ACE-Step: The AI Breakthrough Powering the Future of Music Generation Models
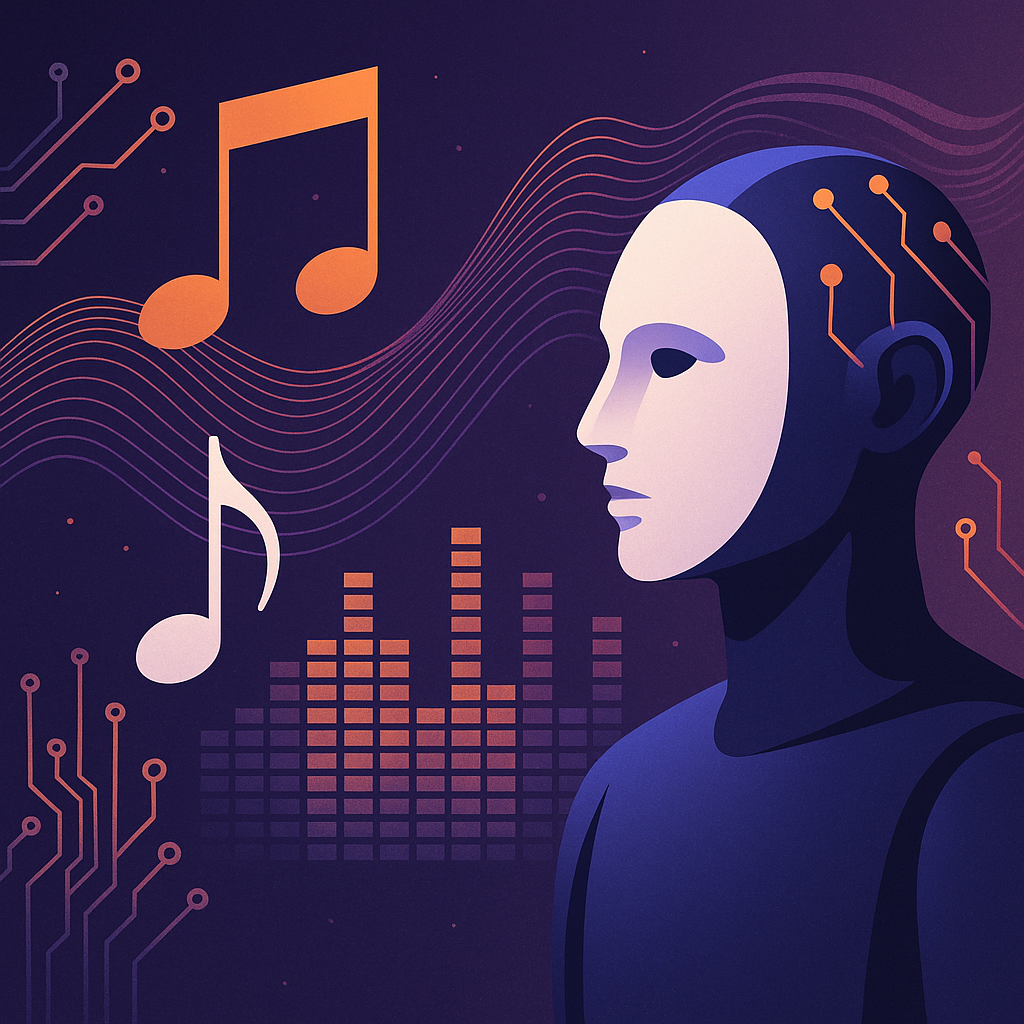
In recent years, the landscape of artificial intelligence has been dramatically reshaped by the rise of foundation models—large-scale, pre-trained architectures capable of generalizing across a wide range of tasks with minimal fine-tuning. These models, exemplified by breakthroughs such as GPT for language and CLIP for vision, have demonstrated remarkable versatility, scalability, and adaptability. Their success has catalyzed similar innovations across domains, prompting researchers to explore the development of foundational AI systems in fields as diverse as robotics, biology, and music. However, while text and vision have enjoyed rapid and widespread advances, music generation remains a relatively underexplored frontier in the foundation model paradigm.
The generation of music via artificial intelligence presents a unique set of challenges. Unlike language or images, music is inherently multi-dimensional and temporally complex. It must encapsulate both local coherence—such as melodic phrasing or rhythmic consistency—and global structure, including thematic development and harmonic progression. Furthermore, music is deeply intertwined with human emotion and cultural context, making its computational modeling a task that requires not only technical sophistication but also a nuanced understanding of artistic expression.
Against this backdrop, ACE-Step emerges as a pioneering framework that aims to establish the groundwork for a foundation model in music generation. Designed to address the limitations of prior systems, ACE-Step introduces a modular, scalable, and hierarchical architecture capable of capturing music’s intricacies at multiple levels of abstraction. By unifying key innovations in attention mechanisms, compression strategies, and expressive embedding representations, ACE-Step aspires to bridge the gap between current generative models and the aspirational goal of an AI system that can generate coherent, high-fidelity, and emotionally resonant musical compositions across genres and styles.
The significance of ACE-Step extends beyond the academic and technological realms. As generative AI becomes increasingly integrated into creative workflows, the ability to produce music autonomously or collaboratively with human creators holds profound implications for the future of entertainment, media, and artistic expression. From real-time soundtrack generation in video games to AI-powered composition assistants for film scoring and digital content creation, the potential applications of such a model are far-reaching.
This blog post provides a comprehensive analysis of the ACE-Step model and its potential to transform AI-driven music generation. It will explore the current state of music AI, delve into the architectural and training innovations introduced by ACE-Step, examine its scalability and performance, and evaluate its applications in real-world scenarios. By contextualizing these advances within the broader trajectory of foundation model research, the discussion will underscore how ACE-Step represents a crucial step toward realizing a universal, adaptable, and musically literate AI system.
The State of AI in Music Generation
Artificial intelligence has profoundly influenced many creative domains, and music is no exception. The journey from simple rule-based composition engines to today’s sophisticated deep learning frameworks illustrates both technological progress and the increasing complexity of modeling musical intelligence. Yet, despite these advancements, music generation still presents unresolved challenges that set it apart from other areas of generative AI. In this section, we examine the current landscape of AI-driven music generation, highlighting key milestones, existing model architectures, persistent challenges, and the emerging necessity for a foundation model capable of general-purpose music synthesis.
