AI Content Degradation: Understanding the Challenge and Solutions for High-Quality Outputs
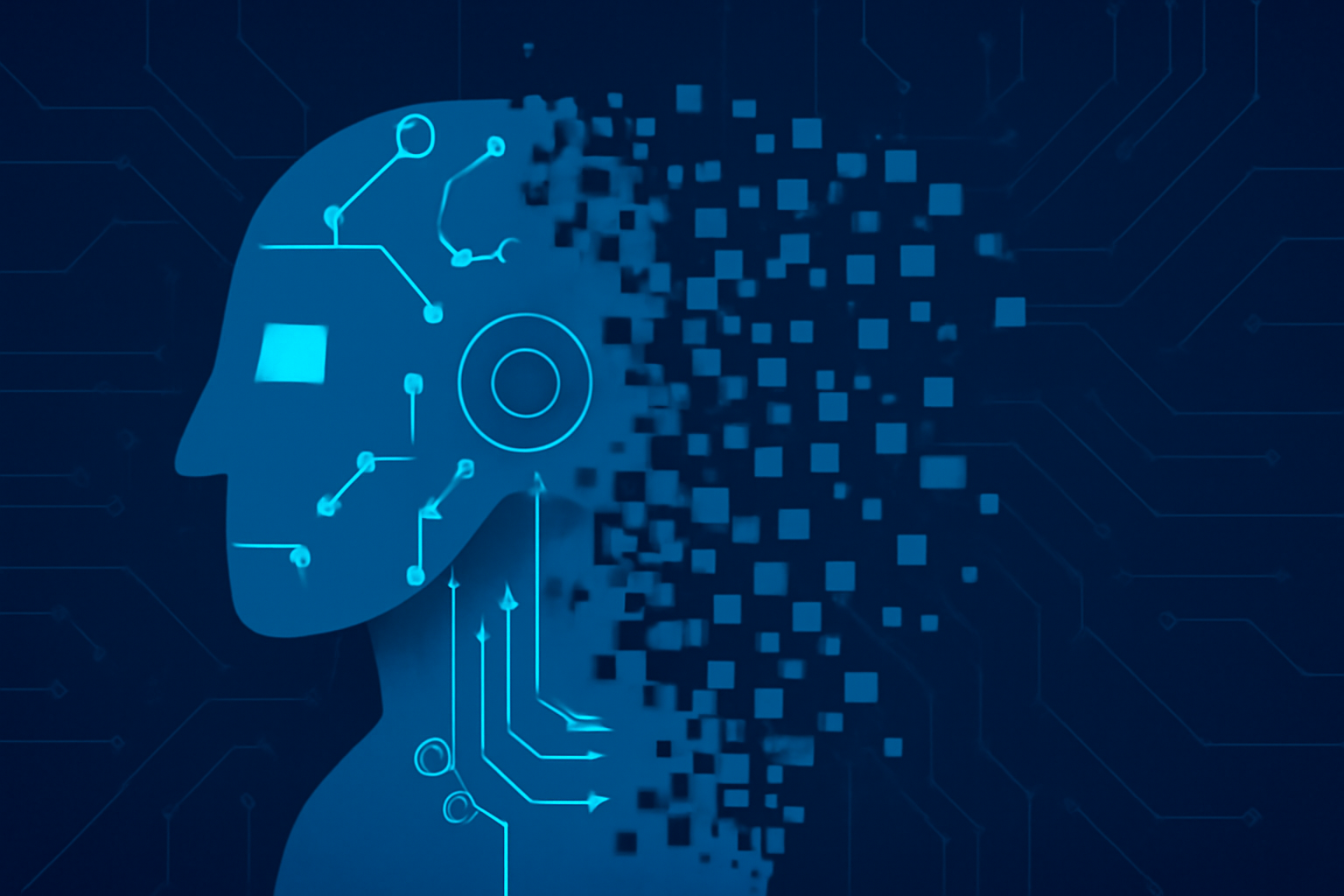
In recent years, artificial intelligence (AI) has made significant strides in transforming industries and processes, with its ability to generate content being one of its most notable accomplishments. From automated customer service responses to content creation for digital marketing, AI-generated content has become increasingly prevalent. However, as AI systems become more widespread, a new and growing challenge has emerged: AI content degradation. This phenomenon refers to the decline in the quality and relevance of content generated by AI models over time, leading to outputs that are increasingly less accurate, less coherent, and less aligned with user expectations.
AI content degradation presents a pressing issue for businesses, developers, and consumers alike, as it can affect the effectiveness of machine learning models and reduce the value of AI-driven content in critical applications. This challenge is particularly important in areas where high-quality content is essential—such as newsrooms, legal services, e-commerce, and marketing. AI-generated content that becomes outdated or less relevant can erode consumer trust, disrupt business operations, and even expose companies to legal risks if inaccuracies or biases are present.
