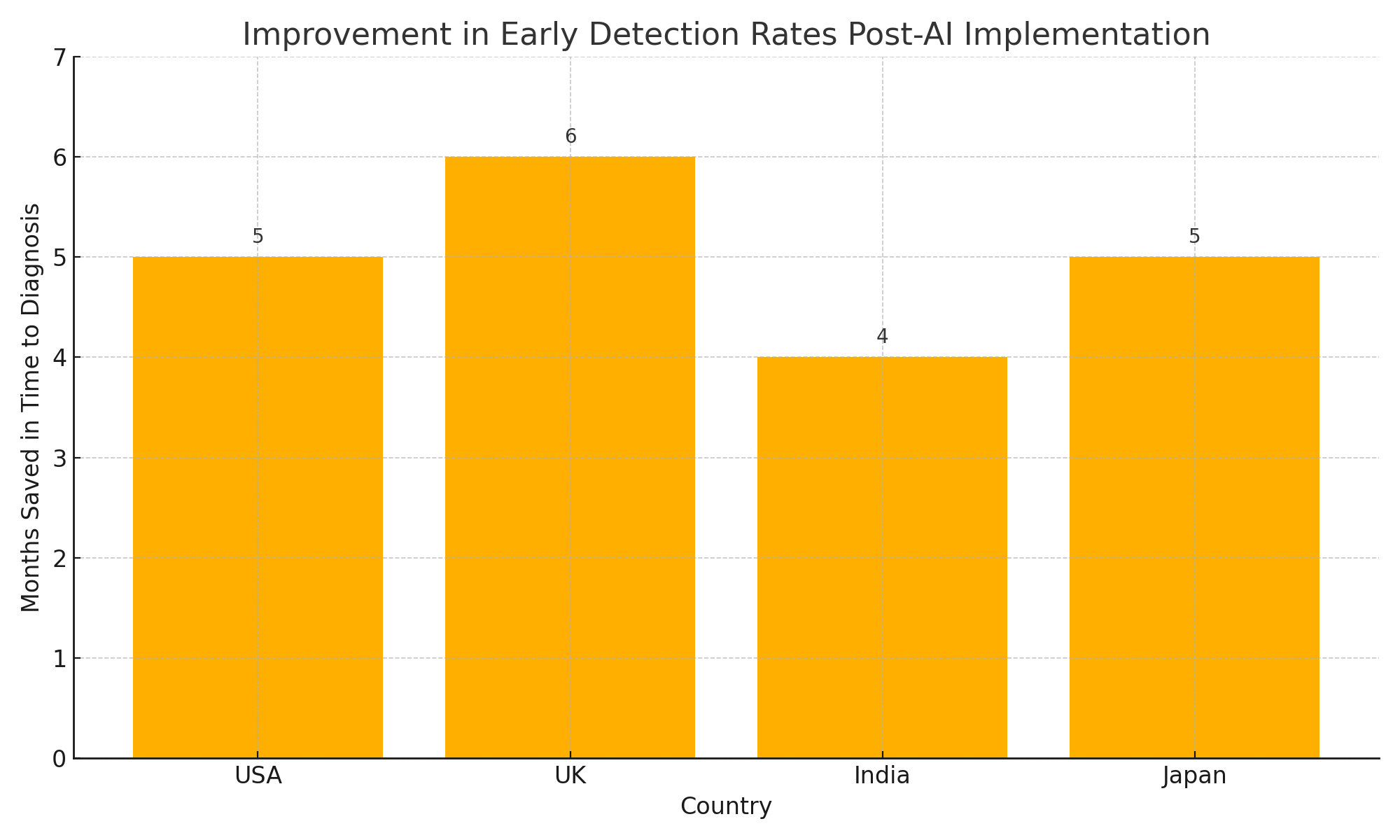
These findings not only validate the operational feasibility of AI handwriting analysis but also demonstrate its value as a proactive educational and clinical instrument. By surfacing learning disorders at earlier stages, these tools are enabling a shift from reactive to preventive care models, with significant long-term benefits for both individuals and systems.
Broader Implications – Educational, Technological, and Clinical
The implementation of artificial intelligence (AI) handwriting analysis in detecting learning disorders such as dyslexia and dysgraphia represents more than just a technological advancement—it signals a profound shift in how educational systems, healthcare providers, and policymakers approach developmental diagnostics. By operationalizing early intervention at scale, this technology introduces both transformative opportunities and critical responsibilities. The broader implications span three major domains: education, technology, and clinical practice, each of which is increasingly interdependent in the context of child development and inclusive learning.
Educational Implications: Toward Proactive and Inclusive Learning
AI handwriting analysis redefines the traditional educational paradigm, transitioning it from reactive remediation to proactive identification and support. In conventional school systems, learning disorders often go unnoticed until a child experiences pronounced academic failure, which can trigger a cascade of consequences—emotional distress, disengagement, and behavioral issues. AI tools interrupt this cycle by enabling schools to identify at-risk students based on objective markers before failure sets in.