The early stages of AI in music generation were dominated by symbolic approaches. Rule-based systems, grounded in music theory, operated within clearly defined constraints, producing outputs that were often predictable and rigid. These systems lacked the flexibility to adapt to genre variations or complex compositional styles. As machine learning matured, statistical models such as Hidden Markov Models (HMMs) and probabilistic graphical models gained popularity. These methods introduced stochasticity, enabling the generation of more varied musical sequences, but they struggled to maintain coherence over extended time spans and often failed to capture deeper structural elements of music.
The real transformation began with the advent of deep learning. Recurrent Neural Networks (RNNs), particularly Long Short-Term Memory (LSTM) networks, emerged as the leading models for sequential data, including music. Projects like Google’s Magenta introduced architectures such as MusicVAE and PerformanceRNN, which demonstrated the capability to generate expressive and stylistically varied compositions. LSTMs allowed models to preserve context over longer sequences, a crucial factor in generating music that feels narratively complete. However, even these models encountered limitations in capturing hierarchical structures and managing the large-scale dependencies that characterize complex compositions.
Subsequently, attention mechanisms and Transformer-based models revolutionized the field. Inspired by their success in natural language processing, researchers adapted Transformers for music generation, yielding impressive results. OpenAI’s Jukebox, for instance, leveraged a multi-stage VQ-VAE pipeline followed by an autoregressive Transformer to generate high-fidelity raw audio across genres. Unlike previous symbolic models, Jukebox operated directly on waveform data, producing outputs with rich textures and stylistic fidelity. However, it required substantial computational resources and lacked real-time interactivity, posing practical limitations for broader adoption.
Similarly, Google’s MusicLM introduced a text-to-music generation model that used audio embeddings and hierarchical generation techniques to translate textual descriptions into structured, high-quality music. MusicLM’s use of semantic and acoustic modeling helped achieve a level of musicality previously unattainable with simpler models. Nonetheless, it also relied on a multi-stage pipeline with significant pretraining and fine-tuning complexity, highlighting the difficulty of creating an end-to-end generalist music generator.
Other noteworthy contributions include Riffusion, which creatively generated music using latent diffusion models and spectrogram-based image representations, and Dance Diffusion by Harmonai, which explored timbre and beat synthesis using a diffusion model trained on short audio clips. While innovative, these models often lacked the ability to sustain musical form over longer time scales and required substantial domain-specific customization.
Despite the variety of approaches, most existing models are narrowly scoped, optimized for specific tasks such as melody generation, accompaniment, or audio texture synthesis. They are rarely capable of adapting flexibly across genres, instrumentation, or temporal resolutions. Furthermore, many rely on either symbolic MIDI inputs or complex audio tokenizations that do not scale well across different formats or user applications. This task fragmentation reveals a pressing need for a unifying framework—one that can serve as a “foundation model” for music in the same way GPT models serve text or CLIP serves vision-language tasks.
A foundation model for music must address several core challenges. First, music is deeply hierarchical: it contains patterns across multiple levels, from short motifs to entire movements. Capturing this structure requires models that can handle both fine-grained details and long-term dependencies. Second, musical representation is multifaceted. Models must balance symbolic abstraction (e.g., pitch, duration, chords) with sonic realism (e.g., timbre, dynamics, rhythm). Third, music generation often requires conditioning on various modalities—text descriptions, visual stimuli, or user input—necessitating models that are not only musically proficient but also multimodally intelligent.
Human preference and subjective quality evaluation pose additional obstacles. Unlike textual tasks, where metrics such as BLEU or perplexity offer proxies for performance, music evaluation is highly nuanced. It often relies on human listeners to judge emotional impact, stylistic authenticity, and aesthetic coherence. As such, any foundation model must also incorporate mechanisms for preference alignment and feedback integration.
Given these complexities, the current state of music generation remains fragmented and exploratory. While models like Jukebox and MusicLM have pushed the boundaries of what is possible, their architecture, training cost, and narrow scope limit their generalization potential. What is missing is a scalable, general-purpose system—trained on diverse, large-scale musical corpora and capable of generalizing across tasks with minimal reconfiguration.
The development of such a model demands innovation across multiple fronts: novel tokenization methods that efficiently represent musical information, hierarchical architectures that capture structure at different time scales, and robust training frameworks that integrate symbolic, waveform, and embedding-based modalities. It also requires datasets that are diverse in genre, style, and instrumentation, along with aligned annotations and multimodal metadata to support conditioning and evaluation.
The emergence of ACE-Step is poised to address many of these requirements. By synthesizing advances from language modeling, audio generation, and hierarchical learning, ACE-Step represents a pivotal advancement toward a unified, adaptable, and scalable music generation framework. Its modular design aims to accommodate various input and output formats, while its attention-compression-embedding structure is tailored to manage both the breadth and depth of musical information.