The implications of AI content degradation extend beyond business interests, affecting ethical considerations and regulatory compliance as well. With the growing use of AI in content creation, the need to understand and address the factors contributing to content degradation is more important than ever. This blog post explores AI content degradation in depth, including its causes, real-world impact, and strategies to mitigate it. We will also look at how organizations can leverage advanced techniques to ensure their AI models continue to produce high-quality content that meets industry standards.
In this blog, we will delve into the core aspects of AI content degradation, focusing on:
- The root causes of content degradation in AI models.
- The impact of degraded AI content on businesses and consumers.
- Strategies to mitigate degradation and maintain content quality.
- The future outlook on AI content and ways to enhance its sustainability.
Understanding AI content degradation and its effects is crucial to ensuring that machine learning models continue to contribute positively to society and industries. In the next section, we will examine the specific causes of AI content degradation, offering insight into why these issues arise and how they impact the performance of AI systems.
The Causes of AI Content Degradation
AI content degradation is a multifaceted issue, stemming from various underlying factors that influence the performance of machine learning (ML) models. To better understand how and why AI content quality deteriorates over time, it is essential to identify the primary causes of degradation. These causes are often interconnected, and a combination of them can lead to a gradual decline in the relevance, accuracy, and overall quality of the content generated by AI systems. In this section, we will explore the key drivers of AI content degradation, which include issues related to training data, model limitations, overfitting, underfitting, and content drift.
Training Data Issues
The foundation of any AI model is the data it is trained on, and the quality of this data is crucial to ensuring the accuracy and reliability of the AI-generated content. AI content degradation often arises from the use of subpar, incomplete, or biased training data. If an AI model is trained on flawed datasets, it will likely produce outputs that reflect those same issues. For instance, training data that lacks diversity or does not encompass the full range of possible topics and perspectives can lead to AI models that generate narrow, biased, or incomplete content.
Data quality problems also extend to data labeling, which is a key aspect of supervised learning. Poorly labeled data can confuse the AI model and result in incorrect associations, leading to degraded content. For example, an AI model trained on misclassified text data may produce content that is irrelevant or inaccurate, further diminishing its quality. Additionally, if the training data is outdated or not representative of current trends and language usage, the model may fail to generate relevant content, exacerbating the issue of degradation.
Model Limitations
AI models, regardless of their sophistication, have inherent limitations that can contribute to content degradation. One of the main limitations is the model’s inability to fully understand the context in which it operates. While advanced AI models, such as large language models (LLMs), are capable of processing vast amounts of data, they lack true comprehension of the nuances and subtleties of human language. This can lead to the generation of content that is grammatically correct but lacks depth, accuracy, or relevance.
Moreover, many AI models rely on statistical patterns and probabilities to generate content, rather than a true understanding of meaning. As a result, AI models may produce content that seems plausible at first glance but is factually incorrect or incoherent upon closer inspection. These limitations become more pronounced as the complexity of the content increases, leading to further degradation as the model struggles to maintain quality over time.
Overfitting and Underfitting
Overfitting and underfitting are common issues in machine learning that can significantly affect the quality of AI-generated content. Overfitting occurs when an AI model is trained too closely to the training data, capturing even the noise and random fluctuations in the data rather than just the underlying patterns. While this may lead to high performance on the training set, it results in poor generalization when the model is exposed to new, unseen data. In the context of AI content generation, overfitting can lead to content that is overly specific to the training data, limiting the model’s ability to generate diverse or creative content.
On the other hand, underfitting occurs when the model is too simple or does not learn enough from the training data, leading to poor performance overall. An underfitted model may struggle to generate content that is relevant or meaningful, as it fails to capture the necessary complexity of the task at hand. Both overfitting and underfitting can result in AI content that is either too narrow and repetitive or too generic and uninformative, contributing to the degradation of content quality.
Content Drift
Content drift is another significant factor contributing to AI content degradation. As AI models are exposed to new data over time, the content they generate may become less relevant or accurate due to shifts in language, topics, or user expectations. This phenomenon occurs when the AI model’s performance degrades because it was trained on a snapshot of data that no longer represents the current state of knowledge or trends.
For instance, an AI model trained on content from several years ago may struggle to generate relevant and up-to-date information in fields such as technology, science, or politics. As the world evolves and new information becomes available, the model's outputs may become increasingly outdated or disconnected from current realities. Content drift can also occur when AI models fail to adapt to changes in user preferences or cultural shifts, leading to content that no longer resonates with audiences.