Curriculum Integration and Early Screening
One of the most immediate educational implications is the integration of AI-powered screening into early primary curricula. Rather than waiting for teacher referrals or standardized test results, students could be assessed through everyday classroom writing exercises. These assessments, executed seamlessly through digital tablets or styluses, would provide real-time analytics to educators. AI systems could deliver weekly or monthly reports flagging students who exhibit patterns aligned with dyslexia or dysgraphia, prompting timely referrals to support services.
Such integration also supports the principle of universal design for learning (UDL), which emphasizes the creation of educational environments that accommodate diverse learning needs from the outset. By embedding diagnostic tools into the core instructional process, AI helps normalize the practice of differentiated instruction—tailoring educational experiences based on each student’s neurocognitive profile.
Reducing Inequities in Diagnosis
Historically, students from underserved communities have faced systemic barriers in accessing early diagnostic services due to financial, geographic, and institutional limitations. AI handwriting analysis, by virtue of its scalability and low-cost implementation on digital devices, democratizes access to early screening. This has the potential to significantly reduce diagnostic disparities based on socioeconomic status, race, or geographic location.
However, to fully realize this potential, public education systems must ensure equitable distribution of digital infrastructure, teacher training, and parental awareness. Without such structural supports, AI tools could inadvertently widen the gap they are meant to close.
Technological Implications: Innovation, Data Ethics, and Interoperability
The deployment of AI handwriting analysis introduces a host of technological considerations, ranging from model performance to ethical data stewardship. As these tools become more embedded in critical decision-making workflows, ensuring their accuracy, fairness, and accountability becomes paramount.
Model Transparency and Interpretability
Unlike conventional educational software, diagnostic AI models must meet a higher standard of transparency. Educators and clinicians must understand the basis for the system’s recommendations in order to trust and act upon them. This has spurred growing interest in explainable AI (XAI), which focuses on making black-box models interpretable to non-technical users.
For instance, if an AI model flags a student’s handwriting as indicative of dysgraphia, it should also indicate why—e.g., due to excessive pen pressure variation, irregular spacing, or abnormal slant trajectories. Visual overlays, numerical confidence scores, and comparative trendlines are being developed to offer stakeholders clearer insights into the model’s reasoning.
Bias Mitigation and Inclusive Dataset Design
AI systems are only as unbiased as the data they are trained on. If a handwriting analysis model is developed using datasets that disproportionately represent a single language, region, or demographic, it may produce skewed results when applied in diverse contexts. For example, a model trained primarily on English cursive writing may misinterpret certain logographic scripts or left-handed writing styles.
To address this, developers must prioritize the creation and use of multilingual, multiscript, and culturally diverse datasets. Moreover, algorithms must be continuously audited for performance disparities across different student populations. Inclusive dataset design is not merely a technical task but a moral imperative to prevent harm through diagnostic inaccuracy.
Data Privacy and Consent
The collection and analysis of children’s handwriting involve sensitive biometric and behavioral data. This raises important questions about data privacy, ownership, and consent. Regulatory frameworks such as the General Data Protection Regulation (GDPR) and the Family Educational Rights and Privacy Act (FERPA) already impose strict guidelines for the handling of student information.
However, AI handwriting tools introduce novel challenges, such as the long-term storage of keystroke dynamics, pressure sensitivity metrics, and behavioral trends. Policymakers must ensure that data collection practices are transparent, that consent is informed and revocable, and that data is securely stored and used only for its stated purpose.
Open standards for data interoperability will also be essential. As schools and clinics adopt multiple digital tools, seamless integration with student information systems, learning management platforms, and electronic health records will determine the utility and adoption of AI handwriting technologies.
Clinical Implications: Enhancing Early Intervention and Developmental Care
Beyond the classroom, AI handwriting analysis is poised to become a valuable adjunct to pediatric healthcare and developmental psychology. As an initial triage mechanism, it allows clinicians to prioritize cases that require further investigation, thereby reducing diagnostic backlogs and improving case management efficiency.
Multidisciplinary Collaboration
The use of AI handwriting tools promotes a more integrated model of care, where educators, occupational therapists, psychologists, and pediatricians work from a shared evidence base. A flagged handwriting report, complete with temporal and spatial metrics, can be attached to a child’s developmental file, allowing multiple professionals to interpret and act upon the data collaboratively.
This multidisciplinary approach aligns with the broader shift toward personalized and preventive healthcare. Rather than treating symptoms after they become chronic, practitioners can use AI-based insights to initiate early interventions, such as speech-language therapy, motor coordination exercises, or educational counseling.
Enabling Longitudinal Monitoring
Handwriting patterns evolve over time as children develop cognitively and physically. By continuously analyzing writing samples across months or years, AI systems can create longitudinal developmental profiles. These profiles are particularly valuable in monitoring the progression of intervention strategies or the emergence of comorbid conditions such as ADHD or anxiety disorders.