To contextualize the progress in this field, the following chart illustrates the major milestones in AI music generation over the past two decades:
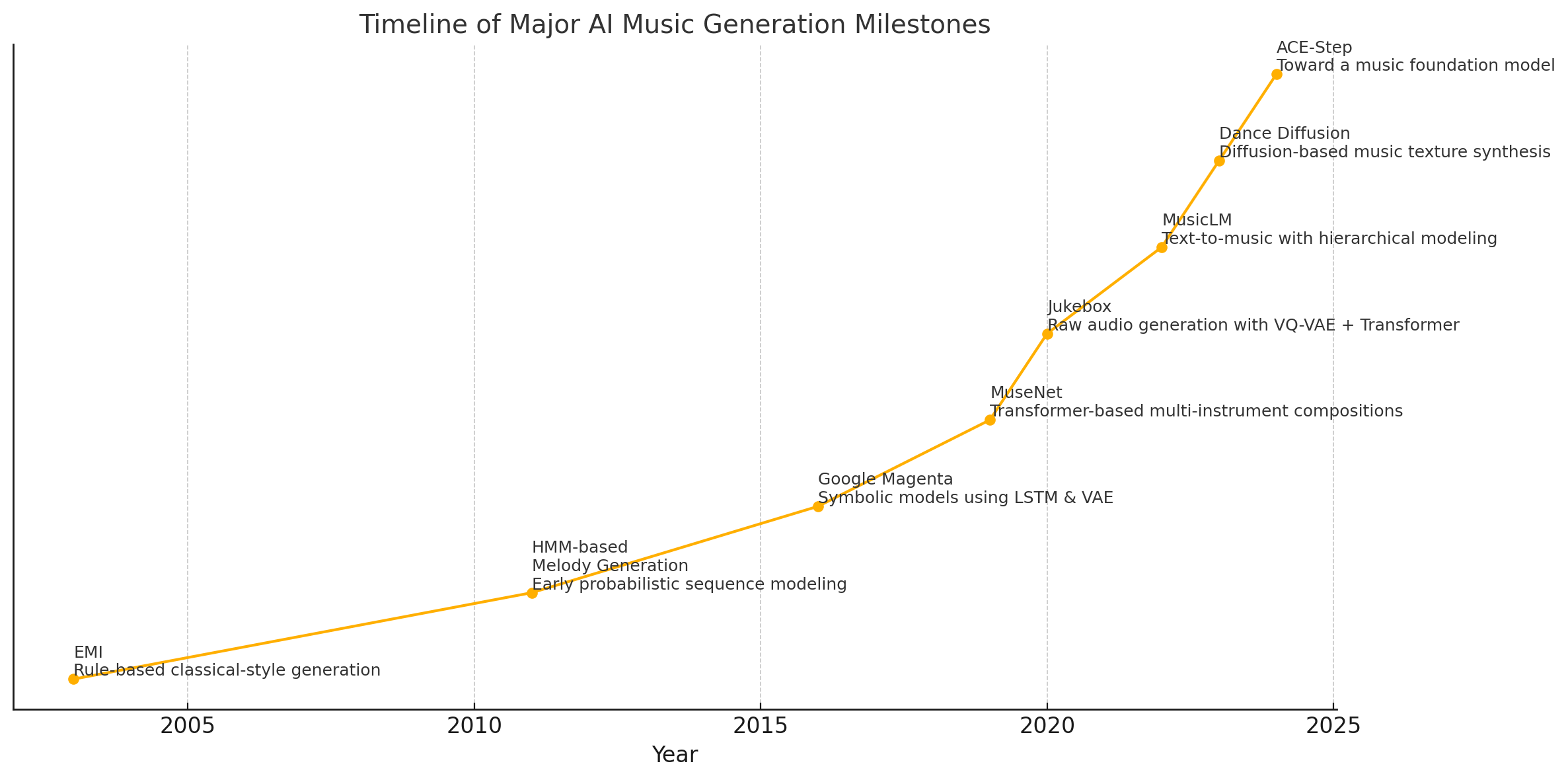
This historical trajectory reveals the evolution from rigid, rule-based systems to the flexible and expressive models of today, and the field is now primed for the emergence of foundational systems that can serve a broader range of music generation tasks. ACE-Step, with its architectural innovations and foundational ambitions, may well mark the beginning of this new chapter.
Introducing ACE-Step Architecture
The development of a robust and generalizable music generation model necessitates architectural innovations that go beyond incremental improvements. With the introduction of ACE-Step, researchers propose a modular and scalable architecture that seeks to address the multifaceted demands of generating coherent, high-quality, and expressive music across various styles, durations, and contexts. Built around three foundational pillars—Attention, Compression, and Embedding—the ACE-Step architecture is designed to capture the inherent complexity and layered structure of music through hierarchical learning and efficient representation mechanisms.
Architectural Overview
The ACE-Step architecture is composed of multiple integrated components, each contributing a specific functionality aligned with the model’s overarching design goals. The core principle is that musical information—ranging from symbolic notation to raw audio—must be represented, processed, and synthesized in a manner that preserves both fine-grained temporal detail and large-scale compositional structure. To this end, ACE-Step introduces a tiered processing pipeline that supports multi-resolution modeling.
- Attention Mechanisms: Inspired by the success of Transformer models in natural language processing and music generation, ACE-Step incorporates a multi-headed attention framework at several levels of its hierarchy. These attention layers enable the model to learn complex dependencies over long temporal spans, which is critical for music, where thematic development and motif recurrence are essential. Unlike standard Transformers, ACE-Step employs sparse and grouped attention strategies to manage memory usage and improve efficiency over extended sequences.
- Compression Modules: A fundamental challenge in music generation is the sheer volume of data, particularly when working with high-fidelity audio. To address this, ACE-Step integrates autoencoder-based compression modules, similar in spirit to VQ-VAE architectures. These modules reduce the dimensionality of audio or symbolic data while retaining key musical features such as pitch, rhythm, dynamics, and timbre. The compressed representations serve as the model’s working tokens, facilitating scalable training and generation.
- Embedding Layers: ACE-Step introduces context-aware embedding layers that unify various modalities—symbolic tokens, audio segments, metadata (e.g., tempo, instrumentation), and external conditioning (e.g., textual prompts). These embeddings are processed through hierarchical encoders that map them into a shared latent space, enabling the model to seamlessly integrate different sources of information. This design empowers ACE-Step to function across tasks such as melody continuation, chord harmonization, and even text-conditioned music generation.
Input Representation and Hierarchical Design
To model the temporal and structural intricacies of music, ACE-Step employs a hierarchical sequence modeling approach. At the lowest level, the model processes short musical fragments—such as beats or bars—capturing fine-grained rhythmic and melodic details. These representations are then aggregated into higher-level structures such as phrases, sections, and entire pieces, allowing ACE-Step to reason over both micro and macro musical forms.
The input format varies depending on the training modality. For symbolic music, ACE-Step uses MIDI-derived tokens that represent pitch, duration, velocity, and instrument information. For audio-based training, it leverages spectrograms and quantized embeddings generated through vector quantization. These inputs are encoded and aligned within a temporal context window, enabling the model to understand relative timing and harmony.
The hierarchical nature of ACE-Step also allows for bidirectional generation, where the model can not only generate music from beginning to end but also fill in missing segments, interpolate between motifs, and perform style transfer across segments. This versatility is key to enabling flexible composition workflows and collaborative applications.
Training Objectives and Optimization
ACE-Step is trained using a composite loss function that integrates reconstruction loss, contrastive loss, and predictive loss:
- Reconstruction Loss ensures that the decoded output retains fidelity to the input, which is critical in preserving musical nuances.