Lack of Adaptability to New Contexts
One of the core limitations of many AI models is their inability to adapt quickly to new contexts or changing circumstances. As AI systems are typically trained on static datasets, they may struggle to adjust to novel or emerging situations. This issue becomes particularly problematic in dynamic environments where user behavior, societal norms, and industry standards are constantly evolving.
In practical terms, this lack of adaptability can result in AI-generated content that is outdated, irrelevant, or even inappropriate. For example, a marketing AI that is not updated regularly may generate campaigns that are not aligned with the latest trends or consumer preferences. Similarly, AI-powered news generation tools may fail to capture the nuances of current events, leading to inaccurate or misleading content.
To better illustrate the long-term effects of AI content degradation, we can visualize how key content quality metrics—such as accuracy, relevance, and user engagement—tend to decline without regular monitoring and updates. The following chart provides a clear depiction of these trends over time, highlighting the gradual deterioration in AI-generated content as a result of outdated training data and the lack of continuous model retraining. By examining this data, it becomes evident that, in the absence of proactive intervention, AI content can quickly fall behind in terms of quality and relevance, reinforcing the importance of maintaining an ongoing update process to sustain high standards.
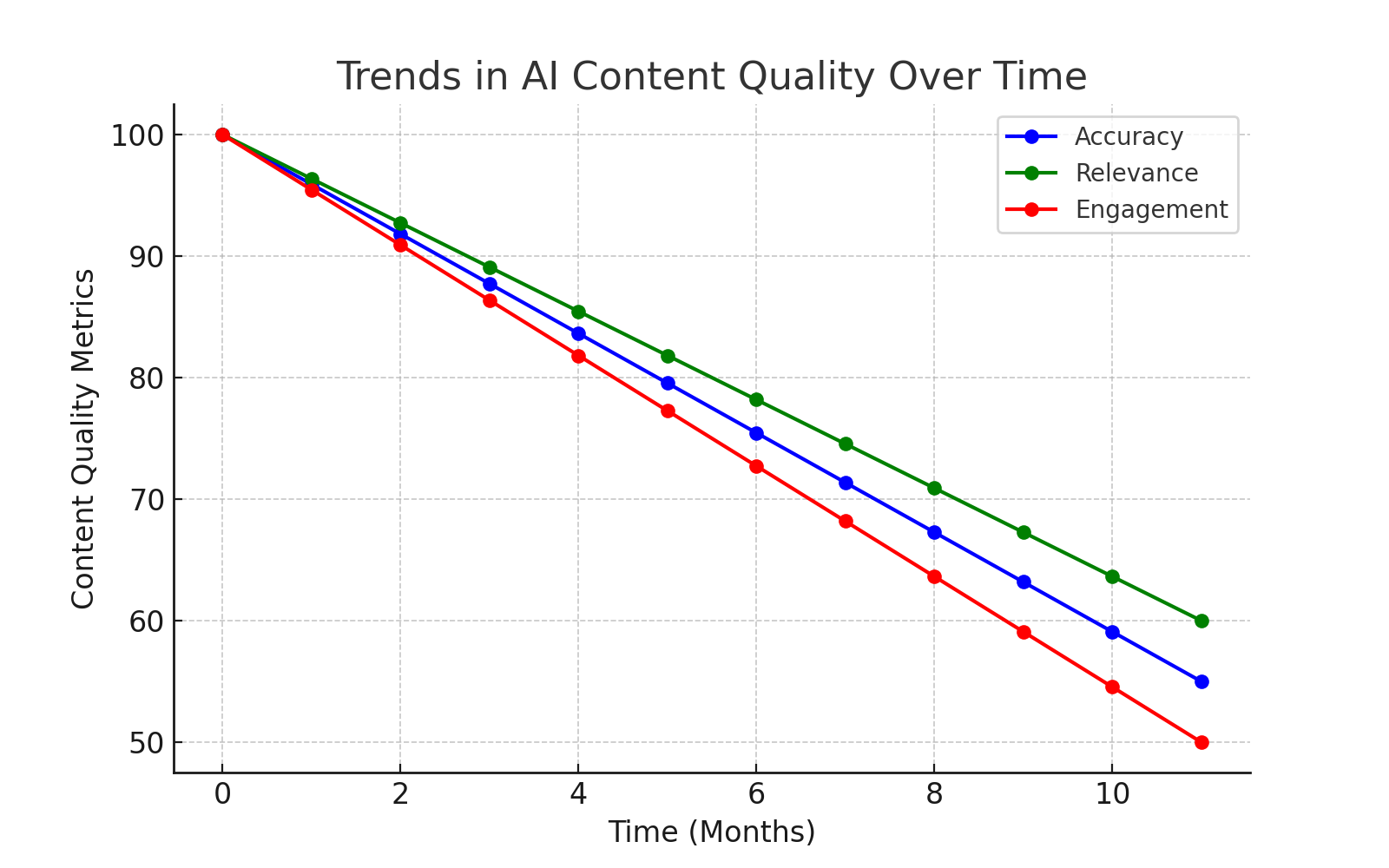
AI content degradation is a complex and multifactorial issue that arises from a combination of factors related to data, model limitations, training issues, and changing contexts. Understanding the causes of content degradation is the first step toward mitigating its effects. In the following sections, we will explore the real-world implications of AI content degradation and the strategies that can be employed to address this growing challenge, ensuring that AI continues to deliver high-quality, relevant, and trustworthy content.