Moreover, longitudinal data allows for comparative benchmarking—not just against population averages, but against an individual’s own trajectory. A student whose writing shows gradual improvement in stroke regularity and spacing over six months may not need intensive intervention, whereas stagnation or regression may prompt additional support.
Telehealth and Remote Diagnostics
AI handwriting tools also extend the reach of clinical diagnostics into remote and underserved areas through telehealth platforms. Parents can upload scanned worksheets or capture handwriting via mobile apps, and clinicians can receive algorithmic assessments alongside traditional observational data. This hybrid model expands access to care and alleviates pressure on centralized diagnostic services.
Policy and Ethical Considerations
The convergence of education, technology, and healthcare in AI handwriting analysis necessitates thoughtful governance. Policymakers must strike a balance between encouraging innovation and safeguarding public interest. Recommended actions include:
- Establishing ethical guidelines for the use of AI in child development diagnostics.
- Providing public funding for inclusive dataset development and educator training.
- Mandating third-party audits of AI systems deployed in public institutions.
- Creating opt-in frameworks for data sharing and consent management.
These measures will ensure that the benefits of AI handwriting analysis are realized without compromising equity, privacy, or accountability.
In sum, the broader implications of AI handwriting analysis extend far beyond technical novelty. They encompass the transformation of educational diagnostics, the reinforcement of ethical technology design, and the enhancement of clinical care for children with learning differences. As these systems mature, the responsibility lies with all stakeholders—technologists, educators, clinicians, and policymakers—to guide their development and deployment in ways that prioritize inclusion, integrity, and human dignity.
Conclusion
Artificial intelligence handwriting analysis has emerged as a pivotal tool in the early detection of dyslexia and dysgraphia, addressing a long-standing gap in traditional educational and clinical diagnostic practices. By harnessing machine learning, computer vision, and behavioral data science, this technology offers a level of precision, speed, and scalability previously unattainable through manual assessments. More importantly, it provides a means to intervene before learning disorders manifest as chronic academic failure, thereby improving both individual outcomes and systemic efficiencies.
Throughout this blog, we have explored the multi-dimensional value that AI handwriting analysis brings to the table. In educational settings, it enables proactive identification of students at risk and supports differentiated instruction that aligns with the principles of universal design. In clinical contexts, it augments developmental screening workflows, facilitates multidisciplinary collaboration, and enables longitudinal monitoring for continuous care. On a broader scale, it offers a powerful lever for equity, making early diagnostics accessible even in resource-constrained environments.
The real-world implementations reviewed—from California public schools to NHS-backed trials in the UK and pilot programs in rural India—demonstrate that this is not a theoretical innovation, but one with immediate, measurable impact. The reduction in diagnostic delays, improvement in intervention planning, and expansion of access to underserved populations collectively affirm the viability and promise of this AI-powered approach.
However, the deployment of such technology also carries ethical and operational responsibilities. Transparent model design, unbiased datasets, privacy safeguards, and stakeholder education are non-negotiable requirements for responsible innovation. As this field continues to evolve, it is imperative that developers, educators, healthcare providers, and policymakers work collaboratively to ensure that these tools are implemented in ways that are both effective and ethical.
Looking ahead, the integration of handwriting analysis with other AI-driven modalities—such as speech pattern analysis, gaze tracking, and cognitive testing—could yield even more holistic diagnostic models. These systems have the potential to redefine how we understand and support neurodiverse learners across their entire educational journey.
In conclusion, AI handwriting analysis is more than a technological breakthrough; it is a catalyst for systemic transformation in how learning disorders are identified and addressed. Its potential to reshape early intervention practices, reduce educational inequities, and support neurodevelopmental research positions it as a cornerstone in the future of inclusive education and developmental healthcare.
References
- International Dyslexia Association – Understanding Dyslexia
https://dyslexiaida.org/dyslexia-basics - Understood.org – What Is Dysgraphia?
https://www.understood.org/en/articles/what-is-dysgraphia - Microsoft – Ink Recognizer Overview
https://learn.microsoft.com/en-us/azure/cognitive-services/ink-recognizer/overview - Wacom – Digital Tools for Education and Handwriting
https://www.wacom.com/en-us/education - Google Research – Teaching Machines to Understand Handwriting
https://ai.googleblog.com/2019/04/teaching-machines-to-read-handwriting.html - MyScript – Digital Handwriting Recognition Technology
https://www.myscript.com/technology/ - Edutopia – How AI Can Help Struggling Readers
https://www.edutopia.org/article/how-ai-can-help-struggling-readers - NHS – Digital Tools for Early Childhood Development
https://www.england.nhs.uk/long-read/using-digital-tools-to-improve-child-development/ - The Brookings Institution – AI and the Future of Learning
https://www.brookings.edu/articles/artificial-intelligence-in-education-promises-and-implications/ - FutureEd – Leveraging Technology for Equitable Education
https://www.future-ed.org/technology-and-equity-in-education/