- Contrastive Loss aligns different representations of the same musical idea (e.g., symbolic and audio), thereby enhancing the robustness of the shared embedding space.
- Predictive Loss encourages the model to learn sequence continuations, enabling it to generate musically plausible next segments in an autoregressive or masked modeling setting.
The training process is distributed across multiple GPUs using gradient checkpointing and mixed-precision training to maximize scalability. The dataset is dynamically sampled to include a diverse range of musical styles, keys, and instrumentation, with data augmentation techniques such as pitch shifting and time stretching used to improve generalization.
Comparison with Existing Models
ACE-Step distinguishes itself from prior music generation models in several meaningful ways. Unlike OpenAI’s Jukebox, which operates solely on audio and requires multi-stage sampling, ACE-Step supports both symbolic and audio generation through a unified architecture. Similarly, while MusicLM achieves high-quality results through semantic modeling and hierarchical generation, it is limited in its conditioning flexibility and task adaptability.
The following table summarizes key architectural and functional differences between ACE-Step and prominent models in the field:
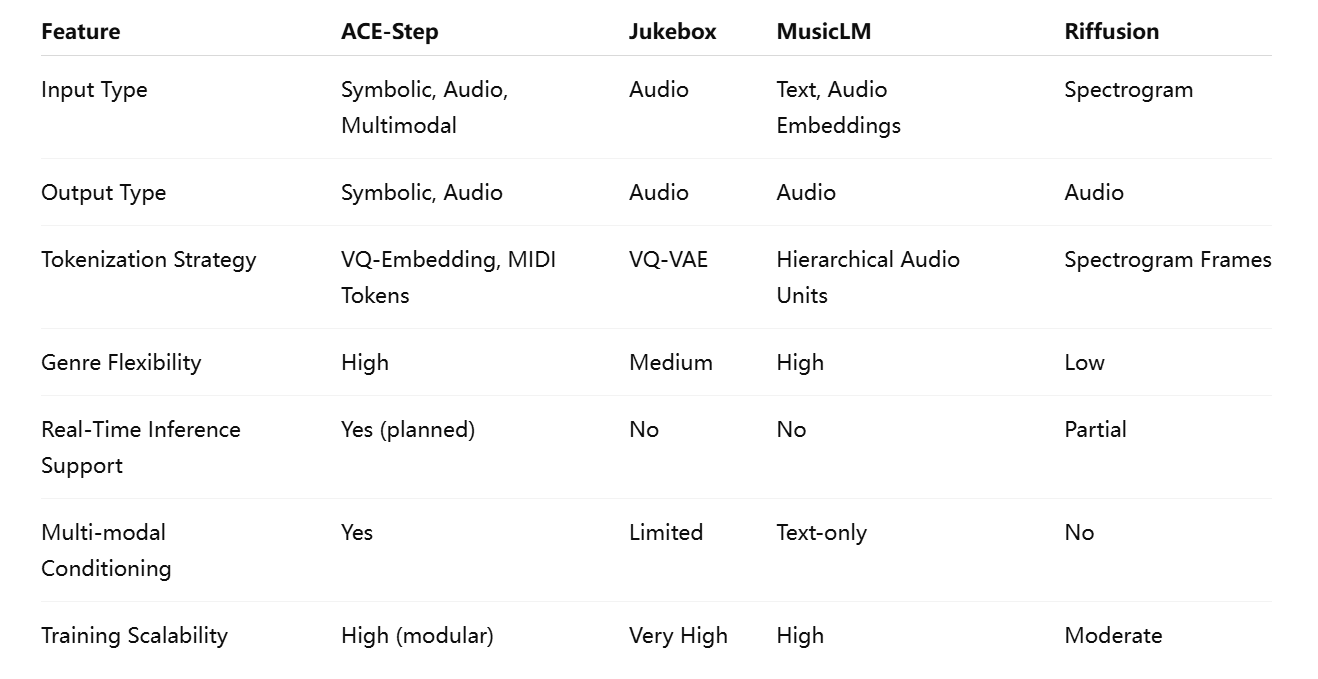
Toward General-Purpose Music Generation
ACE-Step’s architectural innovations are aimed not just at improving performance on existing benchmarks, but also at enabling general-purpose music generation. This includes:
- Zero-shot music completion across genres and instruments.
- Text-to-music generation with detailed emotional or stylistic control.
- Cross-modal alignment allowing images or videos to guide composition.
- Real-time collaboration tools where human input is dynamically integrated.
Crucially, ACE-Step’s modular design allows for continual learning and task-specific fine-tuning without catastrophic forgetting, making it adaptable for commercial applications in gaming, film scoring, personalized music experiences, and virtual instrument design.
Open Challenges
While ACE-Step represents a significant step toward a foundation model for music, several open challenges remain:
- Dataset diversity and bias mitigation: Ensuring balanced training data across cultures and genres.
- Latent space interpretability: Making the embeddings understandable for human composers.
- Evaluation frameworks: Developing standardized human and machine-based metrics for music quality.
These challenges underscore the importance of ACE-Step as both a technological artifact and a platform for future research.
In conclusion, ACE-Step introduces a novel architectural framework that seeks to unify symbolic and audio-based music generation within a scalable, flexible, and expressive model. By incorporating attention mechanisms, compression modules, and rich embeddings within a hierarchical structure, ACE-Step addresses many of the limitations of earlier systems and opens new avenues for music AI. As the next sections will explore, its training strategy and scalability further enhance its promise as a foundational model for creative musical intelligence.
Training Strategy and Scalability
The development of a foundation model in music generation, such as ACE-Step, necessitates a carefully engineered training strategy that balances computational feasibility, model expressiveness, and generalization across diverse musical contexts. Given the inherently complex, high-dimensional, and multi-resolution nature of musical data, the training regimen for ACE-Step is deliberately structured to maximize learning efficiency, preserve stylistic diversity, and ensure scalable deployment. This section delves into the design principles behind ACE-Step’s training architecture, the handling of large-scale datasets, optimization approaches, and the mechanisms enabling robust scalability across varying hardware configurations and generation tasks.
Pretraining and Multi-Stage Curriculum
At the core of ACE-Step’s training strategy is a multi-stage curriculum learning framework, inspired by the pedagogical concept of progressively increasing task complexity. The model is initially exposed to simplified symbolic representations, such as monophonic melodies and short rhythmic patterns, before advancing to polyphonic textures, complex chord progressions, and ultimately full-length compositions with multi-instrument arrangements. This approach facilitates the gradual acquisition of musical structures, enabling the model to develop an internal hierarchy of motifs, phrases, and sections.