Real-World Implications of AI Content Degradation
AI content degradation is not a theoretical concern—its effects are tangible and can significantly impact businesses, consumers, and society at large. As AI-generated content becomes more prevalent in fields such as marketing, journalism, legal services, and customer service, the consequences of degraded AI content have become a pressing issue. The implications are far-reaching, influencing brand reputation, customer trust, ethical considerations, and even legal frameworks. In this section, we will explore the real-world consequences of AI content degradation, focusing on its impact on businesses, consumers, and broader societal issues.
Impact on Business and Consumer Trust
One of the most immediate consequences of AI content degradation is the erosion of consumer trust. Consumers expect high-quality, accurate, and relevant content, especially when interacting with AI-driven systems in domains such as customer service or product recommendations. As AI-generated content degrades in quality, it becomes more difficult for businesses to meet these expectations, leading to frustration, confusion, and dissatisfaction among customers.
In industries such as e-commerce and digital marketing, where personalized content plays a critical role in driving customer engagement, degraded AI content can have direct financial implications. For instance, AI-driven product recommendations or promotional emails that are irrelevant or based on outdated information may result in lower conversion rates and a decline in overall sales. Similarly, AI-generated content in customer service interactions that is inaccurate or lacks empathy can tarnish a company’s reputation, leading to negative reviews, lost business, and diminished customer loyalty.
Over time, businesses that rely on AI-generated content may face increased scrutiny from both consumers and regulators, especially if the degraded content leads to misinformation, misleading advertisements, or unfair business practices. For example, in the financial services industry, AI-generated reports that contain inaccuracies or fail to address emerging trends may mislead investors, potentially causing financial losses and legal repercussions. Therefore, businesses must be vigilant in monitoring and maintaining the quality of AI-generated content to avoid these negative outcomes.
Ethical Concerns and Biases
The ethical implications of AI content degradation are significant, particularly in areas where accuracy and fairness are paramount. AI models, if not properly trained or monitored, may perpetuate biases present in their training data, leading to the generation of biased or discriminatory content. As AI models degrade over time due to issues such as outdated training data or lack of contextual understanding, these biases can become more pronounced, resulting in content that reinforces harmful stereotypes or promotes discriminatory views.
For example, an AI model used to generate hiring recommendations might produce biased content that discriminates against certain groups based on gender, race, or other factors. As the model’s quality degrades, the likelihood of producing such biased content increases, potentially leading to unethical hiring practices and legal challenges for companies using these AI tools. Similarly, AI-generated news articles or social media posts may unintentionally propagate misinformation or offensive language, causing reputational damage to the organizations involved.
Addressing the ethical implications of AI content degradation requires continuous oversight, regular audits of AI systems, and the implementation of robust ethical guidelines to ensure that the content generated by AI models aligns with societal values and legal standards. Failure to do so could exacerbate the risks associated with AI-generated content and result in significant harm to individuals, communities, and businesses alike.
Legal and Regulatory Risks
As AI-generated content continues to permeate industries, the potential for legal and regulatory risks grows. AI content degradation, particularly when it leads to inaccuracies or violations of laws, can expose organizations to legal liabilities. For instance, AI-generated advertisements that contain misleading or false information could violate consumer protection laws, resulting in lawsuits or fines. Similarly, AI-generated content in the form of contracts, legal documents, or policy recommendations that are incorrect or outdated could lead to costly legal disputes, regulatory scrutiny, and damage to an organization’s credibility.
In sectors like healthcare, finance, and law, the consequences of AI content degradation can be even more severe. Inaccurate AI-generated medical advice, financial reports, or legal documents could lead to severe consequences for both individuals and organizations. For example, an AI system used to generate legal advice may provide guidance that is based on outdated or incomplete laws, resulting in improper recommendations that could harm clients and expose the firm to malpractice claims.
Given the increasing reliance on AI across industries, regulatory bodies are starting to introduce guidelines and frameworks aimed at ensuring the accuracy, transparency, and accountability of AI systems. However, as AI models degrade over time, ensuring compliance with these regulations becomes more challenging. Organizations must stay abreast of these regulations and adopt proactive measures to minimize the risk of legal issues arising from degraded AI content.