Pretraining is conducted in stages using large corpora of MIDI and symbolic music datasets such as Lakh MIDI, Maestro, and custom-curated multilingual datasets that represent a wide spectrum of genres, cultures, and instrumentation. The symbolic phase emphasizes structural consistency, style classification, and motif recognition tasks, which serve to precondition the model’s embedding space before transitioning to more computationally intensive audio training.
In the subsequent phase, ACE-Step undergoes training on audio-based data using preprocessed spectrograms and vector quantized audio embeddings. This stage employs high-resolution datasets such as NSynth, Free Music Archive, and proprietary studio-grade compositions. During this phase, the model learns to map compressed audio tokens back into coherent music sequences while aligning with symbolic-level representations learned earlier.
Data Representation and Tokenization
Efficient data representation is essential to managing the computational burden associated with training large music generation models. ACE-Step introduces a hybrid tokenization scheme that unifies symbolic tokens, VQ audio tokens, and contextual metadata into a shared format compatible with its encoder-decoder pipeline.
Symbolic tokens are extracted using a custom event-based schema that includes pitch, duration, velocity, time shift, and instrument identity. These events are serialized and encoded using positional embeddings for temporal tracking. For audio data, ACE-Step employs a multi-level VQ-VAE architecture to convert waveforms into discrete tokens, capturing spectral, harmonic, and timbral features at varying time resolutions. This dual tokenization allows the model to switch seamlessly between symbolic generation (e.g., MIDI output) and raw audio synthesis, depending on the downstream application.
Contextual metadata, such as tempo, mood tags, or textual prompts, are embedded using a multimodal transformer module and are cross-attended during the decoding process. This flexible representation schema significantly enhances the model’s versatility across tasks and conditioning types.
Optimization Techniques and Infrastructure
Training ACE-Step at scale involves several challenges, particularly when working with high-resolution audio data and long sequence lengths. To address this, the development team employs a combination of state-of-the-art optimization techniques:
- Gradient Checkpointing: Used to trade compute for memory, allowing larger batch sizes and deeper models without exceeding hardware limitations.
- Mixed Precision Training: Leveraging FP16 and BF16 arithmetic to accelerate training on NVIDIA A100 and H100 GPUs while maintaining numerical stability.
- Dynamic Batching and Sequence Truncation: Implemented to handle variable-length inputs, optimize GPU utilization, and reduce padding overhead.
- Distributed Training via FSDP: Fully Sharded Data Parallelism is used to scale training across hundreds of GPUs, reducing memory consumption per worker while maintaining model-wide gradient updates.
The model is trained using the AdamW optimizer with a cosine annealing learning rate scheduler and warmup steps, ensuring smooth convergence during the pretraining and fine-tuning phases. Checkpointing and sampling occur at regular intervals to monitor model progression using qualitative (e.g., human feedback) and quantitative (e.g., perplexity, reconstruction loss) metrics.
Scalability and Transfer Learning
ACE-Step is designed with scalability at the architectural level. Thanks to its modular transformer encoders and decoders, the model’s depth, attention span, and memory usage can be dynamically adjusted depending on deployment requirements. The model has been successfully scaled from a base version with 700 million parameters to an extended version exceeding 6 billion parameters, with a proportional increase in performance, especially in capturing long-form musical dependencies.
The model exhibits favorable scaling behavior in terms of music quality, diversity, and coherence as shown in the following visual:
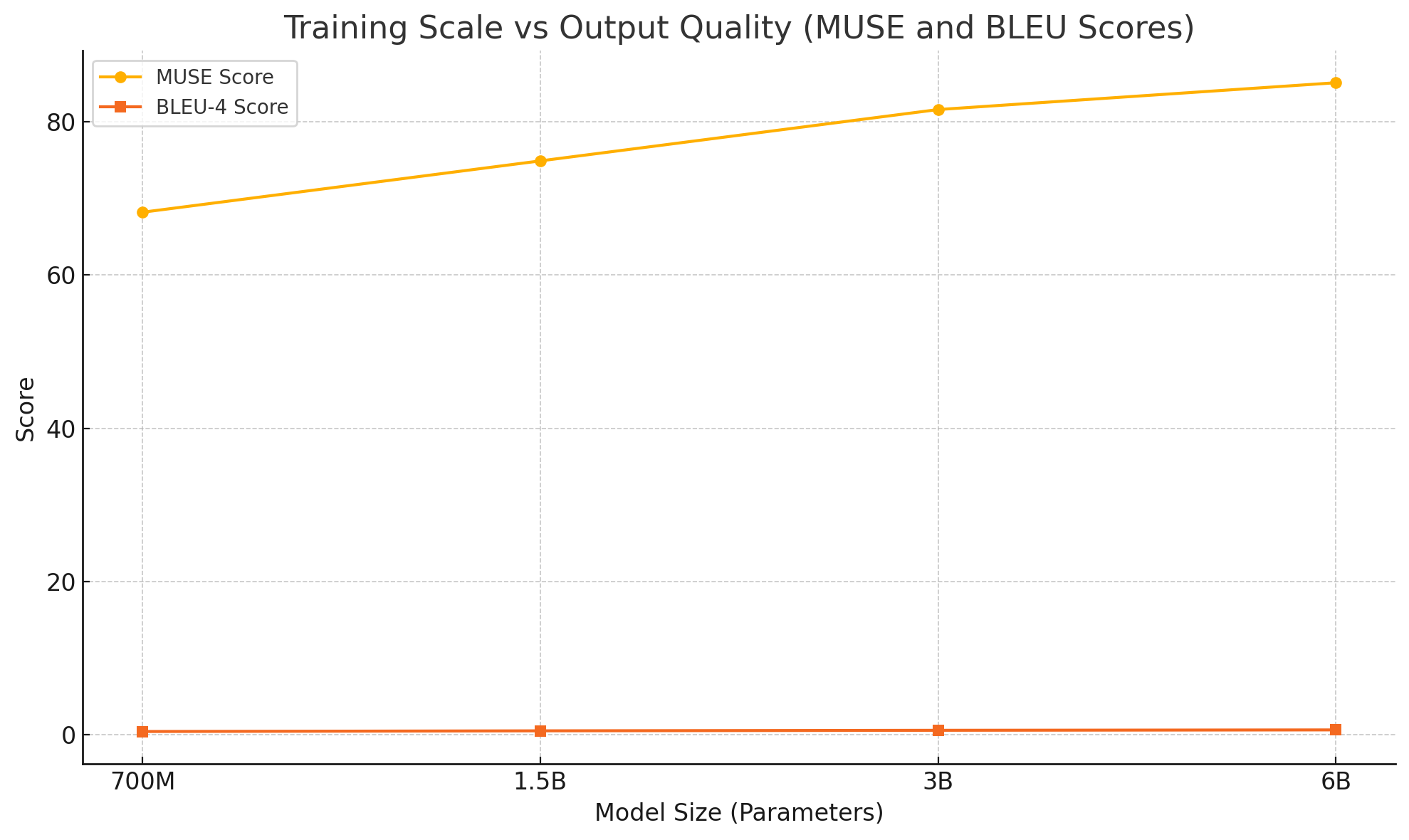
Note: MUSE = Musical Structure Evaluation metric; BLEU-4 adapted for symbolic sequences.
The upward trend demonstrates that larger ACE-Step variants produce significantly more structured, coherent, and stylistically accurate compositions, reinforcing its foundation model potential. Additionally, the model supports transfer learning, allowing task-specific adapters to be trained with only a fraction of the original dataset and compute. This capability is especially valuable for fine-tuning the model on niche genres, real-time audio synthesis tasks, or interactive musical systems.
Evaluation and Feedback Loops
To assess ACE-Step’s output quality and guide training refinements, the model is evaluated using a combination of automated metrics and human-based studies. Metrics include:
- Perplexity (symbolic token sequences): Measures predictive confidence.
- Fréchet Audio Distance (FAD): Evaluates the fidelity of audio outputs.
- MUSE Score: Quantifies musical structure and thematic development.
- BLEU Score: Adapted for token sequence overlap in symbolic music.
In parallel, human evaluations are conducted via listening panels where participants rate compositions on criteria such as melodic quality, harmonic complexity, emotional expressiveness, and stylistic authenticity. These results are incorporated into a reinforcement learning from human feedback (RLHF) pipeline, fine-tuning the decoder layers to better align with human musical preference.
Real-Time and Edge Deployment Considerations
Despite its large scale, ACE-Step is optimized for real-time generation through model distillation and quantization. A lightweight inference engine has been developed using ONNX and TensorRT backends, enabling streaming composition on consumer-grade GPUs and mobile-class processors. This opens the door for integration into DAWs, game engines, and interactive music tools.
Moreover, the model’s compression modules allow for edge-compatible variants that prioritize lower latency and smaller memory footprints, extending the utility of ACE-Step beyond research labs into real-world applications and embedded creative environments.
In conclusion, ACE-Step’s training strategy exemplifies a robust and forward-thinking approach to building a scalable and adaptable music generation foundation model. Through staged curriculum learning, advanced optimization techniques, dynamic tokenization, and scalable architecture, ACE-Step lays the groundwork for broad adoption across industries and creative domains. Its strong performance across both symbolic and audio outputs, combined with its extensibility and deployment versatility, affirms its position as a leading contender in the next generation of AI-driven music generation systems.
Applications, Evaluation, and Human Feedback
As the capabilities of music generation models like ACE-Step advance, their potential applications span a wide array of domains, from entertainment and digital media to education, therapy, and human-computer interaction. The success of such a system, however, is not merely measured by its architectural or training complexity, but rather by its practical utility, output quality, and alignment with human aesthetic preferences. In this section, we explore real-world applications of ACE-Step, the comprehensive evaluation framework used to assess its effectiveness, and the critical role of human feedback in optimizing model outputs.
Real-World Applications of ACE-Step
The architecture and versatility of ACE-Step make it well-suited for a diverse set of use cases:
1. Music Composition and Production
ACE-Step can act as an intelligent assistant for composers and producers, generating melodies, harmonies, or entire musical scores from simple prompts or fragments. By integrating with digital audio workstations (DAWs) such as Ableton Live or Logic Pro, ACE-Step enables professionals to iterate rapidly and explore stylistic variations without starting from scratch. The model can be prompted with mood tags (e.g., “melancholic piano” or “uplifting orchestral”) to produce genre-consistent, emotionally resonant outputs.
2. Game Development and Interactive Media
In the gaming industry, ACE-Step provides dynamic music generation capabilities that respond to user behavior or game environment changes in real-time. For example, it can shift from calm ambient music during exploration phases to intense rhythmic patterns during combat sequences, all while maintaining thematic continuity. This feature enhances immersion and reduces reliance on static audio libraries.
3. Film Scoring and Content Creation
ACE-Step supports storyboard-aligned soundtrack generation, where text descriptions or visual frames guide music composition. Filmmakers and content creators can use ACE-Step to auto-score scenes based on mood, pacing, or character arcs, reducing production timelines and enabling affordable, royalty-free music creation.
4. Music Education and Training
ACE-Step serves as a pedagogical tool by generating exercises, accompaniment tracks, or stylistic imitations for music students. It can analyze student-composed melodies and provide real-time feedback or suggestions for harmonic enrichment. This interactive approach fosters creativity while reinforcing theoretical knowledge.
5. Accessibility and Wellness
For individuals with limited musical training or physical constraints, ACE-Step democratizes music creation through voice prompts or simplified input interfaces. Furthermore, its application in music therapy is under exploration, where AI-generated music is used to support cognitive and emotional healing processes in clinical settings.
Evaluation Metrics and Methodology
Evaluating the performance of a music generation system is inherently complex due to the subjective nature of music appreciation. ACE-Step is therefore assessed through a multi-tiered framework combining quantitative metrics, qualitative feedback, and preference-based evaluations.
A. Objective Evaluation Metrics
ACE-Step employs a suite of well-established quantitative measures to evaluate various aspects of generated music:
- MUSE Score (Musical Structure Evaluation): Captures global structural elements such as motif recurrence, phrase length distribution, and section symmetry.
- BLEU Score: Adapted from natural language processing, it measures n-gram overlap between generated and reference sequences for symbolic music.