Social and Cultural Implications
Beyond the business and legal challenges, AI content degradation has broader social and cultural implications. AI-generated content plays a crucial role in shaping public discourse, influencing opinions, and providing information to diverse audiences. As AI models degrade, the quality and reliability of content can deteriorate, leading to the spread of misinformation, confusion, and polarization.
For example, AI-generated content in the form of news articles, social media posts, or online reviews may become less trustworthy over time if the underlying models are not regularly updated or retrained. In a world where digital platforms are a primary source of information for many people, the degradation of AI-generated content can contribute to the spread of false narratives, conspiracy theories, and divisive content.
The societal impact of degraded AI content is particularly pronounced in areas such as politics, public health, and education. Misinformation generated by AI systems can influence elections, public health decisions, and educational outcomes, ultimately affecting the well-being of entire communities. As AI continues to evolve, addressing the social and cultural implications of content degradation will be critical to ensuring that AI serves as a positive force in society rather than contributing to its fragmentation.
While the first chart underscores the natural decline in AI-generated content over time, it is equally important to assess how AI compares to human-generated content in terms of core performance metrics. The following chart contrasts the effectiveness of AI content with that produced by humans across a range of criteria, including accuracy, creativity, relevance, and user engagement. This comparison highlights the areas where AI excels and where it still lags behind human content creators, reinforcing the need for ongoing refinement in AI models to bridge these performance gaps and ensure that AI can eventually meet or exceed the standards set by human creators.
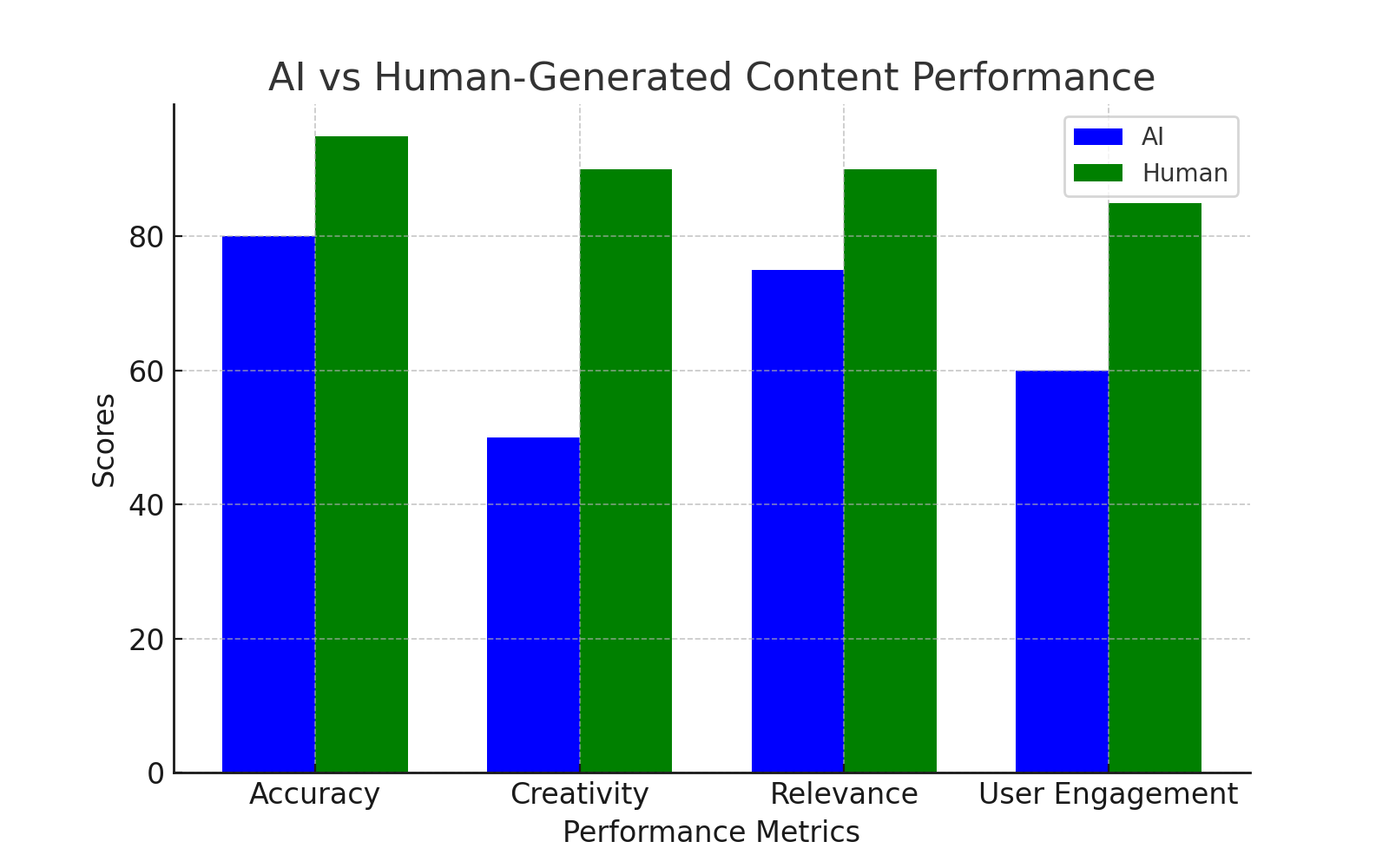
AI content degradation poses significant challenges to businesses, consumers, and society. From undermining trust in AI systems to perpetuating ethical issues and exposing organizations to legal risks, the effects of degraded content are far-reaching. Addressing these challenges requires a multifaceted approach that includes continuous monitoring of AI systems, regular updates to training data, and a commitment to maintaining ethical standards in content generation. In the next section, we will explore strategies for mitigating AI content degradation and maintaining the quality of AI-generated content.