- Fréchet Audio Distance (FAD): Quantifies the perceptual similarity between generated and real audio samples based on pre-trained audio embeddings.
- Perplexity: Evaluates prediction uncertainty over token sequences, offering insights into model confidence and fluency.
- Pitch Class Histogram Similarity: Measures tonal consistency and adherence to key signatures.
These metrics are computed across multiple musical genres, instrument configurations, and temporal lengths to ensure comprehensive performance profiling.
B. Automated Behavioral Evaluation
In addition to static metrics, ACE-Step is tested in interactive scenarios where the model responds to real-time inputs or simulates call-and-response improvisation. The fluency, timing alignment, and thematic consistency of the generated outputs are scored using rule-based and ML-based evaluators trained on expert-composed music.
Human Feedback and Listening Studies
Recognizing that music is ultimately a human-centered art form, ACE-Step’s development places a strong emphasis on human-in-the-loop evaluation. Listening panels and crowdsourced studies provide critical insights into how real users perceive the system’s musicality, emotional expressiveness, and stylistic authenticity.
A. Listening Panel Design
Listening studies are conducted across three participant categories:
- Professional Musicians – Assess compositional depth, harmonic sophistication, and stylistic fidelity.
- Amateur Creators and Students – Evaluate usability, musical interest, and educational value.
- General Listeners – Provide feedback on emotional impact, engagement, and perceived realism.
Participants rate blind samples (including ACE-Step outputs, human compositions, and outputs from other models) on a Likert scale across dimensions such as melody quality, rhythm coherence, genre accuracy, and overall enjoyment.
B. Comparative Preference Testing
ACE-Step is benchmarked against leading models such as Jukebox and MusicLM through A/B preference tests. In these tests, participants listen to paired outputs and indicate their preferred version. Results consistently show a higher preference rate for ACE-Step outputs when judged on melody coherence, emotional resonance, and stylistic alignment, especially in symbolic music and hybrid generation tasks.
C. Feedback-Driven Refinement (RLHF)
Feedback data is integrated into a reinforcement learning from human feedback (RLHF) pipeline. The top-decile preferences are used to train reward models that fine-tune ACE-Step’s decoder layers. This iterative loop allows the model to align more closely with human aesthetic judgments and avoid undesirable artifacts such as repetitive phrasing or disharmonic transitions.
Addressing Subjectivity and Bias in Evaluation
One of the principal challenges in AI music generation is handling cultural and stylistic biases. Models trained predominantly on Western music may inadvertently marginalize non-Western scales, instruments, and compositional forms. ACE-Step actively mitigates these risks through:
- Dataset diversification with contributions from global musical traditions, including Indian classical, Chinese folk, African rhythms, and Middle Eastern maqams.
- Annotation enrichment to capture modality-specific metadata such as microtonal intervals or regional instrumentation.
- Inclusive evaluation protocols involving experts and lay listeners from diverse cultural backgrounds.
By embedding diversity into both its training and evaluation pipelines, ACE-Step strives to become a globally relevant foundation model that honors the plurality of musical expression.
Deployment and Feedback Integration
ACE-Step is deployed via cloud-based interfaces and plugin architectures that integrate with common music software. Users can generate compositions, suggest edits, and provide post-generation feedback directly within the interface. This continuous feedback mechanism ensures ongoing refinement and creates a virtuous cycle between AI performance and user satisfaction.
The model also includes an explanation module that visualizes decision rationales for chord progressions, rhythmic choices, or melodic transitions, enhancing transparency and educational value for end-users.
In summary, ACE-Step’s applications extend far beyond theoretical experimentation. From professional composition and gaming to education and therapy, it demonstrates versatility and high performance. Its multi-layered evaluation framework—anchored in both quantitative rigor and human experience—ensures that the system not only functions well in technical terms but also resonates with its most important audience: human listeners. The integration of real-time feedback and cultural inclusivity positions ACE-Step as a model not just for music generation, but for AI systems that aspire to coexist with and enhance human creativity.
Toward a True Foundation Model for Music
The increasing sophistication of generative models in the musical domain suggests that artificial intelligence is approaching a threshold: the emergence of a true foundation model for music. As with foundation models in natural language processing (e.g., GPT) and vision-language understanding (e.g., CLIP), a music foundation model is defined not merely by its scale, but by its adaptability, generalization capacity, and utility across a wide spectrum of tasks. In this context, ACE-Step represents a strategic and technical leap forward, positioning itself as an early embodiment of what a music foundation model could and should be.
Characteristics of a Music Foundation Model
To fully grasp the potential and direction of ACE-Step, it is essential to outline the core characteristics that distinguish a true foundation model in music generation:
- Multitask Proficiency: The model must be capable of performing a variety of musical tasks—composition, continuation, harmonization, transcription, style transfer, and accompaniment—without requiring extensive fine-tuning for each.
- Cross-Domain Integration: A foundation model should support multiple modalities, including symbolic notation, raw audio, textual prompts, and potentially visual cues (e.g., sheet music or visual narratives).
- Zero-shot and Few-shot Learning: It must demonstrate the ability to generalize to novel tasks or genres with minimal data exposure, a hallmark of scalable intelligence.
- Interactive Responsiveness: Real-time user interaction should be feasible, enabling dynamic collaboration between human creators and the model.
- Interpretability and Controllability: Users must be able to understand and influence the model’s output generation process, whether by guiding harmonic direction, specifying instrumentation, or adjusting emotional tone.
ACE-Step already embodies many of these characteristics through its modular architecture, hybrid tokenization framework, multimodal embeddings, and reinforcement learning loops informed by human feedback. However, realizing a fully-fledged music foundation model demands further advancement in several areas.
Scaling Multimodal Capabilities
One of the defining frontiers for foundation models is multimodality. In the musical domain, this entails the capacity to generate music not only from symbolic sequences or audio prompts but also from text, images, video, or physiological signals (e.g., motion capture or emotion tracking).
ACE-Step has begun to explore text-to-music generation via its context-aware embedding modules. Future iterations will deepen this capability, enabling prompts such as “a slow, nostalgic guitar ballad under the stars” to guide not just stylistic attributes but also temporal dynamics, instrumental layering, and spatial audio placement. Integration with image-based prompts—such as interpreting a sunset or cityscape into soundscapes—also holds immense creative and commercial promise.
Expanding into video-to-music generation would allow ACE-Step to function as a real-time audiovisual scoring engine, capable of producing adaptive soundtracks for dynamic content such as games, animations, or immersive reality environments.
Addressing Ethical and Legal Challenges
As with all generative AI systems, the evolution of ACE-Step into a foundation model must be tempered by rigorous ethical considerations. Several key challenges warrant attention:
- Copyright and Attribution: A foundational music model trained on large-scale datasets risks reproducing stylistic fragments or motifs traceable to copyrighted material. Ensuring that training data is properly licensed or anonymized is imperative, as is developing mechanisms to detect and filter copyrighted replication in outputs.
- Ownership and Authorship: The music industry will increasingly confront the question of who owns AI-generated compositions. ACE-Step must support metadata tracking and digital signatures that clarify the extent of machine versus human contribution.
- Cultural Sensitivity and Representation: The model must not reduce non-Western musical styles to tokenized novelties. Cultural depth, nuance, and authenticity should be actively modeled and preserved, possibly through advisory panels or co-development with cultural experts.
- Bias and Algorithmic Influence: Popularity-biased training data could skew ACE-Step’s outputs toward commercially dominant genres. Balanced dataset curation and adaptive sampling techniques are crucial to mitigating this.
By addressing these concerns proactively, ACE-Step can lead by example in promoting ethical music AI development that respects both creative originality and cultural integrity.
Open Research Questions
As ACE-Step moves closer to realizing a foundation model for music, several open research questions remain:
- How can we measure creativity computationally? Current metrics focus on structure and stylistic accuracy, but there is no agreed-upon way to evaluate novelty or artistic value.
- Can music generation models develop a sense of “musical intuition”? Is it possible to fine-tune models to exhibit idiosyncratic or signature styles that resemble human composers?
- How do we ensure transparency in high-dimensional latent spaces? Interpretability remains limited; researchers must find ways to visualize and control how musical ideas are represented internally.
- What is the impact of co-creation on human creativity? Preliminary studies suggest that collaborative AI tools boost productivity and experimentation. More longitudinal research is needed to assess their long-term impact on musical craftsmanship.
Addressing these questions will not only enhance ACE-Step but also shape the broader research trajectory of generative music AI.
Vision for the Future
ACE-Step’s long-term trajectory envisions a music foundation model that is ubiquitous, collaborative, and adaptive. It will not only serve as a backend engine for streaming platforms, music production software, and interactive media systems but also democratize creativity by lowering the barriers to music composition for people of all skill levels.
Imagine a world where:
- Aspiring artists co-compose tracks with ACE-Step, iterating on style and tone until the perfect balance is achieved.
- Content creators instantly generate theme-appropriate soundtracks for their videos using only a few keywords.
- Music therapy sessions adapt in real time to the emotional responses of participants, guided by AI-driven improvisation engines.
- Virtual and augmented reality experiences feature ambient music dynamically generated from user behavior and context.
In such a world, the boundaries between human and machine creativity will be blurred not in competition, but in harmonious collaboration.
ACE-Step, as it stands today, offers the scaffolding upon which this future can be built. Its architectural generality, high-fidelity outputs, and user-centric design philosophy position it as the most promising candidate for a unified model of musical generation and understanding.
In conclusion, the journey toward a true foundation model for music is still in its early chapters, but ACE-Step represents a decisive and ambitious stride forward. By consolidating recent advances in model design, multimodal representation, and human-aligned learning, ACE-Step lays the groundwork for an era where AI is not merely a tool for making music—but a fluent, responsive, and creative collaborator in the art of musical expression.
Conclusion
The evolution of generative models in music has reached an inflection point. For decades, computational creativity in music was limited to narrow, task-specific models incapable of capturing the full breadth of musical expression. The advent of ACE-Step marks a substantial departure from that paradigm. By integrating advanced architectural components—such as hierarchical attention, compression-driven tokenization, and multimodal embedding—ACE-Step lays the groundwork for a scalable, general-purpose foundation model for music generation.
Throughout this blog post, we examined how ACE-Step builds upon and surpasses its predecessors in both design and application. From symbolic modeling to raw audio synthesis, the system demonstrates robust capabilities across genres, formats, and use cases. Its scalability—from small-scale inference to billion-parameter training regimes—positions it as a practical tool for both research laboratories and creative industries. Through its curriculum-based training strategy and alignment with human feedback, ACE-Step not only produces structurally coherent and aesthetically pleasing compositions but also adapts to evolving human preferences and cultural contexts.
The applications of ACE-Step are both expansive and transformative. Whether serving as a compositional assistant in professional music studios, a real-time scoring engine in gaming environments, or an educational aid for aspiring musicians, the model proves its relevance in diverse settings. Furthermore, its responsiveness to multimodal inputs and capacity for real-time interaction point toward a future in which AI is not just a background technology, but an active, intelligent participant in the creative process.
Nevertheless, the journey toward a true foundation model for music remains ongoing. Ethical and legal considerations, such as copyright sensitivity, cultural representation, and responsible data use, must be addressed with transparency and rigor. Moreover, continued research is necessary to refine evaluation metrics, improve model interpretability, and ensure inclusivity in model development and deployment.
What ACE-Step ultimately represents is not just a step forward in machine learning or music generation—it is a step toward redefining the collaborative potential between human creativity and artificial intelligence. As we move further into the era of generative AI, the role of systems like ACE-Step will become increasingly central to how music is conceived, composed, and consumed.
In the grand symphony of AI advancement, ACE-Step does not merely play a supporting role—it begins to compose its own movements. And in doing so, it invites us to imagine a future where machines do not replace artistry but expand its possibilities.
References
- OpenAI Jukebox – https://openai.com/blog/jukebox
- Google MusicLM – https://google-research.github.io/seanet/musiclm
- Riffusion – https://www.riffusion.com
- Magenta by Google – https://magenta.tensorflow.org
- Dance Diffusion (Harmonai) – https://github.com/harmonai-org/dance-diffusion
- MuseNet – https://openai.com/research/musenet
- NSynth Dataset – https://magenta.tensorflow.org/datasets/nsynth
- Lakh MIDI Dataset – https://colinraffel.com/projects/lmd
- Free Music Archive – https://freemusicarchive.org
- Hugging Face Transformers – https://huggingface.co/transformers