Strategies to Mitigate AI Content Degradation
Mitigating AI content degradation is not only a matter of enhancing the quality of generated content but also of ensuring that AI systems remain robust, adaptable, and aligned with user needs and ethical standards. Addressing this growing challenge requires a combination of technical strategies, proactive management of training data, and the integration of human oversight in AI-driven processes. In this section, we will explore several key strategies that can be employed to mitigate AI content degradation, ranging from improving data quality to implementing continuous monitoring and retraining protocols.
Data Quality Improvement
One of the most effective ways to mitigate AI content degradation is to ensure the quality and diversity of the training data used to train machine learning models. High-quality data serves as the foundation for producing accurate, relevant, and trustworthy AI-generated content. As discussed earlier, degraded AI content is often a result of biased, outdated, or incomplete data. To address this, organizations must invest in curating diverse, comprehensive, and up-to-date datasets that accurately represent the full range of contexts in which the AI model will operate.
Improving data quality involves several key actions:
- Data Cleansing: Remove inconsistencies, errors, and redundancies from the training dataset to ensure that the AI model is learning from clean, accurate data.
- Bias Mitigation: Take steps to identify and eliminate biases present in the data, ensuring that the model produces fair and impartial content. This can involve rebalancing datasets, implementing techniques like adversarial debiasing, and using fairness-enhancing algorithms.
- Continuous Data Updates: Regularly update the training data to reflect the most current trends, facts, and developments in the relevant domain. This helps ensure that the AI model remains in tune with emerging knowledge and language patterns.
By continuously improving the quality and relevance of training data, businesses can ensure that AI-generated content remains accurate, engaging, and aligned with user expectations.
Continuous Monitoring and Retraining
Another essential strategy for mitigating content degradation is the continuous monitoring and retraining of AI models. Over time, even the most advanced AI models can become outdated as they are exposed to new information, changing user behavior, and evolving societal trends. To prevent content degradation, organizations must implement systems for regularly evaluating the performance of their AI models and retraining them with fresh data.
Continuous monitoring involves tracking key performance indicators (KPIs) that reflect the quality of AI-generated content, such as accuracy, relevance, engagement, and user satisfaction. By setting up automated systems for performance evaluation, businesses can identify when an AI model begins to show signs of degradation, such as generating irrelevant or factually inaccurate content. Once degradation is detected, the model can be retrained using updated datasets and new learning techniques to restore its effectiveness.
Retraining is particularly important in fast-changing domains, such as technology, healthcare, and finance, where new developments occur frequently. In these industries, AI models that are not retrained regularly risk producing outdated or misleading content that may harm users or undermine trust in the organization’s services. A proactive approach to model retraining, combined with periodic evaluations, can help mitigate these risks and ensure that the AI model continues to deliver high-quality content.
Human-in-the-Loop (HITL) Solutions
While AI has made remarkable progress in generating content autonomously, it still struggles with tasks that require nuanced understanding, creativity, or context. Human oversight remains a critical element in maintaining the quality of AI-generated content. Human-in-the-loop (HITL) solutions combine the efficiency of AI with the expertise and judgment of human beings to ensure that the generated content meets the desired quality standards.
In the context of AI content generation, HITL approaches can take various forms:
- Human Review and Feedback: AI-generated content can be reviewed and approved by human experts before being published or distributed. This is particularly important in sensitive domains like law, healthcare, and finance, where accuracy and precision are paramount.
- Human Editing and Refinement: Even if an AI model generates high-quality content, it may still require human intervention to improve clarity, tone, or style. Human editors can refine the content to ensure it resonates with the target audience and aligns with brand values.
- Active Learning: This technique involves using human feedback to iteratively improve the model’s performance. When the model produces content that is subpar, human experts can provide corrections or annotations that guide the model’s learning process, helping it generate better outputs over time.
Incorporating human oversight into AI systems helps mitigate the risks of content degradation by ensuring that the model is producing content that aligns with ethical standards, user needs, and industry requirements. HITL solutions are particularly valuable in contexts where the consequences of errors—such as misinformation or biased content—can have serious repercussions.
Benchmarking and Evaluation Frameworks
Another key strategy to combat AI content degradation is the implementation of benchmarking and evaluation frameworks. By regularly assessing the performance of AI-generated content against predefined benchmarks, organizations can identify when content quality begins to degrade and take corrective action before it becomes a significant issue.
Benchmarking involves setting clear, measurable criteria for content quality, such as:
- Accuracy: Ensuring that AI-generated content is factually correct and up to date.
- Relevance: Evaluating whether the content aligns with the user’s intent and provides useful information.
- Engagement: Measuring how well the content resonates with the target audience, whether through metrics such as click-through rates or social media shares.
- Bias and Fairness: Assessing whether the content is free from bias and meets ethical standards.
Establishing these benchmarks allows organizations to track the performance of their AI models over time, ensuring that any degradation is detected early and addressed swiftly. Evaluation frameworks also provide valuable insights into areas where the AI model may need improvement, enabling businesses to refine their models to meet the evolving needs of their users.
Regularly Updating Algorithms and Model Architecture
As AI technology evolves, so too must the algorithms and model architectures used in content generation. Outdated algorithms or models with suboptimal architecture can contribute to content degradation by limiting the AI’s ability to adapt to new information and contexts. Regularly updating algorithms and adopting state-of-the-art architectures ensures that AI models remain competitive and capable of producing high-quality content.
For example, incorporating recent advancements in deep learning, natural language processing, or transfer learning can improve the model’s understanding of complex language and its ability to generate contextually relevant content. By staying abreast of the latest developments in AI research and integrating these advancements into existing models, businesses can enhance the resilience of their AI systems against content degradation.
Mitigating AI content degradation requires a comprehensive approach that combines technical strategies, continuous monitoring, and human oversight. By improving data quality, retraining models regularly, incorporating human-in-the-loop solutions, and establishing benchmarking frameworks, organizations can ensure that their AI systems continue to produce high-quality, reliable content. In doing so, businesses can maintain user trust, uphold ethical standards, and stay compliant with legal and regulatory requirements. The next section will examine the future of AI content generation and the ongoing challenges and opportunities in combating content degradation.
The Future of AI Content and Mitigating Degradation
As artificial intelligence (AI) continues to transform industries and reshape the way content is generated, the challenge of content degradation is likely to grow in significance. The growing reliance on AI-generated content across sectors such as marketing, journalism, healthcare, finance, and entertainment means that ensuring the accuracy, relevance, and ethical integrity of this content is more critical than ever. AI content degradation, driven by factors like poor training data, model limitations, overfitting, and content drift, poses real risks to businesses, consumers, and society. However, as this issue gains recognition, strategies to mitigate content degradation are becoming more sophisticated, and the future of AI content generation holds promise for more reliable, high-quality outputs.
The Evolving Role of AI in Content Creation
The future of AI-generated content is not one of diminishing quality but rather one of continuous improvement and refinement. Over the coming years, advancements in machine learning models, natural language processing, and neural networks will likely lead to significant improvements in the quality and reliability of AI-generated content. Already, we are seeing breakthroughs in areas like context understanding, creativity, and multilingual content generation, which promise to address some of the limitations contributing to content degradation. As AI systems become better at learning and adapting to diverse contexts, the outputs generated will become more nuanced, accurate, and aligned with user needs.
Moreover, the integration of cutting-edge technologies such as reinforcement learning and transfer learning will enable AI models to become more adaptable to dynamic environments, reducing the impact of content drift. These advancements will not only improve content quality but will also make AI-generated content more personalized and contextually relevant, enhancing its utility across a broader range of industries.
However, despite these promising developments, the challenges associated with content degradation are unlikely to disappear entirely. AI models will continue to require ongoing updates, regular monitoring, and human oversight to maintain their relevance and effectiveness. Ensuring that AI-generated content remains high-quality will be a constant balancing act, requiring a combination of technological innovation and human intervention.
The Importance of Collaboration Between AI and Human Expertise
One of the most significant takeaways from addressing AI content degradation is the need for collaboration between AI systems and human expertise. While AI has the potential to revolutionize content generation, it is not without its limitations, particularly when it comes to understanding context, creativity, and ethical considerations. The future of AI content creation will likely involve hybrid models where AI and human intelligence work in tandem to produce content that is both accurate and engaging.
Human oversight, as discussed earlier, will remain a critical component in maintaining the quality of AI-generated content. Whether through human-in-the-loop (HITL) solutions or expert reviews, humans can provide the nuanced judgment and ethical considerations that AI models, regardless of their sophistication, cannot fully replicate. This collaborative approach will help ensure that AI content meets the highest standards of accuracy, relevance, and fairness.
Furthermore, integrating human expertise into AI systems can help address the growing concerns surrounding AI content biases, misinformation, and ethical risks. Humans can provide essential feedback on AI-generated outputs, correcting any inaccuracies, adjusting for biases, and ensuring that content aligns with social values and regulatory requirements. This partnership will be essential for maintaining public trust in AI systems and preventing the spread of harmful content.
Ethical Considerations and Regulation
As AI becomes more integrated into content creation processes, the ethical implications of AI-generated content will continue to be a central concern. The degradation of AI content, particularly when it leads to misinformation, biased narratives, or unethical content, poses risks not only to businesses but also to society at large. In the future, we can expect a greater emphasis on ethical AI development, with stricter guidelines and regulatory frameworks to govern the use of AI in content generation.
Governments, regulatory bodies, and industry leaders will need to collaborate to develop comprehensive frameworks that ensure AI content is produced ethically, transparently, and with accountability. These regulations will likely focus on data transparency, the prevention of biased or discriminatory content, and the establishment of standards for content accuracy and authenticity. As AI technologies continue to evolve, regulations must also adapt to address new challenges and ensure that AI-generated content serves the public interest.
Moreover, the increased focus on ethical AI will also prompt organizations to adopt best practices for the development and deployment of AI models. This could involve adopting explainable AI principles, where the decision-making processes of AI systems are made transparent and understandable to users and stakeholders. By ensuring that AI systems operate in a transparent, accountable, and ethical manner, businesses can reduce the risks associated with content degradation and enhance the societal value of AI-generated content.
The Role of AI Governance and Accountability
As the volume of AI-generated content continues to rise, organizations must prioritize AI governance and accountability. Proper governance frameworks will ensure that AI systems remain under continuous scrutiny, with clear guidelines for evaluating performance, addressing degradation, and ensuring that models align with both business objectives and societal values.
Governance will need to cover several key areas, including:
- Performance Metrics: Establishing comprehensive metrics to evaluate the quality, accuracy, and relevance of AI-generated content. These metrics will help organizations track and mitigate content degradation over time.
- Data Stewardship: Ensuring that training data is carefully curated, regularly updated, and free from biases or inaccuracies that could contribute to content degradation.
- Ethical Oversight: Implementing ethical guidelines and oversight mechanisms to prevent the generation of biased or harmful content. This includes regular audits and reviews of AI-generated content to ensure compliance with ethical standards.
- Transparency and Accountability: Ensuring that AI systems are transparent, with clear documentation of their decision-making processes and the ability to explain how content is generated. This will help build trust among users and stakeholders, fostering greater acceptance of AI-generated content.
As organizations implement these governance practices, they will be better positioned to address the challenges of AI content degradation while harnessing the full potential of AI for content generation.
Looking Ahead: The Ongoing Challenge
Despite the advancements in AI technologies and the strategies discussed in this blog, mitigating content degradation will remain an ongoing challenge. AI models are inherently limited by their data and design, and as they are exposed to new information, shifts in societal norms, and emerging trends, they will continue to require regular updates, retraining, and oversight. However, by investing in quality data, integrating human oversight, establishing ethical guidelines, and fostering AI governance, businesses and organizations can ensure that AI continues to generate high-quality, relevant content.
The future of AI content lies in striking a balance between technological innovation and responsible implementation. By proactively addressing content degradation and maintaining a commitment to ethical AI practices, we can harness the potential of AI to enhance content creation without compromising its quality, relevance, or societal impact. The road ahead is one of continuous learning and adaptation, but with the right strategies in place, AI content can continue to evolve and thrive in an ever-changing world.
References
- https://www.technologyreview.com/ai-content-degradation/
- https://www.forbes.com/sites/ai-content-challenges/
- https://www.sciencedirect.com/ai-content-quality
- https://www.theverge.com/ai-content-implications
- https://www.wired.com/ai-ethics-content-degradation/
- https://www.bbc.com/ai-generated-content-risks
- https://www.springer.com/ai-degradation-in-machine-learning
- https://www.analyticsvidhya.com/ai-content-degradation-explained
- https://www.techradar.com/news/ai-generated-content-quality-future
- https://www.cnbc.com/ai-degradation-and-data-quality-in-machine-learning